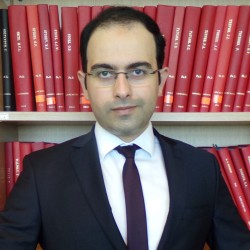
Dr Abdulrahman Kerim
Academic and research departments
Computer Science Research Centre, School of Computer Science and Electronic Engineering.About
Biography
Dr Kerim is a researcher and author in computer vision and machine learning. He worked on visual object tracking, semantic segmentation, and video stabilization problems. He is a fellow of the British Machine Vision Association (BMVA). He is currently interested in explainable machine learning (XML) and its applications in remote and satellite sensing.
Areas of specialism
My qualifications
ResearchResearch collaborations
Xiaowei Gu (Surrey University)
Washington L. S. Ramos (Google)
Leandro Soriano Marcolino (Lancaster University)
Erickson R. Nascimento (Microsoft)
Richard Jiang (Lancaster University)
Research collaborations
Xiaowei Gu (Surrey University)
Washington L. S. Ramos (Google)
Leandro Soriano Marcolino (Lancaster University)
Erickson R. Nascimento (Microsoft)
Richard Jiang (Lancaster University)
Publications
Stabilization plays a central role in improving the quality of videos. However, current methods perform poorly under adverse conditions. In this paper, we propose a synthetic-aware adverse weather video stabilization algorithm that dispenses real data for training, relying solely on synthetic data. Our approach leverages specially generated synthetic data to avoid the feature extraction issues faced by current methods. To achieve this, we present a novel data generator to produce the required training data with an automatic ground-truth extraction procedure. We also propose a new dataset, VSAC105Real, and compare our method to five recent video stabilization algorithms using two benchmarks. Our method generalizes well on real-world videos across all weather conditions and does not require large-scale synthetic training data. Implementations for our proposed video stabilization algorithm, generator, and datasets are available at https://github.com/A-Kerim/SyntheticData4VideoStabilization_WACV_2024.
Recent semantic segmentation models perform well under standard weather conditions and sufficient illumination but struggle with adverse weather conditions and nighttime. Collecting and annotating training data under these conditions is expensive, time-consuming, error-prone, and not always practical. Usually, synthetic data is used as a feasible data source to increase the amount of training data. However, just directly using synthetic data may actually harm the model’s performance under normal weather conditions while getting only small gains in adverse situations. Therefore, we present a novel architecture specifically designed for using synthetic training data for domain adaptation. We propose a simple yet powerful addition to DeepLabV3+ by using weather and time-of-the-day supervisors trained with multi-task learning, making it both weather and nighttime aware, which improves its mIoU accuracy by 14 percentage points on the ACDC dataset while maintaining a score of 75% mIoU on the Cityscapes dataset. Our code is available at https://github.com/lsmcolab/Semantic-Segmentation-under-Adverse-Conditions.
Description
The machine learning (ML) revolution has made our world unimaginable without its products and services. However, training ML models requires vast datasets, which entails a process plagued by high costs, errors, and privacy concerns associated with collecting and annotating real data. Synthetic data emerges as a promising solution to all these challenges. This book is designed to bridge theory and practice of using synthetic data, offering invaluable support for your ML journey. Synthetic Data for Machine Learning empowers you to tackle real data issues, enhance your ML models' performance, and gain a deep understanding of synthetic data generation. You’ll explore the strengths and weaknesses of various approaches, gaining practical knowledge with hands-on examples of modern methods, including Generative Adversarial Networks (GANs) and diffusion models. Additionally, you’ll uncover the secrets and best practices to harness the full potential of synthetic data. By the end of this book, you’ll have mastered synthetic data and positioned yourself as a market leader, ready for more advanced, cost-effective, and higher-quality data sources, setting you ahead of your peers in the next generation of ML.
What you will learn
- Understand real data problems, limitations, drawbacks, and pitfalls
- Harness the potential of synthetic data for data-hungry ML models
- Discover state-of-the-art synthetic data generation approaches and solutions
- Uncover synthetic data potential by working on diverse case studies
- Understand synthetic data challenges and emerging research topics
- Apply synthetic data to your ML projects successfully
Key benefits
- Avoid common data issues by identifying and solving them using synthetic data-based solutions
- Master synthetic data generation approaches to prepare for the future of machine learning
- Enhance performance, reduce budget, and stand out from competitors using synthetic data
- Purchase of the print or Kindle book includes a free PDF eBook
Predicting the success of a mobile game is a prime issue in game industry. Thousands of games are being released each day. However, a few of them succeed while the majority fail. Toward the goal of investigating the potential correlation between the success of a mobile game and its specific attributes, this work was conducted. More than 17 thousand games were considered for that reason. We show that IAPs (In-App Purchases), genre, number of supported languages, developer profile, and release month have a clear effect on the success of a mobile game. We also develop a novel success score reflecting multiple objectives. Furthermore, we show that game icons with certain visual characteristics tend to be associated with more rating counts. We employ different machine learning models to predict a novel success score metric of a mobile game given its attributes. The trained models were able to predict this score, as well as the expected rating average and rating count for a mobile game with 70% accuracy.
Traffic sign classification is a prime issue for autonomous platform industries such as autonomous cars. Towards the goal of recognition, most recent classification methods deploy Artificial Neural Networks (ANNs), Support Vector Machines (SVMs) and Convolutional Neural Networks (CNNs). In this work, we provide a novel dataset and a hybrid ANN that achieves accurate results that are very close to the state-of-the-art ones. When training and testing on German Traffic Sign Recognition Benchmarks (GTSRB) a top-5 classification accuracy of 80% was achieved for 43 classes. On the other hand, a top-2 classification accuracy of 95% was reached on our novel dataset for 10 classes. This accomplishment can be linked to the fact that the proposed hybrid ANN combines 9 different models trained on color intensity, HOG (Histograms of Oriented Gradients) and LBP (Local Binary Pattern) features.
Today, the cutting edge of computer vision research greatly depends on the availability of large datasets, which are critical for effectively training and testing new methods. Manually annotating visual data, however, is not only a labor-intensive process but also prone to errors. In this study, we present NOVA, a versatile framework to create realistic-looking 3D rendered worlds containing procedurally generated humans with rich pixel-level ground truth annotations. NOVA can simulate various environmental factors such as weather conditions or different times of day, and bring an exceptionally diverse set of humans to life, each having a distinct body shape, gender and age. To demonstrate NOVA's capabilities, we generate two synthetic datasets for person tracking. The first one includes 108 sequences, each with different levels of difficulty like tracking in crowded scenes or at nighttime and aims for testing the limits of current state-of-the-art trackers. A second dataset of 97 sequences with normal weather conditions is used to show how our synthetic sequences can be utilized to train and boost the performance of deep-learning based trackers. Our results indicate that the synthetic data generated by NOVA represents a good proxy of the real-world and can be exploited for computer vision tasks.
Robust visual tracking plays a vital role in many areas such as autonomous cars, surveillance and robotics. Recent trackers were shown to achieve adequate results under normal tracking scenarios with clear weather conditions, standard camera setups and lighting conditions. Yet, the performance of these trackers, whether they are correlation filter-based or learning-based, degrade under adverse weather conditions. The lack of videos with such weather conditions, in the available visual object tracking datasets, is the prime issue behind the low performance of the learning-based tracking algorithms. In this work, we provide a new person tracking dataset of real-world sequences (PTAW172Real) captured under foggy, rainy and snowy weather conditions to assess the performance of the current trackers. We also introduce a novel person tracking dataset of synthetic sequences (PTAW217Synth) procedurally generated by our NOVA framework spanning the same weather conditions in varying severity to mitigate the problem of data scarcity. Our experimental results demonstrate that the performances of the state-of-the-art deep trackers under adverse weather conditions can be boosted when the available real training sequences are complemented with our synthetically generated dataset during training.
Large-scale synthetic data is needed to support the deep learning big-bang that started in the recent decade and influenced almost all scientific fields. Most of the synthetic data generation solutions are task-specific or unscalable while the others are expensive, based on commercial games, or unreliable. In this work, a new rendering engine called Silver is presented in detail. Photo-realism, diversity, scalability, and full 3D virtual world generation at run-time are the key aspects of this work. The photo-realism was approached by utilizing the state-of-the-art High Definition Render Pipeline (HDRP) of the Unity game engine. In parallel, the Procedural Content Generation (PCG) concept was employed to create a full 3D virtual world at run-time, while the scalability of the system was attained by taking advantage of the modular approach followed as we built the system from scratch. Silver can be used to provide clean, unbiased, and large-scale training and testing data for various computer vision tasks.