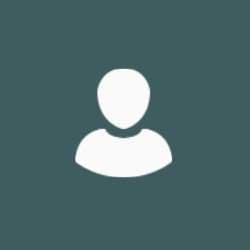
Dr Panagiotis Demis
About
My research project
Publications
The year 2020 has seen the emergence of a global pandemic as a result of the disease COVID-19. This report reviews knowledge of the transmission of COVID-19 indoors, examines the evidence for mitigating measures, and considers the implications for wintertime with a focus on ventilation.
Distributed Energy Systems (DES) are set to play a vital role in achieving emission targets and meeting higher global energy demand by 2050. However, implementing these systems has been challenging, particularly due to uncertainties in local energy demand and renewable energy generation, which imply uncertain operational costs. In this work we are implementing a Mixed-Integer Linear Programming (MILP) model for the operation of a DES, and analysing impacts of uncertainties in electricity demand, heating demand and solar irradiance on the main model output, the total daily operational cost, using Global Sensitivity Analysis (GSA). Representative data from a case study involving nine residential areas at the University of Surrey are used to test the model for the winter season. Distribution models for uncertain variables, obtained through statistical analysis of raw data, are presented. Design results show reduced costs and emissions, whilst GSA results show that heating demand has the largest influence on the variance of total daily operational cost. Challenges and design limitations are also discussed. Overall, the methodology can be easily applied to improve DES design and operation.
The design and operation of process systems are required to meet multiple constraints related to production schedules, product quality, safety, economic performance and environmental footprint. These constraints define the set of feasible design and/or operational parameters which is called process Design Space (DS). In most instances, process constraints are defined as functions of state variables of the system in which case the full-scale process model must be solved for their verification which can be a computationally demanding task for large-scale nonlinear models. This a challenge for online applications such as model-predictive control or real-time optimisation. In this study we present a computationally efficient method of evaluating the feasibility of a set of model parameters using a surrogate indicator function of the DS through Gaussian Process approximation of deterministic inequality model constraints. The method allows finding a compromise between the computational effort required and the level of confidence reflecting the accuracy of DS approximation.
The manufacture of protein-based therapeutics presents unique challenges due to limited control over the biotic phase. This typically gives rise to a wide range of protein structures of varying safety and in vivo efficacy. Herein we propose a computational methodology, enabled by the application of constrained Global Sensitivity Analysis, for efficiently exploring the operating range of process inputs in silico and identifying a design space that meets output constraints. The methodology was applied to an antibody- producing Chinese hamster ovary (CHO) cell culture system: we explored > 80 0 0 feeding strategies to identify a subset of manufacturing conditions that meet constraints on antibody titre and glycan distri- bution as an attribute of product quality. Our computational findings were then verified experimentally, confirming the applicability of this approach to a challenging production system. We envisage that this methodology can significantly expedite bioprocess development and increase operational flexibility.