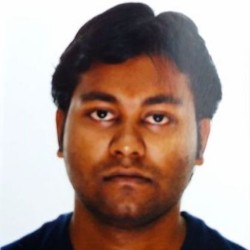
Amit Kumar Jaiswal
Academic and research departments
Surrey Academy for Blockchain and Metaverse Applications, Surrey Business School.About
Biography
Amit was trained as a computer scientist, and he held the Marie Skłodowska Curie Early Stage fellowship for his Ph.D. in Quantum-inspired Information Retrieval from the University of Bedfordshire in 2022. His graduate work is specifically in the field of information retrieval and quantum probabilistic modelling. He worked on several topics as part of his doctoral research, including user behavioural signals across various types of search systems (text/image and multimodal search), retrieval, and recommendations. Earlier, he was a Postdoctoral fellow at University College London developing temporal multimodal deep neural networks for Prostate cancer progression and collaborated with clinicians in the Radiology department at the University of Cambridge on risk stratification of future Prostate cancer. Before postdoc, he worked in the department of Statistics within School of Mathematics at the University of Leeds on an industry-led (Credit Suisse, Finastra) Financial AI project as a research engineer on building production-grade synthetic data generator for mortgage lending and stock portfolio use-cases.
University roles and responsibilities
- Postdoctoral Research Fellow
Previous roles
ResearchResearch interests
His current focus is at the intersection of machine learning and information access/retrieval to a broad set of problems, focussed on multimodal search and recommendations.
More recently, he has also begun working on applications of machine learning to healthcare and financial topics connected with information representation and modelling, such as disease (Prostate cancer, Alzheimer's) progression prediction, cognitive functions during context switching in the brain, user behaviours and risks in decentralised financial markets, and the applications of language models for conversational agents.
Research interests
His current focus is at the intersection of machine learning and information access/retrieval to a broad set of problems, focussed on multimodal search and recommendations.
More recently, he has also begun working on applications of machine learning to healthcare and financial topics connected with information representation and modelling, such as disease (Prostate cancer, Alzheimer's) progression prediction, cognitive functions during context switching in the brain, user behaviours and risks in decentralised financial markets, and the applications of language models for conversational agents.
Publications
Traditional neural word embeddings are usually dependent on a richer diversity of vocabulary. However, the language models recline to cover major vocabularies via the word embedding parameters, in particular, for multilingual language models that generally cover a significant part of their overall learning parameters. In this work, we present a new compact embedding structure to reduce the memory footprint of the pre-trained language models with a sacrifice of up to 4% absolute accuracy. The embeddings vectors reconstruction follows a set of subspace embeddings and an assignment procedure via the contextual relationship among tokens from pre-trained language models. The subspace embedding structure1 calibrates to masked language models, to evaluate our compact embedding structure on similarity and textual entailment tasks, sentence and paraphrase tasks. Our experimental evaluation shows that the subspace embeddings achieve compression rates beyond 99.8% in comparison with the original embeddings for the language models on XNLI and GLUE benchmark suites.
We contribute the first publicly available dataset of factual claims from different platforms and fake YouTube videos on the 2023 Israel-Hamas war for automatic fake YouTube video classification. The FakeClaim data is collected from 60 fact-checking organizations in 30 languages and enriched with metadata from the fact-checking organizations curated by trained journalists specialized in fact-checking. Further, we classify fake videos within the subset of YouTube videos using textual information and user comments. We used a pre-trained model to classify each video with different feature combinations. Our best-performing fine-tuned language model, Universal Sentence Encoder (USE), achieves a Macro F1 of 87%, which shows that the trained model can be helpful for debunking fake videos using the comments from the user discussion.
Item representation holds significant importance in recommendation systems, which encompasses domains such as news, retail, and videos. Retrieval and ranking models utilise item representation to capture the user-item relationship based on user behaviours. While existing representation learning methods primarily focus on optimising item-based mechanisms, such as attention and sequential modelling. However, these methods lack a modelling mechanism to directly reflect user interests within the learned item representations. Consequently, these methods may be less effective in capturing user interests indirectly. To address this challenge, we propose a novel Interest-aware Capsule network (IaCN) recommendation model, a model-agnostic framework that directly learns interest-oriented item representations. IaCN serves as an auxiliary task, enabling the joint learning of both item-based and interest-based representations. This framework adopts existing recommendation models without requiring substantial redesign. We evaluate the proposed approach on benchmark datasets, exploring various scenarios involving different deep neural networks, behaviour sequence lengths, and joint learning ratios of interest-oriented item representations. Experimental results demonstrate significant performance enhancements across diverse recommendation models, validating the effectiveness of our approach.
The visitor economy is responsible for a substantial percentage of the global carbon footprint. The mechanisms used to decarbonize it are insufficient, and the industry is relying on carbon trading with substandard credits that allow businesses to outsource the responsibility to decarbonize. We aim to transform carbon markets, help finance climate investments, and support decarbonization strategies. We identify and define the problem, outline the components and their interactions, and develop a conceptual model to transform carbon markets. The new, blockchain-based Carbon Tokenomics Model rolls out a decentralized database to store, trade, and manage carbon credits, with the goal of enabling sustainable climate finance investment. We outline the criteria needed for an industry-wide carbon calculator. We explain the process needed to increase rigor in climate investments in the visitor economy and introduce a delegated Proof of Commitment consensus mechanism. Our inclusive and transparent model illustrates how to reduce transaction costs and how to build consumer and industry trust, generating much-needed investments for decarbonization.
Item representation holds significant importance in recommendation systems, which encompasses domains such as news, retail, and videos. Retrieval and ranking models utilise item representation to capture the user-item relationship based on user behaviours. While existing representation learning methods primarily focus on optimising item-based mechanisms, such as attention and sequential modelling. However, these methods lack a modelling mechanism to directly reflect user interests within the learned item representations. Consequently, these methods may be less effective in capturing user interests indirectly. To address this challenge, we propose a novel Interest-aware Capsule network (IaCN) recommendation model, a model-agnostic framework that directly learns interest-oriented item representations. IaCN serves as an auxiliary task, enabling the joint learning of both item-based and interest-based representations. This framework adopts existing recommendation models without requiring substantial redesign. We evaluate the proposed approach on benchmark datasets, exploring various scenarios involving different deep neural networks, behaviour sequence lengths, and joint learning ratios of interest-oriented item representations. Experimental results demonstrate significant performance enhancements across diverse recommendation models, validating the effectiveness of our approach.