11am - 12 noon BST
Monday 14 June 2021
Spherical based human tracking and 3D pose estimation for immersive entertainment production
PhD open viva presentation by PhD student: Matthew Shere. All welcome!
Free
This event has passed
Speakers
Abstract
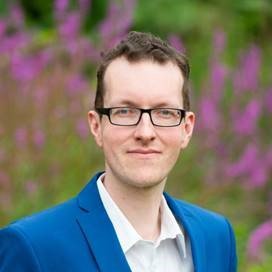
Human tracking and 3D pose estimation are two core activities of computer vision, identifying and following an individual within a scene in the case of the former, and producing a three-dimensional estimate of an individuals body pose and configuration for the latter. Intuitively, we can combine these operations, using produced tracking results to isolate each individual within a scene, then estimating their pose as the individual moves throughout. Such a combination of processes could be applied to scenarios such as entertainment production, home health monitoring or sports analysis, where there is a relatively low person count, and a requirement to know more than just the approximate three-dimensional position of a person. However, such systems generally require non-complimentary camera configurations, with tracking solutions dispersing cameras to maximise scene coverage at the cost of camera overlap, and multi-view pose estimation concentrating cameras on a single area to maximise camera overlap at the cost of scene coverage. This drives the camera count ever higher, since each room or area effectively requires its own pose estimation camera rig.
An ideal solution to this would therefore need to maximise both scene coverage and camera overlap, something which can easily be achieved using wide angle, panoramic or 360° cameras. Through careful placement of these cameras, we simultaneously view the entire scene, and also produce multiple views of an individual in order to inform our pose estimate. However, such cameras bring their own representation problems, hampering the performance of existing solutions, or preventing them from operating entirely. Therefore, we explore this facet of the problem, producing tracking and pose estimation solutions that natively function from 360° imagery.
To facilitate this, we firstly contribute a tracker and pose estimation system, operating from a pair of horizontally disjoint 360° cameras. We use provided person segmentation masks to create a series of descriptors suitable for use at differing resolutions, while the specific camera configuration allows us to share these descriptors, which combined with spatial information are used to identify an individual regardless of their distance from either camera. With a person isolated, we then create a joint-wise pose estimate directly from the spherical co-ordinate space, eliminating the need for either reprojection operations, or intrinsic calibration information to be provided.
Building upon this, our second contribution reduces the size of the physical camera rig, combining a pair of 360° cameras into a vertical configuration with low baseline. From this, we simultaneously track each individual in the scene using only two-dimensional joint location estimates, exploiting the camera arrangement to assume an epipolar relationship, and simultaneously eliminating the need to use any image information within the tracking process. From this, we then construct a temporally consistent 3D human pose estimate, first producing a coarse, Principal Component Analysis (PCA) model based estimate from joint likelihood fields, then refining this in a joint-wise fashion over successive iterations, smoothing out any unrealistic jumps in motion produced as a result of the low baseline between the vertical camera pair.
Having established tracking in a local area, our final contribution moves beyond the confines of a single room, and tracks individuals as they move throughout a scene comprised of multiple rooms or regions. We perform this with no prior knowledge of the scene layout or content, and use only camera extrinsics and person movements to iteratively build tracks for each individual simultaneously, with each stage informing the next. We build intra-region temporal descriptors for each camera by combining colour information around 2D joint estimates, before establishing how people move between cameras, and finally combining these temporal descriptors to reconcile the inter-region tracks.
Overall, we demonstrate that 360° imagery presents many advantages that can be utilised or exploited in both human tracking, and in three-dimensional human pose estimation, and that these advantages outweigh any costs of working with 360° footage. We enable tracking in a variety of situations where traditional methods are impractical or impossible, and position methods to provide training data for the next generation of multi-camera, 360° capable deep-learning based tracking approaches. We also produce pose estimates that bridge the gap between multi-view systems and monocular systems. Finally, we create a range of 360° datasets that can be used for the development and testing of future algorithms.
Attend the event
You can attend this event by Zoom.