11am - 12 noon
Thursday 11 August 2022
Deep Learning with Limited Annotated Data
PhD Open Viva Presentation by Zhihe Lu
All Welcome!
Free
This event has passed
Speakers
Deep Learning with Limited Annotated Data
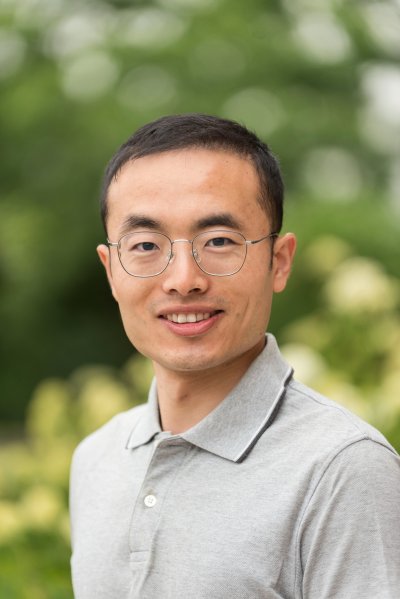
Abstract:
Supervised deep models have achieved the state-of-the-art performance on many vision tasks, relying on the large-scale labeled datasets and advanced algorithms. However, annotating images, especially pixel-wise segmentation masks, is a highly labor-intensive process. The high labor-cost results in the impracticability of labeling sufficient data for a new task. Towards that end, utilizing limited annotations to train a generalized deep model has been topical and drawn much attention in recent years. Consequently, many annotation-efficient learning paradigms have been proposed, such as self-supervised learning, semi-supervised learning, unsupervised learning, few-shot learning, etc. In this thesis, four concrete applications: Unsupervised Domain Adaptation (UDA), Source-Free Domain Adaptive Semantic Segmentation (SFDASS), Few-shot Semantic Segmentation (FSS) and Generalized Few-shot Semantic Segmentation (GFSS) will be explored.
The first contribution of this thesis is to propose STochastic clAssifieRs (STAR) to identify the misaligned local regions between a source and target domain for UDA. Specifically, instead of representing one classifier as a weight vector, STAR models it as a Gaussian distribution with its variance representing the inter-classifier discrepancy. This enables infinite number of classifiers being used with the same amount of parameters as having two normal classifiers. Second, a novel Bayesian Neural Network (BNN) based uncertainty-aware framework is proposed for SFDASS. With the uncertainty estimation of BNN, two novel self-training based components, i.e., Uncertainty-aware Online Teacher-Student Learning (UOTSL) and Uncertainty-aware FeatureMix (UFM), have been introduced. The third contribution is to propose a novel meta-learning pipeline by focusing solely on the simplest component -- classifier, in a FSS system. In particular, a Classifier Weight Transformer (CWT) is designed and meta-learned to dynamically adapt the support-set trained classifier’s weights to each query image in an inductive way. Finally, a novel Prediction Calibration Network (PCN) is proposed to address GFSS. Instead of classifier parameter fusion, it fuses the scores produced separately by the base and novel classifiers. To ensure that the fused scores are not biased to either the base or novel classes, a new Transformer-based calibration module is introduced to enforce feature and score consistency.
Overall, all presented works are under annotation-efficient setting with the purpose to learn a robust and generalized model with limited annotations. Extensive experiments demonstrate the superiority of the proposed methods on each task.
Attend the Event
This is a free online event open to everyone. You can attend via Zoom