11am - 12 noon
Tuesday 10 January 2023
Multi-task autonomy for robotics
PhD Viva Open Presentation by Xihan Bian.
All welcome!
Free
University of Surrey
Guildford
Surrey
GU2 7XH
This event has passed
Abstract
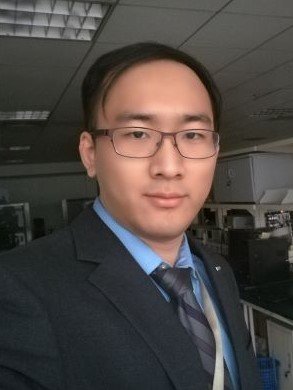
Robotic technology is integrating into more aspects of our society. The ability to perform multiple different functions in various environments will be an important research challenge. Recent developments in multi-task learning in artificial intelligence (AI) and machine learning could enable a new generation of robotic technology.
This thesis aims to explore techniques which combine AI with robotics to enable generalisation across a range of environments. As the level of abstraction possible in deep learning has grown a very promising avenue of research has become feasible: hybrid systems. In these approaches, an AI system is used to undertake high-level planning and strategisation, while the execution of the plans is undertaken by classical non-learned approaches.
This enables a mutual compensation of AI and traditional robotic systems, as the AI agents excel at generalisation for high-level problems and long term goal-oriented planning, while the robotics techniques specialise in precise and repeatable operations. This thesis attempts to build upon this and further explore the multi-task-enabled AI agent and its potential in robotic tasks.
First, general-purpose robots need to be able to work in multiple different environments. Even when performing similar tasks, different behaviour should be deployed to best fit the current environment. In this thesis, we propose a new approach to navigation, where it is treated as a multi-task learning problem. This enables the robot to learn to behave differently in visual navigation tasks for different environments while also learning shared expertise across environments. We evaluate our approach in both simulated environments as well as real-world data.
Following this, we introduce a new perspective for learning transferable content in multi-task imitation learning. Humans are able to transfer skills and knowledge to new tasks. If we can cycle to work and drive to the store, we can also cycle to the store and drive to work. We take inspiration from this and hypothesize the latent memory of a policy network can be disentangled into two partitions.
These contain either the knowledge of the environmental context for the task or the generalizable skill needed to solve the task. This allows improved training efficiency and better generalization over previously unseen combinations of skills in the same environment, and the same task in unseen environments.
We used the proposed approach to train a disentangled agent for two different multi-task IL environments. In both cases, we outperformed the SOTA by 30% in task success rate. We also demonstrated this for navigation on a real robot, including the visual navigation task of the previous chapter. This demonstration was also ported to a real robot.
This ability to generalize to previously unseen tasks with little to no supervision is a key challenge in modern machine learning research. Researchers often rely on reinforcement and imitation learning to provide online adaptation to new tasks, through trial and error learning. However, this can be challenging for complex tasks which require many timesteps or large numbers of subtasks to complete.
These “long horizon” tasks suffer from sample inefficiency and can require extremely long training times before the agent can learn to perform the necessary long-term planning. Therefore, we finally introduce CASE which attempts to address these issues by utilising adaptive “near future” subgoals.
These subgoals are re-calculated at each step using compositional arithmetic in a learned latent representation space similar to the previous chapter. In addition to improving learning efficiency for standard long-term tasks, this approach also makes it possible to perform one-shot generalization to previously unseen tasks, given only a single reference trajectory for the task in a different environment.
When we combine the works in this thesis, these contributions provide insights for a better multitask generalization framework for long and complex robotic planning. The contributions centre on multi-task learning and crossover to robotic navigation, reinforcement/imitation learning, and generalization. The experiments in this thesis demonstrate that an AI-controlled robot can perform simple tasks as well as a non-AI robot, while also handling more difficult tasks. This thesis presents a general direction for robotic AI capable of handling multiple complex tasks and sequential generalization. This enables better autonomy in both AI and robotics in the future.
Attend the Event
This is a free hybrid event open to everyone.
Visitor information
Find out how to get to the University, make your way around campus and see what you can do when you get here.