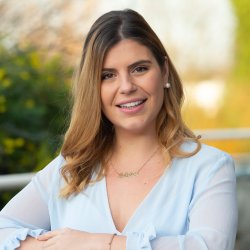
Dr Anthoulla Phella
About
University roles and responsibilities
- PhD. Students' Representative
My qualifications
ResearchResearch interests
Applied Econometrics
Econometric Theory
Empirical Macroeconomics
Research projects
This paper proposes a test for the joint hypothesis of correct dynamic specification and no omitted latent factors for the Quantile Autoregression. If the composite null is rejected we proceed to disentangle the cause of rejection, i.e., dynamic misspecification or an omitted variable. We establish the asymptotic distribution of the test statistics under fairly weak conditions and show that factor estimation error is negligible. A Monte Carlo study shows that the suggested tests have good finite sample properties. Finally, we undertake an empirical illustration of modelling growth and inflation in the United Kingdom, where we find evidence that factor augmented models are correctly specified in contrast with their non augmented counterparts when it comes to GDP growth, while also exploring the asymmetric behaviour of the growth and inflation distribution.
This paper considers forecasts of the growth and inflation distributions of the United Kingdom with factor-augmented quantile autoregressions under a model averaging framework. We investigate model combinations across models using weights that minimise the Akaike Information Criterion (AIC), the Bayesian Information Criterion (BIC), the Quantile Regression Information Criterion (QRIC) as well as the leave-one-out cross validation criterion. The unobserved factors are estimated by principal components of a large panel with N predictors over T periods under a recursive estimation scheme. We apply the aforementioned methods to the UK GDP growth and CPI inflation rate. We find that, on average, for GDP growth, in terms of coverage and final prediction error, the equal weights or the weights obtained by the AIC and BIC perform equally well but are outperformed by the QRIC and the Jackknife approach on the majority of the quantiles of interest. In contrast, the naive QAR(1) model of inflation outperforms all model averaging methodologies.
Research interests
Applied Econometrics
Econometric Theory
Empirical Macroeconomics
Research projects
This paper proposes a test for the joint hypothesis of correct dynamic specification and no omitted latent factors for the Quantile Autoregression. If the composite null is rejected we proceed to disentangle the cause of rejection, i.e., dynamic misspecification or an omitted variable. We establish the asymptotic distribution of the test statistics under fairly weak conditions and show that factor estimation error is negligible. A Monte Carlo study shows that the suggested tests have good finite sample properties. Finally, we undertake an empirical illustration of modelling growth and inflation in the United Kingdom, where we find evidence that factor augmented models are correctly specified in contrast with their non augmented counterparts when it comes to GDP growth, while also exploring the asymmetric behaviour of the growth and inflation distribution.
This paper considers forecasts of the growth and inflation distributions of the United Kingdom with factor-augmented quantile autoregressions under a model averaging framework. We investigate model combinations across models using weights that minimise the Akaike Information Criterion (AIC), the Bayesian Information Criterion (BIC), the Quantile Regression Information Criterion (QRIC) as well as the leave-one-out cross validation criterion. The unobserved factors are estimated by principal components of a large panel with N predictors over T periods under a recursive estimation scheme. We apply the aforementioned methods to the UK GDP growth and CPI inflation rate. We find that, on average, for GDP growth, in terms of coverage and final prediction error, the equal weights or the weights obtained by the AIC and BIC perform equally well but are outperformed by the QRIC and the Jackknife approach on the majority of the quantiles of interest. In contrast, the naive QAR(1) model of inflation outperforms all model averaging methodologies.
Teaching
Academic Year 2018/19/20
ECO3044: Derivatives Markets (Autumn Semester, UG)
ECO1020: Statistics For Economics (Spring Semester, UG)
EC210: Macroeconomic Principles (Autumn & Spring Semester, UG) at LSE
Previously
ECO3044: Derivatives Markets (2017/18, UG)