11am - 12 noon
Wednesday 21 September 2022
Parametric Human Modelling for Shape and Texture Representation from Video
PhD Open Viva Presentation by Lewis Bridgeman
All Welcome!
Free
University of Surrey
Guildford
Surrey
GU2 7XH
This event has passed
Speakers
Parametric Human Modelling for Shape and Texture Representation from Video
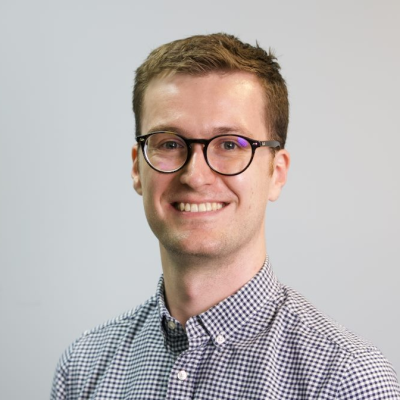
Abstract:
The modelling of human geometry and appearance from videos has been a focus of computer vision researchers for decades, driven by an exciting range of possible applications in video games, healthcare and the TV & film industry. Research in this field has advanced on two fronts: bottom-up methods that reconstruct human geometry from raw image data; and top-down approaches that explain the image data with existing models of human shape. There has been an increased focus into model-based approaches over the last 5 years, largely due to the emergence of new statistical models of human shape and pose - notably the SMPL model - and also due to advances in human joint estimation from images. While model-free human reconstruction methods have been mostly limited to constrained environments, model-based methods can exploit priors in the statistical body model to capture the human geometry in more challenging scenarios, including: monocular video, partially occluded images, and multiple people. In this thesis we demonstrate the advantage of parametric human models in representing the shape and texture of humans from video input, by applying them to a range of tasks.
The first contribution demonstrates the effectiveness of a parametric human body model as a tool for generating free-viewpoint video renderings of humans in motion. In this chapter we introduce an optimisation framework for aligning the SMPL body model with multi-view video of a human capture in a studio. The model-based approach consistently provides a full-body reconstruction with fine details around the face and hands. Further, the model-based pipeline allows for considerable compression of the reconstructed sequence: the geometry is encoded simply as a set of model parameters; and the model structure provides a temporally consistent texture map layout, allowing for efficient video compression of the human appearance. These benefits allow for efficient and computationally inexpensive playback in a game engine, and in virtual reality.
The second contribution is the model-based reconstruction of multiple people in sports. Sports datasets are especially challenging due to multiple interacting players, heavy occlusion, low effective player resolution and poor calibration. To extend our reconstruction pipeline to multiple people, we introduce an algorithm for the association of 2D pose estimations of multiple people between camera views. We also introduce a novel method for the correction of errors that are often associated with 2D pose estimates. Finally, we introduce an algorithm for the tracking of skeletons over time, which is robust to missing detections. We use the associated and temporally tracked pose detections in our model-based reconstruction pipeline to generate model-based reconstructions of multiple players in sports sequences, despite the heavy occlusion and low detail in the original footage.
The third contribution is a method for the capture and modelling of dynamic human texture appearance from a minimal set of input cameras. Previous methods to capture dynamic appearance of a human from multi-view video rely on large camera setups, and typically store texture on a per-frame basis. We generate a parametric reconstruction from minimal cameras (as few as 3) to generate partial texture observations. The parametric reconstruction provides a temporally consistent texture map layout, as well as the human pose each frame. The partial texture observations are combined in a learned framework to generate full-body textures with dynamic details given an input pose. Inspired by traditional multi-view texturing algorithms, we adopt a multi-band weighted loss function to train our network, which minimizes texture artefacts.
Our final contribution is a novel continuous displacement field representation for the reconstruction of clothed human body shape from a single image. Recent model-free monocular human shape estimation methods struggle with highly varied poses and occlusions, whereas parametric methods are more robust but limited to tight clothing. Our learnt continuous displacement field representation reconstructs detailed shape for humans in challenging poses. We combine local image features with canonical parametric body model coordinates to build a displacement field that models the distance between the underlying parametric model and the true human surface. Our ParamCDF representation is also able to handle the task of inferring detailed human shape from partially occluded images of humans.
Attend the Event
This is a hybrid free event open to everyone.