10am - 11am
Friday 10 June 2022
Sketching and Streaming based Subspace Clustering for Large-scale Data Classification
PhD Viva Open Presentation by Shuoyang Li - All welcome!
Free
University of Surrey
Guildford
Surrey
This event has passed
Speakers
Sketching and Streaming based Subspace Clustering for Large-scale Data Classification
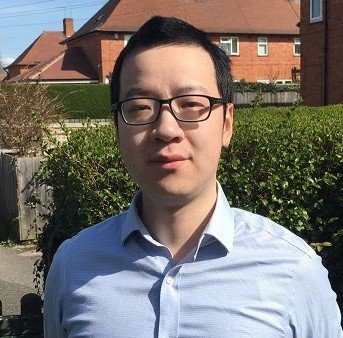
Abstract:
Subspace clustering (SC), as an unsupervised learning method, has shown effectiveness in learning class labels for high-dimensional data without prior knowledge of data labels. SC is especially useful for the cases when the data labelling is difficult or expensive. Recently, spectral clustering-based SC algorithms have drawn extensive attention due to their effectiveness and easy implementation.
Spectral clustering based SC algorithms usually consist of two phases: constructing an affinity matrix and segmenting data points with spectral clustering. The affinity matrix reveals the coherence between data points, and its computation would become costly while the input dataset is large. As a result, despite its great success, the performance of SC is often limited in the scenarios involving high dimensional or high-volume data, due to the prohibitive computational and memory costs required.
To broaden the usage of SC, we propose several methods that aim at handling both computational and memory problems. To begin with, we introduce a random sketching and a dimension selection method to reduce the feature dimensionality for sparse subspace clustering (SSC), which is one of the most popular spectral clustering-based SC methods. By random sketching or dimension selection, the subspace clustering algorithm can take a subset of the full dimensions, thus input data with high-dimension can be clustered more efficiently. Afterwards,
we introduce a streaming-based subspace clustering algorithm that exploits the low-rank factorisation, which allows the data to be clustered on the fly, making the computational and memory costs limited even with a large-volume input dataset. In addition, we propose a residual-based online subspace clustering algorithm that uses online low-rank optimisation and a dictionary matrix updated iteratively. The algorithm manages to improve the above two methods further, with reduced memory demands and improved clustering performance for subspace changing with respect to time. The proposed methods are evaluated on synthetic and real data (such as acoustic, image and video datasets) to demonstrate the improved performance of these proposed methods in a variety of conditions including computational efficiency and clustering performance.
Attend the event
This is a free event for everyone to join