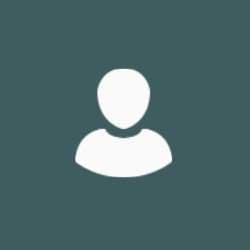
Publications
Study Objectives: Portable electroencephalography (EEG) devices offer the potential for accurate quantification of sleep at home but have not been evaluated in relevant populations. We assessed the Dreem headband (DHB) and its automated sleep staging algorithm in 62 older adults [age (mean±SD) 70.5±6.7 years; 12 Alzheimer’s]. Methods: The accuracy of sleep measures, epoch-by-epoch staging, and the quality of EEG signals for quantitative EEG (qEEG) analysis were compared to standard polysomnography (PSG) in a sleep laboratory. Results: The DHB algorithm accurately estimated total sleep time (TST) and sleep efficiency (SEFF) with a Symmetric Mean Absolute Percentage Error (SMAPE)
Background: Contactless sleep technologies (CSTs) hold promise for longitudinal, unobtrusive sleep monitoring in the community and at scale. They may be particularly useful in older populations wherein sleep disturbance, which may be indicative of the deterioration of physical and mental health, is highly prevalent. However, few CSTs have been evaluated in older people. Objective: This study evaluated the performance of 3 CSTs compared to polysomnography (PSG) and actigraphy in an older population. Methods: Overall, 35 older men and women (age: mean 70.8, SD 4.9 y; women: n=14, 40%), several of whom had comorbidities, including sleep apnea, participated in the study. Sleep was recorded simultaneously using a bedside radar (Somnofy [Vital Things]: n=17), 2 undermattress devices (Withings sleep analyzer [WSA; Withings Inc]: n=35; Emfit-QS [Emfit; Emfit Ltd]: n=17), PSG (n=35), and actigraphy (Actiwatch Spectrum [Philips Respironics]: n=18) during the first night in a 10-hour time-in-bed protocol conducted in a sleep laboratory. The devices were evaluated through performance metrics for summary measures and epoch-by-epoch classification. PSG served as the gold standard. Results: The protocol induced mild sleep disturbance with a mean sleep efficiency (SEFF) of 70.9% (SD 10.4%; range 52.27%-92.60%). All 3 CSTs overestimated the total sleep time (TST; bias: >90 min) and SEFF (bias: >13%) and underestimated wake after sleep onset (bias: >50 min). Sleep onset latency was accurately detected by the bedside radar (bias: 16 min). CSTs did not perform as well as actigraphy in estimating the all-night sleep summary measures. In an epoch-by-epoch concordance analysis, the bedside radar performed better in discriminating sleep versus wake (Matthew correlation coefficient [MCC]: mean 0.63, SD 0.12, 95% CI 0.57-0.69) than the undermattress devices (MCC of WSA: mean 0.41, SD 0.15, 95% CI 0.36-0.46; MCC of Emfit: mean 0.35, SD 0.16, 95% CI 0.26-0.43). The accuracy of identifying rapid eye movement and light sleep was poor across all CSTs, whereas deep sleep (ie, slow wave sleep) was predicted with moderate accuracy (MCC: >0.45) by both Somnofy and WSA. The deep sleep duration estimates of Somnofy correlated (r2=0.60; P
Introduction Longitudinal monitoring of vital signs provides a method for identifying changes to general health in an individual and particularly so in older adults. The nocturnal sleep period provides a convenient opportunity to assess vital signs. Contactless technologies that can be embedded into the bedroom environment are unintrusive and burdenless and have the potential to enable seamless monitoring of vital signs. To realise this potential, these technologies need to be evaluated against gold standard measures and in relevant populations. Methods We evaluated the accuracy of heart rate and breathing rate measurements of three contactless technologies (two under-mattress trackers: Withings sleep analyser (WSA) and Emfit QS (Emfit) and a bedside radar: Somnofy) in a sleep laboratory environment and assessed their potential to capture vital signs (heart rate and breathing rate) in a real-world setting. Data were collected in 35 community dwelling older adults aged between 65 and 83 years (mean ± SD: 70.8 ± 4.9; 21 men) during a one-night clinical polysomnography (PSG) in a sleep laboratory, preceded by 7 to 14 days of data collection at-home. Several of the participants had health conditions including type-2 diabetes, hypertension, obesity, and arthritis and ≈49% (n = 17) had moderate to severe sleep apnea while ≈29% (n = 10) had periodic leg movement disorder. The under-mattress trackers provided estimates of both heart rate and breathing rate while the bedside radar provided only breathing rate. The accuracy of the heart rate and breathing rate estimated by the devices was compared to PSG electrocardiogram (ECG) derived heart rate (beats per minute, bpm) and respiratory inductance plethysmography thorax (RIP thorax) derived breathing rate (cycles per minute, cpm). We also evaluated breathing disturbance indices of snoring and the apnea-hypopnea index (AHI) available from the WSA. Results All three contactless technologies provided acceptable accuracy in estimating heart rate [mean absolute error (MAE) < 2.2 bpm and mean absolute percentage error (MAPE) < 5%] and breathing rate (MAE ≤ 1.6 cpm and MAPE < 12%) at 1 minute resolution. All three contactless technologies were able to capture changes in heart rate and breathing rate across the sleep period. The WSA snoring and breathing disturbance estimates were also accurate compared to PSG estimates (R-squared: WSA Snore: 0.76, p < 0.001; WSA AHI: 0.59, p < 0.001). Conclusion Contactless technologies offer an unintrusive alternative to conventional wearable technologies for reliable monitoring of heart rate, breathing rate, and sleep apnea in community dwelling older adults at scale. They enable assessment of night-to-night variation in these vital signs, which may allow the identification of acute changes in health, and longitudinal monitoring which may provide insight into health trajectories.
Abstract Background Increased daytime napping and excessive sleepiness are associated with cognitive decline in older adults, especially in people living with dementia (PLWD) [1]. Subjective assessments of naps are burdensome and maybe unreliable in PLWD and hence there is a need for technologies that provide objective longitudinal assessment of the incidence and duration of naps. Here we compare two contactless sleep technologies (CSTs) against sleep diary and actigraphy for monitoring daytime napping in community dwelling non‐demented older adults. Method Two under‐mattress CSTs (Withings Sleep Analyser [WSA] and Emfit QS [Emfit]) along with actigraphy (Actiwatch Spectrum [AWS]) were deployed in the home of 17 older adults for a period of 14 days ( = 65 years; Mean Age ± SD = 72 ± 4.49; 6 Women). The ground truth nap information was collected using an extended consensus sleep diary that included additional information about naps (timing, duration, and location). We analyzed the agreement of the napping events and duration estimated by WSA, Emfit and AWS against the sleep diary reported events. Result The CSTs only detected in‐bed naps whilst the AWS detected both in‐bed and not‐in‐bed naps. Although all the compared devices detected spurious naps unreported in the sleep diary, it was highest in AWS (81% of total naps detected) followed by Emfit (63%) and WSA (16%) as shown in Figure 1. Among the CSTs, WSA accurately detected more in bed naps while registering less spurious naps compared to Emfit but had lower duration agreement to sleep diary (Figure 2). Further, when the contribution of daytime naps to 24‐h total sleep time was computed, the WSA estimate (12.8±6.1%) was closest to the sleep diary estimate (12.9±9.1%) followed by AWS (15.6±9.9%) and Emfit (17.2±11.1%). Conclusion CSTs, with their ability to provide both contextual location information and objective measures of napping, such as timing and duration, offer a reliable and unobtrusive alternative to traditional methods such as sleep diary and actigraphy for long‐term round‐the‐clock monitoring of sleep in older adults. References: [1] Li, P, Gao, L, Yu, L, et al. Daytime napping, and Alzheimer’s dementia: A potential bidirectional relationship. Alzheimer’s Dement. 2023; 19: 158– 168. https://doi.org/10.1002/alz.12636
Background The incidence of sleep disturbances increases with normal aging and is highly prevalent among people living with dementia (PLWD). To facilitate management and improvement of sleep quality in PLWD, validated unintrusive contactless technologies for long term objective monitoring of sleep are needed. Here we evaluate the ability of a contactless sleep tracker to accurately determine Time in Bed (TIB), Wake vs Sleep and Sleep stages (wake, light, deep, and REM sleep). Method We deployed the Emfit (Emfit QS), a contactless sleep tracker placed under the mattress. The Emfit uses ballistography to estimate respiration and heart rate and sleep stages. We collected data from 16 participants (Age: Mean‐72.12; SD‐4.6 years [6F:10M]) at home for a 14‐day period followed by a single overnight laboratory polysomnography (PSG) sleep assessment. The Emfit outputs a) timeseries at 30 s intervals (four sleep stages) and b) overnight summary sleep parameters. Sleep staging and sleep parameter estimation by Emfit was compared to, a) in‐lab gold standard PSG, and b) at‐home wristworn accelerometer (Actiwatch spectrum (AWS)) and sleep diary (SD) data. The epoch‐to‐epoch sleep staging concordance of Emfit was estimated over the total recording interval (∼10hrs) of the PSG for the laboratory session and between 1800hrs and 1200hrs for each SD entry for the home recordings. The concordance analysis for the sleep parameters, bed entry and exit times were performed using the summary data automatically generated by Emfit. Result The concordance between the four‐class sleep staging of the Emfit and PSG was poor (Figure 1). The two class (sleep/wake) analysis (Table 1) showed high sleep classification accuracy (sensitivity) but poor wake classification accuracy (specificity) compared to PSG. The sleep parameter estimates of Emfit also showed poor agreement with PSG (Figure 2). The home analysis indicated excellent accuracy for Time in Bed (TIB) (i.e., the bed entry and exit times) as registered by the SD (Table 2) and total sleep time (TST) for both sleep diary and AWS (Figure 3). Conclusion : The contactless sleep tracker provides accurate information about Time in Bed (TIB), but there is a lack of consensus of the sleep state classification with the PSG.
Background Sleep disturbance is common among people living with dementia as well as their caregivers. Non‐contact video technology can be used to characterise such disturbances as well as quantifying sleep quality by measuring the number of sleep body positions (poses). Such an approach may be beneficial for home‐based longitudinal clinical monitoring of sleep pattern changes and disturbances at all stages of dementia. Here we present our pilot results of a personalised data‐driven method applied to video data for quantification of sleep disturbance comparing older and younger participants. Method Data were collected in two separate studies which included an overnight 10‐12 hour laboratory sleep recording from thirteen older (65‐80 years, 9 male:4 female) and eleven younger (18‐34 years, 7 male:4 female) participants in a dedicated sleep facility. A data‐driven analysis using Principal Component Analysis and k‐means clustering was applied to infrared video data extracted from a clinical polysomnography (PSG) system. The data‐driven analysis automatically determined statistically significant groupings or clusters of unique body poses for each individual. Pose number, number of pose transitions, pose duration, and pose transition duration were computed for each participant. Result The number of data‐driven poses in older and younger participants was remarkably similar with 15.2±3.9 and 15.8±1.8 (mean±SD) poses per participant, respectively. However, the older group had a higher number of pose transitions (33.0±8.82) compared to the younger group (23.9±6.83) (p = 0.03). A significant 20% difference (p = 0.03) in the average duration of each body position was observed, with 62.3±7.1 minutes and 78.9±15.9 minutes for the older and younger groups, respectively (see Figure 1 and Figure 2). Pose transition duration was 19.2±7.25 seconds and 14.6±4.32 seconds for the older and younger groups, respectively where they were not significantly different. Conclusion Although the number of body positions did not vary significantly between the two cohorts, the older group changed body position more frequently and it took them longer to do so. Data‐driven automated analysis of video‐based sleep monitoring holds significant promise for quantifying age‐related and inter‐individual differences in sleep behavior.
Nocturnal disturbance is frequently observed in dementia and is a major contributor to institutionalisation. Unobtrusive technology that can quantify sleep/wake and determine bed occupancy during the major nocturnal sleep episode may be beneficial for long-term clinical monitoring and the carer. Such technologies have, however, not been validated in older people. Here we assessed the performance of the Withings Sleep Mattress (WSM) in a heterogenous older population to ensure external validity.
Wearable heart rate monitors offer a cost-effective way of non-invasive, long-term monitoring of cardiac health. Validation of wearable technologies in an older populations is essential for evaluating their effectiveness during deployment in healthcare settings. To this end, we evaluated the validity of heart rate measures from a wearable device, Empatica E4, and compared them to the electrocardiography (ECG). We collected E4 data simultaneously with ECG in thirty-five older men and women during an overnight sleep recording in the laboratory. We propose a robust approach to resolve the missing inter-beat interval (IBI) data and improve the quality of E4 derived measures. We also evaluated the concordance of heart rate (HR) and heart rate variability (HRV) measures with ECG. The results demonstrate that the automatic E4 heart rate measures capture long-term HRV whilst the short-term metrics are affected by missing IBIs. Our approach provides an effective way to resolve the missing IBI issue of E4 and extracts reliable heart rate measures that are concordant with ECG. Clinical Relevance— This work discusses data quality challenges in heart rate data acquired by wearables and provides an efficient and reliable approach for extracting heart rate measures from the E4 wearable device and validates the metrics in older adults
Quantification of sleep is important for the diagnosis of sleep disorders and sleep research. However, the only widely accepted method to obtain sleep staging is by visual analysis of polysomnography (PSG), which is expensive and time consuming. Here, we investigate automated sleep scoring based on a low‐cost, mobile electroencephalogram (EEG) platform consisting of a lightweight EEG amplifier combined with flex‐printed cEEGrid electrodes placed around the ear, which can be implemented as a fully self‐applicable sleep system. However, cEEGrid signals have different amplitude characteristics to normal scalp PSG signals, which might be challenging for visual scoring. Therefore, this study evaluates the potential of automatic scoring of cEEGrid signals using a machine learning classifier (“random forests”) and compares its performance with manual scoring of standard PSG. In addition, the automatic scoring of cEEGrid signals is compared with manual annotation of the cEEGrid recording and with simultaneous actigraphy. Acceptable recordings were obtained in 15 healthy volunteers (aged 35 ± 14.3 years) during an extended nocturnal sleep opportunity, which induced disrupted sleep with a large inter‐individual variation in sleep parameters. The results demonstrate that machine‐learning‐based scoring of around‐the‐ear EEG outperforms actigraphy with respect to sleep onset and total sleep time assessments. The automated scoring outperforms human scoring of cEEGrid by standard criteria. The accuracy of machine‐learning‐based automated scoring of cEEGrid sleep recordings compared with manual scoring of standard PSG was satisfactory. The findings show that cEEGrid recordings combined with machine‐learning‐based scoring holds promise for large‐scale sleep studies.
Sleep and its sub-states are assumed to be important for brain function across the lifespan but which aspects of sleep associate with various aspects of cognition, mood and self-reported sleep quality has not yet been established in detail. Sleep was quantified by polysomnography, quantitative Electroencephalogram (EEG) analysis and self-report in 206 healthy men and women, aged 20–84 years, without sleep complaints. Waking brain function was quantified by five assessments scheduled across the day covering objectively assessed performance across cognitive domains including sustained attention and arousal, decision and response time, motor and sequence control, working memory, and executive function as well as self-reports of alertness, mood and affect. Controlled for age and sex, self-reported sleep quality was negatively associated with number of awakenings and positively associated with the duration of Rapid Eye Movement (REM) sleep, but no significant associations with Slow Wave Sleep (SWS) measures were observed. Controlling only for age showed that associations between objective and subjective sleep quality were much stronger in women than in men. Analysis of 51 performance measures demonstrated that, after controlling for age and sex, fewer awakenings and more REM sleep were associated significantly with better performance on the Goal Neglect task, which is a test of executive function. Factor analysis of the individual performance measures identified four latent variables labeled Mood/Arousal, Response Time, Accuracy, and Visual Perceptual Sensitivity. Whereas Mood/Arousal improved with age, Response Times became slower, while Accuracy and Visual perceptual sensitivity showed little change with age. After controlling for sex and age, nominally significant association between sleep and factor scores were observed such that Response Times were faster with more SWS, and Accuracy was reduced where individuals woke more often or had less REM sleep. These data identify a positive contribution of SWS to processing speed and in particular highlight the importance of sleep continuity and REM sleep for subjective sleep quality and performance accuracy across the adult lifespan. These findings warrant further investigation of the contribution of sleep continuity and REM sleep to brain function.
Sleep and circadian rhythm disturbance are predictors of poor physical and mental health, including dementia. Long-term digital technology-enabled monitoring of sleep and circadian rhythms in the community has great potential for early diagnosis, monitoring of disease progression, and assessing the effectiveness of interventions. Before novel digital technology-based monitoring can be implemented at scale, its performance and acceptability need to be evaluated and compared to gold-standard methodology in relevant populations. Here, we describe our protocol for the evaluation of novel sleep and circadian technology which we have applied in cognitively intact older adults and are currently using in people living with dementia (PLWD). In this protocol, we test a range of technologies simultaneously at home (7-14 days) and subsequently in a clinical research facility in which gold standard methodology for assessing sleep and circadian physiology is implemented. We emphasize the importance of assessing both nocturnal and diurnal sleep (naps), valid markers of circadian physiology, and that evaluation of technology is best achieved in protocols in which sleep is mildly disturbed and in populations that are relevant to the intended use-case. We provide details on the design, implementation, challenges, and advantages of this protocol, along with examples of datasets.
[This corrects the article DOI: 10.2196/46338.].
Background Nocturnal disturbance is frequently observed in dementia and is a major contributor to institutionalisation. Unobtrusive technology that can quantify sleep/wake and determine bed occupancy during the major nocturnal sleep episode may be beneficial for long-term clinical monitoring and the carer. Such technologies have, however, not been validated in older people. Here we assessed the performance of the Withings Sleep Mattress (WSM) in a heterogenous older population to ensure external validity. Method Eighteen participants (65 – 80 years, 10M:8F) completed 7-12 days of sleep/wake monitoring at home prior to an overnight laboratory session. WSM performance was compared to gold-standard (laboratory polysomnography [PSG] with video) and silver standard (actiwatch [AWS] and sleep diary at home). WSM data were downloaded from a third party API and the minute-to-minute sleep/wake timeseries extracted and time-ordered to create a sleep profile. Discontinuities in the timeseries were labelled as ‘missing data’ events. Results Participants contributed 107 nights with WSM and PSG or AWS data. In the laboratory, the overall epoch to epoch agreement (accuracy) of sleep/wake detection of WSM compared to PSG was 0.71 (sensitivity 0.8; specificity 0.45) and to AWS was 0.74 (sensitivity 0.77; specificity 0.53). Visual inspection of video recordings demonstrated that 20 of 21 ‘missing data’ events were true ‘out of bed’ events. These events were always associated with an increase in activity (AWS). At home, all 97 WSM ‘missing data’ events that occurred within the major nocturnal sleep episode defined by sleep diary data, were associated with an increase in activity levels in the AWS data and 36 of these events were also associated with an increase in light levels, indicating that the participant had left the bed. In several participants, data recorded by the WSM during daytime coincided with reported naps in the sleep diary. Conclusion Although WSM cannot reliably distinguish between sleep and wake, the presence/absence of data in WSM seem to be an accurate representation of whether older people are in or out of bed (bed occupancy). Thus, in dementia, this contactless, low-burden technology may be able to provide information about nocturnal disturbances and daytime naps in bed.
Next-day residual effects of single evening doses of 3 mg of eszopiclone, 7.5 mg of zopiclone, and placebo were assessed in a randomized, double-blind, placebo-controlled, 3-way crossover study that used a mild sleep restriction protocol (sleep duration, 7 hours). During each period, 91 healthy volunteers spent 2 consecutive nights in the laboratory with time in bed restricted to 7 hours. Volunteers completed the Continuous Tracking Test, Critical Flicker Fusion task, Digit Symbol Substitution Test, N-back tasks, and Linear Analogue Rating Scales every half-hour from 7.5 to 11.5 hours after dose, commencing 15 minutes after awakening. Nighttime dosing of both eszopiclone (3 mg) and racemic zopiclone (7.5 mg) was associated with next-day performance impairment, and these residual effects dissipated over time. Eszopiclone did not differ from zopiclone on the primary end point, mean Continuous Tracking Test tracking error averaged from 7.5 to 9.5 hours after dose; however, a prespecified post hoc parametric analysis of reciprocal-transformed data favored eszopiclone over racemic zopiclone (P = 0.026). © 2012 Lippincott Williams & Wilkins.
Background Sleep disturbances are both risk factors for and symptoms of dementia. Current methods for assessing sleep disturbances are largely based on either polysomnography (PSG) which is costly and inconvenient, or self‐ or care‐giver reports which are prone to measurement error. Low‐cost methods to monitor sleep disturbances longitudinally and at scale can be useful for assessing symptom development. Here, we develop deep learning models that use multimodal variables (accelerometers and temperature) recorded by the AX3 to accurately identify sleep and wake epochs and derive sleep parameters. Method Eighteen men and women (65‐80y) participated in a sleep laboratory‐based study in which multiple devices for sleep monitoring were evaluated. PSGs were recorded over a 10‐h period and scored according to established criteria per 30 sec epochs. Tri‐axial accelerometers and temperature signals were captured with an Axivity AX3, at 100Hz and 1Hz, respectively, throughout a 19‐h period, including 10‐h concurrent PSG recording and 9‐h of wakefulness. We developed and evaluated a supervised deep learning algorithm to detect sleep and wake epochs and determine sleep parameters from the multimodal AX3 raw data. We validated our results with gold standard PSG measurements and compared our algorithm to the Biobank accelerometer analysis toolbox. Single modality (accelerometer or temperature) and multimodality (both signals) approaches were evaluated using the 3‐fold cross‐validation. Result The proposed deep learning model outperformed baseline models such as the Biobank accelerometer analysis toolbox and conventional machine learning classifiers (Random Forest and Support Vector Machine) by up to 25%. Using multimodal data improved sleep and wake classification performance (up to 18% higher) compared with the single modality. In terms of the sleep parameters, our approach boosted the accuracy of estimations by 11% on average compared to the Biobank accelerometer analysis toolbox. Conclusion In older adults without dementia, combining multimodal data from AX3 with deep learning methods allows satisfactory quantification of sleep and wakefulness. This approach holds promise for monitoring sleep behaviour and deriving accurate sleep parameters objectively and longitudinally from a low‐cost wearable sensor. A limitation of our current study is that the participants were healthy older adults: future work will focus on people living with dementia.
Electroencephalography (EEG) recordings represent a vital component of the assessment of sleep physiology, but the methodology presently used is costly, intrusive to participants, and laborious in application. There is a recognized need to develop more easily applicable yet reliable EEG systems that allow unobtrusive long-term recording of sleep-wake EEG ideally away from the laboratory setting. cEEGrid is a recently developed flex-printed around-the-ear electrode array, which holds great potential for sleep-wake monitoring research. It is comfortable to wear, simple to apply, and minimally intrusive during sleep. Moreover, it can be combined with a smartphone-controlled miniaturized amplifier and is fully portable. Evaluation of cEEGrid as a motion-tolerant device is ongoing, but initial findings clearly indicate that it is very well suited for cognitive research. The present study aimed to explore the suitability of cEEGrid for sleep research, by testing whether cEEGrid data affords the signal quality and characteristics necessary for sleep stage scoring. In an accredited sleep laboratory, sleep data from cEEGrid and a standard PSG system were acquired simultaneously. Twenty participants were recorded for one extended nocturnal sleep opportunity. Fifteen data sets were scored manually. Sleep parameters relating to sleep maintenance and sleep architecture were then extracted and statistically assessed for signal quality and concordance. The findings suggest that the cEEGrid system is a viable and robust recording tool to capture sleep and wake EEG. Further research is needed to fully determine the suitability of cEEGrid for basic and applied research as well as sleep medicine.