3pm - 4pm
Tuesday 18 October 2022
Towards Practicality of Sketch-Based Visual Understanding
PhD Open Presentation Viva by Ayan Bhunia
Free
This event has passed
Speakers
Towards Practicality of Sketch-Based Visual Understanding
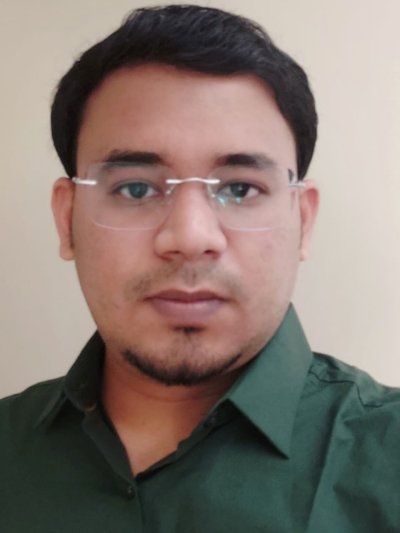
Abstract:
Sketches have been used to conceptualise and depict visual objects from pre-historic times. Sketch research has flourished in the past decade, particularly with the proliferation of touchscreen devices. Much of the utilisation of sketch has been anchored around the fact that it can be used to delineate visual concepts universally irrespective of age, race, language, or demography. The fine-grained interactive nature of sketches facilitates the application of sketches to various visual understanding tasks, like image retrieval, image-generation or editing, segmentation, 3D-shape modelling etc. However, sketches are highly abstract and subjective based on the perception of individuals. Although most agree that sketches provide fine-grained control to the user to depict a visual object, many consider sketching a tedious process due to their limited sketching skills compared to other query/support modalities like text/tags. Furthermore, collecting fine-grained sketch-photo association is a significant bottleneck to commercialising sketch applications. Therefore, this thesis aims to progress sketch-based visual understanding towards more practicality.
Being able to model fine-grained details of a visual concept easily, sketch understandably holds immense potential as a medium of query -- even better than texts that are at times insufficient to pin down fine-grained visual details. Out of all sketch-related applications therefore, fine-grained sketch-based image retrieval (FG-SBIR) has received the most attention, due to its significant commercial potential in the retail industry. FG-SBIR aims at retrieving a particular photo instance given a user’s query sketch, out of a gallery of photos having a particular category. Given the dominant prevalence of touchscreen devices now, the world is already primed for using sketch as a practical query modality for fine-grained retrieval. However, talking of applicability at an industrial scale, practically using sketch as a query-medium for retrieval is yet to gain traction due to a few significant barriers. Breaking these barriers, this thesis addresses the practicality of FG-SBIR via two themes putting forth five major contributions. The first theme comprises three contributions which focus particularly on the practical deployment of FG-SBIR which is one of the major forefronts of sketch research. The second theme caters to the widespread applicability of sketches for real-world applications, consisting of two more contributions.
In the first theme, our first chapter starts by figuring out that the widespread applicability of FG-SBIR is hindered as drawing a sketch takes time, and most people struggle to draw a complete and faithful sketch. We thus reformulate the conventional FG-SBIR framework to tackle these challenges, with the ultimate goal of retrieving the target photo with the least number of strokes possible. We further propose an on-the-fly design that starts retrieving as soon as the user starts drawing. Accordingly, we devise a reinforcement learning-based cross-modal retrieval framework that optimises the ground-truth photo's rank over a complete sketch drawing episode.
The second chapter discovers that the lack of sketch-photo pairs largely bottlenecks FG-SBIR performance. We therefore introduce a novel semi-supervised framework for instance-level cross-modal retrieval that can leverage large-scale unlabelled photos to account for data scarcity. The core of our semi-supervision design is a sequential photo-to-sketch generation model that aims to generate paired sketches for unlabelled photos. We further introduce a discriminator guided mechanism to guide against unfaithful generation, together with a distillation loss-based regulariser to provide tolerance against noisy training samples.
Thirdly, we notice that the fear-to-sketch problem (i.e., “I can’t sketch”) has proven to be fatal for the widespread adoption of fine-grained SBIR. A pilot study revealed that the secret lies in the existence of noisy strokes, but not so much of the “I can’t sketch”. We thus design a stroke subset selector that detects noisy strokes, leaving only those that positively contribute to a successful retrieval. Our Reinforcement Learning based formulation quantifies the importance of each stroke present in a given subset, based on the extent to which that stroke contributes to retrieval.
Moving on to our second theme, in the fourth chapter we focus on learning powerful representations via self-supervised learning from unlabelled data, thus increasing the scope of sketches. Towards this, we advocate for the dual-modality nature of sketches -- rasterized images and vector coordinate sequences, which is pivotal in designing a self-supervised pre-text task for our goal. We address this dual representation by proposing two novel cross-modal translation pre-text tasks for self-supervised feature learning: Vectorization and Rasterization. Vectorization learns to map image space to vector coordinates, and rasterization the opposite. We show that our learned encoder modules benefit both raster-based and vector-based downstream tasks to analysing hand-drawn data.
The final chapter further contributes to the second theme by opening up a new avenue of sketch research with a novel sketch-based visual understanding task. Here we dictate the potential of sketch as a support modality for few-shot class incremental learning (FSCIL) -- a setup that explicitly highlights the relevance of sketch as an information-modality. Pushing FSCIL further, we address two key questions that bottleneck its ubiquitous application (i) can the model learn from diverse modalities other than just photo (as humans do), and (ii) what if photos are not readily accessible (due to ethical and privacy constraints). The product is a “Doodle It Yourself” (DIY) FSCIL framework where the users can freely sketch a few examples of a novel class for the model to learn recognising photos of that class.
Attend the Event
This is a free online event to everyone. You can attend via Zoom