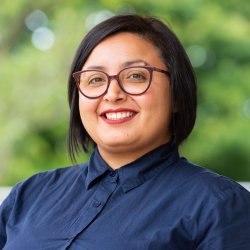
Ivelina Yonova
About
I am an experienced research programme manager with >9 years’ experience spanning the delivery of a £4m+ healthcare projects portfolio and a £3.5m Research England-funded programme.
In my current role as the FASS' Research and Innovation Manager, I lead on the set-up and overall management of the Faculty's Research and Innovation Office (FRIO). As part of this, I work very closely with researchers and professional services staff in different functional areas across the university to ensure that researchers receive co-ordinated and streamlined research support enabling them to focus on conducting their research and innovation activities in an integrated and effective manner.
Publications
Highlights
- de Lusignan S, Hoang U, Liyanage H, Yonova I, Ferreira F, Diez-Domingo J, Clark T. Feasibility of Point-of-Care Testing for Influenza Within a National Primary Care Sentinel Surveillance Network in England: Protocol for a Mixed Methods Study. JMIR Res Protoc. 2019 Nov 11;8(11):e14186. doi: 10.2196/14186. PubMed PMID: 31710303.
- Pebody RG, Whitaker H, Ellis J, Andrews N, Marques DFP, Cottrell S, Reynolds AJ, Gunson R, Thompson C, Galiano M, Lackenby A, Robertson C, O'Doherty MG, Owens K, Yonova I, Shepherd SJ, Moore C, Johnston J, Donati M, McMenamin J, Lusignan S, Zambon M. End of season influenza vaccine effectiveness in primary care in adults and children in the United Kingdom in 2018/19. Vaccine. 2019 Nov 1. pii: S0264-410X(19)31460-4. doi: 10.1016/j.vaccine.2019.10.071. PubMed PMID: 31685296.
- Hill EM, Petrou S, de Lusignan S, Yonova I, Keeling MJ. Seasonal influenza: Modelling approaches to capture immunity propagation. PLoS Comput Biol. 2019 Oct 28;15(10):e1007096. doi: 10.1371/journal.pcbi.1007096. eCollection 2019 Oct. PubMed PMID: 31658250
- Pebody R, Djennad A, Ellis J, Andrews N, Marques DFP, Cottrell S, Reynolds AJ, Gunson R, Galiano M, Hoschler K, Lackenby A, Robertson C, O'Doherty M, Sinnathamby M, Panagiotopoulos N, Yonova I, Webb R, Moore C, Donati M, Sartaj M, Shepherd SJ, McMenamin J, de Lusignan S, Zambon M. End of season influenza vaccine effectiveness in adults and children in the United Kingdom in 2017/18. Euro Surveill. 2019 Aug;24(31). doi: 10.2807/1560-7917.ES.2019.24.31.1800488. PubMed PMID: 31387673.
- de Lusignan S, Smith N, Livina V, Yonova I, Webb R, Thomas SA. Analysis of Primary Care Computerised Medical Records with Deep Learning. Stud Health Technol Inform. 2019; 258:249-250. PubMed PMID: 30942761.
- de Lusignan S, Borrow R, Tripathy M, Linley E, Zambon M, Hoschler K, Ferreira F, Andrews N, Yonova I, Hriskova M, Rafi I, Pebody R. Serological surveillance of influenza in an English sentinel network: pilot study protocol. BMJ Open. 2019 Mar 8;9(3):e024285. doi: 10.1136/bmjopen-2018-024285. PubMed PMID: 30852535; PubMed Central PMCID: PMC6429844.
- Kissling E, Rose A, Emborg HD, Gherasim A, Pebody R, Pozo F, Trebbien R, Mazagatos C, Whitaker H, Valenciano M; European Ive Group. Interim 2018/19 influenza vaccine effectiveness: six European studies, October 2018 to January 2019. Euro Surveill. 2019 Feb;24(8). doi: 10.2807/1560-7917.ES.2019.24.1900121. PubMed PMID: 30808440; PubMed Central PMCID: PMC6446950.
- de Lusignan S, Ferreira F, Damaso S, Byford R, Pathirannehelage S, Yeakey A, Yonova I, Schuind A, Dos Santos G. Enhanced passive surveillance of influenza vaccination in England, 2016-2017- an observational study using an adverse event reporting card. Hum Vaccin Immunother. 2019 Mar 20:1-12. doi: 10.1080/21645515.2019.1565258. [Epub ahead of print] PubMed PMID: 30648923.
- de Lusignan S, McGee C, Webb R, Joy M, Byford R, Yonova I, Hriskova M, Matos Ferreira F, Elliot AJ, Smith G, Rafi I. Conurbation, Urban, and Rural Living as Determinants of Allergies and Infectious Diseases: Royal College of General Practitioners Research and Surveillance Centre Annual Report 2016-2017. JMIR Public Health Surveill. 2018 Nov 26;4(4):e11354. doi: 10.2196/11354. PubMed PMID: 30478022; PubMed Central PMCID: PMC6288591.
- Pebody RG, Warburton F, Andrews N, Sinnathamby M, Yonova I, Reynolds A, Robertson C, Cottrell S, Sartaj M, Gunson R, Donati M, Moore C, Ellis J, de Lusignan S, McMenamin J, Zambon M. Uptake and effectiveness of influenza vaccine in those aged 65 years and older in the United Kingdom, influenza seasons 2010/11 to 2016/17. Euro Surveill. 2018 Sep;23(39). doi:10.2807/1560-7917.ES.2018.23.39.1800092. PubMed PMID: 30280688; PubMed Central PMCID: PMC6169201
- de Lusignan S, Dos Santos G, Byford R, Schuind A, Damaso S, Shende V, McGee C, Yonova I, Ferreira F. Enhanced Safety Surveillance of Seasonal Quadrivalent Influenza Vaccines in English Primary Care: Interim Analysis. Adv Ther. 2018 Jul 11. doi: 10.1007/s12325-018-0747-4. [Epub ahead of print] PubMed PMID: 29995300; PubMed Central PMCID: PMC6096981.
- Pebody RG, Sinnathamby MA, Warburton F, Andrews N, Boddington NL, Zhao H, Yonova I, Ellis J, Tessier E, Donati M, Elliot AJ, Hughes HE, Pathirannehelage S, Byford R, Smith GE, de Lusignan S, Zambon M. Uptake and impact of vaccinating primary school-age children against influenza: experiences of a live attenuated influenza vaccine programme, England, 2015/16. Euro Surveill. 2018 Jun;23(25). doi: 10.2807/1560-7917.ES.2018.23.25.1700496. PubMed PMID: 29945698.
- de Lusignan S, Correa A, Pebody R, Yonova I, Smith G, Byford R, Pathirannehelage SR, McGee C, Elliot AJ, Hriskova M, Ferreira FI, Rafi I, Jones S. Incidence of Lower Respiratory Tract Infections and Atopic Conditions in Boys and Young Male Adults: Royal College of General Practitioners Research and Surveillance Centre Annual Report 2015-2016. JMIR Public Health Surveill. 2018 Apr 30;4(2):e49. doi: 10.2196/publichealth.9307. PubMed PMID: 29712621.
- Pathirannehelage S, Kumarapeli P, Byford R, Yonova I, Ferreira F, de Lusignan S. Uptake of a dashboard designed to give realtime feedback to a sentinel network about key data required for influenza vaccine effectiveness studies. Accepted for Publication: Studies in Health Technology and Informatics, proceedings of MIE2018. 2018.
- Smith N, Livina V, Byford R, Ferreira F, Yonova I, de Lusignan S. Automated Differentiation of Incident and Prevalent Cases in Primary Care Computerised Medical Records (CMR). Stud Health Technol Inform. 2018;247:151-155. PubMed PMID: 29677941.
- Rondy M, Kissling E, Emborg HD, Gherasim A, Pebody R, Trebbien R, Pozo F, Larrauri A, McMenamin J, Valenciano M; I-MOVE/I-MOVE+ group. Interim 2017/18 influenza seasonal vaccine effectiveness: combined results from five European studies. Euro Surveill. 2018 Mar;23(9). doi: 10.2807/1560-7917.ES.2018.23.9.18-00086. PubMed PMID: 29510782; PubMed Central PMCID: PMC5840921
- Pebody R, Warburton F, Ellis J, Andrews N, Potts A, Cottrell S, Reynolds A, Gunson R, Thompson C, Galiano M, Robertson C, Gallagher N, Sinnathamby M, Yonova I, Correa A, Moore C, Sartaj M, de Lusignan S, McMenamin J, Zambon M. End-of-season influenza vaccine effectiveness in adults and children, United Kingdom, 2016/17. Euro Surveill. 2017 Nov;22(44). doi:10.2807/1560-7917.ES.2017.22.44.17-00306. PubMed PMID: 29113630.
- de Lusignan S, Correa A, Smith GE, Yonova I, Pebody R, Ferreira F, Elliot AJ, Fleming D. RCGP Research and Surveillance Centre: 50 years' surveillance of influenza, infections, and respiratory conditions. Br J Gen Pract. 2017 Oct;67(663):440-441. doi: 10.3399/bjgp17X692645. PubMed PMID: 28963401.
- de Lusignan S, Shinneman S, Yonova I, van Vlymen J, Elliot AJ, Bolton F, Smith GE, O'Brien S. An Ontology to Improve Transparency in Case Definition and Increase Case Finding of Infectious Intestinal Disease: Database Study in English General Practice. JMIR Med Inform. 2017 Sep 28;5(3):e34. doi: 10.2196/medinform.7641. PubMed PMID: 28958989.
- de Lusignan S, Dos Santos G, Correa A, Haguinet F, Yonova I, Lair F, Byford R, Ferreira F, Stuttard K, Chan T. Post-authorisation passive enhanced safety surveillance of seasonal influenza vaccines: protocol of a pilot study in England. BMJ Open. 2017 May 17;7(5):e015469. doi: 10.1136/bmjopen-2016-015469. PMID: 28515198
- de Lusignan S, Correa A, Pathirannehelage S, Byford R, Yonova I, Elliot AJ, Lamagni T, Amirthalingam G, Pebody R, Smith G, Jones S, Rafi I. RCGP Research and Surveillance Centre Annual Report 2014-2015: disparities in presentations to primary care. Br J Gen Pract. 2017 Jan;67(654):e29-e40. doi:10.3399/bjgp16X688573. Epub 2016 Dec 19. PubMed PMID: 27993900; PMCID: PMC5198624.
- R Pebody, F Warburton, J Ellis, N Andrews, A Potts, S Cottrell, J Johnston, A Reynolds, R Gunson, C Thompson, M Galiano, C Robertson, R Byford, N Gallagher, M Sinnathamby, I Yonova, S Pathirannehelage, M Donati, C Moore, S de Lusignan, J McMenamin, M Zambon. Effectiveness of seasonal influenza vaccine for adults and children in preventing laboratory-confirmed influenza in primary care in the United Kingdom: 2015/16 end-of-season results. Euro Surveill. 2016 Sep 22;21(38). doi: 10.2807/1560-7917.ES.2016.21.38.30348. PubMed PMID: 27684603; PubMed Central PMCID: PMC5073201.
- Correa A, Hinton W, McGovern A, van Vlymen J, Yonova I, Jones S, de Lusignan S. Royal College of General Practitioners Research and Surveillance Centre (RCGP RSC) sentinel network: a cohort profile. BMJ Open. 2016 Apr 20;6(4):e011092. doi: 10.1136/bmjopen-2016-011092.
- Pebody RG, Green HK, Andrews N, Boddington NL, Zhao H, Yonova I, Ellis J, Steinberger S, Donati M, Elliot AJ, Hughes HE, Pathirannehelage S, Mullett D, Smith GE, de Lusignan S, Zambon M. Uptake and impact of vaccinating school age children against influenza during a season with circulation of drifted influenza A and B strains, England, 2014/15. Euro Surveill. 2015 Oct 1;20(39). doi: 10.2807/1560-7917.ES.2015.20.39.30029.
- Pebody R1, Warburton F, Ellis J, Andrews N, Potts A, Cottrell S, Johnston J, Reynolds A, Gunson R, Thompson C, Galiano M, Robertson C, Mullett D, Gallagher N, Sinnathamby M, Yonova I, Moore C, McMenamin J, de Lusignan S, Zambon M. Effectiveness of seasonal influenza vaccine in preventing laboratory-confirmed influenza in primary care in the United Kingdom: 2015/16 mid-season results. Euro Surveill. 2016 Mar 31;21(13). doi: 10.2807/1560-7917.ES.2016.21.13.30179.
- Pebody R, Warburton F, Andrews N, Ellis J, von Wissmann B, Robertson C, Yonova I, Cottrell S, Gallagher N, Green H, Thompson C, Galiano M, Marques D, Gunson R, Reynolds A, Moore C, Mullett D, Pathirannehelage S, Donati M, Johnston J, de Lusignan S, McMenamin J, Zambon M. Effectiveness of seasonal influenza vaccine in preventing laboratory-confirmed influenza in primary care in the United Kingdom: 2014/15 end of season results. Euro Surveill. 2015 Sep 10;20(36). doi: 10.2807/1560-7917.ES.2015.20.36.30013.
I was also acknowledged in a paper published in the Lancet:
Williams R, Alexander G, Armstrong I, Baker A, Bhala N, Camps-Walsh G, CrampME, de Lusignan S, Day N, Dhawan A, Dillon J, Drummond C, Dyson J, Foster G, Gilmore I, Hudson M, Kelly D, Langford A, McDougall N, Meier P, Moriarty K,Newsome P, O'Grady J, Pryke R, Rolfe L, Rice P, Rutter H, Sheron N, Taylor A, Thompson J, Thorburn D, Verne J, Wass J, Yeoman A. Disease burden and costs from excess alcohol consumption, obesity, and viral hepatitis: fourth report of the Lancet Standing Commission on Liver Disease in the UK. Lancet. 2017 Nov 29. pii: S0140-6736(17)32866-0. doi: 10.1016/S0140-6736(17)32866-0. [Epub ahead of print] Review. Erratum in: Lancet. 2017 Dec 7: PubMed PMID: 29198562.
To pilot enhanced safety surveillance of seasonal influenza vaccine meeting the European Medicines Agency (EMA) requirement to rapidly detect a significant increase in the frequency or severity of adverse events of interest (AEIs), which may indicate risk from the new season?s vaccine.
Study designA prospective passive enhanced safety surveillance combining data collection from adverse drug reaction (ADR) cards with automated collection of pseudonymised routinely collected electronic health record (EHR) data. This study builds on a feasibility study carried out at the start of the 2015/2016 influenza season. We will report influenza vaccine exposure and any AEIs reported via ADR card or recorded directly into the EHR, from the commencement of influenza vaccination and ends as specified by EMA (30 November 2016).
SettingTen volunteer English general practices, primarily using the GSK influenza vaccines. They had selected this vaccine in advance of the study.
ParticipantsPeople who receive a seasonal influenza vaccine, in each age group defined in EMA interim guidance: 6 months to 5 years, 6?12 years, 13?17 years, 18?65 years and >65 years.
Outcome measuresThe primary outcome measure is the rate of AEIs occurring within 7 days postvaccination, using passive surveillance of general practitioner (GP) EHR systems enhanced by a card-based ADR reporting system. Extracted data will be presented overall by brand (Fluarix Tetra vs others), by age strata and risk groups. The secondary outcome measure is the vaccine uptake among the subjects registered in the enrolled general practices.
Additional publications
Posters:
- Galena Kuyumdzhieva, Nick Andrews, Gayatri Amirthalingam, Simon de Lusignan, Ivelina Yonova. (2019) “Evaluation of the impact of the Herpes Zoster vaccination programme in England five years following its introduction in September 2013”. Poster presented at: 5th ESCMID Conference on Vaccines. 2019 Sep 6-8, Bilbao, Spain
- Mary A. Sinnathamby, Fiona Warburton, Arlene Reynolds, Simon Cottrell, Mark O’Doherty, Lisa Domegan, Joan O’Donnell, Muhammad Sartaj, Jillian Johnston, Ivelina Yonova, Suzanne Elgohari, Nicola Boddington, Nick Andrews, Joanna Ellis, Simon de Lusignan, Jim McMenamin, Richard G. Pebody. (2019) “Impact of the introduction of the paediatric live attenuated influenza vaccine (LAIV) programme: an intercountry comparison across the United Kingdom and the Republic of Ireland”. Poster presented at internal Public Health England event, London, England
- De Lusignan, S., Correa, A., Pebody, R., Smith, G.E., Yonova, I., Byford, R., Pathirannehelage, S., Elliot, A.J., Andrews, N., Hriskova, M., Ferreira, F., Jones, S. (2017) “Higher incidence of respiratory disease and infections in boys and young male adults”. Poster presented at: Royal College of General Practitioners Annual Conference. 2017 Oct 12-14, Liverpool, England
- S de Lusignan, A Correa, I Yonova, R Pebody, R Byford, G Smith, F Ferreira, AJ Elliot (2017) “The Royal College of General Practitioners Research and Surveillance Centre”. Poster presented at: Royal College of General Practitioners Annual Conference. 2017 Oct 12-14, Liverpool, England
- de Lusignan, S., Correa, A., Yonova, I., Pebody, R., Byford, R., Smith, G.E., Ferreira, F., Elliot, A.J. (2017) “Evolution of the RCGP RSC network, a 50-year cohort profile”. Poster presented at: Public Health England Annual Conference. 2017 Sep 12-13; Coventry, England.
- Correa, A., Pebody, R., Andrews, N., Byford, R., Yonova, I., Ferreira, F., Warburton, F., de Lusignan, S. (2017) “Identifying adverse events following vaccination by brand”. Poster presented at: Public Health England Annual Conference. 2017 Sep 12-13; Coventry, England.
- N. Smith, V. Livina, S. de Lusignan, I. Yonova, F. Ferreira (2017) “Methodology for Correction of Miscoded Episode Types in Primary Care Computerised Medical Records”. Poster presented at: Big Data in Medicine - Tools, Transformation and Translation”. 2017 July 4; Cambridge, England.
- de Lusignan, S., Correa, A., Yonova, I., Byford, R. (2017) “Identifying brand-specific adverse events from routine data in England”. Poster presented at: Public Health England Annual Epidemiology Conference. 2017 Mar 21-22; Coventry, England.
- Correa, A., Yonova, I., Byford, R., O’Sullivan, S., de Lusignan, S. (2016) “Disparities in seasonal influenza vaccine uptake in primary care”. Poster presented at: Royal College of General Practitioners Annual Conference. 2016 Oct 6-8; Harrogate, England.
- Correa, A., Lamagni, T., Yonova, I., Pathirannehelage, S., O’Sullivan, S., de Lusignan, S. (2016) “Changing presentations of scarlet fever in general practice”. Poster presented at: Royal College of General Practitioners Annual Conference. 2016 Oct 6-8; Harrogate, England.
- Correa, A., Yonova, I., Byford, R., O’Sullivan, S., de Lusignan, S. (2016) “Making better use of real world data to assess population health”. Poster presented at: Royal College of General Practitioners Annual Conference. 2016 Oct 6-8; Harrogate, England.
- Mullett D., Burleigh D., Pathirannehelage S., Yonova I., Ferreira F., de Lusignan S. (2015) “7% of events in GP data are not recorded on the day of event”. Poster presented at: Royal College of General Practitioners Annual Conference. 2015 Oct 1-3; Glasgow, Scotland