11am - 12 noon
Friday 29 September 2023
Learned representations of artistic style for image retrieval, description and stylization
PhD Viva Open Presentation by Dan Ruta.
All welcome!
Free
University of Surrey
Guildford
Surrey
GU2 7XH
This event has passed
Abstract
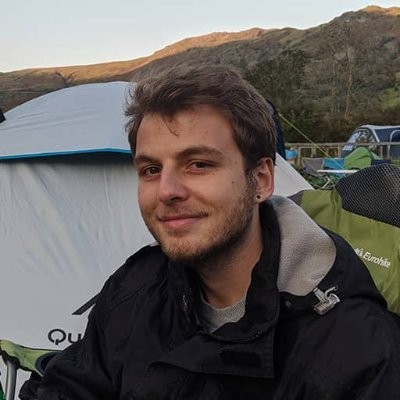
This thesis establishes a comprehensive framework by bridging various areas of machine learning research through a shared representation of artistic style. Our first contribution explores ways to learn a representation of visual artistic style, in our ALADIN model. We experiment with various degrees of supervision, using our novel BAM-FG dataset. We pursue a fine-grained representation to model a highly expressive metric embedding space able to discriminate between small nuances in artistic style. We demonstrate the strengths of weak supervision for this task, using the fine-grained style groupings in BAM-FG.
We pursue multiple downstream research directions with this embedding, such as stylebased image retrieval, where visual search can focus on purely the artistic style, for the first time. We further extend our representation research into cross-modal learning, bridging a connection between vision and language modalities in StyleBabel. By applying our ALADIN representation, we can adapt machine learning techniques to perform automatic tagging, natural language captioning, and tag-based visual search - again for the first time purely in the artistic style domain.
We also extend our research to generative applications, exploring ways of integrating a shared style modality representation into the process. In our NeAT project, we explore using ALADIN as a pre-processing step in curating the first ever large scale high resolution and diverse Neural Style Transfer (NST) dataset. This was crucial in extending NST to achieve state-of-the-art quality and generality. We also show how ALADIN can be used as a conditioning factor in driving stylization in generative models, by exploring the use of HyperNetworks in our HyperNST project. This novel approach induces metric style control capabilities over existing StyleGAN models, enabling novel ways of controlling stylization.
Finally, we again demonstrate the merits of a style embedding for style conditioning in diffusion-based generative models. We build DIFF-NST to leverage the Stable Diffusion model to not only guide stylization using ALADIN, but to also achieve a wider gamut of style changes. For the first time, we introduce a general method to achieve style-based form deformation in NST, extending our field’s stylization capabilities to a broader set of style factors, pushing past previous limitations in definitions of style in NST. Through our contributions in this thesis, we address the challenge of representation of artistic style, unifying downstream tasks for style through shared representation. We leverage this representation to extend state-of-the-art for multiple downstream tasks. We document our findings, and propose future directions for the field.
Visitor information
Find out how to get to the University, make your way around campus and see what you can do when you get here.