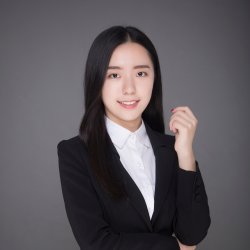
Dr Yufan Liu
About
My research project
Chemical safety assessment using omics and machine learning technologyMy project aims to utilize machine learning technology to investigate the role of proteomics in identifying in vitro safety levels of chemicals with underlying relevant human biological mechanistic understandings.
My project aims to utilize machine learning technology to investigate the role of proteomics in identifying in vitro safety levels of chemicals with underlying relevant human biological mechanistic understandings.
Publications
Comprehensive analysis of multi-omics data can reveal alterations in regulatory pathways induced by cellular exposure to chemicals by characterizing biological processes at the molecular level. Data-driven omics analysis, conducted in a dosedependent or dynamic manner, can facilitate comprehending toxicity mechanisms. This study introduces a novel multi-omics data analysis designed to concurrently examine dose-dependent and temporal patterns of cellular responses to chemical perturbations. This analysis, encompassing preliminary exploration, pattern deconstruction, and network reconstruction of multi-omics data, provides a comprehensive perspective on the dynamic behaviors of cells exposed to varying levels of chemical stimuli. Importantly, this analysis is adaptable to any number of any omics layers, including site-specific phosphoproteomics. We implemented this analysis on multiomics data obtained from HepG2 cells exposed to a range of caffeine doses over varying durations and identified six response patterns, along with their associated biomolecules and pathways. Our study demonstrates the effectiveness of the proposed multi-omics data analysis in capturing multi-dimensional patterns of cellular response to chemical perturbation, enhancing understanding of pathway regulation for chemical risk assessment.
The effect of COVID-19 infection on the human metabolome has been widely reported, but to date all such studies have focused on a single wave of infection. COVID-19 has generated numerous waves of disease with different clinical presentations, and therefore it is pertinent to explore whether metabolic disturbance changes accordingly, to gain a better understanding of its impact on host metabolism and enable better treatments. This work used a targeted metabolomics platform (Biocrates Life Sciences) to analyze the serum of 164 hospitalized patients, 123 with confirmed positive COVID-19 RT-PCR tests and 41 providing negative tests, across two waves of infection. Seven COVID-19-positive patients also provided longitudinal samples 2–7 months after infection. Changes to metabolites and lipids between positive and negative patients were found to be dependent on collection wave. A machine learning model identified six metabolites that were robust in diagnosing positive patients across both waves of infection: TG (22:1_32:5), TG (18:0_36:3), glutamic acid (Glu), glycolithocholic acid (GLCA), aspartic acid (Asp) and methionine sulfoxide (Met-SO), with an accuracy of 91%. Although some metabolites (TG (18:0_36:3) and Asp) returned to normal after infection, glutamic acid was still dysregulated in the longitudinal samples. This work demonstrates, for the first time, that metabolic dysregulation has partially changed over the course of the pandemic, reflecting changes in variants, clinical presentation and treatment regimes. It also shows that some metabolic changes are robust across waves, and these can differentiate COVID-19-positive individuals from controls in a hospital setting. This research also supports the hypothesis that some metabolic pathways are disrupted several months after COVID-19 infection.
Background:Annual national diabetes audit data consistently shows most people with diabetes do not consistently achieve blood glucose targets for optimal health, despite the large range of treatment options available.Aim:To explore the efficacy of a novel clinical intervention to address physical and mental health needs within routine diabetes consultations across health care settings.Methods:A multicenter, parallel group, individually randomized trial comparing consultation duration in adults diagnosed with T1D or T2D for ≥6 months using the Spotlight-AQ platform versus usual care. Secondary outcomes were HbA1c, depression, diabetes distress, anxiety, functional health status, and healthcare professional burnout. Machine learning models were utilized to analyze the data collected from the Spotlight-AQ platform to validate the reliability of question-concern association; as well as to identify key features that distinguish people with type 1 and type 2 diabetes, as well as important features that distinguish different levels of HbA1c.Results:n = 98 adults with T1D or T2D; any HbA1c and receiving any diabetes treatment participated (n = 49 intervention). Consultation duration for intervention participants was reduced in intervention consultations by 0.5 to 4.1 minutes (3%-14%) versus no change in the control group (−0.9 to +1.28 minutes). HbA1c improved in the intervention group by 6 mmol/mol (range 0-30) versus control group 3 mmol/mol (range 0-8). Moderate improvements in psychosocial outcomes were seen in the intervention group for functional health status; reduced anxiety, depression, and diabetes distress and improved well-being. None were statistically significant. HCPs reported improved communication and greater focus on patient priorities in consultations. Artificial Intelligence examination highlighted therapy and psychological burden were most important in predicting HbA1c levels. The Natural Language Processing semantic analysis confirmed the mapping relationship between questions and their corresponding concerns. Machine learning model revealed type 1 and type 2 patients have different concerns regarding psychological burden and knowledge. Moreover, the machine learning model emphasized that individuals with varying levels of HbA1c exhibit diverse levels of psychological burden and therapy-related concerns.Conclusion:Spotlight-AQ was associated with shorter, more useful consultations; with improved HbA1c and moderate benefits on psychosocial outcomes. Results reflect the importance of a biopsychosocial approach to routine care visits. Spotlight-AQ is viable across health care settings for improved outcomes.