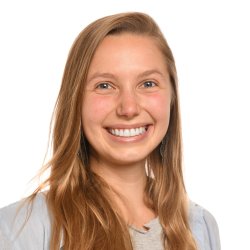
Dr Danielle Kurtin
About
My research project
Switch It Up: Characterizing, modelling, and optimizing the neural networks underpinning task switchingI am a PhD student studying neural network dynamics at the University of Surrey and Imperial College London. During the course of my PhD I plan to use multi-modal imaging techniques and mathematical modeling to determine optimal stimulation parameters. I will construct a mathematical model of the brain during a task switching paradigm using fMRI, high-density EEG, and DTI data to pinpoint optimal nodes for stimulation. Then, using stimulation, I hope to lower the switch cost in task-switching paradigms. This could improve task performance, and have translation to disorders characterized by pathological cognitive inflexibility (OCD, MDD, etc). This would not only result in further validation of the mechanisms determining tDCS's effects, but further expand the potential of this rapidly developing technique.
I am a PhD student studying neural network dynamics at the University of Surrey and Imperial College London. During the course of my PhD I plan to use multi-modal imaging techniques and mathematical modeling to determine optimal stimulation parameters. I will construct a mathematical model of the brain during a task switching paradigm using fMRI, high-density EEG, and DTI data to pinpoint optimal nodes for stimulation. Then, using stimulation, I hope to lower the switch cost in task-switching paradigms. This could improve task performance, and have translation to disorders characterized by pathological cognitive inflexibility (OCD, MDD, etc). This would not only result in further validation of the mechanisms determining tDCS's effects, but further expand the potential of this rapidly developing technique.
Publications
Transcranial direct current stimulation (tDCS) is a form of noninvasive brain stimulation whose potential as a cognitive therapy is hindered by our limited understanding of how participant and experimental factors influence its effects. Using functional MRI to study brain networks, we have previously shown in healthy controls that the physiological effects of tDCS are strongly influenced by brain state. We have additionally shown, in both healthy and traumatic brain injury (TBI) populations, that the behavioral effects of tDCS are positively correlated with white matter (WM) structure. In this study we investigate how these two factors, WM structure and brain state, interact to shape the effect of tDCS on brain network activity. We applied anodal, cathodal and sham tDCS to the right inferior frontal gyrus (rIFG) of healthy (n = 22) and TBI participants (n = 34). We used the Choice Reaction Task (CRT) performance to manipulate brain state during tDCS. We acquired simultaneous fMRI to assess activity of cognitive brain networks and used Fractional Anisotropy (FA) as a measure of WM structure. We find that the effects of tDCS on brain network activity in TBI participants are highly dependent on brain state, replicating findings from our previous healthy control study in a separate, patient cohort. We then show that WM structure further modulates the brain-state dependent effects of tDCS on brain network activity. These effects are not unidirectional - in the absence of task with anodal and cathodal tDCS, FA is positively correlated with brain activity in several regions of the default mode network. Conversely, with cathodal tDCS during CRT performance, FA is negatively correlated with brain activity in a salience network region. Our results show that experimental and participant factors interact to have unexpected effects on brain network activity, and that these effects are not fully predictable by studying the factors in isolation. •We replicated the brain state and polarity dependent effects of tDCS.•White matter structure influences tDCS's state-dependent changes in neural activity•The parameters of tDCS may operate under a hierarchy of influence.
The spread of diseases like severe acute respiratory syndrome coronavirus 2 (SARS-CoV-2) in human populations involve a large number of variables, making it difficult to predict how it will spread across communities and populations. Reduced representation simulations allow us to reduce the complexity of disease spread and model transmission based on a few key variables. Here we have created a Viral Transmission Education Simulator (VTES) that simulates the spread of disease through the interactions between circles representing individual people bouncing around a bounded, 2D plane. Infections are transmitted via person-to-person contact and the course of an outbreak can be tracked over time. Using this approach, we are able to simulate the influence of variables like infectivity, population density, and social distancing on the course of an outbreak. We also describe how VTES's code can be used to calculate R0 for the simulated pandemic. VTES is useful for modeling how small changes in variables that influence disease transmission can have large changes on the outcome of an epidemic. Additionally, VTES serves as an educational tool where users can easily visualize how disease spreads, and test how interventions, like masking, can influence an outbreak. VTES is designed to be simple and clear to encourage user modifications. These properties make VTES an educational tool that uses accessible, clear code and dynamic simulations to provide a richer understanding of the behaviors and factors underpinning a pandemic. VTES is available from: https://github.com/sstagg/disease-transmission.
Switching is a difficult cognitive process characterised by costs in task performance; specifically, slowed responses and reduced accuracy. It is associated with the recruitment of a large coalition of task-positive regions including those referred to as the multiple demand cortex (MDC). The neural correlates of switching not only include the MDC, but occasionally the default mode network (DMN), a characteristically task-negative network. To unpick the role of the DMN during switching we collected fMRI data from 24 participants playing a switching paradigm that perturbed predictability (i.e., cognitive load) across three switch dimensions—sequential, perceptual, and spatial predictability. We computed the activity maps unique to switch vs. stay trials and all switch dimensions, then evaluated functional connectivity under these switch conditions by computing the pairwise mutual information functional connectivity (miFC) between regional timeseries. Switch trials exhibited an expected cost in reaction time while sequential predictability produced a significant benefit to task accuracy. Our results showed that switch trials recruited a broader activity map than stay trials, including regions of the DMN, the MDC, and task-positive networks such as visual, somatomotor, dorsal, salience/ventral attention networks. More sequentially predictable trials recruited increased activity in the somatomotor and salience/ventral attention networks. Notably, changes in sequential and perceptual predictability, but not spatial predictability, had significant effects on miFC. Increases in perceptual predictability related to decreased miFC between control, visual, somatomotor, and DMN regions, whereas increases in sequential predictability increased miFC between regions in the same networks, as well as regions within ventral attention/ salience, dorsal attention, limbic, and temporal parietal networks. These results provide novel clues as to how DMN may contribute to executive task performance. Specifically, the improved task performance, unique activity, and increased miFC associated with increased sequential predictability suggest that the DMN may coordinate more strongly with the MDC to generate a temporal schema of upcoming task events, which may attenuate switching costs.
Transient patterns of interregional connectivity form and dissipate in response to varying cognitive demands. Yet, it is not clear how different cognitive demands influence brain state dynamics, and whether these dynamics relate to general cognitive ability. Here, using functional magnetic resonance imaging (fMRI) data, we characterised shared, recurrent, global brain states in 187 participants across the working memory, emotion, language, and relation tasks from the Human Connectome Project. Brain states were determined using Leading Eigenvector Dynamics Analysis (LEiDA). In addition to the LEiDA-based metrics of brain state lifetimes and probabilities, we also computed information-theoretic measures of Block Decomposition Method of complexity, Lempel-Ziv complexity and transition entropy. Information theoretic metrics are notable in their ability to compute relationships amongst sequences of states over time, compared to lifetime and probability, which capture the behaviour of each state in isolation. We then related task-based brain state metrics to fluid intelligence. We observed that brain states exhibited stable topology across a range of numbers of clusters (K = 2:15). Most metrics of brain state dynamics, including state lifetime, probability, and all information theoretic metrics, reliably differed between tasks. However, relationships between state dynamic metrics and cognitive abilities varied according to the task, the metric, and the value of K, indicating that there are contextual relationships between task-dependant state dynamics and trait cognitive ability. This study provides evidence that the brain reconfigures across time in response to cognitive demands, and that there are contextual, rather than generalisable, relationships amongst task, state dynamics, and cognitive ability.
•Computational models are useful for investigating the effects of brain stimulation•There are common construction choices when building generative brain models•We propose three phases to generate testable, model-informed stimulation targets Brain stimulation is an increasingly popular neuromodulatory tool used in both clinical and research settings; however, the effects of brain stimulation, particularly those of non-invasive stimulation, are variable. This variability can be partially explained by an incomplete mechanistic understanding, coupled with a combinatorial explosion of possible stimulation parameters. Computational models constitute a useful tool to explore the vast sea of stimulation parameters and characterise their effects on brain activity. Yet the utility of modelling stimulation in-silico relies on its biophysical relevance, which needs to account for the dynamics of large and diverse neural populations and how underlying networks shape those collective dynamics. The large number of parameters to consider when constructing a model is no less than those needed to consider when planning empirical studies. This piece is centred on the application of phenomenological and biophysical models in non-invasive brain stimulation. We first introduce common forms of brain stimulation and computational models, and provide typical construction choices made when building phenomenological and biophysical models. Through the lens of four case studies, we provide an account of the questions these models can address, commonalities, and limitations across studies. We conclude by proposing future directions to fully realise the potential of computational models of brain stimulation for the design of personalized, efficient, and effective stimulation strategies.
Abstract Deep brain stimulation (DBS) via implanted electrodes is used worldwide to treat patients with severe neurological and psychiatric disorders. However, its invasiveness precludes widespread clinical use and deployment in research. Temporal interference (TI) is a strategy for non-invasive steerable DBS using multiple kHz-range electric fields with a difference frequency within the range of neural activity. Here we report the validation of the non-invasive DBS concept in humans. We used electric field modeling and measurements in a human cadaver to verify that the locus of the transcranial TI stimulation can be steerably focused in the hippocampus with minimal exposure to the overlying cortex. We then used functional magnetic resonance imaging and behavioral experiments to show that TI stimulation can focally modulate hippocampal activity and enhance the accuracy of episodic memories in healthy humans. Our results demonstrate targeted, non-invasive electrical stimulation of deep structures in the human brain.