2pm - 3pm
Wednesday 30 November 2022
Defocus Modelling for 3D Reconstruction and Rendering
PhD Open Viva Presentation by Mr Matthew Bailey
All welcome
Free
University of Surrey
Guildford
Surrey
GU2 7XH
This event has passed
Defocus Modelling for 3D Reconstruction and Rendering
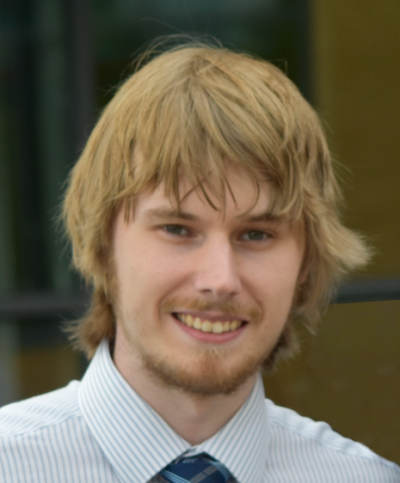
Abstract:
Defocus formation from a finite aperture is a well-known phenomenon, occurring in many forms of photographic media. Although defocus is often exploited for artistic reasons, a surprising amount of information about the scene structure is encoded in the camera’s blurring function. The aim of this work is to explore how this can be leveraged to recover 3D geometry from scenes with complex reflectance.
Depth from defocus (DFD) is a well-established field that aims to reconstruct scene geometry from analysis of the defocus appearance, usually by modelling the camera as a thin lens. While many existing methods achieve approximate depth maps suitable for some applications, the majority are limited to geometrically inconsistent single-view reconstructions. The first contribution generalises image formation to a thick lens, and proposes a novel calibration procedure for accurate defocus modelling. This approach is shown to significantly outperform traditional thin lens assumptions in macro-scale scene reconstruction.
The second contribution generalises reconstruction to multiple views, and evaluates the complementary properties of defocus and stereo information in a novel reconstruction framework. Unlike conventional multi-view stereo (MVS), which depends on photometric consistency between views, DFD requires only a single viewpoint for reconstruction. This makes defocus-based approaches naturally robust to view-dependent materials considered challenging for traditional MVS. Conversely, textures which are invariant to defocus can be suitable for correspondence. This complementary relationship is investigated to determine the benefits of combining defocus information with stereo cues; with performance evaluated on per-viewpoint depth maps as well as complete 3D reconstructions. The results demonstrate an improvement over DFD alone even in specular and reflective datasets, and outperforms state-of-the-art MVS.
The third contribution explores the novel application of neural rendering to defocus modelling. Specifically, the recent advances in deep learning are leveraged to solve for three latent variables encoded as pixel intensities in a focal stack: the scene depth and radiance, and the camera point spread function. This is in contrast to the majority of existing defocus-based literature, which assume at least one of these variables is known. These quantities are disentangled by modelling each as a multilayer perception network, and trained end-to-end on the appearance of each pixel under different camera settings. This approach allows novel refocused images to be rendered that accurately capture the bokeh produced by specular highlights with arbitrary aperture shapes. Since the networks are trained according to a convolutional defocus model, the synthesised images can generalise to unconstrained aperture diameters, and achieve depth of field effects that exceed what was observed during training.
Attend the event
Hybrid event open to everyone. You can attend in person or online.