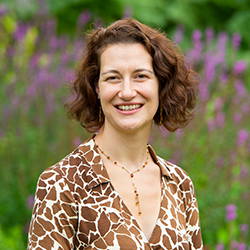
Dr Helen Cooper
About
Biography
I am the CVSSP Lab Facilities Manager, co-ordinating the lab and IT facilities within CVSSP and ensuring that the researchers have the equipment and tools they need to do their research. With my technical background combined with project management and event organisation experience I am well equipped to see that the academics and researchers in CVSSP are supported, so that they can continue to produce world leading research and train the next generation of engaged engineers and researchers. In addition to this role I also support CVSSP academics with project management and co-ordination on their larger research projects.
I came to Surrey to study Electronic Engineering with French at undergraduate level and stayed to do a PhD with Prof Richard Bowden in Sign Language Recognition. I worked as a researcher for 3 years on the EU funded project DictaSign before moving into a professional services and technical role within CVSSP. At first this role was supported by organising the leading UK conference in machine vision, BMVC, but the long term support for the role was to come from the management of research projects and lab management.
Affiliations and memberships
ResearchResearch projects
The aim of this Innovative Training Network is to train a new generation of creative, entrepreneurial and innovative early stage researchers (ESRs) in the research area of measurement and estimation of signals using knowledge or data about the underlying structure.
With its combination of ideas from machine learning and sensing, we refer to this research topic as “Machine Sensing”. We will train all ESRs in research skills needed to obtain an internationally-recognized PhD; to experience applying their research a non-Academic sector; and to gain transferable skills such as entrepreneurship and communication skills.
We will further encourage an open “reproducible research” approach to research, through open publication of research papers, data and software, and foster an entrepreneurial and innovation-oriented attitude through exposure to SME and spin-out Partners in the network. In the research we undertake, we will go beyond the current, and hugely popular, sparse representation and compressed sensing approaches, to develop new signal models and sensing paradigms. These will include those based on new structures, non-linear models, and physical models, while at the same time finding computationally efficient methods to perform this processing.
We will develop new robust and efficient Machine Sensing theory and algorithms, together methods for a wide range of signals, including: advanced brain imaging; inverse imaging problems; audio and music signals; and non-traditional signals such as signals on graphs. We will apply these methods to real-world problems, through work with non-Academic partners, and disseminate the results of this research to a wide range of academic and non-academic audiences, including through publications, data, software and public engagement events.
MacSeNet is funded under the H2020-MSCA-ITN-2014 call and is part of the Marie Sklodowska-Curie Actions — Innovative Training Networks (ITN) funding scheme
The SpaRTaN Initial Training Network will train a new generation of interdisciplinary researchers in sparse representations and compressed sensing, contributing to Europe’s leading role in scientific innovation.
By bringing together leading academic and industry groups with expertise in sparse representations, compressed sensing, machine learning and optimisation, and with an interest in applications such as hyperspectral imaging, audio signal processing and video analytics, this project will create an interdisciplinary, trans-national and inter-sectorial training network to enhance mobility and training of researchers in this area.
SpaRTaN is funded under the FP7-PEOPLE-2013-ITN call and is part of the Marie Curie Actions — Initial Training Networks (ITN) funding scheme: Project number - 607290
DICTA-SIGN was a three-year EU-funded research project that aimed to make online communications more accessible to deaf sign language users. My role was to research SLR technologies, a continuation of the work I did during my PhD.
The development of Web 2.0 technologies made the WWW a place where people constantly interact with each other, by posting information (e.g. blogs, discussion forums), modifying and enhancing other people's contributions (e.g. Wikipedia), and sharing information (e.g., Facebook, social news sites). Unfortunately, these technologies are not friendly to sign language users, because they require the use of written language. Can't sign language videos fulfill the same role as written text in these new technologies? In a word, no. Videos have two problems: Firstly, they are not anonymous – anyone making a contribution can be recognised from the video, which holds many people back who otherwise would be eager to contribute. Secondly, people cannot easily edit and add to a video that someone else has produced, so a Wikipedia-like web site in sign language is not possible. DICTA-SIGN's goal was to develop the necessary technologies to make Web 2.0 interactions in sign language possible: Users sign to a webcam using a dictation style. The computer recognises the signed phrases, converts them into an internal representation of sign language, and then has an animated avatar sign them back to the users. Content on the Web is then contributed and disseminated via the signing avatars. Moreover, the internal representation also allows us to develop sign language-to-sign language translation services, analogous to the Google translator. In this way, DICTA-SIGN aimed to solve both of the problems that sign language videos have. The avatar is anonymous, and its uniform signing style guarantees that contributions can be easily altered and expanded upon by any sign language user.
Research projects
The aim of this Innovative Training Network is to train a new generation of creative, entrepreneurial and innovative early stage researchers (ESRs) in the research area of measurement and estimation of signals using knowledge or data about the underlying structure.
With its combination of ideas from machine learning and sensing, we refer to this research topic as “Machine Sensing”. We will train all ESRs in research skills needed to obtain an internationally-recognized PhD; to experience applying their research a non-Academic sector; and to gain transferable skills such as entrepreneurship and communication skills.
We will further encourage an open “reproducible research” approach to research, through open publication of research papers, data and software, and foster an entrepreneurial and innovation-oriented attitude through exposure to SME and spin-out Partners in the network. In the research we undertake, we will go beyond the current, and hugely popular, sparse representation and compressed sensing approaches, to develop new signal models and sensing paradigms. These will include those based on new structures, non-linear models, and physical models, while at the same time finding computationally efficient methods to perform this processing.
We will develop new robust and efficient Machine Sensing theory and algorithms, together methods for a wide range of signals, including: advanced brain imaging; inverse imaging problems; audio and music signals; and non-traditional signals such as signals on graphs. We will apply these methods to real-world problems, through work with non-Academic partners, and disseminate the results of this research to a wide range of academic and non-academic audiences, including through publications, data, software and public engagement events.
MacSeNet is funded under the H2020-MSCA-ITN-2014 call and is part of the Marie Sklodowska-Curie Actions — Innovative Training Networks (ITN) funding scheme
The SpaRTaN Initial Training Network will train a new generation of interdisciplinary researchers in sparse representations and compressed sensing, contributing to Europe’s leading role in scientific innovation.
By bringing together leading academic and industry groups with expertise in sparse representations, compressed sensing, machine learning and optimisation, and with an interest in applications such as hyperspectral imaging, audio signal processing and video analytics, this project will create an interdisciplinary, trans-national and inter-sectorial training network to enhance mobility and training of researchers in this area.
SpaRTaN is funded under the FP7-PEOPLE-2013-ITN call and is part of the Marie Curie Actions — Initial Training Networks (ITN) funding scheme: Project number - 607290
DICTA-SIGN was a three-year EU-funded research project that aimed to make online communications more accessible to deaf sign language users. My role was to research SLR technologies, a continuation of the work I did during my PhD.
The development of Web 2.0 technologies made the WWW a place where people constantly interact with each other, by posting information (e.g. blogs, discussion forums), modifying and enhancing other people's contributions (e.g. Wikipedia), and sharing information (e.g., Facebook, social news sites). Unfortunately, these technologies are not friendly to sign language users, because they require the use of written language. Can't sign language videos fulfill the same role as written text in these new technologies? In a word, no. Videos have two problems: Firstly, they are not anonymous – anyone making a contribution can be recognised from the video, which holds many people back who otherwise would be eager to contribute. Secondly, people cannot easily edit and add to a video that someone else has produced, so a Wikipedia-like web site in sign language is not possible. DICTA-SIGN's goal was to develop the necessary technologies to make Web 2.0 interactions in sign language possible: Users sign to a webcam using a dictation style. The computer recognises the signed phrases, converts them into an internal representation of sign language, and then has an animated avatar sign them back to the users. Content on the Web is then contributed and disseminated via the signing avatars. Moreover, the internal representation also allows us to develop sign language-to-sign language translation services, analogous to the Google translator. In this way, DICTA-SIGN aimed to solve both of the problems that sign language videos have. The avatar is anonymous, and its uniform signing style guarantees that contributions can be easily altered and expanded upon by any sign language user.
Publications
This chapter covers the key aspects of sign-language recognition (SLR), starting with a brief introduction to the motivations and requirements, followed by a précis of sign linguistics and their impact on the field. The types of data available and the relative merits are explored allowing examination of the features which can be extracted. Classifying the manual aspects of sign (similar to gestures) is then discussed from a tracking and non-tracking viewpoint before summarising some of the approaches to the non-manual aspects of sign languages. Methods for combining the sign classification results into full SLR are given showing the progression towards speech recognition techniques and the further adaptations required for the sign specific case. Finally the current frontiers are discussed and the recent research presented. This covers the task of continuous sign recognition, the work towards true signer independence, how to effectively combine the different modalities of sign, making use of the current linguistic research and adapting to larger more noisy data sets
This paper presents a method to perform person independent sign recognition. This is achieved by implementing generalising features based on sign linguistics. These are combined using two methods. The first is traditional Markov models, which are shown to lack the required generalisation. The second is a discriminative approach called Sequential Pattern Boosting, which combines feature selection with learning. The resulting system is introduced as a dictionary application, allowing signers to query by performing a sign in front of a Kinect. Two data sets are used and results shown for both, with the query-return rate reaching 99.9% on a 20 sign multi-user dataset and 85.1% on a more challenging and realistic subject independent, 40 sign test set.
This paper introduces a fully-automated, unsupervised method to recognise sign from subtitles. It does this by using data mining to align correspondences in sections of videos. Based on head and hand tracking, a novel temporally constrained adaptation of apriori mining is used to extract similar regions of video, with the aid of a proposed contextual negative selection method. These regions are refined in the temporal domain to isolate the occurrences of similar signs in each example. The system is shown to automatically identify and segment signs from standard news broadcasts containing a variety of topics.
This paper proposes a method for sign language recognition that bypasses the need for tracking by classifying the motion directly. The method uses the natural extension of haar like features into the temporal domain, computed efficiently using an integral volume. These volumetric features are assembled into spatio-temporal classifiers using boosting. Results are presented for a fast feature extraction method and 2 different types of boosting. These configurations have been tested on a data set consisting of both seen and unseen signers performing 5 signs producing competitive results.
The availability of video format sign language corpora limited. This leads to a desire for techniques which do not rely on large, fully-labelled datasets. This paper covers various methods for learning sign either from small data sets or from those without ground truth labels. To avoid non-trivial tracking issues; sign detection is investigated using volumetric spatio-temporal features. Following this the advantages of recognising the component parts of sign rather than the signs themselves is demonstrated and finally the idea of using a weakly labelled data set is considered and results shown for work in this area.
We describe a prototype Search-by-Example or look-up tool for signs, based on a newly developed 1000-concept sign lexicon for four national sign languages (GSL, DGS, LSF,BSL), which includes a spoken language gloss, a HamNoSys description, and a video for each sign. The look-up tool combines an interactive sign recognition system, supported by Kinect technology, with a real-time sign synthesis system,using a virtual human signer, to present results to the user. The user performs a sign to the system and is presented with animations of signs recognised as similar. The user also has the option to view any of these signs performed in the other three sign languages. We describe the supporting technology and architecture for this system, and present some preliminary evaluation results.
This article presents a dictionary for Sign Language using visual sign recognition based on linguistic subcomponents. We demonstrate a system where the user makes a query, receiving in response a ranked selection of similar results. The approach uses concepts from linguistics to provide sign sub-unit features and classifiers based on motion, sign-location and handshape. These sub-units are combined using Markov Models for sign level recognition. Results are shown for a video dataset of 984 isolated signs performed by a native signer. Recognition rates reach 71.4% for the first candidate and 85.9% for retrieval within the top 10 ranked signs.
This paper presents an approach to large lexicon sign recog- nition that does not require tracking. This overcomes the issues of how to accurately track the hands through self occlusion in unconstrained video, instead opting to take a detection strategy, where patterns of motion are identi ed. It is demonstrated that detection can be achieved with only minor loss of accuracy compared to a perfectly tracked sequence using coloured gloves. The approach uses two levels of classi cation. In the rst, a set of viseme classi ers detects the presence of sub-Sign units of activity. The second level then assembles visemes into word level Sign using Markov chains. The system is able to cope with a large lexicon and is more expandable than traditional word level approaches. Using as few as 5 training examples the proposed system has classi cation rates as high as 74.3% on a randomly selected 164 sign vocabulary performing at a comparable level to other tracking based systems.
This paper discusses sign language recognition using linguistic sub-units. It presents three types of sub-units for consideration; those learnt from appearance data as well as those inferred from both 2D or 3D tracking data. These sub-units are then combined using a sign level classifier; here, two options are presented. The first uses Markov Models to encode the temporal changes between sub-units. The second makes use of Sequential Pattern Boosting to apply discriminative feature selection at the same time as encoding temporal information. This approach is more robust to noise and performs well in signer independent tests, improving results from the 54% achieved by the Markov Chains to 76%.
This work proposes to learn linguistically-derived sub-unit classifiers for sign language. The responses of these classifiers can be combined by Markov models, producing efficient sign-level recognition. Tracking is used to create vectors of hand positions per frame as inputs for sub-unit classifiers learnt using AdaBoost. Grid-like classifiers are built around specific elements of the tracking vector to model the placement of the hands. Comparative classifiers encode the positional relationship between the hands. Finally, binary-pattern classifiers are applied over the tracking vectors of multiple frames to describe the motion of the hands. Results for the sub-unit classifiers in isolation are presented, reaching averages over 90%. Using a simple Markov model to combine the sub-unit classifiers allows sign level classification giving an average of 63%, over a 164 sign lexicon, with no grammatical constraints.
This chapter discusses sign language recognition using linguistic sub-units. It presents three types of sub-units for consideration; those learnt from appearance data as well as those inferred from both 2D or 3D tracking data. These sub-units are then combined using a sign level classifier; here, two options are presented. The first uses Markov Models to encode the temporal changes between sub-units. The second makes use of Sequential Pattern Boosting to apply discriminative feature selection at the same time as encoding temporal information. This approach is more robust to noise and performs well in signer independent tests, improving results from the 54% achieved by the Markov Chains to 76%.
This paper introduces a fully-automated, unsupervised method to recognise sign from subtitles. It does this by using data mining to align correspondences in sections of videos. Based on head and hand tracking, a novel temporally constrained adaptation of apriori mining is used to extract similar regions of video, with the aid of a proposed contextual negative selection method. These regions are refined in the temporal domain to isolate the occurrences of similar signs in each example. The system is shown to automatically identify and segment signs from standard news broadcasts containing a variety of topics.
This paper presents a novel, discriminative, multi-class classifier based on Sequential Pattern Trees. It is efficient to learn, compared to other Sequential Pattern methods, and scalable for use with large classifier banks. For these reasons it is well suited to Sign Language Recognition. Using deterministic robust features based on hand trajectories, sign level classifiers are built from sub-units. Results are presented both on a large lexicon single signer data set and a multi-signer Kinect™ data set. In both cases it is shown to out perform the non-discriminative Markov model approach and be equivalent to previous, more costly, Sequential Pattern (SP) techniques.
We propose a novel hybrid approach to static pose estimation called Connected Poselets. This representation combines the best aspects of part-based and example-based estimation. First detecting poselets extracted from the training data; our method then applies a modified Random Decision Forest to identify Poselet activations. By combining keypoint predictions from poselet activitions within a graphical model, we can infer the marginal distribution over each keypoint without any kinematic constraints. Our approach is demonstrated on a new publicly available dataset with promising results.