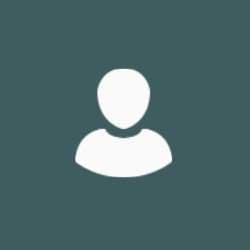
Dr Zhou Hao
Publications
PLATOR is a new electrothermal thruster for space logistics applications, developed by the University of Surrey and the University of Leicester. This paper describes the technology behind the development of the thruster and presents a mission scenario where a PLATOR-propelled spacecraft is used to capture and de-orbit the European Space Agency (ESA)'s Envisat satellite. The orbital transfer trajectory is designed using a time-optimal control approach, and the spacecraft's state vector's uncertainties are assessed through a covariance analysis. A navigation analysis is then performed to evaluate the spacecraft's capability to autonomously track its motion during the transfer using GPS measurements. Finally, a target proximity phase is then simulated to demonstrate the spacecraft's capability to rendezvous and dock with Envisat, using the uncertainties obtained from the covariance analysis, showing the potential of the PLATOR thruster for in-orbit servicing and active debris removal applications.
Astrophysicists demand larger (mirror diameter > 10m) space optical telescopes to investigate more distant events that happened during the very early period of the universe, for example formations of the earliest stars. The deployable telescope design like James Webb Space Telescope that has a 6.5m diameter primary mirror has already reached the capacity limits of the existing launch vehicles. Therefore, the space industry has been considering using robotic technologies to build future optical reflecting three-mirror structured space telescopes in orbit from smaller components. One of the design paradigms is to use a high-DOF manipulator on a free-flying platform to build the optical telescope in orbit. This approach requires high precision and accuracy in the robotic manipulation GNC system that has several challenges yet to be addressed: 1. Orbital environmental parameters that affect sensing and perception; 2. Limitations in robotic hardware, trajectory planning algorithms and controllers. To investigate these problems for in-orbit manipulation, the UK national hub on future AI and robotics for space (FAIR-SPACE) at the Surrey Space Centre (SSC) has been developing a ground-based hardware-in-the-loop (HIL) robotic demonstrator to simulate in-orbit manipulation. The key elements of the demonstrator are two 6-DOF manipulators and a re-configurable sensor system. One of the manipulators with a > 3-DOF gripping mechanism represents the assembly manipulator on a spacecraft whose orbital dynamics, kinematics, and environmental disturbances and uncertainties are propagated in a computer. The other 6-DOF manipulator with a torque/force sensor is used as a gravity offoad mechanism to carry the space telescope mirror segment. The relative motions between the service/manipulation arm and the mirror segment are computed and then executed by the second arm. The sensor system provides visual feedback of the end-effector and uses computer vision and AI to estimate the pose and position of the mirror segment respectively. The demonstrator aims to verify and validate the manipulator assembly approach for future large space optical telescopes against ground truth and benchmarks. This paper explains the motivation behind developing this testbed and introduces the current hardware setup of the testbed and its key features.
In order to improve the accuracy and speed of simulation, a piecewise linear (PWL) network simulation method based on FPGA is proposed. Through RT-LAB hardware in the loop simulation platform and supporting FPGA development tools, a simulation system based on FPGA is established. The nonlinear element is modeled by PWL approximation method. The system matrix method that accurately supports the characteristics of any PWL element is applied, so that the realtime simulation system can fit the real operating state of the system, and has very good simulation accuracy and simulation results. In order to verify the effectiveness of the system, an energy storage grid connected test system is established by using the PWL network system based on FPGA. The experiment proves the effectiveness of the PWL network simulation based on FPGA.
The Enhanced Model Reference Adaptive Control (EMRAC) algorithm, augmenting the MRAC strategy with adaptive integral and adaptive switching control actions, is an effective solution to impose reference dynamics to plants affected by parameter uncertainties, unmodeled dynamics and disturbances. However, the design of the EMRAC solutions has so far been limited to single-input systems. To cover the gap, this paper presents two extensions of EMRAC to multi-input systems. The adaptive mechanism of both solutions includes the -modification strategy to assure the boundedness of the adaptive gains also in presence of persistent disturbances. The closed-loop system is analytically studied, and conditions for the asymptotic convergence of the tracking error are presented. Furthermore, when the plant is subjected to unmatched disturbances, the ultimate boundedness of the closed-loop dynamics, which are made discontinuous by the adaptive switching control actions, is systematically proven by using Lyapunov theory for Filippov systems. The problem of trajectory tracking for space robotic arms in presence of unknown and noncooperative targets is used to test the effectiveness of the novel multi-input EMRAC algorithms for taming uncertain systems. Four EMRAC solutions are designed for this engineering application, and tested within a high fidelity simulation framework based on the Robot Operating System. Finally, the tracking performance of the EMRAC implementations is quantitatively evaluated via a set of key performance indicators in the joint space and operational space, and compared with that of four benchmarking controllers.
Conventional spacecraft Guidance, Navigation, and Control (GNC) architectures have been designed to receive and execute commands from ground control with minimal automation and autonomy onboard spacecraft. In contrast, Artificial Intelligence (AI)-based systems can allow real-time decision-making by considering system information that is difficult to model and incorporate in the conventional decision-making process involving ground control or human operators. With growing interests in on-orbit services with manipulation, the conventional GNC faces numerous challenges in adapting to a wide range of possible scenarios, such as removing unknown debris, potentially addressed using emerging AI-enabled robotic technologies. However, a complete paradigm shift may need years' efforts. As an intermediate solution, we introduce a novel visual GNC system with two state-of-the-art AI modules to replace the corresponding functions in the conventional GNC system for on-orbit manipulation. The AI components are as follows: (i) A Deep Learning (DL)-based pose estimation algorithm that can estimate a target's pose from two-dimensional images using a pre-trained neural network without requiring any prior information on the dynamics or state of the target. (ii) A technique for modeling and controlling space robot manipulator trajectories using probabilistic modeling and reproduction to previously unseen situations to avoid complex trajectory optimizations on board. This also minimizes the attitude disturbances of spacecraft induced on it due to the motion of the robot arm. This architecture uses a centralized camera network as the main sensor, and the trajectory learning module of the 7 degrees of freedom robotic arm is integrated into the GNC system. The intelligent visual GNC system is demonstrated by simulation of a conceptual mission—AISAT. The mission is a micro-satellite to carry out on-orbit manipulation around a non-cooperative CubeSat. The simulation shows how the GNC system works in discrete-time simulation with the control and trajectory planning are generated in Matlab/Simulink. The physics rendering engine, Eevee, renders the whole simulation to provide a graphic realism for the DL pose estimation. In the end, the testbeds developed to evaluate and demonstrate the GNC system are also introduced. The novel intelligent GNC system can be a stepping stone toward future fully autonomous orbital robot systems.
This paper adds on to the on-going efforts to provide more autonomy to space robots and introduces the concept of programming by demonstration or imitation learning for trajectory planning of manipulators on free-floating spacecraft. A redundant 7-DoF robotic arm is mounted on small spacecraft dedicated for debris removal, on-orbit servicing and assembly, autonomous and rendezvous docking. The motion of robot (or manipulator) arm induces reaction forces on the spacecraft and hence its attitude changes prompting the Attitude Determination and Control System (ADCS) to take large corrective action. The method introduced here is capable of finding the trajectory that minimizes the attitudinal changes thereby reducing the load on ADCS. One of the critical elements in spacecraft trajectory planning and control is the power consumption. The approach introduced in this work carry out trajectory learning offline by collecting data from demonstrations and encoding it as a probabilistic distribution of trajectories. The learned trajectory distribution can be used for planning in previously unseen situations by conditioning the probabilistic distribution. Hence almost no power is required for computations after deployment. Sampling from a conditioned distribution provides several possible trajectories from the same start to goal state. To determine the trajectory that minimizes attitudinal changes, a cost term is defined and the trajectory which minimizes this cost is considered the optimal one.
The increased complexity of the tasks that on-orbit robots have to undertake has led to an increased need for manipulation dexterity. Space robots can become more dexterous by adopting grasping and manipulation methodologies and algorithms from terrestrial robots. In this paper, we present a novel methodology for evaluating the stability of a robotic grasp that captures a piece of space debris, a spent rocket stage. We calculate the Intrinsic Stiffness Matrix of a 2-fingered grasp on the surface of an Apogee Kick Motor nozzle and create a stability metric that is a function of the local contact curvature, material properties, applied force, and target mass. We evaluate the efficacy of the stability metric in a simulation and two real robot experiments. The subject of all experiments is a chasing robot that needs to capture a target AKM and pull it back towards the chaser body. In the V-REP simulator, we evaluate four grasping points on three AKM models, over three pulling profiles, using three physics engines. We also use a real robotic testbed with the capability of emulating an approaching robot and a weightless AKM target to evaluate our method over 11 grasps and three pulling profiles. Finally, we perform a sensitivity analysis to demonstrate how a variation on the grasping parameters affects grasp stability. The results of all experiments suggest that the grasp can be stable under slow pulling profiles, with successful pulling for all targets. The presented work offers an alternative way of capturing orbital targets and a novel example of how terrestrial robotic grasping methodologies could be extended to orbital activities.