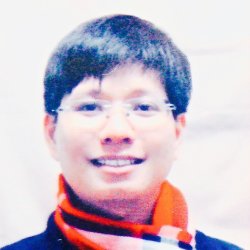
Dr Viet Hung Tran
About
Biography
Viet Hung Tran received the B.Eng. degree from Hochiminh city University of Technology, Vietnam in 2008, the master's degree from ENS Cachan, Paris, France in 2009, and the Ph.D. degree from the Trinity College Dublin, Ireland in 2014. His thesis was entitled "Variational Bayes inference for digital receivers".
From 2014 to 2016 he held a post-doctoral position with Telecom Paris institute, Paris, France. He is currently a Research Fellow at CVSSP, University of Surrey, U.K. His research interest is optimal algorithms for Bayesian learning network and information theory. He was awarded the best mathematical paper prize at IEEE Irish Signals and Systems Conference, 2011.
Areas of specialism
University roles and responsibilities
- CVSSP, Faculty of Electronics and Physical Sciences (FEPS), University of Surrey, U.K.
Previous roles
- Best mathematical paper prize at IEEE ISSC 2011
Publications
Highlights
VH. Tran and W.Wang, "Bayesian inference for PCA and MUSIC algorithms with unknown number of sources", IEEE Trans. on Signal Processing 2018 https://arxiv.org/abs/1809.10168
VH. Tran, "Copula Variational Bayes inference via information geometry", IEEE Trans. on Information Theory 2018 https://arxiv.org/abs/1803.10998
VH. Tran and M. Coupechoux, “Cost-constrained Viterbi algorithm for resource allocation in solar base stations”, IEEE Trans. on wireless communications, 2017. http://ieeexplore.ieee.org/document/7898519/
Solar energy is currently a popular renewable resource, yet limited daily. In green cellular networks, multiple constraints optimization (MCO) problems arise naturally.
In this paper, we formulate this generic MCO problem as a quantized Markovian cost-reward model, with no assumption on input data. We then propose a novel algorithm, namely cost-constrained Viterbi algorithm (CVA), which recursively returns the optimal policy with linear computational complexity for this model.
Our simulation shows that, iterative CVA can save up to 85% of consumed grid energy in typical scenario of hexagonal base station network, given the same QoS constraint of fixed transmission power scheme.
Our iterative CVA is flexible, optimal and applicable to constrained cost-reward problems in generic continuous and discrete networks.
Variational Bayes (VB), also known as independent mean-field approximation, has become a popular method for Bayesian network inference in recent years. Its application is vast, e.g. in neural network, compressed sensing, clustering, etc. to name just a few. In this paper, the independence constraint in VB will be relaxed to a conditional constraint class, called copula in statistics. Since a joint probability distribution always belongs to a copula class, the novel copula VB (CVB) approximation is a generalized form of VB. Via information geometry, we will see that CVB algorithm iteratively projects the original joint distribution to a copula constraint space until it reaches a local minimum Kullback-Leibler (KL) divergence. By this way, all mean-field approximations, e.g. iterative VB, Expectation-Maximization (EM), Iterated Conditional Mode (ICM) and k-means algorithms, are special cases of CVB approximation.
For a generic Bayesian network, an augmented hierarchy form of CVB will also be designed. While mean-field algorithms can only return a locally optimal approximation for a correlated network, the augmented CVB network, which is an optimally weighted average of a mixture of simpler network structures, can potentially achieve the globally optimal approximation for the first time. Via simulations of Gaussian mixture clustering, the classification's accuracy of CVB will be shown to be far superior to that of state-of-the-art VB, EM and k-means algorithms.
Principal component analysis (PCA) is a popular method for projecting data onto uncorrelated components in lower dimension, although the optimal number of components is not specified. Likewise, multiple signal classification (MUSIC) algorithm is a popular PCA-based method for estimating directions of arrival (DOAs) of sinusoidal sources, yet it requires the number of sources to be known a priori. The accurate estimation of the number of sources is hence a crucial issue for performance of these algorithms. In this paper, we will show that both PCA and MUSIC actually return the exact joint maximum-a-posteriori (MAP) estimate for uncorrelated steering vectors, although they can only compute this MAP estimate approximately in correlated case. We then use Bayesian method to, for the first time, compute the MAP estimate for the number of sources in PCA and MUSIC algorithms. Intuitively, this MAP estimate corresponds to the highest probability that signal-plus-noise's variance still dominates projected noise's variance on signal subspace. In simulations of overlapping multi-tone sources for linear sensor array, our exact MAP estimate is far superior to the asymptotic Akaike information criterion (AIC), which is a popular method for estimating the number of components in PCA and MUSIC algorithms.