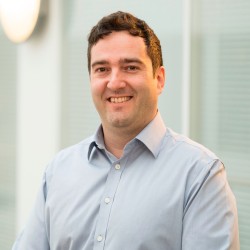
Dr Riccardo Pozza
Publications
The occurrence of short but recurrent opportunistic contacts between static infrastructure and mobile devices largely characterizes recent Internet of Things (IoT) applications for Smart Cities and Smart Buildings scenarios. In order to efficiently exploit such existing communication opportunities to access services, share and collect data, IoT applications cannot rely on standard discovery mechanisms that periodically probe the environment to discover resources. Discovery protocols resilient to different contact opportunities and able to optimize energy consumption when device contacts are not present are therefore required in order to avoid waste of energy especially in battery operated user devices. Additionally, such new discovery protocols need to optimize the time available for communication operations, while being able to adjust to application requirements, and balance energy consumption with respect to latency for contacts discovery. To this aim, we introduce CARD, a Context Aware Resource Discovery framework that leveraging Q-Learning techniques extends the functionalities of asynchronous neighbor discovery protocols, while being capable to reduce energy wastage and discovery latency. Simulation results show that CARD performs better than existing approaches and is resilient to a variety of real scenarios characterizing Smart Cities and Smart Building deployments.
There has been increasing interest in deploying Internet of Things (IoT) devices to study human behavior in locations such as homes and offices. Such devices can be deployed in a laboratory or “in the wild” in natural environments. The latter allows one to collect behavioral data that is not contaminated by the artificiality of a laboratory experiment. Using IoT devices in ordinary environments also brings the benefits of reduced cost, as compared with lab experiments, and less disturbance to the participants’ daily routines, which in turn helps with recruiting them into the research. However, in this case, it is essential to have an IoT infrastructure that can be easily and swiftly installed and from which real-time data can be securely and straightforwardly collected. In this article, we present MakeSense, an IoT testbed that enables real-world experimentation for large-scale social research on indoor activities through real-time monitoring and/or situation-aware applications. The testbed features quick setup, flexibility in deployment, the integration of a range of IoT devices, resilience, and scalability. We also present two case studies to demonstrate the use of the testbed: one in homes and one in offices.
There has been increasing interest in deploying IoT devices to study human behaviour in locations such as homes and offices. Such devices can be deployed in a laboratory or `in the wild' in natural environments. The latter allows one to collect behavioural data that is not contaminated by the artificiality of a laboratory experiment. Using IoT devices in ordinary environments also brings the benefits of reduced cost, as compared with lab experiments, and less disturbance to the participants' daily routines which in turn helps with recruiting them into the research. However, in this case, it is essential to have an IoT infrastructure that can be easily and swiftly installed and from which real-time data can be securely and straightforwardly collected. In this paper, we present MakeSense, an IoT testbed that enables real-world experimentation for large scale social research on indoor activities through real-time monitoring and/or situation-aware applications. The testbed features quick setup, flexibility in deployment, the integration of a range of IoT devices, resilience, and scalability. We also present two case studies to demonstrate the use of the testbed, one in homes and one in offices.
In this article, an Arrival and Departure Time Predictor (ADTP) for scheduling communication in opportunistic Internet of Things (IoT) is presented. The proposed algorithm learns about temporal patterns of encounters between IoT devices and predicts future arrival and departure times, therefore future contact durations. By relying on such predictions, a neighbour discovery scheduler is proposed, capable of jointly optimizing discovery latency and power consumption in order to maximize communication time when contacts are expected with high probability and, at the same time, saving power when contacts are expected with low probability. A comprehensive performance evaluation with different sets of synthetic and real world traces shows that ADTP performs favourably with respect to previous state of the art. This prediction framework opens opportunities for transmission planners and schedulers optimizing not only neighbour discovery, but the entire communication process.
The evolution of network technologies has witnessed a paradigm shift toward open and intelligent networks, with the Open Radio Access Network (O-RAN) architecture emerging as a promising solution. O-RAN introduces disaggregation and virtualization, enabling network operators to deploy multi-vendor and interoperable solutions. However, managing and automating the complex O-RAN ecosystem presents numerous challenges. To address this, machine learning (ML) techniques have gained considerable attention in recent years, offering promising avenues for network automation in O-RAN. This paper presents a comprehensive survey of the current research efforts on network automation usingML in O-RAN.We begin by providing an overview of the O-RAN architecture and its key components, highlighting the need for automation. Subsequently, we delve into O-RAN support forML techniques. The survey then explores challenges in network automation usingML within the O-RAN environment, followed by the existing research studies discussing application of ML algorithms and frameworks for network automation in O-RAN. The survey further discusses the research opportunities by identifying important aspects whereML techniques can benefit.
What activities take place at home? When do they occur, for how long do they last and who is involved? Asking such questions is important in social research on households, e.g., to study energyrelated practices, assisted living arrangements and various aspects of family and home life. Common ways of seeking the answers rest on self-reporting which is provoked by researchers (interviews, questionnaires, surveys) or non-provoked (time use diaries). Longitudinal observations are also common, but all of these methods are expensive and time-consuming for both the participants and the researchers. The advances of digital sensors may provide an alternative. For example, temperature, humidity and light sensors report on the physical environment where activities occur, while energy monitors report information on the electrical devices that are used to assist the activities. Using sensor-generated data for the purposes of activity recognition is potentially a very powerful means to study activities at home. However, how can we quantify the agreement between what we detect in sensor-generated data and what we know from self-reported data, especially nonprovoked data? To give a partial answer, we conduct a trial in a household in which we collect data from a suite of sensors, as well as from a time use diary completed by one of the two occupants. For activity recognition using sensor-generated data, we investigate the application of mean shift clustering and change points detection for constructing features that are used to train a Hidden Markov Model. Furthermore, we propose a method for agreement evaluation between the activities detected in the sensor data and that reported by the participants based on the Levenshtein distance. Finally, we analyse the use of different features for recognising different types of activities.
Understanding home activities is important in social research to study aspects of home life, e.g., energy-related practices and assisted living arrangements. Common approaches to identifying which activities are being carried out in the home rely on self-reporting, either retrospectively (e.g., interviews, questionnaires, and surveys) or at the time of the activity (e.g., time use diaries). The use of digital sensors may provide an alternative means of observing activities in the home. For example, temperature, humidity and light sensors can report on the physical environment where activities occur, while energy monitors can report information on the electrical devices that are used to assist the activities. One may then be able to infer from the sensor data which activities are taking place. However, it is first necessary to calibrate the sensor data by matching it to activities identified from self-reports. The calibration involves identifying the features in the sensor data that correlate best with the self-reported activities. This in turn requires a good measure of the agreement between the activities detected from sensor-generated data and those recorded in self-reported data. To illustrate how this can be done, we conducted a trial in three single-occupancy households from which we collected data from a suite of sensors and from time use diaries completed by the occupants. For sensor-based activity recognition, we demonstrate the application of Hidden Markov Models with features extracted from mean-shift clustering and change points analysis. A correlation-based feature selection is also applied to reduce the computational cost. A method based on Levenshtein distance for measuring the agreement between the activities detected in the sensor data and that reported by the participants is demonstrated. We then discuss how the features derived from sensor data can be used in activity recognition and how they relate to activities recorded in time use diaries.
Neighbor discovery was initially conceived as a means to deal with energy issues at deployment, where the main objective was to acquire information about network topology for subsequent communication. Nevertheless, over recent years, it has been facing new challenges due to the introduction of mobility of nodes over static networks mainly caused by the opportunistic presence of nodes in such a scenario. The focus of discovery has, therefore, shifted toward more challenging environments, where connectivity opportunities need to be exploited for achieving communication. In fact, discovery has traditionally been focused on tradeoffs between energy and latency in order to reach an overlapping of communication times between neighboring nodes. With the introduction of opportunistic networking, neighbor discovery has instead aimed toward the more challenging problem of acquiring knowledge about the patterns of encounters between nodes. Many Internet of Things applications (e.g., smart cities) can, in fact, benefit from such discovery, since end-to-end paths may not directly exist between sources and sinks of data, thus requiring the discovery and exploitation of rare and short connectivity opportunities to relay data. While many of the older discovery approaches are still valid, they are not entirely designed to exploit the properties of these new challenging scenarios. A recent direction in research is, therefore, to learn and exploit knowledge about mobility patterns to improve the efficiency in the discovery process. In this paper, a new classification and taxonomy is presented with an emphasis on recent protocols and advances in this area, summarizing issues and ways for potential improvements. As we will show, knowledge integration in the process of neighbor discovery leads to a more efficient scheduling of the resources when contacts are expected, thus allowing for faster discovery, while, at the same time allowing for energy savings when such contacts are not expec- ed.