Detection of peripheral artery disease from photoplethysmography signals using machine learning
This multidisciplinary digital health project will use the novel Symmetric Projection Attractor Reconstruction method applied to photoplethysmography signals to detect peripheral artery disease using machine/deep learning.
Start date
1 January 2023Duration
3 yearsApplication deadline
Funding source
UKRIFunding information
The Department of Mathematics has a number of fully-funded PhD studentships for UK nationals. These studentships will include tuition fees and a tax-free stipend. The Department has also a few scholarships for partial and full funding for overseas fees for exceptional applicants. However, funding for overseas students is limited and applicants are encouraged to find suitable funding themselves.
About
This multidisciplinary project is concerned with detecting peripheral artery disease (PAD) from a photoplethysmography (PPG) signal collected from a sensor on the foot. PAD is characterised by narrowing of the arteries that results in reduced blood flow to the limbs. It is common in people with diabetes and increases the risk of ulcers, amputation, and mortality. The mortality risk exceeds 70% within 5 years which is similar to many cancers. Costs of diabetic foot care in the UK are estimated to be ~£950m annually (~0.9% of NHS expenditure). However, 9/10 people with PAD are undetected. A simple method for earlier detection of PAD would enable life-changing early treatment for many patients.
The project will use the novel Symmetric Projection Attractor Reconstruction (SPAR) method to represent the signal as a two-dimensional bounded attractor that encapsulates the morphology and variability of the signal. Attractor features will be derived as input to machine learning and attractor images used as input to deep learning to address two fundamental questions: (i) does the patient have PAD? and (ii) if they do, what is the degree of severity of the disease? Interpretability methods will be used to explain the aspects of the features and the images and of the signal that are important for the predictions in order to give confidence in the results. Generalisability of the model will also be explored by testing it on other datasets collected at different hospitals and with different devices.
The student will be involved in the full life cycle of a digital health data-driven project. They will help with data collection at the Vascular Clinic of the East Surrey Hospital at the start of the project and they will then gain experience with signal processing and machine learning techniques in analysing the data that has been collected.
Supervisors
Supervisor 1: Prof Philip Aston (Department of Mathematics)
Supervisor 2: Prof Christian Heiss (Department of Clinical and Experimental Medicine)
Supervisor 3: Dr Jenny Venton (Data Science Department, NPL)
Supervisor 4: Dr Manasi Nandi (Faculty of Life Science and Medicine, KCL)
Supervisor 5: Mr Alexander Rodway (Surrey and Sussex Healthcare Trust).
Eligibility criteria
Applicants should have a minimum of a first class honours degree in mathematics, the physical sciences or engineering. Preferably applicants will hold an MMath, MPhys or MSc degree, though exceptional BSc students will be considered.
English language requirements
IELTS minimum 6.5 or above (or equivalent) with 6.0 in each individual category.
How to apply
Applications should be submitted via the Mathematics PhD Research programme page on the "Apply" tab. Please clearly state the studentship title and supervisor on your application.
Studentship FAQs
Read our studentship FAQs to find out more about applying and funding.
Application deadline
Contact details
Cesare Tronci
Email: maths-phd@surrey.ac.uk
Research
Research group
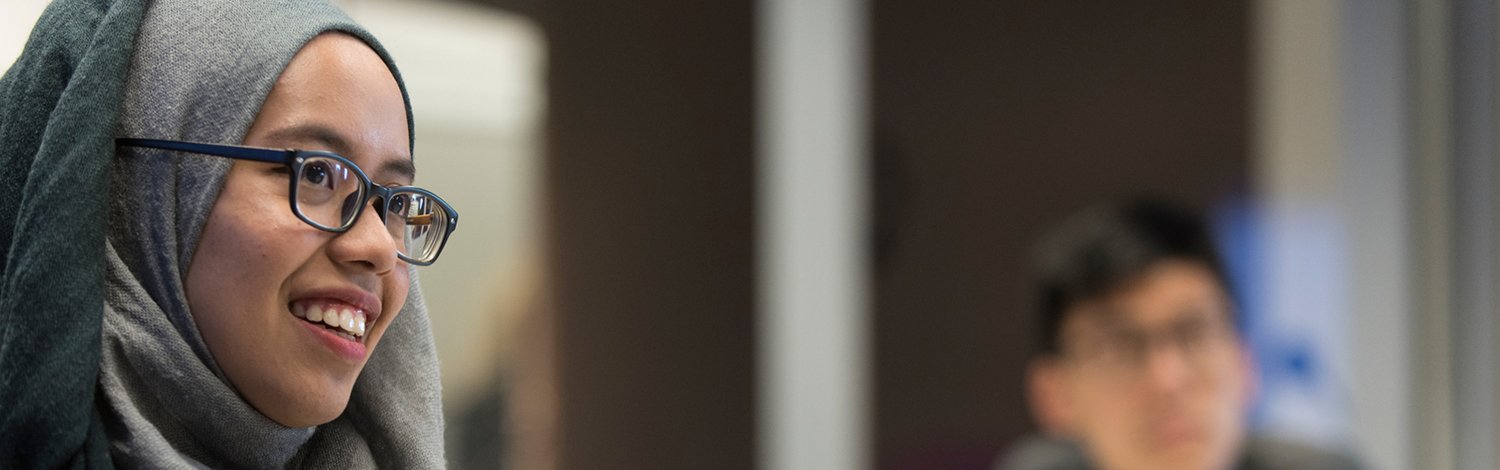
Studentships at Surrey
We have a wide range of studentship opportunities available.