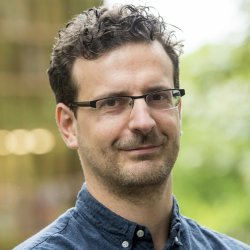
Dr Federico Martellosio
About
Biography
Federico Martellosio graduated in Management, Economics and Industrial Engineering from the Polytechnic of Milan in 1999. He completed his MSc degree in Economics at the University of Southampton in 2001, and received his PhD in Economics from the same institution in 2006. From 2004 to 2005 he was a teaching fellow at the University of Southampton. He then spent a year at the University of Amsterdam as a PostDoctoral Fellow. In 2006 he moved to the University of Reading as a Lecturer in Economics, and in 2012 he joined the School of Economics at Surrey.
Personal website: https://sites.google.com/site/federicomartellosio/
Research interests
Theoretical and applied econometrics, spatial econometrics, multivariate statistics.
Departmental duties
PhD Programme Director.
Teaching
ECOD022 Topics in Econometrics (PhD)
ECO2047 Introductory Econometrics (UG)
ECO1005 Mathematics for Economics (UG)
Publications
This paper explores the estimation of a panel data model with cross-sectional interaction that is flexible both in its approach to specifying the network of connections between cross-sectional units, and in controlling for unobserved heterogeneity. It is assumed that there are different sources of information available on a network, which can be represented in the form of multiple weights matrices. These matrices may reflect observed links, different measures of connectivity, groupings or other network structures, and the number of matrices may be increasing with sample size. A penalised quasi-maximum likelihood estimator is proposed which aims to alleviate the risk of network misspecification by shrinking the coefficients of irrelevant weights matrices to exactly zero. Moreover, controlling for unobserved factors in estimation provides a safeguard against the misspecification that might arise from unobserved heterogeneity. The asymptotic properties of the estimator are derived in a framework where the true value of each parameter remains fixed as the total number of parameters increases. A Monte Carlo simulation is used to assess finite sample performance, and in an empirical application the method is applied to study the prevalence of network spillovers in determining growth rates across countries.
Least squares estimation has casually been dismissed as an inconsistent estimation method for mixed regressive, spatial autoregressive models with or without spatial correlated disturbances. Although this statement is correct for a wide class of models, we show that, in economic spatial environments where each unit can be influenced aggregately by a significant portion of units in the population, least squares estimators can be consistent. Indeed, they can even be asymptotically efficient relative to some other estimators. Their computations are easier than alternative instrumental variables and maximum likelihood approaches.
This paper derives some exact power properties of tests for spatial autocorrelation in the context of a linear regression model. In particular, we characterize the circumstances in which the power vanishes as the autocorrelation increases, thus extending the work of Krämer (2005). More generally, the analysis in the paper sheds new light on how the power of tests for spatial autocorrelation is affected by the matrix of regressors and by the spatial structure. We mainly focus on the problem of residual spatial autocorrelation, in which case it is appropriate to restrict attention to the class of invariant tests, but we also consider the case when the autocorrelation is due to the presence of a spatially lagged dependent variable among the regressors. A numerical study aimed at assessing the practical relevance of the theoretical results is included. © Cambridge University Press, 2009.
We show that any invariant test for spatial autocorrelation in a spatial error or spatial lag model with equal weights matrix has power equal to size. This result holds under the assumption of an elliptical distribution. Under Gaussianity, we also show that any test whose power is larger than its size for at least one point in the parameter space must be biased. © Cambridge University Press 2011.
This paper investigates how the correlations implied by a first-order simultaneous autoregressive (SAR(1)) process are affected by the weights matrix W and the autocorrelation parameter . We provide an interpretation of the covariances between the random variables observed at two spatial units, based on a particular type of walks connecting the two units. The interpretation serves to explain a number of correlation properties of SAR(1) models, and clarifies why it is impossible to control the correlations through the specification of W.
The quasi-maximum likelihood estimator for the autoregressive parameter in a spatial autoregression usually cannot be written explicitly in terms of the data. A rigorous analysis of the first-order asymptotic properties of the estimator, under some assumptions on the evolution of the spatial design matrix, is available in Lee (2004), but very little is known about its exact or higher-order properties. In this paper we first show that the exact cumulative distribution function of the estimator can, under mild assumptions, be written in terms of that of a particular quadratic form. Simple examples are used to illustrate important exact properties of the estimator that follow from this representation. In general models a complete exact analysis is not possible, but a higher-order (saddlepoint) approximation is made available by the main result. We use this approximation to construct confidence intervals for the autoregressive parameter. Coverage properties of the proposed confidence intervals are studied by Monte Carlo simulation, and are found to be excellent in a variety of circumstances
The paper provides significant simplifications and extensions of results obtained by Gorsich, Genton, and Strang (J. Multivariate Anal. 80 (2002) 138) on the structure of spatial design matrices. These are the matrices implicitly defined by quadratic forms that arise naturally in modelling intrinsically stationary and isotropic spatial processes. We give concise structural formulae for these matrices, and simple generating functions for them. The generating functions provide formulae for the cumulants of the quadratic forms of interest when the process is Gaussian, second-order stationary and isotropic. We use these to study the statistical properties of the associated quadratic forms, in particular those of the classical variogram estimator, under several assumptions about the actual variogram.
The paper studies spatial autoregressive models with group interaction structure, focussing on estimation and inference for the spatial autoregressive parameter λ. The quasi-maximum likelihood estimator for λ usually cannot be written in closed form, but using an exact result obtained earlier by the authors for its distribution function, we are able to provide a complete analysis of the properties of the estimator, and exact inference that can be based on it, in models that are balanced. This is presented first for the so-called pure model, with no regression component, but is also extended to some special cases of the more general model. We then study the much more difficult case of unbalanced models, giving analogues of some, but by no means all, of the results obtained for the balanced case earlier. In both balanced and unbalanced models, results obtained for the pure model generalize immediately to the model with group-specific regression components.
The cumulants of quadratic forms associated to the so-called spatial design matrices are often needed for inference in the context of isotropic processes on uniform grids. Because the eigenvalues of the matrices involved are generally unknown, the computation of the cumulants can be very demanding if the grids are large. This paper first replaces the spatial design matrices with circular counterparts having known eigenvalues. It then studies some of the properties of the approximating matrices, and analyzes their performance in a number of applications to well-known inferential procedures. © 2011 Elsevier B.V.
The (quasi-) maximum likelihood estimator (QMLE) for the autoregres-sive parameter in a spatial autoregressive model cannot in general be written explicitly in terms of the data. The only known properties of the estimator have hitherto been its first-order asymptotic properties (Lee, 2004, Econometrica), derived under specific assumptions on the evolution of the spatial weights matrix involved. In this paper we show that the exact cumulative distribution function of the estimator can, under mild assumptions, be written in terms of that of a particular quadratic form. A number of immediate consequences of this result are discussed, and some examples are analyzed in detail. The examples are of interest in their own right, but also serve to illustrate some unexpected features of the distribution of the MLE. In particular, we show that the distribution of the MLE may not be supported on the entire parameter space, and may be nonanalytic at some points in its support.
The paper studies spatial autoregressive models with group interaction structure, focussing on estimation and inference for the spatial autoregressive parameter λ. The quasi-maximum likelihood estimator for λ usually cannot be written in closed form, but using an exact result obtained earlier by the authors for its distribution function, we are able to provide a complete analysis of the properties of the estimator, and exact inference that can be based on it, in models that are balanced. This is presented first for the so-called pure model, with no regression component, but is also extended to some special cases of the more general model. We then study the much more difficult case of unbalanced models, giving analogues of some, but by no means all, of the results obtained for the balanced case earlier. In both balanced and unbalanced models, results obtained for the pure model generalize immediately to the model with group-specific regression components.
One simple, and often very effective, way to attenuate the impact of nuisance parameters on maximum likelihood estimation of a parameter of interest is to recenter the profile score for that parameter. We apply this general principle to the quasi-maximum likelihood estimator (QMLE) of the autoregressive parameter λ in a spatial autoregression. The resulting estimator for λ has better finite sample properties compared to the QMLE for λ, especially in the presence of a large number of covariates. It can also solve the incidental parameterproblem that arises, for example, in social interaction models with network fixed effects, or infspatial panel models with individual or time fixed effects. However, spatial autoregressions present specific challenges for this type of adjustment, because recentering the profile score may cause the adjusted estimate to be outside the usual parameter space for λ. Conditions for this to happen are given, and implications are discussed. For inference, we propose confidence intervals based on a Lugannani{Rice approximation to the distribution of the adjusted QMLE of λ. Based on our simulations, the coverage properties of these intervals are excellent even in models with a large number of covariates.
Previous results have indicated that the OLS estimator of the vector of regression coefficients can be nearly as efficient as the best linear unbiased estimator when the regression errors follow a spatial process with root in the vicinity of unity. Such results were derived under the assumption of a symmetric weights matrix, which simplifies the analysis considerably, but is very often not satisfied in applications. This paper provides nontrivial generalizations to the important case of nonsymmetric weights matrices. © 2011 Elsevier B.V.
The paper provides significant simplifications and extensions of results obtained by Gorsich, Genton, and Strang (J. Multivariate Anal. 80 (2002) 138) on the structure of spatial design matrices. These are the matrices implicitly defined by quadratic forms that arise naturally in modelling intrinsically stationary and isotropic spatial processes. We give concise structural formulae for these matrices, and simple generating functions for them. The generating functions provide formulae for the cumulants of the quadratic forms of interest when the process is Gaussian, second-order stationary and isotropic. We use these to study the statistical properties of the associated quadratic forms, in particular those of the classical variogram estimator, under several assumptions about the actual variogram. © 2004 Elsevier Inc. All rights reserved.
We show that for any sample size, any size of the test, and any weights matrix outside a small class of exceptions, there exists a positive measure set of regression spaces such that the power of the Cliff–Ord test vanishes as the autocorrelation increases in a spatial error model. This result extends to the tests that define the Gaussian power envelope of all invariant tests for residual spatial autocorrelation. In most cases, the regression spaces such that the problem occurs depend on the size of the test, but there also exist regression spaces such that the power vanishes regardless of the size. A characterization of such particularly hostile regression spaces is provided.