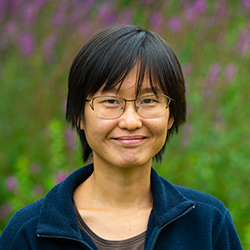
Dr Qingju Liu
Publications
Object-based audio is an emerging representation for audio content, where content is represented in a reproductionformat- agnostic way and thus produced once for consumption on many different kinds of devices. This affords new opportunities for immersive, personalized, and interactive listening experiences. This article introduces an end-to-end object-based spatial audio pipeline, from sound recording to listening. A high-level system architecture is proposed, which includes novel audiovisual interfaces to support object-based capture and listenertracked rendering, and incorporates a proposed component for objectification, i.e., recording content directly into an object-based form. Text-based and extensible metadata enable communication between the system components. An open architecture for object rendering is also proposed. The system’s capabilities are evaluated in two parts. First, listener-tracked reproduction of metadata automatically estimated from two moving talkers is evaluated using an objective binaural localization model. Second, object-based scene capture with audio extracted using blind source separation (to remix between two talkers) and beamforming (to remix a recording of a jazz group), is evaluated with perceptually-motivated objective and subjective experiments. These experiments demonstrate that the novel components of the system add capabilities beyond the state of the art. Finally, we discuss challenges and future perspectives for object-based audio workflows.
In this paper, we compare different deep neural networks (DNN) in extracting speech signals from competing speakers in room environments, including the conventional fullyconnected multilayer perception (MLP) network, convolutional neural network (CNN), recurrent neural network (RNN), and the recently proposed capsule network (CapsNet). Each DNN takes input of both spectral features and converted spatial features that are robust to position mismatch, and outputs the separation mask for target source estimation. In addition, a psychacoustically-motivated objective function is integrated in each DNN, which explores perceptual importance of each TF unit in the training process. Objective evaluations are performed on the separated sounds using the converged models, in terms of PESQ, SDR as well as STOI. Overall, all the implemented DNNs have greatly improved the quality and speech intelligibility of the embedded target source as compared to the original recordings. In particular, bidirectional RNN, either along the temporal direction or along the frequency bins, outperforms the other DNN structures with consistent improvement.
The intelligibility of speech in noise can be improved by modifying the speech. But with object-based audio, there is the possibility of altering the background sound while leaving the speech unaltered. This may prove a less intrusive approach, affording good speech intelligibility without overly compromising the perceived sound quality. In this study, the technique of spectral weighting was applied to the background. The frequency-dependent weightings for adaptation were learnt by maximising a weighted combination of two perceptual objective metrics for speech intelligibility and audio quality. The balance between the two objective metrics was determined by the perceptual relationship between intelligibility and quality. A neural network was trained to provide a fast solution for real-time processing. Tested in a variety of background sounds and speech-to-background ratios (SBRs), the proposed method led to a large intelligibility gain over the unprocessed baseline. Compared to an approach using constant weightings, the proposed method was able to dynamically preserve the overall audio quality better with respect to SBR changes.
In object-based spatial audio system, positions of the audio objects (e.g. speakers/talkers or voices) presented in the sound scene are required as important metadata attributes for object acquisition and reproduction. Binaural microphones are often used as a physical device to mimic human hearing and to monitor and analyse the scene, including localisation and tracking of multiple speakers. The binaural audio tracker, however, is usually prone to the errors caused by room reverberation and background noise. To address this limitation, we present a multimodal tracking method by fusing the binaural audio with depth information (from a depth sensor, e.g., Kinect). More specifically, the PHD filtering framework is first applied to the depth stream, and a novel clutter intensity model is proposed to improve the robustness of the PHD filter when an object is occluded either by other objects or due to the limited field of view of the depth sensor. To compensate mis-detections in the depth stream, a novel gap filling technique is presented to map audio azimuths obtained from the binaural audio tracker to 3D positions, using speaker-dependent spatial constraints learned from the depth stream. With our proposed method, both the errors in the binaural tracker and the mis-detections in the depth tracker can be significantly reduced. Real-room recordings are used to show the improved performance of the proposed method in removing outliers and reducing mis-detections.
A novel multimodal (audio-visual) approach to the problem of blind source separation (BSS) is evaluated in room environments. The main challenges of BSS in realistic environments are: 1) sources are moving in complex motions and 2) the room impulse responses are long. For moving sources the unmixing filters to separate the audio signals are difficult to calculate from only statistical information available from a limited number of audio samples. For physically stationary sources measured in rooms with long impulse responses, the performance of audio only BSS methods is limited. Therefore, visual modality is utilized to facilitate the separation. The movement of the sources is detected with a 3-D tracker based on a Markov Chain Monte Carlo particle filter (MCMC-PF), and the direction of arrival information of the sources to the microphone array is estimated. A robust least squares frequency invariant data independent (RLSFIDI) beamformer is implemented to perform real time speech enhancement. The uncertainties in source localization and direction of arrival information are also controlled by using a convex optimization approach in the beamformer design. A 16 element circular array configuration is used. Simulation studies based on objective and subjective measures confirm the advantage of beamforming based processing over conventional BSS methods. © 2011 EURASIP.
Object-based audio can be used to customize, personalize, and optimize audio reproduction depending on the speci?c listening scenario. To investigate and exploit the bene?ts of object-based audio, a framework for intelligent metadata adaptation was developed. The framework uses detailed semantic metadata that describes the audio objects, the loudspeakers, and the room. It features an extensible software tool for real-time metadata adaptation that can incorporate knowledge derived from perceptual tests and/or feedback from perceptual meters to drive adaptation and facilitate optimal rendering. One use case for the system is demonstrated through a rule-set (derived from perceptual tests with experienced mix engineers) for automatic adaptation of object levels and positions when rendering 3D content to two- and ?ve-channel systems.
In this paper, a novel probabilistic Bayesian tracking scheme is proposed and applied to bimodal measurements consisting of tracking results from the depth sensor and audio recordings collected using binaural microphones. We use random finite sets to cope with varying number of tracking targets. A measurement-driven birth process is integrated to quickly localize any emerging person. A new bimodal fusion method that prioritizes the most confident modality is employed. The approach was tested on real room recordings and experimental results show that the proposed combination of audio and depth outperforms individual modalities, particularly when there are multiple people talking simultaneously and when occlusions are frequent.
© 2014 IEEE.The visual modality, deemed to be complementary to the audio modality, has recently been exploited to improve the performance of blind source separation (BSS) of speech mixtures, especially in adverse environments where the performance of audio-domain methods deteriorates steadily. In this paper, we present an enhancement method to audio-domain BSS with the integration of voice activity information, obtained via a visual voice activity detection (VAD) algorithm. Mimicking aspects of human hearing, binaural speech mixtures are considered in our two-stage system. Firstly, in the off-line training stage, a speaker-independent voice activity detector is formed using the visual stimuli via the adaboosting algorithm. In the on-line separation stage, interaural phase difference (IPD) and interaural level difference (ILD) cues are statistically analyzed to assign probabilistically each time-frequency (TF) point of the audio mixtures to the source signals. Next, the detected voice activity cues (found via the visual VAD) are integrated to reduce the interference residual. Detection of the interference residual takes place gradually, with two layers of boundaries in the correlation and energy ratio map. We have tested our algorithm on speech mixtures generated using room impulse responses at different reverberation times and noise levels. Simulation results show performance improvement of the proposed method for target speech extraction in noisy and reverberant environments, in terms of signal-to-interference ratio (SIR) and perceptual evaluation of speech quality (PESQ).
Deep neural networks (DNN) have recently been shown to give state-of-the-art performance in monaural speech enhancement. However in the DNN training process, the perceptual difference between different components of the DNN output is not fully exploited, where equal importance is often assumed. To address this limitation, we have proposed a new perceptually-weighted objective function within a feedforward DNN framework, aiming to minimize the perceptual difference between the enhanced speech and the target speech. A perceptual weight is integrated into the proposed objective function, and has been tested on two types of output features: spectra and ideal ratio masks. Objective evaluations for both speech quality and speech intelligibility have been performed. Integration of our perceptual weight shows consistent improvement on several noise levels and a variety of different noise types.
State-of-the-art binaural objective intelligibility measures (OIMs) require individual source signals for making intelligibility predictions, limiting their usability in real-time online operations. This limitation may be addressed by a blind source separation (BSS) process, which is able to extract the underlying sources from a mixture. In this study, a speech source is presented with either a stationary noise masker or a fluctuating noise masker whose azimuth varies in a horizontal plane, at two speech-to-noise ratios (SNRs). Three binaural OIMs are used to predict speech intelligibility from the signals separated by a BSS algorithm. The model predictions are compared with listeners' word identification rate in a perceptual listening experiment. The results suggest that with SNR compensation to the BSS-separated speech signal, the OIMs can maintain their predictive power for individual maskers compared to their performance measured from the direct signals. It also reveals that the errors in SNR between the estimated signals are not the only factors that decrease the predictive accuracy of the OIMs with the separated signals. Artefacts or distortions on the estimated signals caused by the BSS algorithm may also be concerns.
The work on 3D human pose estimation has seen a significant amount of progress in recent years, particularly due to the widespread availability of commodity depth sensors. However, most pose estimation methods follow a tracking-as-detection approach which does not explicitly handle occlusions, thus introducing outliers and identity association issues when multiple targets are involved. To address these issues, we propose a new method based on Probability Hypothesis Density (PHD) filter. In this method, the PHD filter with a novel clutter intensity model is used to remove outliers in the 3D head detection results, followed by an identity association scheme with occlusion detection for the targets. Experimental results show that our proposed method greatly mitigates the outliers, and correctly associates identities to individual detections with low computational cost.
In this paper, we propose an iterative deep neural network (DNN)-based binaural source separation scheme, for recovering two concurrent speech signals in a room environment. Besides the commonly-used spectral features, the DNN also takes non-linearly wrapped binaural spatial features as input, which are refined iteratively using parameters estimated from the DNN output via a feedback loop. Different DNN structures have been tested, including a classic multilayer perception regression architecture as well as a new hybrid network with both convolutional and densely-connected layers. Objective evaluations in terms of PESQ and STOI showed consistent improvement over baseline methods using traditional binaural features, especially when the hybrid DNN architecture was employed. In addition, our proposed scheme is robust to mismatches between the training and testing data.
A non-intrusive method is introduced to predict binaural speech intelligibility in noise directly from signals captured using a pair of microphones. The approach combines signal processing techniques in blind source separation and localisation, with an intrusive objective intelligibility measure (OIM). Therefore, unlike classic intrusive OIMs, this method does not require a clean reference speech signal and knowing the location of the sources to operate. The proposed approach is able to estimate intelligibility in stationary and fluctuating noises, when the noise masker is presented as a point or diffused source, and is spatially separated from the target speech source on a horizontal plane. The performance of the proposed method was evaluated in two rooms. When predicting subjective intelligibility measured as word recognition rate, this method showed reasonable predictive accuracy with correlation coefficients above 0.82, which is comparable to that of a reference intrusive OIM in most of the conditions. The proposed approach offers a solution for fast binaural intelligibility prediction, and therefore has practical potential to be deployed in situations where on-site speech intelligibility is a concern.
Binaural features of interaural level difference and interaural phase difference have proved to be very effective in training deep neural networks (DNNs), to generate timefrequency masks for target speech extraction in speech-speech mixtures. However, effectiveness of binaural features is reduced in more common speech-noise scenarios, since the noise may over-shadow the speech in adverse conditions. In addition, the reverberation also decreases the sparsity of binaural features and therefore adds difficulties to the separation task. To address the above limitations, we highlight the spectral difference between speech and noise spectra and incorporate the log-power spectra features to extend the DNN input. Tested on two different reverberant rooms at different signal to noise ratios (SNR), our proposed method shows advantages over the baseline method using only binaural features in terms of signal to distortion ratio (SDR) and Short-Time Perceptual Intelligibility (STOI).
Object-based audio has the potential to enable multime- dia content to be tailored to individual listeners and their reproduc- tion equipment. In general, object-based production assumes that the objects|the assets comprising the scene|are free of noise and inter- ference. However, there are many applications in which signal separa- tion could be useful to an object-based audio work ow, e.g., extracting individual objects from channel-based recordings or legacy content, or recording a sound scene with a single microphone array. This paper de- scribes the application and evaluation of blind source separation (BSS) for sound recording in a hybrid channel-based and object-based workflow, in which BSS-estimated objects are mixed with the original stereo recording. A subjective experiment was conducted using simultaneously spoken speech recorded with omnidirectional microphones in a rever- berant room. Listeners mixed a BSS-extracted speech object into the scene to make the quieter talker clearer, while retaining acceptable au- dio quality, compared to the raw stereo recording. Objective evaluations show that the relative short-term objective intelligibility and speech qual- ity scores increase using BSS. Further objective evaluations are used to discuss the in uence of the BSS method on the remixing scenario; the scenario shown by human listeners to be useful in object-based audio is shown to be a worse-case scenario.
Representing a complex acoustic scene with audio objects is desirable but challenging in object-based spatial audio production and reproduction, especially when concurrent sound signals are present in the scene. Source separation (SS) provides a potentially useful and enabling tool for audio object extraction. These extracted objects are often remixed to reconstruct a sound field in the reproduction stage. A suitable SS method is expected to produce audio objects that ultimately deliver high quality audio after remix. The performance of these SS algorithms therefore needs to be evaluated in this context. Existing metrics for SS performance evaluation, however, do not take into account the essential sound field reconstruction process. To address this problem, here we propose a new SS evaluation method which employs a remixing strategy similar to the panning law, and provides a framework to incorporate the conventional SS metrics. We have tested our proposed method on real-room recordings processed with four SS methods, including two state-of-the art blind source separation (BSS) methods and two classic beamforming algorithms. The evaluation results based on three conventional SS metrics are analysed.
We propose a new method for source separation by synthesizing the source from a speech mixture corrupted by various environmental noise. Unlike traditional source separation methods which estimate the source from the mixture as a replica of the original source (e.g. by solving an inverse problem), our proposed method is a synthesis-based approach which aims to generate a new signal (i.e. “fake” source) that sounds similar to the original source. The proposed system has an encoder-decoder topology, where the encoder predicts intermediate-level features from the mixture, i.e. Mel-spectrum of the target source, using a hybrid recurrent and hourglass network, while the decoder is a state-of-the-art WaveNet speech synthesis network conditioned on the Mel-spectrum, which directly generates time-domain samples of the sources. Both objective and subjective evaluations were performed on the synthesized sources, and show great advantages of our proposed method for high-quality speech source separation and generation.
Apparatus and methods are disclosed for performing object-based audio rendering on a plurality of audio objects which define a sound scene, each audio object comprising at least one audio signal and associated metadata. The apparatus comprises: a plurality of renderers each capable of rendering one or more of the audio objects to output rendered audio data; and object adapting means for adapting one or more of the plurality of audio objects for a current reproduction scenario, the object adapting means being configured to send the adapted one or more audio objects to one or more of the plurality of renderers.