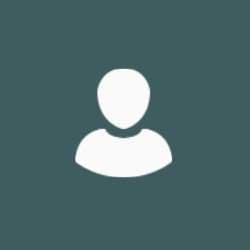
Dominic Ward
Research Software Developer
Publications
Ward Dominic, Wierstorf Hagen, Mason Russell, Plumbley Mark, Hummersone Christopher (2017) Estimating the loudness balance of musical mixtures using audio source separation,Proceedings of the 3rd Workshop on Intelligent Music Production (WIMP 2017)
To assist with the development of intelligent mixing systems,
it would be useful to be able to extract the loudness
balance of sources in an existing musical mixture. The
relative-to-mix loudness level of four instrument groups was
predicted using the sources extracted by 12 audio source
separation algorithms. The predictions were compared with
the ground truth loudness data of the original unmixed stems
obtained from a recent dataset involving 100 mixed songs.
It was found that the best source separation system could
predict the relative loudness of each instrument group with
an average root-mean-square error of 1.2 LU, with superior
performance obtained on vocals.
Wierstorf H, Ward D, Mason R, Girgis E, Hummersone C, Plumbley M (2017) Perceptual Evaluation of Source Separation for Remixing
Music,143rd AES Convention Paper No 9880 Audio Engineering Society
Music remixing is difficult when the original multitrack recording is not available. One solution is to estimate the elements of a mixture using source separation. However, existing techniques suffer from imperfect separation and perceptible artifacts on single separated sources. To investigate their influence on a remix, five state-of-the-art source separation algorithms were used to remix six songs by increasing the level of the vocals. A listening test was conducted to assess the remixes in terms of loudness balance and sound quality. The results show that some source separation algorithms are able to increase the level of the vocals by up to 6 dB at the cost of introducing a small but perceptible degradation in sound quality.
Ward Dominic, Wierstorf Hagen, Mason Russell, Grais Emad M., Plumbley Mark (2018) BSS eval or peass? Predicting the perception of singing-voice separation,Proceedings of the 2018 IEEE International Conference on Acoustics, Speech and Signal Processing (ICASSP)pp. 596-600 Institute of Electrical and Electronics Engineers (IEEE)
There is some uncertainty as to whether objective metrics for
predicting the perceived quality of audio source separation are
sufficiently accurate. This issue was investigated by employing
a revised experimental methodology to collect subjective
ratings of sound quality and interference of singing-voice
recordings that have been extracted from musical mixtures
using state-of-the-art audio source separation. A correlation
analysis between the experimental data and the measures of
two objective evaluation toolkits, BSS Eval and PEASS, was
performed to assess their performance. The artifacts-related
perceptual score of the PEASS toolkit had the strongest correlation
with the perception of artifacts and distortions caused
by singing-voice separation. Both the source-to-interference
ratio of BSS Eval and the interference-related perceptual
score of PEASS showed comparable correlations with the
human ratings of interference.
Grais Emad M, Wierstorf Hagen, Ward Dominic, Plumbley Mark D (2018) Multi-Resolution Fully Convolutional Neural Networks for Monaural Audio Source Separation,Proceedings of LVA/ICA 2018 (Lecture Notes in Computer Science)10891pp. 340-350 Springer Verlag
In deep neural networks with convolutional layers, all the
neurons in each layer typically have the same size receptive fields (RFs)
with the same resolution. Convolutional layers with neurons that have
large RF capture global information from the input features, while layers
with neurons that have small RF size capture local details with high
resolution from the input features. In this work, we introduce novel deep
multi-resolution fully convolutional neural networks (MR-FCN), where
each layer has a range of neurons with different RF sizes to extract multi-
resolution features that capture the global and local information from its
input features. The proposed MR-FCN is applied to separate the singing
voice from mixtures of music sources. Experimental results show that
using MR-FCN improves the performance compared to feedforward deep
neural networks (DNNs) and single resolution deep fully convolutional
neural networks (FCNs) on the audio source separation problem.
Grais Emad M, Ward Dominic, Plumbley Mark D (2018) Raw Multi-Channel Audio Source Separation using
Multi-Resolution Convolutional Auto-Encoders,Proceedings of the 2018 26th European Signal Processing Conference (EUSIPCO)pp. 1577-1581 Institute of Electrical and Electronics Engineers (IEEE)
Supervised multi-channel audio source separation
requires extracting useful spectral, temporal, and spatial features
from the mixed signals. The success of many existing systems is
therefore largely dependent on the choice of features used for
training. In this work, we introduce a novel multi-channel, multiresolution
convolutional auto-encoder neural network that works
on raw time-domain signals to determine appropriate multiresolution
features for separating the singing-voice from stereo
music. Our experimental results show that the proposed method
can achieve multi-channel audio source separation without the
need for hand-crafted features or any pre- or post-processing.
Ward, Dominic (2018) Latent Variable Analysis and Signal Separation: 14th International Conference, LVA/ICA 2018, Guildford, UK, July 2?5, 2018, Proceedings,10891 Springer International Publishing
This book constitutes the proceedings of the 14th International Conference on Latent Variable Analysis and Signal Separation, LVA/ICA 2018, held in Guildford, UK, in July 2018.The 52 full papers were carefully reviewed and selected from 62 initial submissions. As research topics the papers encompass a wide range of general mixtures of latent variables models but also theories and tools drawn from a great variety of disciplines such as structured tensor decompositions and applications; matrix and tensor factorizations; ICA methods; nonlinear mixtures; audio data and methods; signal separation evaluation campaign; deep learning and data-driven methods; advances in phase retrieval and applications; sparsity-related methods; and biomedical data and methods.
Ward Dominic, Mason Russell D., Kim Ryan Chungeun, Stöter Fabian-Robert, Liutkus Antoine, Plumbley Mark D. (2018) SISEC 2018: state of the art in musical audio source separation - Subjective selection of the best algorithm,Proceedings of the 4th Workshop on Intelligent Music Production, Huddersfield, UK, 14 September 2018 University of Huddersfield
The Signal Separation Evaluation Campaign (SiSEC) is a
large-scale regular event aimed at evaluating current progress
in source separation through a systematic and reproducible
comparison of the participants? algorithms, providing the
source separation community with an invaluable glimpse of
recent achievements and open challenges. This paper focuses
on the music separation task from SiSEC 2018, which
compares algorithms aimed at recovering instrument stems
from a stereo mix. In this context, we conducted a subjective
evaluation whereby 34 listeners picked which of six competing
algorithms, with high objective performance scores,
best separated the singing-voice stem from 13 professionally
mixed songs. The subjective results reveal strong differences
between the algorithms, and highlight the presence
of song-dependent performance for state-of-the-art systems.
Correlations between the subjective results and the scores of
two popular performance metrics are also presented.