Modelling and predicting the progression of chronic kidney disease
Start date
September 2015End date
August 2018Abstract
Chronic Kidney Disease (CKD) is a significant cause of morbidity and mortality across the developed world. Patients with CKD have increased risk of death from cardiovascular disease and End Stage Kidney Failure, leading to dialysis and kidney transplant. Indeed, according to an NHS Kidney Care report in 2012, CKD was estimated to cost £1.45 billion in 2009-10; 1.8 million people were diagnosed with CKD in England; and, there were potentially 900,000 to 1.8 million people with undiagnosed CKD. Therefore, the importance and urgency of managing CKD cannot be overemphasised.
An overarching objective of this MRC research is to revisit the problem of modelling the progression of CKD using state-of-the-art machine learning techniques and methodologies. We introduce three innovations in this project.
First, we shall investigate statistical models that directly predict key clinical variables so that clinicians can make more informed decisions. This approach is more consistent with guidelines-based prescribing that is used by general practitioners.
Second, we will develop a way to identify patient groups by using data-driven methods. The approach used is similar to 'market segmentation' used in Business Intelligence. The hypothesis is that patients can be divided into groups not by their disease or stages (as currently practised) but by their patient records that essentially capture their health history. In essence, this method of grouping will naturally group patients with similar treatments including drugs and procedures and similar physiological and pathological characteristics.
Third, as part of the process in predicting the efficacy of kidney function, we will develop a risk model for predicting and detecting Acute Kidney Injury (AKI). This novel model will inform clinicians how likely it is that a patient will suffer from AKI. In short, we propose a unified framework to predict the efficacy of kidney function that also considers the possibility of AKI. This represents a potential advancement in modelling and understanding CKD because the risks of end-stage of CKD and AKI are so far often treated independently.
The potential advantages of the proposed method include:
- Better tailoring of the method to patient subgroups via data-driven stratification
- Ability to exploit many more variables that are specific to each patient stratum
- Ability to predict eGFR (or estimated Glomerular Filtration rate, which characterises the efficiency of kidney function) and ACR (or Albumin:creatinine ratio) that can be used in conjunction with guidelines-based prescribing
- Ability to predict the risk of acute kidney injury.
These outputs are significant in the following ways:
First, although risk models for CKD exist, there is no predictor for eGFR and ACR to date. Directly predicting these variables have significant clinical implication because the approach is consistent with guidelines-based prescribing. Unlike risk models, by directly predicting the observable outputs, these predictors convey the notions of severity and uncertainty at the same time (whilst risk models often predict only the worst outcome).
Second, there is no AKI risk model and its association with CKD remains unclear; our model considers AKI risk when predicting eGFR, thus, combining the two pieces of related information in a principled way via the Bayesian framework.
Third, we propose data-driven patient stratification as an alternative to disease and state-specific stratification. This will lead to a better understanding of CKD pathways and patient profiling. Indeed, our proof-of-concept experiment suggests that patients who have eGFR may be categorised into some 60 clusters. This patient stratification strategy inherently considers co-morbidities and disease-staging at the same time.
Funding amount
£89,117
Funder
Team
Principal investigator
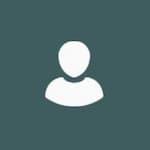
Dr Norman Poh
Associate member
See profile