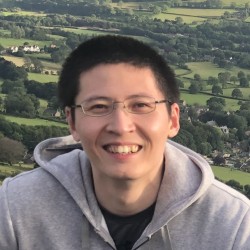
Dr Xiaowei Gu
Academic and research departments
Computer Science Research Centre, School of Computer Science and Electronic Engineering.About
Biography
Dr. Xiaowei Gu received his PhD in Computer Science from Lancaster University (UK) in 2018. Before joining Surrey, Xiaowei was a Lecturer in Computing at the University of Kent (UK), a Lecturer in Computer Science at Aberystwyth University (UK) and a Senior Research Associate at Lancaster University.
Areas of specialism
University roles and responsibilities
- MSc Employability Lead
My qualifications
ResearchResearch interests
Xiaowei’s research is focused on developing novel machine learning models that 1) have a transparent system structure and human-interpretable reasoning process, and 2) are capable of offering the state-of-the-art performance but with less demand of human expertise involvement. Xiaowei is also interested in developing explainable semi-supervised machine learning models to tackle streaming data problems.
Research projects
Xiaowei Gu, New Investigator Award funded by Engineering and Physical Sciences Research Council (EPSRC), funding amount £267,600, 2024-2026
An Explainable Generic Design of Self-Evolving Intelligent Security Systems for Cyber attack DetectionXiaowei Gu and Gareth Howells, research funded by Frazer-Nash Consultancy Ltd. on behalf of the Defence Science and Technology Laboratory (Dstl), funding amount £122,556, 2023-2024
Indicators of esteem
Associate Editor - Neurocomputing, Elsevier, since 2022
Area Editor - Evolving Systems, Springer, since 2025
Associate Editor - Evolving Systems, Springer, 2020-2024
Member of IEEE Computational Intelligence Society Standards Committee, since 2024
Program Committee Co-chair of IEEE International Conference on Machine Learning and Applications, Miami, Florida, US, Dec 18-20, 2024
Web & Publicity Chair of IEEE Conference on Evolving and Adaptive Intelligent Systems, Madrid, Spain, May 23-24, 2024
International Neural Network Society Doctoral Dissertation Award, 2020
Chinese Government Award for Outstanding Self-Financed Students Abroad, 2019
Research interests
Xiaowei’s research is focused on developing novel machine learning models that 1) have a transparent system structure and human-interpretable reasoning process, and 2) are capable of offering the state-of-the-art performance but with less demand of human expertise involvement. Xiaowei is also interested in developing explainable semi-supervised machine learning models to tackle streaming data problems.
Research projects
Xiaowei Gu, New Investigator Award funded by Engineering and Physical Sciences Research Council (EPSRC), funding amount £267,600, 2024-2026
Xiaowei Gu and Gareth Howells, research funded by Frazer-Nash Consultancy Ltd. on behalf of the Defence Science and Technology Laboratory (Dstl), funding amount £122,556, 2023-2024
Indicators of esteem
Associate Editor - Neurocomputing, Elsevier, since 2022
Area Editor - Evolving Systems, Springer, since 2025
Associate Editor - Evolving Systems, Springer, 2020-2024
Member of IEEE Computational Intelligence Society Standards Committee, since 2024
Program Committee Co-chair of IEEE International Conference on Machine Learning and Applications, Miami, Florida, US, Dec 18-20, 2024
Web & Publicity Chair of IEEE Conference on Evolving and Adaptive Intelligent Systems, Madrid, Spain, May 23-24, 2024
International Neural Network Society Doctoral Dissertation Award, 2020
Chinese Government Award for Outstanding Self-Financed Students Abroad, 2019
Supervision
Postgraduate research supervision
Exceptional PhD applicants/visitors with a proven track record of academic excellence and a strong research background are very welcome! If you are interested, please contact me via email: xiaowei.gu@surrey.ac.uk.
To facilitate efficient communication, please ensure your email includes the following two items:
- A one-page CV highlighting your academic achievements and relevant experience.
- A one-page document outlining your research idea and its alignment with my research.
Due to the high volume of emails received, queries missing these two items may not receive a response.
Teaching
COM1027 - Programming Fundamentals
EEEM005 - AI and AI Programming
Publications
High-dimensional data classification is widely considered as a challenging task in machine learning due to the so-called "curse of dimensionality". In this paper, a novel multilayer jointly evolving and compressing fuzzy neural network (MECFNN) is proposed to learn highly compact multi-level latent representations from high-dimensional data. As a meta-level stacking ensemble system, each layer of MECFNN is based on a single jointly evolving and compressing neural fuzzy inference system (ECNFIS) that self-organises a set of human-interpretable fuzzy rules from input data in a sample-wise manner to perform approximate reasoning. ECNFISs associate a unique compressive projection matrix to each individual fuzzy rule to compress the consequent part into a tighter form, removing redundant information whilst boosting the diversity within the stacking ensemble. The compressive projection matrices of the cascading ECNFISs are self-updating to minimise the prediction errors via error backpropagation together with the consequent parameters, empowering MECFNN to learn more meaningful, discriminative representations from data at multiple levels of abstraction. An adaptive activation control scheme is further introduced in MECFNN to dynamically exclude less activated fuzzy rules, effectively reducing the computational complexity and fostering generalisation. Numerical examples on popular high-dimensional classification problems demonstrate the efficacy of MECFNN.
In this paper, a novel autonomous centreless algorithm is proposed for data partitioning. The proposed algorithm firstly constructs the nearest neighbour affinity graph and identifies the local peaks of data density to build micro-clusters. Unlike the vast majority of partitional clustering algorithms, the proposed algorithm does not rely on singleton prototypes, namely, centres or medoids of the micro-clusters to partition the data space. Instead, these micro-clusters are directly utilised to attract nearby data samples to form shape-free Voronoi tessellations, hence, being centreless and robust to noisy data. A fusion scheme is further implemented to fuse these data clouds with higher intra-cluster similarity together to attain a more compact partitioning of data. The proposed algorithm is able to perform data partitioning on a chunk-wise basis and is highly computationally efficient with the default distance measure. Therefore, it is suitable for both static data partitioning in offline scenarios and streaming data partitioning in online scenarios. Numerical examples on a variety of benchmark datasets demonstrate the efficacy of the proposed algorithm.
In contemporary organizational research, when dealing with large heterogeneous datasets and complex relationships, statistical modeling focused on developing substantive explanations typically results in low predictive accuracy. In contrast, machine learning (ML) exhibits remarkable strength for prediction, but suffers from an unexplainable analytical process and output—thus ML is often known as a “black box” approach. The recent development of Explainable Machine Learning (XML) integrates high predictive accuracy with explainability, which combines the advantages inherent in both statistical modeling and ML paradigms. This paper compares XML with statistical modeling and the traditional ML approaches, focusing on an advanced application of XML known as Explanatory Fuzzy System (EFS), which enhances model transparency by clarifying the unique contribution of each modeled predictor. In an illustrative study, we demonstrate two EFS-based XML models and conduct comparative analyses among XML, ML, and statistical models with a commonly-used database in organizational research. Our study offers a thorough description of analysis procedures for implementing XML in organizational research, along with best-practice recommendations for each step as well as Python code to aid future research using XML. Finally, we discuss the benefits of XML for organizational research and its potential development.
The escalating cyber-attacks in recent years have created a significant demand for advanced intrusion detection systems. However, the dynamic characteristics of network data streams, scarcity of labelled data, imbalance of class distribution and concerns about the explainability of predictive models have greatly restricted the applicability of conventional machine learning and cutting-edge deep learning techniques in real-world scenarios for intrusion detection. In this paper, a novel soft prototype-based fuzzy ensemble intrusion detection system is proposed to autonomously exploit semi-supervised learning from network data streams by exploiting the pseudo-labelling method. To reduce the pseudo-labelling errors, although the proposed ensemble system involves all the base models for joint pseudo-labelling, it only utilises pseudo-labelled data with the highest consensus for self-improvement. To foster generalisation, the proposed ensemble system leverages sampling techniques to address the class imbalance within the labelled and pseudo-labelled data, and the qualities of base models are constantly monitored, ensuring that weaker base models are efficiently substituted. Additionally, instead of discarding these challenging-to-classify samples during online semi-supervised learning, the proposed ensemble system summarises them into a smaller number of unlabelled soft prototypes, allowing human experts to contribute to the learning at any point by manually labelling these soft prototypes to further augment the learned knowledge base. Numerical examples on public network intrusion detection datasets demonstrated the superior performance of the proposed ensemble system.
Anomaly detection from data streams is a hotly studied topic in the machine learning domain. It is widely considered a challenging task because the underlying patterns exhibited by the streaming data may dynamically change at any time. In this paper, a new algorithm is proposed to detect anomalies autonomously for streaming data. The proposed algorithm is nonparametric and does not require any threshold to be preset by users. The algorithmic procedure of the proposed algorithm is composed of the following three complementary stages. Firstly, the potentially anomalous samples that represent highly different patterns from others are identified from data streams based on data density. Then, these potentially anomalous samples are clustered online using the evolving autonomous data partitioning algorithm. Finally, true anomalies are identified from these minor clusters with the least amounts of samples associated with them. Numerical examples based on three benchmark datasets demonstrated the potential of the proposed algorithm as a highly effective approach for anomaly detection from data streams.
The complex nature of the foreign exchange (FOREX) market along with the increased interest towards the currency exchange market has prompted extensive research from various academic disciplines. With the inclusion of more in-depth analysis and forecasting methods, traders will be able to make an informed decision when trading. Therefore, an approach incorporating the use of historical data along with computational intelligence for analysis and forecasting is proposed in this paper. Firstly, the Gaussian Mixture Model method is applied for data partitioning on historical observations. While the antecedent part of the neurofuzzy system of AnYa type is initialised by the partitioning result, the consequent part is trained using the fuzzily weighted RLS algorithm based on the same data. Numerical examples based on the real currency exchange data demonstrated that the proposed approach trained with historical data produce promising results when used to forecast the future foreign exchange rates over a long-term period. Although implemented in an offline environment, it could potentially be utilised in real-time application in the future. (C) 2018 The Authors. Published by Elsevier Ltd.
Satellite scene images contain multiple sub-regions of different land use categories; however, traditional approaches usually classify them into a particular category only. In this paper, a new approach is proposed for automatically analyzing the semantic content of sub-regions of satellite images. At the core of the proposed approach is the recently introduced deep rule-based image classification method. The proposed approach includes a self-organizing set of transparent zero order fuzzy IF THEN rules with human-interpretable prototypes identified from the training images and a pre-trained deep convolutional neural network as the feature descriptor. It requires a very short, nonparametric, highly parallelizable training process and can perform a highly accurate analysis on the semantic features of local areas of the image with the generated IF-THEN rules in a fully automatic way. Examples based on benchmark datasets demonstrate the validity and effectiveness of the proposed approach.
Mobile medical app evaluation can be modelled as a multi-attribute decision-making (MADM) problem with multiple assessment attributes. Due to the increasing complexity and high uncertainty of decision environments, numerical numbers and/or traditional fuzzy sets may not be appropriate to model attribute information of mobile medical apps. In addition, heterogeneous relationships are often observed among different attributes in various practical decision situations. To deal with these issues, a q-rung orthopair fuzzy (q-ROF) MADM approach, which is a very powerful tool for describing vague information occurring in real decision circumstances, is proposed to handle decision-making problems in medical app evaluation. In particular, q-rung orthopair fuzzy numbers (q-ROFNs) are first applied to better express the preference information and expert assessment information. Then, q-ROFNs are extended by combining with Zhenyuan integral, resulting in the qROF Zhenyuan integral (q-ROFZI). This integral can capture complementary, redundant and/or independent characteristics among the attributes and is superior to existing operators on q-ROFNs. Next, based on the bestworst method (BWM) and Shapley value, two optimization models are constructed to objectively identify optimal fuzzy measures on the attribute set. Finally, a novel integrated q-ROF MADM approach is proposed and its computation procedure is presented and illustrated with its application to the problem of mobile medical app evaluation. A comparative analysis is carried out to demonstrate the validity, rationality, robustness and superiority of the developed method.
In this paper, a novel autonomous data-driven clustering approach, called AD_clustering, is presented for live data streams processing. This newly proposed algorithm is a fully unsupervised approach and entirely based on the data samples and their ensemble properties, in the sense that there is no need for user-predefined or problem-specific assumptions and parameters, which is a problem most of the current clustering approaches suffer from. Moreover, the proposed approach automatically evolves its structure according to the experimentally observable streaming data and is able to recursively update its self-defined parameters using only the current data sample; meanwhile, it discards all the previously processed data samples. Experimental results based on benchmark datasets exhibit the higher performance of the proposed fully autonomous approach compared with the comparative approaches requiring user- and problem-specific parameters to be predefined. This new clustering algorithm is a promising tool for further applications in the field of real-time streaming data analytics.
Evolving fuzzy systems (EFSs) are now well developed and widely used, thanks to their ability to self-adapt both their structures and parameters online. Since the concept was first introduced two decades ago, many different types of EFSs have been successfully implemented. However, there are only very few works considering the stability of the EFSs, and these studies were limited to certain types of membership functions with specifically predefined parameters, which largely increases the complexity of the learning process. At the same time, stability analysis is of paramount importance for control applications and provides the theoretical guarantees for the convergence of the learning algorithms. In this paper, we introduce the stability proof of a class of EFSs based on data clouds, which are grounded at the AnYa type fuzzy systems and the recently introduced empirical data analytics (EDA) methodological framework. By employing data clouds, the class of EFSs of AnYa type considered in this paper avoids the traditional way of defining membership functions for each input variable in an explicit manner and its learning process is entirely data driven. The stability of the considered EFS of AnYa type is proven through the Lyapunov theory, and the proof of stability shows that the average identification error converges to a small neighborhood of zero. Although, the stability proof presented in this paper is specially elaborated for the considered EFS, it is also applicable to general EFSs. The proposed method is illustrated with Box-Jenkins gas furnace problem, one nonlinear system identification problem, Mackey-Glass time series prediction problem, eight real-world benchmark regression problems as well as a high-frequency trading prediction problem. Compared with other EFSs, the numerical examples show that the considered EFS in this paper provides guaranteed stability as well as a better approximation accuracy.
In this paper, a new deep rule-based approach using high-level ensemble feature descriptor is proposed for aerial scene classification. By creating an ensemble of three pre-trained deep convolutional neural networks for feature extraction, the proposed approach is able to extract more discriminative representations from the local regions of aerial images. With a set of massively parallel IF ... THEN rules built upon the prototypes identified through a self-organizing, nonparametric, transparent and highly human-interpretable learning process, the proposed approach is able to produce the state-of-the-art classification results on the unlabeled images outperforming the alternatives. Numerical examples on benchmark datasets demonstrate the strong performance of the proposed approach.
Pioneering the traditional fuzzy rule-based (FRB) systems, deep rule-based (DRB) classifiers are able to offer both human-level performance and transparent system structure on image classification problems by integrating zero-order fuzzy rule base with a multi-layer image-processing architecture that is typical for deep learning. Nonetheless, it is frequently observed that the inner structure of DRB can become over sophisticated and not interpretable for humans when applied to large-scale, complex problems. To tackle the issue, one feasible solution is to construct a tree structural classification model by aggregating the possibly huge number of prototypes identified from data into a much smaller number of more descriptive and highly abstract ones. Therefore, in this paper, we present a novel hierarchical deep rule-based (H-DRB) approach that is capable of summarizing the less descriptive raw prototypes into highly generalized ones and self-arranging them into a hierarchical prototype-based structure according to their descriptive abilities. By doing so, H-DRB can offer high-level performance and, most importantly, full transparency and human-interpretability on various problems including large-scale ones. The proposed concept and generical principles are verified through numerical experiments based on a wide variety of popular benchmark image sets. Numerical results demonstrate that the promise of H-DRB. •A generic approach for deep rule-based systems to self-organize a multi-layer structure is proposed.•The proposed system can offer higher transparency and human-interpretability for large-scale, complex problems.•The proposed approach can perform highly efficient decision-making with attractive classification precision.•The effectiveness and validity of the proposed approach are demonstrated on a variety of popular benchmark image sets.
It is widely recognized that learning systems have to go deeper to exchange for more powerful representational learning capabilities in order to precisely approximate nonlinear complex problems. However, the best-known computational intelligence approaches with such characteristics, namely, deep neural networks, are often criticized for lacking transparency. In this article, a novel multilayer evolving fuzzy neural network (MEFNN) with a transparent system structure is proposed. The proposed MEFNN is a metalevel stacking ensemble learning system composed of multiple cascading evolving neuro-fuzzy inference systems (ENFISs), processing input data layer-by-layer to automatically learn multilevel nonlinear distributed representations from data. Each ENFIS is an evolving fuzzy system capable of learning from new data sample by sample to self-organize a set of human-interpretable IF- THEN fuzzy rules that facilitate approximate reasoning. Adopting ENFIS as its ensemble component, the multilayer system structure of the MEFNN is flexible and transparent, and its internal reasoning and decision-making mechanism can be explained and interpreted to/by humans. To facilitate information exchange between different layers and attain stronger representation learning capability, the MEFNN utilizes error backpropagation to self-update the consequent parameters of the IF-THEN rules of each ensemble component based on the approximation error propagated backward. To enhance the capability of the MEFNN to handle complex problems, a nonlinear activation function is introduced to modeling the consequent parts of the IF-THEN rules of ENFISs, thereby empowering both the representation and the reflection of nonlinearity in the resulting fuzzy outputs. Numerical examples on a wide variety of challenging (benchmark and real-world) classification and regression problems demonstrate the superior practical performance of the MEFNN, revealing the effectiveness and validity of the proposed approach.
In this paper, a detailed mathematical analysis of the optimality of the premise and consequent parts of the recently introduced first-order Autonomous Learning Multi-Model (ALMMo) neuro-fuzzy system is conducted. A novel self-boosting algorithm for structure- and parameter-optimization is, then, introduced to the ALMMo, which results in the self-boosting ALMMo (SBALMMo) neuro-fuzzy system. By minimizing the objective functions with the previously collected data, the SBALMMo is able to optimize its system structure and parameters in few iterations. Numerical examples based benchmark datasets and real-world problems demonstrate the effectiveness and validity of the SBALMMo, and show the strong potential of the proposed approach for real applications. •A systematic analysis of the optimality of the ALMMo neuro-fuzzy system is conducted.•A novel self-boosting algorithm for structure and parameter-optimization is proposed.•The self-boosting ALMMo (SBALMMo) neuro-fuzzy system is introduced.•The effectiveness and validity of the SBALMMo are demonstrated on real world problems.
New satellite remote sensing and machine learning techniques offer untapped possibilities to monitor global biodiversity with unprecedented speed and precision. These efficiencies promise to reveal novel ecological insights at spatial scales which are germane to the management of populations and entire ecosystems. Here, we present a robust transferable deep learning pipeline to automatically locate and count large herds of migratory ungulates (wildebeest and zebra) in the Serengeti-Mara ecosystem using fine-resolution (38-50 cm) satellite imagery. The results achieve accurate detection of nearly 500,000 individuals across thousands of square kilometers and multiple habitat types, with an overall F1-score of 84.75% (Precision: 87.85%, Recall: 81.86%). This research demonstrates the capability of satellite remote sensing and machine learning techniques to automatically and accurately count very large populations of terrestrial mammals across a highly heterogeneous landscape. We also discuss the potential for satellite-derived species detections to advance basic understanding of animal behavior and ecology.
Adaptive boosting (AdaBoost) is a widely used technique to construct a stronger ensemble classifier by combining a set of weaker ones. Zero-order fuzzy inference systems (FISs) are very powerful prototype-based predictive models for classification, offering both great prediction precision and high user interpretability. However, the use of zero-order FISs as base classifiers in AdaBoost has not been explored yet. To bridge the gap, in this article, a novel multiclass fuzzily weighted AdaBoost (FWAdaBoost)-based ensemble system with a self-organizing fuzzy inference system (SOFIS) as the ensemble component is proposed. To better incorporate the SOFIS, FWAdaBoost utilizes the confidence scores produced by the SOFIS in both sample weight updating and ensemble output generation, resulting in more accurate classification boundaries and greater prediction precision. Numerical examples on a wide range of benchmark classification problems demonstrate the efficacy of the proposed approach.
In this paper, a novel hierarchical prototype-based approach for classification is proposed. This approach is able to perceive the data space and derive the multimodal distributions from streaming data at different levels of granularity in an online manner, based on which it further identifies meaningful prototypes to self-organize and self-evolve its hierarchical structure for classification. Thanks to the prototype-based nature, the system structure of the proposed classifier is highly transparent, and its learning process is of “one pass” type and computationally lean. Its decision-making process follows the “nearest prototype” principle and is fully explainable. The proposed approach is capable of presenting the learned knowledge from data in an easy-to-interpret prototype-based hierarchical form to users, and is an attractive tool for solving large-scale, complex real-world problems. Numerical examples based on various benchmark problems justify the validity and effectiveness of the proposed concept and general principles.
This study proposes to construct a model using random forest method, an efficient machine learning-based method, to predict the spatial structure and temporal evolution of the sea surface temperature (SST) cooling induced by northwest Pacific tropical cyclones (TCs), a process of the so-called wind pump. The predictors in use include 12 predictors related to TC characteristics and pre-storm ocean conditions. The model is shown to skillfully predict the spatiotemporal evolutions of the cold wake generated by TCs of different intensity groups, and capture the cross-case variance in the observed SST response. Another model is further built based on the same method to assess the relative importance of the 12 predictors in determining the magnitude of the maximum cooling. Computations of feature scores of those predictors show that TC intensity, translation speed and size, and pre-storm mixed layer depth and SST dominate, depending on the area where the cooling is considered. While many studies have been devoted to understanding the processes and mechanisms underlying the sea surface temperature (SST) cooling induced by tropical cyclones (TCs), few studies have attempted to predict the spatial and temporal evolution of the sea surface temperature (SST) cooling triggered by TCs. In this study, we proposed to achieve this goal by building a model using an efficient and robust machine learning-based method. The constructed model uses 12 predictors associated with TC characteristics (e.g., intensity, and translation speed) and pre-storm ocean states (e.g., mixed layer depth). The model performs well in producing the TC-induced spatial structure and temporal evolution of the cold wake and can capture most of the variance in the observed SST response. We quantified the relative importance of the 12 predictors, and found that TC intensity, translation speed and size, and pre-storm mixed layer depth and SST dominate in deciding the magnitude of the SST response. The results and proposed method have important implications for predicting the response of ocean primary production to the TC wind pump effects. A machine learning-based model is built to predict the spatiotemporal evolution of the tropical cyclone-induced sea surface temperature responseThe model well predicts the spatial structure and temporal evolution of the observed response and captures the observed cross-case varianceFeature scores are computed to assess the relative importance of the predictors in determining the magnitude of the SST response
In this paper, a novel empirical data analysis approach (abbreviated as EDA) is introduced which is entirely data-driven and free from restricting assumptions and pre-defined problem- or user-specific parameters and thresholds. It is well known that the traditional probability theory is restricted by strong prior assumptions which are often impractical and do not hold in real problems. Machine learning methods, on the other hand, are closer to the real problems but they usually rely on problem- or user-specific parameters or thresholds making it rather art than science. In this paper we introduce a theoretically sound yet practically unrestricted and widely applicable approach that is based on the density in the data space. Since the data may have exactly the same value multiple times we distinguish between the data points and unique locations in the data space. The number of data points, k is larger or equal to the number of unique locations, l and at least one data point occupies each unique location. The number of different data points that have exactly the same location in the data space (equal value), f can be seen as frequency. Through the combination of the spatial density and the frequency of occurrence of discrete data points, a new concept called multimodal typicality, τ MM is proposed in this paper. It offers a closed analytical form that represents ensemble properties derived entirely from the empirical observations of data. Moreover, it is very close (yet different) from the histograms, from the probability density function (pdf) as well as from fuzzy set membership functions. Remarkably, there is no need to perform complicated pre-processing like clustering to get the multimodal representation. Moreover, the closed form for the case of Euclidean, Mahalanobis type of distance as well as some other forms (e.g. cosine-based dissimilarity) can be expressed recursively making it applicable to data streams and online algorithms. Inference/estimation of the typicality of data points that were not present in the data so far can be made. This new concept allows to rethink the very foundations of statistical and machine learning as well as to develop a series of anomaly detection, clustering, classification, prediction, control and other algorithms.
Prototype-based approaches generally provide better explainability and are widely used for classification. However, the majority of them suffer from system obesity and lack transparency on complex problems. In this paper, a novel classification approach with a multi-layered system structure self-organized from data is proposed. This approach is able to identify local peaks of multi-modal density derived from static data and filter out more representative ones at multiple levels of granularity acting as prototypes. These prototypes are then optimized to their locally optimal positions in the data space and arranged in layers with meaningful dense links in-between to form pyramidal hierarchies based on the respective levels of granularity accordingly. After being primed offline, the constructed classification model is capable of self-developing continuously from streaming data to self-expend its knowledge base. The proposed approach offers higher transparency and is convenient for visualization thanks to the hierarchical nested architecture. Its system identification process is objective, data-driven and free from prior assumptions on data generation model with user- and problem- specific parameters. Its decision-making process follows the “nearest prototype” principle, and is highly explainable and traceable. Numerical examples on a wide range of benchmark problems demonstrate its high performance.
In this chapter, a new type of deep rule-based (DRB) classifier with a multi-layer architecture is presented for image classification, which combines the computer vision techniques with a massively parallel set of zero-order fuzzy rules as its learning engine. With its prototype-based nature, the DRB classifiers are able to identify a transparent and human-understandable fuzzy rule-based (FRB) system structure from the data through an autonomous, non-iterative, non-parametric and highly parallel online learning process, and offer extremely high classification accuracy. The DRB classifier can start “from scratch”, and conduct classification from the very first image of each class in the same way as humans do. The DRB classifier can also learn in a semi-supervised mode initialized with only a small proportion of the labelled data and continue in a fully unsupervised mode after that. The ability of semi-supervised learning further allows the DRB classifier to learn new classes actively without human experts’ involvement. Thanks to the prototype-based nature of the DRB classifier, it is free from prior assumptions about the type of the data distribution, their random or deterministic nature, and there are no requirements to make ad hoc decisions. Its supervised and semi-supervised learning processes are fully transparent and human-interpretable. The semi-supervised DRB classifiers can perform classification on out-of-sample images and also support recursive online training on a sample-by-sample basis or a batch-by-batch basis.
In this chapter, we will describe the fundamentals of the proposed new “empirical” approach as a systematic methodology with its nonparametric quantities derived entirely from the actual data with no subjective and/or problem-specific assumptions made. It has a potential to be a powerful extension of (and/or alternative to) the traditional probability theory, statistical learning and computational intelligence methods. The nonparametric quantities of the proposed new empirical approach include: (1) the cumulative proximity; (2) the eccentricity, and the standardized eccentricity; (3) the data density, and (4) the typicality. They can be recursively updated on a sample-by-sample basis, and they have unimodal and multimodal, discrete and continuous forms/versions. The nonparametric quantities are based on ensemble properties of the data and not limited by prior restrictive assumptions. The discrete version of the typicality resembles the unimodal probability density function, but is in a discrete form. The discrete multimodal typicality resembles the probability mass function.
In this paper, a fast, transparent, self-evolving, deep learning fuzzy rule-based (DLFRB) image classifier is proposed. This new classifier is a cascade of the recently introduced DLFRB classifier called MICE and an auxiliary SVM. The DLFRB classifier serves as the main engine and can identify a number of human interpretable fuzzy rules through a very short, transparent, highly parallelizable training process. The SVM based auxiliary plays the role of a conflict resolver when the DLFRB classifier produces two highly confident labels for a single image. Only the fundamental image transformation techniques (rotation, scaling and segmentation) and feature descriptors (GIST and HOG) are used for pre-processing and feature extraction, but the proposed approach significantly outperforms the state-of-art methods in terms of both time and precision. Numerical experiments based on a handwritten digits recognition problem are used to demonstrate the highly accurate and repeatable performance of the proposed approach.
•A novel semi-supervised deep rule-based (SSDRB) classifier with a prototype-based nature is introduced.•The semi-supervised learning process of the SSDRB classifier is self-organising and highly transparent.•The SSDRB classifier is able to generate human interpretable IF...THEN... rules.•The SSDRB classifier is able to perform classification on out-of-sample images.•The SSDRB classifier outperforms the state-of-art approaches in classification accuracy. In this paper, a semi-supervised learning approach based on a deep rule-based (DRB) classifier is introduced. With its unique prototype-based nature, the semi-supervised DRB (SSDRB) classifier is able to generate human interpretable IF...THEN...rules through the semi-supervised learning process in a self-organising and highly transparent manner. It supports online learning on a sample-by-sample basis or on a chunk-by-chunk basis. It is also able to perform classification on out-of-sample images. Moreover, the SSDRB classifier can learn new classes from unlabelled images in an active way becoming dynamically self-evolving. Numerical examples based on large-scale benchmark image sets demonstrate the strong performance of the proposed SSDRB classifier as well as its distinctive features compared with the “state-of-the-art” approaches.
In this paper, a new type of multilayer rule-based classifier is proposed and applied to image classification problems. The proposed approach is entirely data-driven and fully automatic. It is generic and can be applied to various classification and prediction problems, but in this paper we focus on image processing, in particular. The core of the classifier is a fully interpretable, understandable, self-organized set of IF...THEN... fuzzy rules based on the prototypes autonomously identified by using a one-pass type training process. The classifier can self-evolve and be updated continuously without a full retraining. Due to the prototype-based nature, it is non-parametric; its training process is non-iterative, highly parallelizable and computationally efficient. At the same time, the proposed approach is able to achieve very high classification accuracy on various benchmark datasets surpassing most of the published methods, be comparable with the human abilities. In addition, it can start classification from the first image of each class in the same way as humans do, which makes the proposed classifier suitable for real-time applications. Numerical examples of benchmark image processing demonstrate the merits of the proposed approach. (C) 2018 Elsevier Inc. All rights reserved.
In this article, a new data-driven autonomous fuzzy clustering (AFC) algorithm is proposed for static data clustering. Employing a Gaussian-type membership function, AFC first uses all the data samples as microcluster medoids to assign memberships to each other and obtains the membership matrix. Based on this, AFC chooses these data samples that represent local models of data distribution as cluster medoids for initial partition. It then continues to optimize the cluster medoids iteratively to obtain a locally optimal partition as the algorithm output. Moreover, an online extension is introduced to AFC enabling the algorithm to cluster streaming data chunk-by-chunk in a "one pass" manner. Numerical examples based on a variety of benchmark problems demonstrate the efficacy of the AFC algorithm in both offline and online application scenarios, proving the effectiveness and validity of the proposed concept and general principles.
In recent years, numerous techniques have been proposed for human activity recognition (HAR) from images and videos. These techniques can be divided into two major categories: handcrafted and deep learning. Deep Learning-based models have produced remarkable results for HAR. However, these models have several shortcomings, such as the requirement for a massive amount of training data, lack of transparency, offline nature, and poor interpretability of their internal parameters. In this paper, a new approach for HAR is proposed, which consists of an interpretable, self-evolving, and self-organizing set of 0-order If...THEN rules. This approach is entirely data-driven, and non-parametric; thus, prototypes are identified automatically during the training process. To demonstrate the effectiveness of the proposed method, a set of high-level features is obtained using a pre-trained deep convolution neural network model, and a recently introduced deep rule-based classifier is applied for classification. Experiments are performed on a challenging benchmark dataset UCF50; results confirmed that the proposed approach outperforms state-of-the-art methods. In addition to this, an ablation study is conducted to demonstrate the efficacy of the proposed approach by comparing the performance of our DRB classifier with four state-of-the-art classifiers. This analysis revealed that the DRB classifier could perform better than state-of-the-art classifiers, even with limited training samples.
Future intelligent machines will be more human-friendly and human-like, while offering much higher throughput and automation, thus augmenting our (human) capabilities. Anthropomorphic machine learning is an emerging direction for future development in artificial intelligence (AI) and data science. This revolutionary shift offers human-like abilities to the next generation of machine learning with greater potential for underpinning breakthroughs in technology development as well as in various aspects of everyday life.
In this paper, we propose an approach to data analysis, which is based entirely on the empirical observations of discrete data samples and the relative proximity of these points in the data space. At the core of the proposed new approach is the typicalityan empirically derived quantity that resembles probability. This nonparametric measure is a normalized form of the square centrality (centrality is a measure of closeness used in graph theory). It is also closely linked to the cumulative proximity and eccentricity (a measure of the tail of the distributions that is very useful for anomaly detection and analysis of extreme values). In this paper, we introduce and study two types of typicality, namely its local and global versions. The local typicality resembles the well-known probability density function (pdf), probability mass function, and fuzzy set membership but differs from all of them. The global typicality, on the other hand, resembles well-known histograms but also differs from them. A distinctive feature of the proposed new approach, empirical data analysis (EDA), is that it is not limited by restrictive impractical prior assumptions about the data generation model as the traditional probability theory and statistical learning approaches are. Moreover, it does not require an explicit and binary assumption of either randomness or determinism of the empirically observed data, their independence, or even their number (it can be as low as a couple of data samples). The typicality is considered as a fundamental quantity in the pattern analysis, which is derived directly from data and is stated in a discrete form in contrast to the traditional approach where a continuous pdf is assumed a priori and estimated from data afterward. The typicality introduced in this paper is free from the paradoxes of the pdf. Typicality is objectivist while the fuzzy sets and the belief-based branch of the probability theory are subjectivist. The local typicality is expressed in a closed analytical form and can be calculated recursively, thus, computationally very efficiently. The other nonparametric ensemble properties of the data introduced and studied in this paper, namely, the square centrality, cumulative proximity, and eccentricity, can also be updated recursively for various types of distance metrics. Finally, a new type of classifier called naive typicality-based EDA class is introduced, which is based on the newly introduced global typicality. This is only one of the wide range of possible applications of EDA including but not limited for anomaly detection, clustering, classification, control, prediction, control, rare events analysis, etc., which will be the subject of further research.
Remote sensing scene classification plays a critical role in a wide range of real-world applications. Technically, however, scene classification is an extremely challenging task due to the huge complexity in remotely sensed scenes, and the difficulty in acquiring labelled data for model training such as supervised deep learning. To tackle these issues, a novel semi-supervised ensemble framework is proposed here using the self-training hierarchical prototype-based classifier as the base learner for chunk-by-chunk prediction. The framework has the ability to build a powerful ensemble model from both labelled and unlabelled images with minimum supervision. Different feature descriptors are employed in the proposed ensemble framework to offer multiple independent views of images. Thus, the diversity of base learners is guaranteed for ensemble classification. To further increase the overall accuracy, a novel cross-checking strategy was introduced to enable the base learners to exchange pseudolabelling information during the self-training process, and maximize the correctness of pseudo-labels assigned to unlabelled images. Extensive numerical experiments on popular benchmark remote sensing scenes demonstrated the effectiveness of the proposed ensemble framework, especially where the number of labelled images available is limited. For example, the classification accuracy achieved on the OPTIMAL-31, PatternNet and RSI-CB256 datasets was up to 99.91%, 98. 67% and 99.07% with only 40% of the image sets used as labelled training images, surpassing or at least on par with mainstream benchmark approaches trained with double the number of labelled images.
In this chapter, an overview of the theory of probability, statistical and machine learning is made covering the main ideas and the most popular and widely used methods in this area. As a starting point, the randomness and determinism as well as the nature of the real-world problems are discussed. Then, the basic and well-known topics of the traditional probability theory and statistics including the probability mass and distribution, probability density and moments, density estimation, Bayesian and other branches of the probability theory, are recalled followed by a analysis. The well-known data pre-processing techniques, unsupervised and supervised machine learning methods are covered. These include a brief introduction of the distance metrics, normalization and standardization, feature selection, orthogonalization as well as a review of the most representative clustering, classification, regression and prediction approaches of various types. In the end, the topic of image processing is also briefly covered including the popular image transformation techniques, and a number of image feature extraction techniques at three different levels.
In this paper, a novel approach to the self-organization of hierarchical prototype-based classifiers from data is proposed. The approach recursively partitions the data at multiple levels of granularity into shape-free clusters of different sizes, resembling Voronoi tessellation, and naturally aggregates the resulting cluster medoids into a multi-layered prototype-based structure according to their descriptive abilities. Different from conventional classification models, it is nonparametric and entirely data-driven, and the learned model can offer a high-level of transparency and interpretability thanks to the underlying prototype-based nature. The system identification process underpinning the approach is driven by the aim of separating data samples of different classes into nonoverlapping multi-granular clusters. Its associated decision-making process follows the “nearest prototype” principle and hence, the rationales of the subsequent decisions made can be explicitly explained. Experimental studies based on popular benchmark classification problems, as well as on a practical application to remote sensing image classification, demonstrate the efficacy of the proposed approach.
An estimation method of pseudo-random (PN) codes in the periodic long code direct sequence spread spectrum signals using a pair of spreading code and scrambling code [i.e. long scrambling code direct sequence spread spectrum (LSC-DSSS)] is investigated in this study. Via the investigation of properties of triple correlation function (TCF) of m-sequences, the existence of common peaks in the TCFs of different m-sequences is proved, and the corresponding relationship between common peaks and primitive polynomials is further investigated. Four theorems are proposed as supplements of triple correlation theory and a novel estimation algorithm of the PN codes in LSC-DSSS signals is put forward on the basis of the theorems. With certain carrier frequency and chip rate of spreading code, this algorithm first eliminates the influence of information codes through delay-and-multiply operation. Then the TCF of signal is calculated, and the two PN codes in signal are successfully estimated finally by searching and using the common peak coordinates in the TCF. Simulation results show that the proposed algorithm exhibits excellent performance in estimating PN codes in LSC-DSSS signals.
Semi-supervised learning from data streams is widely considered as a highly challenging task to be further researched. In this paper, a novel dual-model self-organizing fuzzy inference system composed of two recently introduced evolving fuzzy systems (EFSs) is proposed for semi-supervised learning from data streams in infinite delay environments. After being primed with a small amount of labelled data during the warm-up period, the proposed model is able to continuously self-learn and self-expand its knowledge base from unlabelled data on a chunk-by-chunk basis with minimal human expert involvement. Thanks to its dual-model structure, the proposed model combines the merits of the two EFS models such that it can continuously identify new prototypes from new pseudo-labelled data to self-improve its knowledge base whilst keeping the impact of pseudo-labelled errors on its decision-making minimized. Numerical examples based on various benchmark problems demonstrate the efficacy of the proposed method, showing its strong potential in real-world applications by offering higher classification accuracy over the state-of-the-art competitors whilst retaining high computational efficiency. •A dual-model semi-supervised system is proposed for data stream classification in infinite label delay scenarios.•A unique semi-supervised learning strategy is designed for its dual-model structure.•The system continuously self-improves its knowledge base from unlabelled streaming data via pseudo-labelling.
This chapter provides a summary of the empirical approaches introduced in this book and further gives the direction of future works.
In this paper, we offer a method aiming to minimize the role of distance metric used in clustering. It is well known that distance metrics used in clustering algorithms heavily influence the end results and also make the algorithms sensitive to imbalanced attribute/feature scales. To solve these problems, a new clustering algorithm using a per-attribute/feature ranking operating mechanism is proposed in this paper. Ranking is a rarely used discrete, nonlinear operator by other clustering algorithms. However, it also has unique advantages over the dominantly used continuous operators. The proposed algorithm is based on the ranks of the data samples in terms of their spatial separation and is able to provide a more objective clustering result compared with the alternative approaches. Numerical examples on benchmark datasets prove the validity and effectiveness of the proposed concept and principles. •A new method is proposed to minimize the role of distance metric used in clustering.•This method employs the rarely-used ranking operator as the “core”.•It is insensitive to the type of distance metric that is used for clustering.•It is insensitive to the imbalanced attribute scales.•It is able to provide a more objective clustering result compared with the alternatives.
Ensemble learning is a widely used methodology to build powerful predictors from multiple individual weaker ones. However, the vast majority of ensemble learning models are designed for offline application scenarios, the use of evolving fuzzy systems in ensemble learning for online learning from data streams has not been sufficiently explored, yet. In this paper, a novel self-adaptive fuzzy learning ensemble system is introduced for data stream prediction. The proposed ensemble system employs the very sparse random projection technique to compress the consequent parts of the learned fuzzy rules by individual base models to a more compressed form, thereby reducing redundant information and improving computational efficiency. To improve the overall prediction performance, a dynamical base model pruning scheme is introduced to the proposed ensemble system together with a novel inferencing scheme, such that less accurate base models will be removed from the ensemble structure at each learning cycle automatically and only these more accurate ones will be involved in joint decision-making. Numerical examples based on a wide range of benchmark datasets demonstrate the stronger prediction performance of the proposed ensemble system over the state-of-the-art alternatives.
Analyzing and predicting the high frequency trading (HFT) financial data stream is very challenging due to the fast arrival times and large amount of the data samples. Aiming at solving this problem, an online evolving fuzzy rule-based prediction model is proposed in this paper. Because this prediction model is based on evolving fuzzy rule-based systems and a novel, simpler form of data density, it can autonomously learn from the live data stream, automatically build/remove its rules and recursively update the parameters. This model responds quickly to all unpredictable sudden changes of financial data and re-adjusts itself to follow the new data pattern. Experimental results show the excellent prediction performance of the proposed approach with real financial data stream regardless of quick shifts of data patterns and frequent appearances of abnormal data samples.
In this paper, a new type of fast deep learning (DL) network for handwriting recognition is proposed. In contrast to the existing DL networks, the proposed approach has a clearly interpretable structure that is entirely data- driven and free from user- or problem-specific assumptions. Firstly, the fundamental image transformation techniques (rotation and scaling) used by other existing DL methods are used to improve the generalization. The commonly used descriptors are then used to extract the global features from the training set and based on them a bank/ensemble of zero order AnYa type fuzzy rule-based (FRB) models is built in parallel through the recently introduced Autonomous Learning Multiple Model (ALMMo) method. The final decision about the winning class label is made by a committee on the basis of the fuzzy mixture of the trained zero order ALMMo models. The training of the proposed MICE system is very efficient and highly parallelizable. It significantly outperforms the best-known methods in terms of time and is on par in terms of precision/accuracy. Critically, it offers a high level of interpretability, transparency of the classification model, full repeatability (unlike the methods that use probabilistic elements) of the results. Moreover, it allows an evolving scenario whereby the data is provided in an incremental, online manner and the system structure evolves in parallel with the classification which opens opportunities for online and real-time applications (on a sample by sample basis). Numerical examples from the well-known handwritten digits recognition problem (MNIST) were used and the results demonstrated the very high repeatable performance after a very short training process exhibiting high level of interpretability, transparency.
In order to tackle high dimensional, complex problems, learning models have to go deeper. In this article, a novel multilayer ensemble learning model with first-order evolving fuzzy systems as its building blocks is introduced. The proposed approach can effectively learn from streaming data on a sample-by-sample basis and self-organizes its multilayered system structure and meta-parameters in a feedforward, noniterative manner. Benefiting from its multilayered distributed representation learning ability, the ensemble system not only demonstrates the state-of-the-art performance on various problems, but also offers high level of system transparency and explainability. Theoretical justifications and experimental investigation show the validity and effectiveness of the proposed concept and general principles.
Large-scale (large-area), fine spatial resolution satellite sensor images are valuable data sources for Earth observation while not yet fully exploited by research communities for practical applications. Often, such images exhibit highly complex geometrical structures and spatial patterns, and distinctive characteristics of multiple land-use categories may appear at the same region. Autonomous information extraction from these images is essential in the field of pattern recognition within remote sensing, but this task is extremely challenging due to the spectral and spatial complexity captured in satellite sensor imagery. In this research, a semi-supervised deep rule-based approach for satellite sensor image analysis (SeRBIA) is proposed, where large-scale satellite sensor images are analysed autonomously and classified into detailed land-use categories. Using an ensemble feature descriptor derived from pre-trained AlexNet and VGG-VD-16 models, SeRBIA is capable of learning continuously from both labelled and unlabelled images through self-adaptation without human involvement or intervention. Extensive numerical experiments were conducted on both benchmark datasets and real-world satellite sensor images to comprehensively test the validity and effectiveness of the proposed method. The novel information mining technique developed here can be applied to analyse large-scale satellite sensor images with high accuracy and interpretability, across a wide range of real-world applications.
In this paper, we introduce a new form of describing fuzzy sets (FSs) and a new form of fuzzy rule-based (FRB) systems, namely, empirical fuzzy sets (epsilon FSs) and empirical fuzzy rule-based (epsilon FRB) systems. Traditionally, the membership functions (MFs), which are the key mathematical representation of FSs, are designed subjectively or extracted from the data by clustering projections or defined subjectively. epsilon FSs, on the contrary, are described by the empirically derived membership functions (epsilon MFs). The new proposal made in this paper is based on the recently introduced Empirical Data Analytics (EDA) computational framework and is closely linked with the density of the data. This allows to keep and improve the link between the objective data and the subjective labels, linguistic terms, and classes definition. Furthermore, epsilon FSs can deal with heterogeneous data combining categorical with continuous and/or discrete data in a natural way. epsilon FRB systems can be extracted from data including data streams and can have dynamically evolving structure. However, they can also be used as a tool to represent expert knowledge. The main difference from the traditional FSs and FRB systems is that the expert does not need to define the MF per variable; instead, possibly multimodal, densities will be extracted automatically from the data and used as epsilon MFs in a vector form for all numerical variables. This is done in a seamless way whereby the human involvement is only required to label the classes and linguistic terms. Moreover, even this intervention is optional. Thus, the proposed new approach to define and design the FSs and FRB systems puts the human in the driving seat. Instead of asking experts to define features and MFs correspondingly, to parameterize them, to define algorithm parameters, to choose types of MFs, or to label each individual item, it only requires (optionally) to select prototypes from data and (again, optionally) to label them. Numerical examples as well as a naive empirical fuzzy (epsilon F) classifier are presented with an illustrative purpose. Due to the very fundamental nature of the proposal, it can have a very wide area of applications resulting in a series of new algorithms such as epsilon F classifiers, epsilon F predictors, epsilon F controllers, and so on. This is left for the future research. (C) 2017 Wiley Periodicals, Inc.
In this paper, we present a self-organising nonparametric fuzzy rule-based classifier. The proposed approach identifies prototypes from the observed data through an offline training process and uses them to build a 0-order AnYa type fuzzy rule-based system for classification. Once primed offline, it is able to continuously learn from the streaming data afterwards to follow the changing data pattern by updating the system structure and meta parameters recursively. The meta-parameters of the proposed approach are derived from data directly. By changing the level of granularity, the proposed approach can make a trade-off between performance and computational efficiency, and, thus, the classifier is able to address a wide variety of problems with specific needs. The classifier also supports different types of distance measures. Numerical examples based on benchmark datasets demonstrate the high performance of the proposed approach and its ability of handling high-dimensional, complex, large-scale problems. (C) 2018 Elsevier Inc. All rights reserved.
A novel self-organizing fuzzy proportional-integral-derivative (SOF-PID) control system is proposed in this paper. The proposed system consists of a pair of control and reference models, both of which are implemented by a first-order autonomous learning multiple model (ALMMo) neuro-fuzzy system. The SOF-PID controller self-organizes and self-updates the structures and meta-parameters of both the control and reference models during the control process "on the fly". This gives the SOF-PID control system the capability of quickly adapting to entirely new operating environments without a full re-training. Moreover, the SOF-PID control system is free from user- and problem-specific parameters and is entirely data-driven. Simulations and real-world experiments with mobile robots demonstrate the effectiveness and validity of the proposed SOF-PID control system.
Optimality of the premise, IF part is critical to a zero-order evolving intelligent system (EIS) because this part determines the validity of the learning results and overall system performance. Nonetheless, a systematic analysis of optimality has not been done yet in the state-of-the-art works. In this paper, we use the recently introduced self-organising neuro-fuzzy inference system (SONFIS) as an example of typical zero-order EISs and analyse the local optimality of its solutions. The optimality problem is firstly formulated in a mathematical form, and detailed optimality analysis is conducted. The conclusion is that SONFIS does not generate a locally optimal solution in its original form. Then, an optimisation method is proposed for SONFIS, which helps the system to attain local optimality in a few iterations using historical data. Numerical examples presented in this paper demonstrate the validity of the optimality analysis and the effectiveness of the proposed optimisation method. In addition, it is further verified numerically that the proposed concept and general principles can be applied to other types of zero-order EISs with similar operating mechanisms.
The antecedent and consequent parts of a first-order evolving intelligent system (EIS) determine the validity of the learning results and overall system performance. Nonetheless, the state-of-the-art techniques mostly stress on the novelty from the system identification point of view but pay less attention to the optimality of the learned parameters. Using the recently introduced autonomous learning multiple model (ALMMo) system as the implementation basis, this article introduces a particle swarm-based approach for the EIS optimization. The proposed approach is able to simultaneously optimize the antecedent and consequent parameters of ALMMo and effectively enhance the system performance by iteratively searching for optimal solutions in the problem spaces. In addition, the proposed optimization approach does not adversely influence the "one pass" learning ability of ALMMo. Once the optimization process is complete, ALMMo can continue to learn from new data to incorporate unseen data patterns recursively without full retraining. The experimental studies with a number of real-world benchmark problems validate the proposed concept and general principles. It is also verified that the proposed optimization approach can be applied to other types of EISs with similar operating mechanisms.
In this chapter, the Autonomous Learning Multi-Model (ALMMo) systems are introduced, which are based on the AnYa type neuro-fuzzy systems and can be seen as an universal self-developing, self-evolving, stable, locally optimal proven universal approximators. This chapter starts with the general concepts and principles of the zero- and first-order ALMMo systems, and, then, describes the architecture followed by the learning methods. The ALMMo system does not impose generation models with parameters on the empirically observed data, and has the advantages of being non-parametric, non-iterative and assumption-free, and, thus, it can objectively disclose the underlying data pattern. With a prototype-based nature, the ALMMo system is able to self-develop, self-learn and evolve autonomously. The theoretical proof (using Lyapunov theorem) of the stability of the first-order ALMMo systems is provided demonstrating that the first-order ALMMo systems are also stable. The theoretical proof of the local optimality which satisfies Karush-Kuhn-Tucker conditions is also given.
In this chapter, the concepts and general principles of the empirical fuzzy sets and the fuzzy rule-based (FRB) systems based on them, named empirical FRB systems are presented, and two approaches for identifying empirical FRB systems, namely, the subjective one, which is based on human expertise, and the objective one, which is based on the autonomous data partitioning algorithm, are also presented. The traditional fuzzy sets and systems suffer from the so-called “curse of dimensionality”, they heavily rely on ad hoc decision and lack objectiveness. In contrast, the empirical approach to identify the empirical fuzzy sets and FRB systems effectively combine the data- and human-derived models and minimize the involvement of human expertise. They have significant advantages over the traditional ones because of the very strong interpretability, high objectiveness, being data driven and free from prior assumptions.
In order to address high dimensional problems, a new 'direction-aware' metric is introduced in this paper. This new distance is a combination of two components: (1) the traditional Euclidean distance and (2) an angular/directional divergence, derived from the cosine similarity. The newly introduced metric combines the advantages of the Euclidean metric and cosine similarity, and is defined over the Euclidean space domain. Thus, it is able to take the advantage from both spaces, while preserving the Euclidean space domain. The direction-aware distance has wide range of applicability and can be used as an alternative distance measure for various traditional clustering approaches to enhance their ability of handling high dimensional problems. A new evolving clustering algorithm using the proposed distance is also proposed in this paper. Numerical examples with benchmark datasets reveal that the direction-aware distance can effectively improve the clustering quality of the k-means algorithm for high dimensional problems and demonstrate the proposed evolving clustering algorithm to be an effective tool for high dimensional data streams processing.
As one of the three pillars in computational intelligence, fuzzy systems are a powerful mathematical tool widely used for modelling nonlinear problems with uncertainties. Fuzzy systems take the form of linguistic IF-THEN fuzzy rules that are easy to understand for human. In this sense, fuzzy inference mechanisms have been developed to mimic human reasoning and decision-making. From a data analytic perspective, fuzzy systems provide an effective solution to build precise predictive models from imprecise data with great transparency and interpretability, thus facilitating a wide range of real-world applications. This paper presents a systematic review of modern methods for autonomously learning fuzzy systems from data, with an emphasis on the structure and parameter learning schemes of mainstream evolving, evolutionary, reinforcement learning-based fuzzy systems. The main purpose of this paper is to introduce the underlying concepts, underpinning methodologies, as well as outstanding performances of the state-of-the-art methods. It serves as a one-stop guide for readers learning the representative methodologies and foundations of fuzzy systems or who desire to apply fuzzy-based autonomous learning in other scientific disciplines and applied fields.
In this chapter, the algorithm summary of the main procedure of the deep rule-based (DRB) classifier described in Chap. 9 is provided. Numerical examples based on popular benchmark image sets including, handwritten digits recognition, remote sensing scene classification, face recognition and object recognition, etc., are presented for evaluating the performance of the DRB algorithm on image classification, and the state-of-the-art approaches are used for comparison. Numerical experiments show that DRB classifier is able to perform highly accurate classification in various image classification problems, and also demonstrate the advantages of its prototype-based nature and transparency over the existing approaches. The pseudo-code of the main procedure of the DRB classifier and the MATLAB implementations can be found in appendices B.5 and C.5, respectively.
In this chapter, a new empirical approach, named autonomous data partitioning, is proposed to partition the data autonomously by creating a Voronoi tessellation around the objectively identified prototypes to form data clouds, which transform the large amount of raw data into a much smaller (manageable) number of more representative aggregations with semantic meaning. The proposed empirical algorithm has two forms/types, namely, the offline version and the evolving version. The offline version is based on the ranks of the observations in terms of their multimodal typicality values and local ensemble properties. The evolving version is for streaming data processing and works with the data density. It is able to start “from scratch”, but can create a hybrid with the offline version as well. Moreover, an algorithm is proposed to guarantee the local optimality of the autonomous data partitioning approach allowing the proposed approach to end up with a locally optimal structure of data clouds represented by their focal points/prototypes, which is then ready to be used for analysis, building a multi-model classifier, predictor, controller or for fault isolation.
In this paper, a novel online clustering approach called Parallel_TEDA is introduced for processing high frequency streaming data. This newly proposed approach is developed within the recently introduced TEDA theory and inherits all advantages from it. In the proposed approach, a number of data stream processors are involved, which collaborate with each other efficiently to achieve parallel computation as well as a much higher processing speed. A fusion center is involved to gather the key information from the processors which work on chunks of the whole data stream and generate the overall output. The quality of the generated clusters is being monitored within the data processors all the time and stale clusters are being removed to ensure the correctness and timeliness of the overall clustering results. This, in turn, gives the proposed approach a stronger ability of handling shifts/drifts that may take place in live data streams. The numerical experiments performed with the proposed new approach Parallel_TEDA on benchmark datasets present higher performance and faster processing speed when compared with the alternative well-known approaches. The processing speed has been demonstrated to fall exponentially with more data processors involved. This new online clustering approach is very suitable and promising for real-time high frequency streaming processing and data analytics.
In this paper, an approach to autonomous learning of a multimodel system from streaming data, named ALMMo, is proposed. The proposed approach is generic and can easily be applied also to probabilistic or other types of local models forming multimodel systems. It is fully data driven and its structure is decided by the nonparametric data clouds extracted from the empirically observed data without making any prior assumptions concerning data distribution and other data properties. All metaparameters of the proposed system are obtained directly from the data and can be updated recursively, which improves memory and calculation efficiencies of the proposed algorithm. The structural evolution mechanism and online data cloud quality monitoring mechanism of the ALMMo system largely enhance the ability of handling shifts and/or drifts in the streaming data pattern. Numerical examples of the use of ALMMo system for streaming data analytics, classification, and prediction are presented as a proof of the proposed concept.
[Display omitted] •A multiscale superpixel reconstruction method was developed to generate the Difference Image.•A two-stage center-constrained FCM algorithm was designed to deal with imbalanced data clustering.•CWNN combined with DCGAN was adopted to classify hard pixels. Small area change detection using synthetic aperture radar (SAR) imagery is a highly challenging task, due to speckle noise and imbalance between classes (changed and unchanged). In this paper, a robust unsupervised approach is proposed for small area change detection using deep learning techniques. First, a multi-scale superpixel reconstruction method is developed to generate a difference image (DI), which can suppress the speckle noise effectively and enhance edges by exploiting local, spatially homogeneous information. Second, a two-stage centre-constrained fuzzy c-means clustering algorithm is proposed to divide the pixels of the DI into changed, unchanged and intermediate classes with a parallel clustering strategy. Image patches belonging to the first two classes are then constructed as pseudo-label training samples, and image patches of the intermediate class are treated as testing samples. Finally, a convolutional wavelet neural network (CWNN) is designed and trained to classify testing samples into changed or unchanged classes, coupled with a deep convolutional generative adversarial network (DCGAN) to increase the number of changed class within the pseudo-label training samples. Numerical experiments on four real SAR datasets demonstrate the validity and robustness of the proposed approach, achieving up to 99.61% accuracy for small area change detection.
In this paper, a novel self-adaptive fuzzy learning (SAFL) system is proposed for streaming data prediction. SAFL self-learns from data streams a predictive model composed of a set of prototype-based fuzzy rules, with each of which representing a certain local data distribution, and continuously self-evolves to follow the changing data patterns in non-stationary environments. Unlike conventional evolving fuzzy systems, both the fuzzy inference and consequent parameter learning schemes utilised by SAFL are simplified so that only a small number of selected fuzzy rules within the rule base are involved in system output generation and parameter updating during a learning cycle. Such simplification not only significantly reduces the system’s computational complexity but also increases its prediction precision. In addition, both theoretical and empirical investigations guarantee the stability of the resulting SAFL. Comparative experimental studies on a wide variety of benchmark and real-world problems demonstrate that SAFL is able to learn from streaming data in a highly efficient manner and to make predictions with a great accuracy, revealing the effectiveness and validity of the proposed approach.
This chapter provides a detailed introduction to the basic concepts and the general principles of the fuzzy sets and systems theory. Three major types of FRB systems are also covered and their differences are analyzed. The design of FRB systems is also covered. This chapter further moves on to the ANNs, which include the feedforward neural networks and three types of deep learning models. Both of the FRB systems and the ANNs have been proven universal approximators and can be designed based on the data. FRB systems have transparent, human-interpretable internal representation and can take advantage of the human domain expert knowledge. They are excellent in dealing with uncertainties, and they can self-organize, self-update both the structures and parameters in an online, dynamic environment. While ANNs are excellent in providing high precisions in most cases, they are fragile when facing new data patterns. They are typical examples of “black box” systems, their training process is usually limited to offline mode and requires huge amount of computation resources and data.
Evolving fuzzy systems (EFSs) are widely known as a powerful tool for streaming data prediction. In this article, a novel zero-order EFS with a unique belief structure is proposed for data stream classification. Thanks to this new belief structure, the proposed model can handle the interclass overlaps in a natural way and better capture the underlying multimodel structure of data streams in the form of prototypes. Utilizing data-driven soft thresholds, the proposed model self-organizes a set of prototype-based if - then fuzzy belief rules from data streams for classification, and its learning outcomes are practically meaningful. With no requirement of prior knowledge in the problem domain, the proposed model is capable of self-determining the appropriate level of granularity for rule-based construction, while enabling users to specify their preferences on the degree of fineness of its knowledge base. Numerical examples demonstrate the superior performance of the proposed model on a wide range of stationary and nonstationary classification benchmark problems.
Evolving intelligent systems (EISs), particularly, the zero-order ones have demonstrated strong performance on many real-world problems concerning data stream classification while offering high model transparency and interpretability thanks to their prototype-based nature. Zero-order EISs typically learn prototypes by clustering streaming data online in a "one pass" manner for greater computation efficiency. However, such identified prototypes often lack optimality, resulting in less precise classification boundaries, thereby hindering the potential classification performance of the systems. To address this issue, a commonly adopted strategy is to minimize the training error of the models on historical training data or alternatively to iteratively minimize the intracluster variance of the clusters obtained via online data partitioning. This recognizes the fact that the ultimate classification performance of zero-order EISs is driven by the positions of prototypes in the data space. Yet, simply minimizing the training error may potentially lead to overfitting while minimizing the intracluster variance does not necessarily ensure the optimized prototype-based models to attain improved classification outcomes. To achieve better classification performance while avoiding overfitting for zero-order EISs, this article presents a novel multiobjective optimization approach, enabling EISs to obtain optimal prototypes via involving these two disparate but complementary strategies simultaneously. Five decision-making schemes are introduced for selecting a suitable solution to deploy from the final nondominated set of the resulting optimized models. Systematic experimental studies are carried out to demonstrate the effectiveness of the proposed optimization approach in improving the classification performance of zero-order EISs.
Cloud removal in optical remote sensing imagery is essential for many Earth observation applications. To recover the cloud obscured information, some preconditions must be satisfied. For example, the cloud must be semitransparent or relationships between contaminated and cloud-free pixels must be assumed. Due to the inherent imaging geometry features in satellite remote sensing, it is impossible to observe the ground under the clouds directly; therefore, cloud removal algorithms are always not perfect owing to the loss of ground truth. Recently, the use of passenger aircraft as a platform for remote sensing has been proposed by some researchers and institutes, including Airbus and the Japan Aerospace Exploration Agency. Passenger aircraft have the advantages of short visitation frequency and low cost. Additionally, because passenger aircraft fly at lower altitudes compared to satellites, they can observe the ground under the clouds at an oblique viewing angle. In this study, we examine the possibility of creating cloud-free remote sensing data by stacking multiangle images captured by passenger aircraft. To accomplish this, a processing framework is proposed, which includes four main steps: first, multiangle image acquisition from passenger aircraft, second, cloud detection based on deep learning semantic segmentation models, third, cloud removal by image stacking, and fourth, image quality enhancement via haze removal. This method is intended to remove cloud contamination without the requirements of reference images and predetermination of cloud types. The proposed method was tested in multiple case studies, wherein the resultant cloud- and haze-free orthophotos were visualized and quantitatively analyzed in various land cover type scenes. The results of the case studies demonstrated that the proposed method could generate high quality, cloud-free orthophotos. Therefore, we conclude that this framework has great potential for creating cloud-free remote sensing images when the cloud removal of satellite imagery is difficult or inaccurate.
Based on a critical analysis of data analytics and its foundations, we propose a functional approach to estimate data ensemble properties, which is based entirely on the empirical observations of discrete data samples and the relative proximity of these points in the data space and hence named empirical data analysis (EDA). The ensemble functions include the nonparametric square centrality (a measure of closeness used in graph theory) and typicality (an empirically derived quantity which resembles probability). A distinctive feature of the proposed new functional approach to data analysis is that it does not assume randomness or determinism of the empirically observed data, nor independence. The typicality is derived from the discrete data directly in contrast to the traditional approach, where a continuous probability density function is assumed a priori. The typicality is expressed in a closed analytical form that can be calculated recursively and, thus, is computationally very efficient. The proposed nonparametric estimators of the ensemble properties of the data can also be interpreted as a discrete form of the information potential (known from the information theoretic learning theory as well as the Parzen windows). Therefore, EDA is very suitable for the current move to a data-rich environment, where the understanding of the underlying phenomena behind the available vast amounts of data is often not clear. We also present an extension of EDA for inference. The areas of applications of the new methodology of the EDA are wide because it concerns the very foundation of data analysis. Preliminary tests show its good performance in comparison to traditional techniques.
In this paper, a new data partitioning algorithm, named "local modes-based data partitioning", is proposed. This algorithm is entirely data-driven and free from any user input and prior assumptions. It automatically derives the modes of the empirically observed density of the data samples and results in forming parameter-free data clouds. The identified focal points resemble Voronoi tessellations. The proposed algorithm has two versions, namely, offline and evolving. The two versions are both able to work separately and start "from scratch", they can also perform a hybrid. Numerical experiments demonstrate the validity of the proposed algorithm as a fully autonomous partitioning technique, and achieve better performance compared with alternative algorithms.
In this paper, we propose a method to detect anomalous behaviour using heterogenous data. This method detects anomalies based on the recently introduced approach known as Recursive Density Estimation (RDE) and the so called eccentricity. This method does not require prior assumptions to be made on the type of the data distribution. A simplified form of the well-known Chebyshev condition (inequality) is used for the standardised eccentricity and it applies to any type of distribution. This method is applied to three datasets which include credit card, loyalty card and GPS data. Experimental results show that the proposed method may simplify the complex real cases of forensic investigation which require processing huge amount of heterogeneous data to find anomalies. The proposed method can simplify the tedious job of processing the data and assist the human expert in making important decisions. In our future research, more data will be applied such as natural language (e.g. email, Twitter, SMS) and images.
In this letter, we propose a new approach for remote sensing scene classification by creating an ensemble of the recently introduced massively parallel deep (fuzzy) rule-based (DRB) classifiers trained with different levels of spatial information separately. Each DRB classifier consists of a massively parallel set of human-interpretable, transparent zero-order fuzzy IF...THEN... rules with a prototype-based nature. The DRB classifier can self-organize "from scratch" and self-evolve its structure. By employing the pretrained deep convolution neural network as the feature descriptor, the proposed DRB ensemble is able to exhibit human-level performance through a transparent and parallelizable training process. Numerical examples using benchmark data set demonstrate the superior accuracy of the proposed approach together with human-interpretable fuzzy rules autonomously generated by the DRB classifier.
In this chapter, the empirical approach to the problem of anomaly detection is presented, which is free from the pre-defined model and user-and problem-specific parameters and is data driven. The well-known Chebyshev inequality has been simplified by using the standardized eccentricity. An autonomous anomaly detection method is proposed, which is composed of two stages. In the first stage, all the potential global anomalies are selected out based on the data density and/or on the typicality, and in the second stage, the local anomalies are identified based on the data clouds formed from the potential global anomalies. In addition, a fully autonomous approach for the problem of fault detection has been outlined, which can also be extended to a fully autonomous fault detection and isolation approach.
In this chapter, the algorithm summary of the main procedure of the semi-supervised deep rule-based (SS_DRB) classifier described in Chap. 9 is provided, which serves as a powerful extension of the DRB classifier. The offline learning process of the SS_DRB classifier is illustrated and the performance of the SS_DRB algorithm is evaluated based on benchmark image sets. Numerical examples and comparison with the state-of-the-art semi-supervised learning approaches demonstrate that SS_DRB classifier can achieve highly accurate classification results with only a handful of labelled training images, and it consistently outperforms the alternative approaches. The pseudo-code of the main procedure of the SS_DRB classifier and the MATLAB implementations can be found in appendices B.6 and C.6, respectively.
Sea surface height anomaly (SSHA) induced by tropical cyclones (TCs) is closely associated with oscillations and is a crucial proxy for thermocline structure and ocean heat content in the upper ocean. The prediction of TC-induced SSHA, however, has been rarely investigated. This study presents a new composite analysis-based random forest (RF) approach to predict daily TC wind pump induced SSHA. The proposed method utilizes TC's characteristics and prestorm upper oceanic parameters as input features to predict TC-induced SSHA up to 30 days after TC passage. Simulation results suggest that the proposed method is skillful at inferring both the amplitude and temporal evolution of SSHA induced by TCs of different intensity groups. Using a TC-centered 5° × 5° box, the proposed method achieves highly accurate prediction of TC-induced SSHA over the Western North Pacific with root mean square error of 0.024 m, outperforming alternative machine learning methods and the numerical model. Moreover, the proposed method also demonstrated good prediction performance in different geographical regions, i.e., the South China Sea and the Western North Pacific subtropical ocean. The study provides insight into the application of machine learning in improving the prediction of SSHA influenced by extreme weather conditions. Accurate prediction of TC-induced SSHA allows for better preparedness and response, reducing the impact of extreme events (e.g., storm surge) on people and property.
An evolving intelligent system (EIS) is able to self-update its system structure and meta-parameters from streaming data. However, since the majority of EISs are implemented on a single-model architecture, their performances on large-scale, complex data streams are often limited. To address this deficiency, a novel self-organizing fuzzy inference ensemble framework is proposed in this paper. As the base learner of the proposed ensemble system, the self-organizing fuzzy inference system is capable of self-learning a highly transparent predictive model from streaming data on a chunk-by-chunk basis through a human-interpretable process. Very importantly, the base learner can continuously self-adjust its decision boundaries based on the inter-class and intra-class distances between prototypes identified from successive data chunks for higher classification precision. Thanks to its parallel distributed computing architecture, the proposed ensemble framework can achieve great classification precision while maintain high computational efficiency on large-scale problems. Numerical examples based on popular benchmark big data problems demonstrate the superior performance of the proposed approach over the state-of-the-art alternatives in terms of both classification accuracy and computational efficiency. •A novel fuzzy ensemble framework proposed for big streaming data classification.•A novel fuzzy inference system to learn from streaming data chunk-by-chunk.•The base learner to self-calibrate precise decision boundaries from streaming data.•A novel ensemble architecture to build diverse committees with high efficiency.
In this chapter, the algorithm summary of the proposed autonomous anomaly detection (AAD) algorithm described in Chap. 10.1007/978-3-030-02384-3_6 is provided. Numerical examples based on both the synthetic and benchmark datasets are presented for evaluating the performance of the AAD algorithm. Well-known traditional anomaly detection approaches are used for a further comparison. It was demonstrated through the numerical experiments that the AAD algorithm is able to provide a more objective, accurate way for anomaly detection, and its performance is not influenced by the structure of the data and is equally effective in detecting collective anomalies as well as individual anomalies. The pseudo-code of the main procedure of the AAD algorithm and the MATLAB implementation can be found in Appendices B.1 and C.1, respectively.
This paper proposes a new extended zero-order Autonomous Learning Multiple-Model (ALMMo-0*) neuro-fuzzy approach in order to classify different heart disorders through sounds. ALMMo-0* is build upon the recently introduced ALMMo-0. In this paper ALMMo-0 is extended by adding a preprocessing structure which improves the performance of the proposed method. ALMMo-0* has as a learning engine composed of hierarchical a massively parallel set of 0-order fuzzy rules, which are able to self-adapt and provide transparent and human understandable IF ... THEN representation. The heart sound recordings considered in the analysis were sourced from several contributors around the world. Data were collected from both clinical and nonclinical environment, and from healthy and pathological patients. Differently from mainstream machine learning approaches, ALMMo-0* is able to learn from unseen data. The main goal of the proposed method is to provide highly accurate models with high transparency, interpretability, and explainability for heart disorder diagnosis. Experiments demonstrated that the proposed neuro-fuzzy-based modeling is an efficient framework for these challenging classification tasks surpassing its state-of-the-art competitors in terms of classification accuracy. Additionally, ALMMo-0* produced transparent AnYa type fuzzy rules, which are human interpretable, and may help specialists to provide more accurate diagnosis. Medical doctors can easily identify abnormal heart sounds by comparing a patient's sample with the identified prototypes from abnormal samples by ALMMo-0*. (C) 2020 Elsevier B.V. All rights reserved.
In this paper, we propose a fully autonomous, local-modes-based data partitioning algorithm, which is able to automatically recognize local maxima of the data density from empirical observations and use them as focal points to form shape-free data clouds, i.e. a form of Voronoi tessellation. The method is free from user- and problem- specific parameters and prior assumptions. The proposed algorithm has two versions: i) offline for static data and ii) evolving for streaming data. Numerical results based on benchmark datasets prove the validity of the proposed algorithm and demonstrate its excellent performance and high computational efficiency compared with the state-of-art clustering algorithms. (C) 2018 Elsevier Inc. All rights reserved.
This paper proposes a new approach that is based on the recently introduced semi-supervised deep rule-based classifier for remote sensing scene classification. The proposed approach employs a pre-trained deep convoluational neural network as the feature descriptor to extract high-level discriminative semantic features from the sub-regions of the remote sensing images. This approach is able to self-organize a set of prototype-based IF...THEN rules from few labeled training images through an efficient supervised initialization process, and continuously self-updates the rule base with the unlabeled images in an unsupervised, autonomous, transparent and human-interpretable manner. Highly accurate classification on the unlabeled images is performed at the end of the learning process. Numerical examples demonstrate that the proposed approach is a strong alternative to the state-of-the-art ones.
Traditionally, in supervised machine learning, (a significant) part of the available data (usually 50%-80%) is used for training and the rest-for validation. In many problems, however, the data are highly imbalanced in regard to different classes or does not have good coverage of the feasible data space which, in turn, creates problems in validation and usage phase. In this paper, we propose a technique for synthesizing feasible and likely data to help balance the classes as well as to boost the performance in terms of confusion matrix as well as overall. The idea, in a nutshell, is to synthesize data samples in close vicinity to the actual data samples specifically for the less represented (minority) classes. This has also implications to the so-called fairness of machine learning. In this paper, we propose a specific method for synthesizing data in a way to balance the classes and boost the performance, especially of the minority classes. It is generic and can be applied to different base algorithms, for example, support vector machines, k-nearest neighbour classifiers deep neural, rule-based classifiers, decision trees, and so forth. The results demonstrated that (a) a significantly more balanced (and fair) classification results can be achieved and (b) that the overall performance as well as the performance per class measured by confusion matrix can be boosted. In addition, this approach can be very valuable for the cases when the number of actual available labelled data is small which itself is one of the problems of the contemporary machine learning.
Today we live in a data-rich environment. This is dramatically different from the last century when the fundamentals of machine learningMachine learning, control theoryControl theory and related subjects were established. Nowadays, vast and exponentially increasing data sets and streamsData sets and streams which are often non-linearNon-linear, non-stationaryNon-stationary and increasingly more multi-modalMulti-modal/heterogeneousHeterogeneous (combining various physical variables, signals with images/videos as well as text) are being generated, transmitted and recorded as a result of our everyday live. This is drastically different from the reality when the fundamental results of the probability theoryProbability theory, statisticsStatistics and statistical learningStatistical learning where developed few centuries ago.
Tropical cyclones (TCs), with an intensive wind pump impact, induce sea surface temperature cooling (SSTC) on the upper ocean. SSTC is a pronounced indicator to reveal TC evolution and oceanic conditions. However, there are few effective methods for accurately approximating the amplitude of the spatial structure of TC-induced SSTC. This study proposes a novel explainable machine learning framework to model and interpret the amplitude of the spatial structure of SSTC over the northwest Pacific (NWP). In particular, 12 predictors related to TC characteristics and pre-storm ocean states are considered as inputs. A composite analysis technique is used to characterize the amplitude of the spatial structure of SSTC across the TC track. Extreme gradient boosting (XGBoost) is utilized to predict the amplitude of SSTC from the 12 predictors. To better interpret the ocean-atmosphere interaction, a SHapely Additive explanations (SHAP) method is further employed to identify the contributions of predictors in determining the amplitude of the TC-induced SSTC, bringing the attribute-oriented explainability to the proposed method. The results showed that the proposed method could accurately predict the amplitude of the spatial structure of SSTC for different TC intensity groups and outperforms a numerical model. The proposed method also serves as an effective tool for reconstructing composite maps of both interannual and seasonal evolutions of SSTC spatial structure. The study offers insight into applying machine learning to model and interpret the responses of oceanic conditions triggered by extreme weather conditions (e.g., TCs).
Nowadays, cyber-attacks have become a common and persistent issue affecting various human activities in modern societies. Due to the continuously evolving landscape of cyber-attacks and the growing concerns around " black box " models, there has been a strong demand for novel explainable and interpretable intrusion detection systems with online learning abilities. In this paper, a novel soft prototype-based autonomous fuzzy inference system (SPAFIS) is proposed for network intrusion detection. SPAFIS learns from network traffic data streams online on a chunk-by-chunk basis and autonomously identifies a set of meaningful, human-interpretable soft prototypes to build an IF-THEN fuzzy rule base for classification. Thanks to the utilization of soft prototypes, SPAFIS can precisely capture the underlying data structure and local patterns, and perform internal reasoning and decision-making in a human-interpretable manner based on the ensemble properties and mutual distances of data. To maintain a healthy and compact knowledge base, a pruning scheme is further introduced to SPAFIS, allowing itself to periodically examine the learned solution and remove redundant soft prototypes from its knowledge base. Numerical examples on public network intrusion detection datasets demonstrated the efficacy of the proposed SPAFIS in both offline and online application scenarios, outperforming the state-of-the-art alternatives. Thanks to the rapid development in electronic manufacturing and information technology, the Internet has become an essential part of everyday life for billions of individuals in modern societies. The Internet has greatly transformed the way people communicate, network and access information. However, the ongoing digitalization in the world has also led to a significant rise in cyber-attacks. According to the Cyber Security Breaches Survey published by the UK government in April 2023 [1], 59% of medium businesses, 69% of large businesses and 56% of high-income charities have encountered cybersecurity breaches and/or cyber-attacks in the last 12 months. Nowadays, the escalating cyber-attacks have posed a major and persistent threat to individuals, businesses and organizations on the Internet. The need for effective techniques to protect information security is highly pronounced. Intrusion detection systems (IDSs) are one of the most effective security techniques to prevent cyber-attacks [2]. The function of an IDS is to monitor the network and identify malicious activities. Traditional IDSs are primarily based on signatures. Such IDSs utilize pattern matching methods to compare current activities against signatures of previous intrusions stored in the database [3]. Signature-based IDSs are highly effective in detecting known attacks, but they are unable to detect novel attacks because of the lack of matching signature in the database. As the technological evolution of cybercrime has made cyber-attacks more sophisticated and difficult to detect, traditional signature-based IDSs have become insufficient in real-world scenarios [4]. Machine learning techniques are capable of learning normal and malicious patterns from empirically observed network activities to constructing accurate predictive models with less human involvement [4]. Conventional machine learning methods, such as decision tree (DT) [5], random forest (RF) [6], support vector machine (SVM) [7], k-nearest neighbour (KNN) [8], etc., have been extensively used for identifying cyber-attacks. IDSs based on conventional machine learning have achieved many successes, but they generally struggle with large-scale, complex intrusion detection problems [9]. Due to the evolving landscape of cyber-attacks, characterized by the increasing sophistication and complexity, there has been a rapidly growing demand for IDSs that leverage more advanced machine learning techniques.
This paper proposes a dynamic evolving fuzzy system (DEFS) for streaming data prediction. DEFS utilises the enhanced data potential and prediction errors of individual local models as the main criteria for fuzzy rule generation. A vital feature of the proposed system is its novel rule merging scheme that can self-adjust its tolerance towards the degree of similarity between two similar fuzzy rules according to the size of the rule base. To better handle the shifts and drifts in the data patterns, a novel rule quality measure based on both the utility values and the prediction accuracy of individual fuzzy rules is further introduced to help DEFS identify these less activated fuzzy rules with poorer descriptive capabilities and, thereby, maintaining a healthier fuzzy rule base by removing these stale rules. Very importantly, the thresholds used by DEFS are self-adaptive towards the input data. The adaptive thresholds can help DEFS to precisely capture the underlying structure and dynamically changing patterns of streaming data, enabling the system performing accurate approximation reasoning. Numerical examples based on several popular benchmark problems show the superior performance of DEFS over the state-of-the-art evolving fuzzy systems. The prediction performance of the proposed method is at least 2.88% better than the best-performing comparative EFSs on each individual regression benchmark problem considered in this study, and the average performance improvement across all the numerical experiments is approximately 30%.
Fuzzy systems offer a formal and practically popular methodology for modelling nonlinear problems with inherent uncertainties, entailing strong performance and model interpretability. Particularly, semi-supervised boosting is widely recognised as a powerful approach for creating stronger ensemble classification models in the absence of sufficient labelled data without introducing any modification to the employed base classifiers. However, the potential of fuzzy systems in semi-supervised boosting has not been systematically explored yet. In this study, a novel semi-supervised boosting algorithm devised for zero-order evolving fuzzy systems is proposed. It ensures both the consistence amongst predictions made by individual base classifiers at successive boosting iterations and the respective levels of confidence towards their predictions throughout the process of sample weight updating and ensemble output generation. In so doing, the base classifiers are empowered to gradually focus more on challenging samples that are otherwise hard to generalise, enabling the development of more precise integrated classification boundaries. Numerical evaluations on a range of benchmark problems are carried out, demonstrating the efficacy of the proposed semi-supervised boosting algorithm for constructing ensemble fuzzy classifiers with high accuracy.