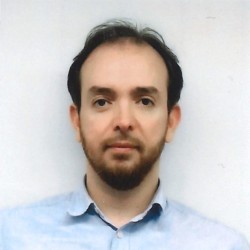
Dr Alaa Marshan
Academic and research departments
Nature Inspired Computing and Engineering Research Group, Computer Science Research Centre, School of Computer Science and Electronic Engineering.About
Biography
Dr Alaa Marshan is a Senior Lecturer at the Computer Science Department, where he teaches a variety of topics related to data science and machine learning. During his PhD, he worked on designing, developing, implementing and evaluating analytical models for data analysis in the banking domain as part of the Semantic Credit Risk Assessment of Business Ecosystems (SCRIBE) project using Social Network Analysis (SNA) and Machine Learning (ML) Techniques. He was also appointed as Primary Investigator (PI) on KTP project titled "Emotion AI for trading on Financial Markets".
My qualifications
Affiliations and memberships
ResearchResearch interests
Alaa Marshan's research focuses primarily on intelligent data analysis, information management and improving operational business information systems. He has a specific interest in applying Social Network Analysis (SNA), and Machine Learning (ML) techniques in various research domains such as Finance and Healthcare to support information inferencing and decision making; developing new methods and models to analyse large transactional-based datasets and enhancing human sense-making within organisational settings for better decision-making.
Research interests
Alaa Marshan's research focuses primarily on intelligent data analysis, information management and improving operational business information systems. He has a specific interest in applying Social Network Analysis (SNA), and Machine Learning (ML) techniques in various research domains such as Finance and Healthcare to support information inferencing and decision making; developing new methods and models to analyse large transactional-based datasets and enhancing human sense-making within organisational settings for better decision-making.
Teaching
Teaching responsibilities include:
- Practical Business Analytics (COMM053 - COM3018) - Module convenor.
- Data Science Principles and Practices (COMM054) - Co-module convenor.
- MSc Dissertation (COMM002) - Module coordinator.
Publications
Social media platforms have become an increasingly popular tool for individuals to share their thoughts and opinions with other people. However, very often people tend to misuse social media posting abusive comments. Abusive and harassing behaviours can have adverse effects on people's lives. This study takes a novel approach to combat harassment in online platforms by detecting the severity of abusive comments, that has not been investigated before. The study compares the performance of machine learning models such as Naïve Bayes, Random Forest, and Support Vector Machine, with deep learning models such as Convolutional Neural Network (CNN) and Bi-directional Long Short-Term Memory (Bi-LSTM). Moreover, in this work we investigate the effect of text pre-processing on the performance of the machine and deep learning models, the feature set for the abusive comments was made using unigrams and bigrams for the machine learning models and word embeddings for the deep learning models. The comparison of the models’ performances showed that the Random Forest with bigrams achieved the best overall performance with an accuracy of (0.94), a precision of (0.91), a recall of (0.94), and an F1 score of (0.92). The study develops an efficient model to detect severity of abusive language in online platforms, offering important implications both to theory and practice.
Introduction In response to the increasing prevalence of electronic medical records (EMRs) stored in databases, healthcare staff are encountering difficulties retrieving these records due to their limited technical expertise in database operations. As these records are crucial for delivering appropriate medical care, there is a need for an accessible method for healthcare staff to access EMRs.Methods To address this, natural language processing (NLP) for Text-to-SQL has emerged as a solution, enabling non-technical users to generate SQL queries using natural language text. This research assesses existing work on Text-to-SQL conversion and proposes the MedT5SQL model specifically designed for EMR retrieval. The proposed model utilizes the Text-to-Text Transfer Transformer (T5) model, a Large Language Model (LLM) commonly used in various text-based NLP tasks. The model is fine-tuned on the MIMICSQL dataset, the first Text-to-SQL dataset for the healthcare domain. Performance evaluation involves benchmarking the MedT5SQL model on two optimizers, varying numbers of training epochs, and using two datasets, MIMICSQL and WikiSQL.Results For MIMICSQL dataset, the model demonstrates considerable effectiveness in generating question-SQL pairs achieving accuracy of 80.63%, 98.937%, and 90% for exact match accuracy matrix, approximate string-matching, and manual evaluation, respectively. When testing the performance of the model on WikiSQL dataset, the model demonstrates efficiency in generating SQL queries, with an accuracy of 44.2% on WikiSQL and 94.26% for approximate string-matching.Discussion Results indicate improved performance with increased training epochs. This work highlights the potential of fine-tuned T5 model to convert medical-related questions written in natural language to Structured Query Language (SQL) in healthcare domain, providing a foundation for future research in this area.