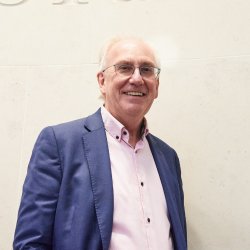
Professor Anthony Whetton
News
Publications
AbstractBackground and HypothesisTreatment response to specific antipsychotic medications is difficult to predict on clinical grounds alone. The current study hypothesizes that the baseline complement pathway activity predicts the treatment response and investigates the relationship between baseline plasma biomarkers with treatment response to antipsychotic medications.Study DesignBaseline plasma samples were collected from first episode of psychosis patients (n = 243) from a multi-center clinical trial. The participants were treated with amisulpride for 4 weeks. Levels of complement and coagulation proteins at baseline were measured using both data-dependent and data-independent mass spectrometry approaches. The primary outcome was remission status at 4 weeks and the secondary outcomes included change in psychotic and functional symptoms over the period of treatment. In addition, immunoassays were performed at baseline for complement C1R, as well as for activation markers C4a and sC5b-9.Study ResultsThe plasma level of complement variant C4A was significantly associated with remission at 4 weeks. Moreover, higher levels of several complement and coagulation pathway proteins were associated with a reduction in psychotic symptoms and an improvement in functioning. Immunoassays showed an association of baseline levels of C1R and C4a as well as complement activation marker sC5b-9 levels with treatment response.ConclusionThe results demonstrated that the response to antipsychotic treatment might be related to pre-treatment levels of plasma complement and coagulation pathway proteins. This is consistent with independent evidence associating immune dysfunction with the pathophysiology of psychosis. Moreover, these results inform the development of novel therapeutic approaches that target the complement system for psychosis.
Consumption of Plant-Based Meat Alternatives (PBMAs) within the vegetarian population is increasing. This study assessed the relationship between PBMA intake and health markers using the UK Biobank cohort. Participants were categorized into vegetarian PBMA consumers and vegetarian PBMA non-consumers. Non-parametric statistical tests were used to evaluate differences in participants’ characteristics, food intake, 30 blood biochemistry measures after assessing data distributions. Metabolomics (168 metabolites) and proteomics (2,923 proteins) data were further examined to identify significant differences between the two participant groups. Relative Risks (RRs) for 45 chronic diseases and mental conditions were calculated using Poisson regression. Sensitivity analysis accounted for sociodemographic factors and the proportion of energy from Ultra-Processed Food (UPF) intake was determined. No substantial differences in sodium, free sugar, total sugar, or saturated fatty acids intake between vegetarian PBMA consumers and non-consumers were found. However, PBMA consumers exhibited higher blood pressure (130/79 mmHg and 129/78 mmHg for consumer and non-consumer groups, respectively) and elevated C-reactive protein (CRP) levels (1.76±3.12 mg/L and 1.57±3.17 mg/L for consumer and non-consumer groups, respectively). Metabolite and protein abundance analysis showed no notable differences. Pathway enrichment analysis suggested that PBMAs may influence immune reactions through cell signalling pathways. PBMA consumers had a 42% increased risk of depression (p=0.03) and 40% reduction in irritable bowel syndrome (IBS) risk (p=0.02), compared to non-consumers. In conclusion, while no clear health risks or benefits were associated with PBMA consumption in vegetarians, the higher risk of depression, elevated C-reactive protein, and lower apolipoprotein A levels in PBMA consumers suggest potential inflammatory concerns that warrant further investigation.
Chronic kidney disease (CKD) is characterised by progressive kidney damage and encompasses a broad range of renal pathologies and aetiologies. In humans, CKD is an increasing global health problem, in particular in the western world, while in cats and dogs, CKD is one of the leading causes of mortality and morbidity. Here, we aimed to develop an enhanced understanding of the knowledge base related to the pathophysiology of renal disease and CKD in cats and dogs. To achieve this, we leveraged a text-mining approach for reviewing trends in the literature and compared the findings to evidence collected from publications related to CKD in humans. Applying a quantitative text-mining technique, we examined data on clinical signs, diseases, clinical and lab methods, cell types, cytokine, and tissue associations (co-occurrences) captured in PubMed biomedical literature. Further, we examined different types of pain within human CKD-related publications, as publications on this topic are sparser in companion animals, but with the growing importance of animal welfare and quality of life, it is an area of interest. Our findings could serve as substance for future research studies. The systematic automated review of relevant literature, along with comparative analysis, has the potential to summarise scientific evidence and trends in a quick, easy, and cost-effective way. Using this approach, we identified targeted and novel areas of investigation for renal disease in cats and dogs.
The use of technologies that provide objective, digital data to clinicians, carers, and service users to improve care and outcomes comes under the unifying term Digital Health. This field, which includes the use of high-tech health devices, telemedicine and health analytics has, in recent years, seen significant growth in the United Kingdom and worldwide. It is clearly acknowledged by multiple stakeholders that digital health innovations are necessary for the future of improved and more economic healthcare service delivery. Here we consider digital health-related research and applications by using an informatics tool to objectively survey the field. We have used a quantitative text-mining technique, applied to published works in the field of digital health, to capture and analyse key approaches taken and the diseases areas where these have been applied. Key areas of research and application are shown to be cardiovascular, stroke, and hypertension; although the range seen is wide. We consider advances in digital health and telemedicine in light of the COVID-19 pandemic.
We report the first demonstration of a microfluidics-based approach to measure lipids in single living cells using widely available liquid chromatography mass spectrometry (LC-MS) instrumentation. The method enables the rapid sorting of live cells into liquid chambers formed on standard Petri dishes and their subsequent dispensing into vials for analysis using LC-MS. This approach facilitates automated sampling, data acquisition, and analysis and carries the additional advantage of chromatographic separation, aimed at reducing matrix effects present in shotgun lipidomics approaches. We demonstrate that our method detects comparable numbers of features at around 200 lipids in populations of single cells versus established live single-cell capillary sampling methods and with greater throughput, albeit with the loss of spatial resolution. We also show the importance of optimization steps in addressing challenges from lipid contamination, especially in blanks, and demonstrate a 75% increase in the number of lipids identified. This work opens up a novel, accessible, and high-throughput way to obtain single-cell lipid profiles and also serves as an important validation of single-cell lipidomics through the use of different sampling methods.
Treatments for COVID-19 infections have improved dramatically since the beginning of the pandemic, and glucocorticoids have been a key tool in improving mortality rates. The UK’s National Institute for Health and Care Excellence guidance is for treatment to be targeted only at those requiring oxygen supplementation, however, and the interactions between glucocorticoids and COVID-19 are not completely understood. In this work, a multi-omic analysis of 98 inpatient-recruited participants was performed by quantitative metabolomics (using targeted liquid chromatography-mass spectrometry) and data-independent acquisition proteomics. Both ‘omics datasets were analysed for statistically significant features and pathways differentiating participants whose treatment regimens did or did not include glucocorticoids. Metabolomic differences in glucocorticoid-treated patients included the modulation of cortisol and bile acid concentrations in serum, but no alleviation of serum dyslipidemia or increased amino acid concentrations (including tyrosine and arginine) in the glucocorticoid-treated cohort relative to the untreated cohort. Proteomic pathway analysis indicated neutrophil and platelet degranulation as influenced by glucocorticoid treatment. These results are in keeping with the key role of platelet-associated pathways and neutrophils in COVID-19 pathogenesis and provide opportunity for further understanding of glucocorticoid action. The findings also, however, highlight that glucocorticoids are not fully effective across the wide range of ‘omics dysregulation caused by COVID-19 infections.
Background: Specific food preferences can determine an individual’s dietary patterns and therefore, may be associated with certain health risks and benefits. Methods: Using food preference questionnaire (FPQ) data from a subset comprising over 180,000 UK Biobank participants, we employed Latent Profile Analysis (LPA) approach to identify the main patterns or profiles among participants. blood biochemistry across groups/profiles was compared using the non-parametric Kruskal-Wallis test. We applied the Limma algorithm for differential abundance analysis on 168 metabolites and 2923 proteins, and utilized the Database for Annotation, Visualization and Integrated Discovery (DAVID) to identify enriched biological processes and pathways. Relative risks (RR) were calculated for chronic diseases and mental conditions per group, adjusting for sociodemographic factors.Results: Based on their food preferences, three profiles were termed: the putative Health-conscious group (low preference for animal-based or sweet foods, and high preference for vegetables and fruits), the Omnivore group (high preference for all foods), and the putative Sweet-tooth group (high preference for sweet foods and sweetened beverages). The Health-conscious group exhibited lower risk of heart failure (RR = 0.86, 95%CI 0.79 – 0.93) and chronic kidney disease (RR = 0.69, 95%CI 0.65 – 0.74) compared to the two other groups. The Sweet-tooth group had greater risk of depression (RR = 1.27, 95%CI 1.21 – 1.34), diabetes (RR = 1.15, 95%CI 1.01 – 1.31), and stroke (RR = 1.22, 95%CI 1.15 – 1.31) compared to the other two groups. Cancer (overall) relative risk showed little difference across the Health-conscious, Omnivore, and Sweet-tooth groups with RR of 0.98 (95%CI 0.96 – 1.01), 1.00 (95%CI 0.98 – 1.03), and 1.01 (95%CI 0.98 – 1.04), respectively. The Health-conscious group was associated with lower levels of inflammatory biomarkers (e.g., C-reactive Protein) which are also known to be elevated in those with common metabolic diseases (e.g., cardiovascular disease). Other markers modulated in the Health-conscious group, ketone bodies, insulin-like growth factor-binding protein (IGFBP), and Growth Hormone 1 were more abundant, while leptin was less abundant. Further, the IGFBP pathway, which influences IGF1 activity, may be significantly enhanced by dietary choices. Conclusions: These observations align with previous findings from studies focusing on weight loss interventions, which include a reduction in leptin levels. Overall, the Health-conscious group, with preference to healthier food options, has better health outcomes, compared to Sweet-tooth and Omnivore groups.
The effect of COVID-19 infection on the human metabolome has been widely reported, but to date all such studies have focused on a single wave of infection. COVID-19 has generated numerous waves of disease with different clinical presentations, and therefore it is pertinent to explore whether metabolic disturbance changes accordingly, to gain a better understanding of its impact on host metabolism and enable better treatments. This work used a targeted metabolomics platform (Biocrates Life Sciences) to analyze the serum of 164 hospitalized patients, 123 with confirmed positive COVID-19 RT-PCR tests and 41 providing negative tests, across two waves of infection. Seven COVID-19-positive patients also provided longitudinal samples 2–7 months after infection. Changes to metabolites and lipids between positive and negative patients were found to be dependent on collection wave. A machine learning model identified six metabolites that were robust in diagnosing positive patients across both waves of infection: TG (22:1_32:5), TG (18:0_36:3), glutamic acid (Glu), glycolithocholic acid (GLCA), aspartic acid (Asp) and methionine sulfoxide (Met-SO), with an accuracy of 91%. Although some metabolites (TG (18:0_36:3) and Asp) returned to normal after infection, glutamic acid was still dysregulated in the longitudinal samples. This work demonstrates, for the first time, that metabolic dysregulation has partially changed over the course of the pandemic, reflecting changes in variants, clinical presentation and treatment regimes. It also shows that some metabolic changes are robust across waves, and these can differentiate COVID-19-positive individuals from controls in a hospital setting. This research also supports the hypothesis that some metabolic pathways are disrupted several months after COVID-19 infection.
Aberrantly high expression ofEVI1in acute myeloid leukaemia (AML) is associated with poor prognosis. For targeted treatment ofEVI1overexpressing AML a more detailed understanding of aspects of spatiotemporal interaction dynamics of the EVI1 protein is important. EVI1 overexpressing SB1690CB AML cells were used for quantification and protein interaction studies of EVI1 and Delta EVI1. Cells were cell cycle-synchronised by mimosine and nocodazole treatment and expression of EVI1 and related proteins assessed by western blot, immunoprecipitation and immunofluorescence. EVI1 protein levels oscillate through the cell cycle, and EVI1 is degraded partly by the proteasome complex. Both EVI1 and Delta EVI1 interact with the co-repressor CtBP1 but dissociate from CtBP1 complexes during mitosis. Furthermore, a large fraction of EVI1, but not Delta EVI1 or CtBP1, resides in the nuclear matrix. In conclusion, EVI1- protein levels and EVI1-CtBP1 interaction dynamics vary though the cell cycle and differ between EVI1 and Delta EVI1. These data ad to the functional characterisation of the EVI1 protein in AML and will be important for the development of targeted therapeutic approaches for EVI1-driven AML.
Background and Hypothesis Treatment response to specific antipsychotic medications is difficult to predict on clinical grounds alone. The current study hypothesizes that the baseline complement pathway activity predicts the treatment response and investigates the relationship between baseline plasma biomarkers with treatment response to antipsychotic medications. Study Design Baseline plasma samples were collected from first episode of psychosis patients (n = 243) from a multi-center clinical trial. The participants were treated with amisulpride for 4 weeks. Levels of complement and coagulation proteins at baseline were measured using both data-dependent and data-independent mass spectrometry approaches. The primary outcome was remission status at 4 weeks and the secondary outcomes included change in psychotic and functional symptoms over the period of treatment. In addition, immunoassays were performed at baseline for complement C1R, as well as for activation markers C4a and sC5b-9. Study Results The plasma level of complement variant C4A was significantly associated with remission at 4 weeks. Moreover, higher levels of several complement and coagulation pathway proteins were associated with a reduction in psychotic symptoms and an improvement in functioning. Immunoassays showed an association of baseline levels of C1R and C4a as well as complement activation marker sC5b-9 levels with treatment response. Conclusion The results demonstrated that the response to antipsychotic treatment might be related to pre-treatment levels of plasma complement and coagulation pathway proteins. This is consistent with independent evidence associating immune dysfunction with the pathophysiology of psychosis. Moreover, these results inform the development of novel therapeutic approaches that target the complement system for psychosis.
Endometrial cancer is the most common malignancy of the female genital tract and its incidence is rising in parallel with the mounting prevalence of obesity. Early diagnosis has great potential to improve outcomes as treatment can be curative, especially for early stage disease. Current tests and procedures for diagnosis are limited by insufficient accuracy in some and unacceptable levels of invasiveness and discomfort in others. There has, therefore, been a growing interest in the search for sensitive and specific biomarkers for endometrial cancer detection based on non-invasive sampling methodologies. Urine, the prototype non-invasive sample, is attractive for biomarker discovery as it is easily accessible and can be collected repeatedly and in quantity. Identification of urinary biomarkers for endometrial cancer detection relies on the excretion of systemic biomarkers by the kidneys or urinary contamination by biomarkers shed from the uterus. In this review, we present the current standing of the search for endometrial cancer urinary biomarkers based on cytology, genomic, transcriptomic, proteomic, and metabolomic platforms. We summarize the biomarker candidates and highlight the challenges inherent in urinary biomarker discovery. We review the various technologies with promise for biomarker detection and assess these novel approaches for endometrial cancer biomarker research.
Juvenile myelomonocytic leukemia (JMML) is an aggressive myeloproliferative neoplasm of early childhood with a poor survival rate, thus there is a requirement for improved treatment strategies. Induced pluripotent stem cells offer the ability to model disease and develop new treatment strategies. JMML is frequently associated with mutations in PTPN11. Children with Noonan syndrome, a development disorder, have an increased incidence of JMML associated with specific germline mutations in PTPN11. We undertook a proteomic assessment of myeloid cells derived from induced pluripotent stem cells obtained from Noonan syndrome patients with PTPN11 mutations, either associated or not associated with an increased incidence of JMML. We report that the proteomic perturbations induced by the leukemia-associated PTPN11 mutations are associated with TP53 and NF-I kappa b signaling. We have previously shown that MYC is involved in the differential gene expression observed in Noonan syndrome patients associated with an increased incidence of JMML. Thus, we employed drugs to target these pathways and demonstrate differential effects on clonogenic hematopoietic cells derived from Noonan syndrome patients, who develop JMML and those who do not. Further, we demonstrated these small molecular inhibitors, JQ1 and CBL0137, preferentially extinguish primitive hematopoietic cells from sporadic JMML patients as opposed to cells from healthy individuals.
Population-based prospective studies, such as UK Biobank, are valuable for generating and testing hypotheses about the potential causes of human disease. We describe how UK Biobank’s study design, data access policies, and approaches to statistical analysis can help to minimize error and improve the interpretability of research findings, with implications for other population-based prospective studies being established worldwide.
Bariatric surgery (BS) results in metabolic pathway recalibration. We have identified potential biomarkers in plasma of people achieving type 2 diabetes mellitus (T2DM) remission after BS. Longitudinal analysis was performed on plasma from 10 individuals following Roux-en-Y gastric bypass (n = 7) or sleeve gastrectomy (n = 3). Sequential window acquisition of all theoretical fragment ion spectra mass spectrometry (SWATH-MS) was done on samples taken at 4 months before (baseline) and 6 and 12 months after BS. Four hundred sixty-seven proteins were quantified by SWATH-MS. Principal component analysis resolved samples from distinct time points after selection of key discriminatory proteins: 25 proteins were differentially expressed between baseline and 6 months post-surgery; 39 proteins between baseline and 12 months. Eight proteins (SHBG, TF, PRG4, APOA4, LRG1, HSPA4, EPHX2 and PGLYRP) were significantly different to baseline at both 6 and 12 months post-surgery. The panel of proteins identified as consistently different included peptides related to insulin sensitivity (SHBG increase), systemic inflammation (TF and HSPA4—both decreased) and lipid metabolism (APOA4 decreased). We found significant changes in the proteome for eight proteins at 6- and 12-months post-BS, and several of these are key components in metabolic and inflammatory pathways. These may represent potential biomarkers of remission of T2DM.
BackgroundA non-invasive endometrial cancer detection tool that can accurately triage symptomatic women for definitive testing would improve patient care. Urine is an attractive biofluid for cancer detection due to its simplicity and ease of collection. The aim of this study was to identify urine-based proteomic signatures that can discriminate endometrial cancer patients from symptomatic controls.MethodsThis was a prospective case-control study of symptomatic post-menopausal women (50 cancers, 54 controls). Voided self-collected urine samples were processed for mass spectrometry and run using sequential window acquisition of all theoretical mass spectra (SWATH-MS). Machine learning techniques were used to identify important discriminatory proteins, which were subsequently combined in multi-marker panels using logistic regression.ResultsThe top discriminatory proteins individually showed moderate accuracy (AUC > 0.70) for endometrial cancer detection. However, algorithms combining the most discriminatory proteins performed well with AUCs > 0.90. The best performing diagnostic model was a 10-marker panel combining SPRR1B, CRNN, CALML3, TXN, FABP5, C1RL, MMP9, ECM1, S100A7 and CFI and predicted endometrial cancer with an AUC of 0.92 (0.96-0.97). Urine-based protein signatures showed good accuracy for the detection of early-stage cancers (AUC 0.92 (0.86-0.9)).ConclusionA patient-friendly, urine-based test could offer a non-invasive endometrial cancer detection tool in symptomatic women. Validation in a larger independent cohort is warranted.
Simple Summary Endometrial cancer is the most common cancer of the female reproductive tract, and its incidence is rising. Early diagnosis has the potential to improve survival as women can receive care at the earliest possible stage when curative treatment is likely. Current tests for endometrial cancer diagnosis are sequentially invasive with low patient acceptability. A detection tool based on minimally invasive samples such as cervico-vaginal fluid would be a major advance in the field. This study focuses on the potential of detecting endometrial cancer based on the proteins and peptides expressed in cervico-vaginal fluid. Using Sequential window acquisition of all theoretical mass spectra (SWATH-MS), we present a spectral library of thousands of proteins in the cervico-vaginal fluid of women with or at risk of endometrial cancer. This important resource will enable the identification of endometrial cancer biomarkers in cervico-vaginal fluid and advances our knowledge of the role of proteomics in endometrial cancer detection. Endometrial cancer is the most common gynaecological malignancy in high-income countries and its incidence is rising. Early detection, aided by highly sensitive and specific biomarkers, has the potential to improve outcomes as treatment can be provided when it is most likely to effect a cure. Sequential window acquisition of all theoretical mass spectra (SWATH-MS), an accurate and reproducible platform for analysing biological samples, offers a technological advance for biomarker discovery due to its reproducibility, sensitivity and potential for data re-interrogation. SWATH-MS requires a spectral library in order to identify and quantify peptides from multiplexed mass spectrometry data. Here we present a bespoke spectral library of 154,206 transitions identifying 19,394 peptides and 2425 proteins in the cervico-vaginal fluid of postmenopausal women with, or at risk of, endometrial cancer. We have combined these data with a library of over 6000 proteins generated based on mass spectrometric analysis of two endometrial cancer cell lines. This unique resource enables the study of protein biomarkers for endometrial cancer detection in cervico-vaginal fluid. Data are available via ProteomeXchange with unique identifier PXD025925.
Severe obesity is a disease associated with multiple adverse effects on health. Metabolic bariatric surgery (MBS) can have significant effects on multiple body systems and was shown to improve inflammatory markers in previous short-term follow-up studies. We evaluated associations between changes in inflammatory markers (CRP, IL6 and TNF alpha) and circulating proteins after MBS. Methods: Sequential window acquisition of all theoretical mass spectra (SWATH-MS) proteomics was performed on plasma samples taken at baseline (pre-surgery) and 6 and 12 months after MBS, and concurrent analyses of inflammatory/metabolic parameters were carried out. The change in absolute abundances of those proteins, showing significant change at both 6 and 12 months, was tested for correlation with the absolute and percentage (%) change in inflammatory markers. Results: We found the following results: at 6 months, there was a correlation between %change in IL-6 and fold change in HSPA4 (rho = -0.659; p = 0.038) and in SERPINF1 (rho = 0.714, p = 0.020); at 12 months, there was a positive correlation between %change in IL-6 and fold change in the following proteins-LGALS3BP (rho = 0.700, p = 0.036), HSP90B1 (rho = 0.667; p = 0.05) and ACE (rho = 0.667, p = 0.05). We found significant inverse correlations at 12 months between %change in TNF alpha and the following proteins: EPHX2 and ACE (for both rho = -0.783, p = 0.013). We also found significant inverse correlations between %change in CRP at 12 months and SHBG (rho = -0.759, p = 0.029), L1CAM (rho = -0.904, p = 0.002) and AMBP (rho = -0.684, p = 0.042). Conclusion: Using SWATH-MS, we identified several proteins that are involved in the inflammatory response whose levels change in patients who achieve remission of T2DM after bariatric surgery in tandem with changes in IL6, TNF alpha and/or CRP. Future studies are needed to clarify the underlying mechanisms in how MBS decreases low-grade inflammation.
HOXA9 is commonly upregulated in acute myeloid leukemia (AML), in which it confers a poor prognosis. Characterizing the protein interactome of endogenous HOXA9 in human AML, we identified a chromatin complex of HOXA9 with the nuclear matrix attachment protein SAFB. SAFB perturbation phenocopied HOXA9 knockout to decrease AML proliferation, increase differentiation and apoptosis in vitro, and prolong survival in vivo. Integrated genomic, transcriptomic, and proteomic analyses further demonstrated that the HOXA9-SAFB (H9SB)-chromatin complex associates with nucleosome remodeling and histone deacetylase (NuRD) and HP1γ to repress the expression of factors associated with differentiation and apoptosis, including NOTCH1, CEBPδ, S100A8, and CDKN1A. Chemical or genetic perturbation of NuRD and HP1γ-associated catalytic activity also triggered differentiation, apoptosis, and the induction of these tumor-suppressive genes. Importantly, this mechanism is operative in other HOXA9-dependent AML genotypes. This mechanistic insight demonstrates the active HOXA9-dependent differentiation block as a potent mechanism of disease maintenance in AML that may be amenable to therapeutic intervention by targeting the H9SB interface and/or NuRD and HP1γ activity.
•Ante-mortem damage to the ribs indicative of a blow by a sharp, possibly curved, object from behind, suggesting that Takabuti was murdered.•mtDNA revealed the rare Eurasian H4a1 haplogroup suggestive of the introduction of new gene pools during the Late Period of ancient Egyptian history.•Proteomics indicate protracted leg muscle activity in the hours before death.•Chemical analysis and microscopy of packing material inserted during mummification discovered wood shavings, including imported cedar, and congealed resin.The resin was identical to the mummification balm from the original bandages.•Packing material’s radiocarbon date indicated mummification in the Third Intermediate Period and in keeping with the previously dated hair and the stylistic dating of the coffin that placed it in the 25th Dynasty. Scientific examination of mummies has provided a better understanding of the ancestry, diet, disease, medical practices, mummification processes, culture and society of human populations worldwide and from different epochs. However, many examinations have been invasive and/or destructive, leading to current-day concerns among archaeologists and mummy custodians about this methodology. Multidisciplinary approaches, notably minimally invasive biopsy, endeavour to maximise the amount of data obtained from a sample whilst minimising its size and the effects on the mummy. As an illustration of the wealth of data that can be obtained from non- and minimally invasive image-guided procedures on wrapped mummified bodies, we report here on such a study of Takabuti, an Egyptian woman who lived in Thebes during the first millennium BCE. Multiplanar and 3D reconstructive computerised whole-body tomographic imaging (CT) revealed age at death and confirmed the inscriptional evidence from the coffin regarding sex. A notable finding was ante-mortem damage to the ribs indicative of a blow by a sharp, possibly curved, object from behind, suggesting that Takabuti was murdered. Fifty milligrams of bone and thigh muscle were taken for respectively genomic and proteomic analysis. mtDNA revealed the rare Eurasian H4a1 haplogroup suggestive of the introduction of new gene pools during the Late Period. Identification and quantification of the structural and functional proteins and metabolically important enzymes indicate protracted leg muscle activity in the hours before death. Chemical analysis, microscopy and radiocarbon dating of 20–30 mg needle biopsy samples of the packing material inserted during mummification discovered wood shavings, including imported cedar, and congealed resin. Organic analyses revealed the resin to be largely vegetal in origin including wood and aromatic/preservative oils derived from Pinaceae species, identical to the mummification balm removed from the original bandages. Once the age of the trees from which the sawdust was derived has been taken into account, the packing material’s radiocarbon date indicated mummification in the Third Intermediate Period and in keeping with the previously dated hair and the stylistic dating of the coffin that placed it in the 25th Dynasty. We have shown that CT scanning combined with targeted invasive biopsy needle sampling provides a wealth of scientific information with minimal disturbance to the mummy. Molecular, biochemical and geochemical analytical techniques allow statistically robust replicate sampling but require the removal of only milligram quantities. This approach forms the basis of a generic ‘palaeobiographical’ approach to the examination of naturally and artificially preserved bodies in which both soft tissues and bone are present.
Background Halting progression of chronic kidney disease (CKD) to established end stage kidney disease is a major goal of global health research. The mechanism of CKD progression involves pro-inflammatory, pro-fibrotic, and vascular pathways, but pathophysiological differentiation is currently lacking. Methods Plasma samples of 414 non-dialysis CKD patients, 170 fast progressors (with ∂ eGFR-3 ml/min/1.73 m2/year or worse) and 244 stable patients (∂ eGFR of − 0.5 to + 1 ml/min/1.73 m2/year) with a broad range of kidney disease aetiologies, were obtained and interrogated for proteomic signals with SWATH-MS. We applied a machine learning approach to feature selection of proteins quantifiable in at least 20% of the samples, using the Boruta algorithm. Biological pathways enriched by these proteins were identified using ClueGo pathway analyses. Results The resulting digitised proteomic maps inclusive of 626 proteins were investigated in tandem with available clinical data to identify biomarkers of progression. The machine learning model using Boruta Feature Selection identified 25 biomarkers as being important to progression type classification (Area Under the Curve = 0.81, Accuracy = 0.72). Our functional enrichment analysis revealed associations with the complement cascade pathway, which is relevant to CKD as the kidney is particularly vulnerable to complement overactivation. This provides further evidence to target complement inhibition as a potential approach to modulating the progression of diabetic nephropathy. Proteins involved in the ubiquitin–proteasome pathway, a crucial protein degradation system, were also found to be significantly enriched. Conclusions The in-depth proteomic characterisation of this large-scale CKD cohort is a step toward generating mechanism-based hypotheses that might lend themselves to future drug targeting. Candidate biomarkers will be validated in samples from selected patients in other large non-dialysis CKD cohorts using a targeted mass spectrometric analysis.
We investigated biological determinants that would associate with the response to a diet and weight loss programme in impaired glucose regulation (IGR) people using sequential window acquisition of all theoretical fragment ion spectra (SWATH) mass spectrometry (MS), a data acquisition method which complement traditional mass spectrometry-based proteomics techniques. Ten women and 10 men with IGR underwent anthropometric measurements and fasting blood tests. SWATH MS was carried out with subsequent immunoassay of specific peptide levels. After a six-month intervention, 40% of participants lost 3% or more in weight, 45% of patients remained within 3% of their starting weight and 15% increased their weight by 3% or more. Hemoglobin A1c (HbA1C) level was reduced with weight loss with improvements in insulin sensitivity. SWATH MS on pre-intervention samples and subsequent principal component analysis identified a cluster of proteins associated with future weight loss, including insulin-like growth factor-II (IGF-II) and Vitamin D binding protein. Individuals who lost 3% in weight had significantly higher baseline IGF-II levels than those who did not lose weight. SWATH MS successfully discriminated between individuals who were more likely to lose weight and potentially improve their sensitivity to insulin. A higher IGF-II baseline was predictive of success with weight reduction, suggesting that biological determinants are important in response to weight loss and exercise regimes. This may permit better targeting of interventions to prevent diabetes in the future.
The anatomical continuity between the uterine cavity and the lower genital tract allows for the exploitation of uterine-derived biomaterial in cervico-vaginal fluid for endometrial cancer detection based on non-invasive sampling methodologies. Plasma is an attractive biofluid for cancer detection due to its simplicity and ease of collection. In this biomarker discovery study, we aimed to identify proteomic signatures that accurately discriminate endometrial cancer from controls in cervico-vaginal fluid and blood plasma. Blood plasma and Delphi Screener-collected cervico-vaginal fluid samples were acquired from symptomatic post-menopausal women with (n = 53) and without (n = 65) endometrial cancer. Digitised proteomic maps were derived for each sample using sequential window acquisition of all theoretical mass spectra (SWATH-MS). Machine learning was employed to identify the most discriminatory proteins. The best diagnostic model was determined based on accuracy and model parsimony. A protein signature derived from cervico-vaginal fluid more accurately discriminated cancer from control samples than one derived from plasma. A 5-biomarker panel of cervico-vaginal fluid derived proteins (HPT, LG3BP, FGA, LY6D and IGHM) predicted endometrial cancer with an AUC of 0.95 (0.91–0.98), sensitivity of 91% (83%–98%), and specificity of 86% (78%–95%). By contrast, a 3-marker panel of plasma proteins (APOD, PSMA7 and HPT) predicted endometrial cancer with an AUC of 0.87 (0.81–0.93), sensitivity of 75% (64%–86%), and specificity of 84% (75%–93%). The parsimonious model AUC values for detection of stage I endometrial cancer in cervico-vaginal fluid and blood plasma were 0.92 (0.87–0.97) and 0.88 (0.82–0.95) respectively. Here, we leveraged the natural shed of endometrial tumours to potentially develop an innovative approach to endometrial cancer detection. We show proof of principle that endometrial cancers secrete unique protein signatures that can enable cancer detection via cervico-vaginal fluid assays. Confirmation in a larger independent cohort is warranted. Cancer Research UK, Blood Cancer UK, National Institute for Health Research.
Inappropriate localization of proteins can interfere with normal cellular function and drive tumor development. To understand how this contributes to the development of acute myeloid leukemia (AML), we compared the nuclear proteome and transcriptome of AML blasts with normal human CD34 cells. Analysis of the proteome identified networks and processes that significantly affected transcription regulation including misexpression of 11 transcription factors with seven proteins not previously implicated in AML. Transcriptome analysis identified changes in 40 transcription factors but none of these were predictive of changes at the protein level. The highest differentially expressed protein in AML nuclei compared with normal CD34 nuclei (not previously implicated in AML) was S100A4. In an extended cohort, we found that over-expression of nuclear S100A4 was highly prevalent in AML (83%; 20/24 AML patients). Knock down of S100A4 in AML cell lines strongly impacted their survival whilst normal hemopoietic stem progenitor cells were unaffected. These data are the first analysis of the nuclear proteome in AML and have identified changes in transcription factor expression or regulation of transcription that would not have been seen at the mRNA level. These data also suggest that S100A4 is essential for AML survival and could be a therapeutic target in AML.
We report the development and validation of an untargeted single-cell lipidomics method based on microflow chromatography coupled to a data-dependent mass spectrometry method for fragmentation-based identification of lipids. Given the absence of single-cell lipid standards, we show how the methodology should be optimized and validated using a dilute cell extract. The methodology is applied to dilute pancreatic cancer and macrophage cell extracts and standards to demonstrate the sensitivity requirements for confident assignment of lipids and classification of the cell type at the single-cell level. The method is then coupled to a system that can provide automated sampling of live, single cells into capillaries under microscope observation. This workflow retains the spatial information and morphology of cells during sampling and highlights the heterogeneity in lipid profiles observed at the single-cell level. The workflow is applied to show changes in single-cell lipid profiles as a response to oxidative stress, coinciding with expanded lipid droplets. This demonstrates that the workflow is sufficiently sensitive to observing changes in lipid profiles in response to a biological stimulus. Understanding how lipids vary in single cells will inform future research into a multitude of biological processes as lipids play important roles in structural, biophysical, energy storage, and signaling functions.
Myelofibrosis is a myeloproliferative neoplasm (MPN) which typically results in reduced length and quality of life due to systemic symptoms and blood count changes arising from fibrotic changes in the bone marrow. While the JAK2 inhibitor ruxolitinib provides some clinical benefit, there remains a substantial unmet need for novel targeted therapies to better modify the disease process or eradicate the cells at the heart of myelofibrosis pathology. Repurposing drugs bypasses many of the hurdles present in drug development, such as toxicity and pharmacodynamic profiling. To this end we undertook a re-analysis of our pre-existing proteomic data sets to identify perturbed biochemical pathways and their associated drugs/inhibitors to potentially target the cells driving myelofibrosis. This approach identified CBL0137 as a candidate for targeting Jak2 mutation-driven malignancies. CBL0137 is a drug derived from curaxin targeting the Facilitates Chromatin Transcription (FACT) complex. It is reported to trap the FACT complex on chromatin thereby activating p53 and inhibiting NF-kB activity. We therefore assessed the activity of CBL0137 in primary patient samples and murine models of Jak2-mutated MPN and found it preferentially targets CD34+ stem and progenitor cells from myelofibrosis patients by comparison with healthy control cells. Further we investigate its mechanism of action in primary haemopoietic progenitor cells and demonstrate its ability to reduce splenomegaly and reticulocyte number in a transgenic murine model of myeloproliferative neoplasms.
Rheumatic heart disease (RHD) remains a major source of morbidity and mortality in developing countries. A deeper insight into the pathogenetic mechanisms underlying RHD could provide opportunities for drug repurposing, guide recommendations for secondary penicillin prophylaxis, and/or inform development of near-patient diagnostics.We performed quantitative proteomics using Sequential Windowed Acquisition of All Theoretical Fragment Ion Mass Spectrometry (SWATH-MS) to screen protein expression in 215 African patients with severe RHD, and 230 controls. We applied a machine learning (ML) approach to feature selection among the 366 proteins quantifiable in at least 40% of samples, using the Boruta wrapper algorithm. The case-control differences and contribution to area under the Receiver Operating Curve for each of the 56 proteins identified by the Boruta algorithm were calculated by Logistic Regression adjusted for age, sex and BMI. Biological pathways and functions enriched for proteins were identified using ClueGo pathway analyses.Adiponectin, complement component C7 and fibulin-1, a component of heart valve matrix, were significantly higher in cases when compared with controls (Table 1). Ficolin-3, a protein with calcium-independent lectin activity that activates the complement pathway, was lower in cases than controls (Table 1). The top six biomarkers, including adiponectin, complement component C7, quiescin sulfhydryl oxidase 1, insulin like growth factor binding protein acid labile subunit, pregnancy zone protein and phosphatidylinositol-glycan-specific phospholipase D, from the Boruta analyses (Fig. 1a) conferred an AUC of 0.90 indicating excellent discriminatory capacity between RHD cases and controls (Fig. 1b).ClueGo pathway analysis results of these biomarkers support the presence of an ongoing inflammatory response in RHD (Fig. 2), at a time when severe valve disease has developed, and distant from previous episodes of acute rheumatic fever. This biomarker signature could have potential utility in recognizing different degrees of ongoing inflammation in RHD patients, which may, in turn, be related to prognostic severity.Conflict of InterestNone
The UK Biobank is a cohort study that collects data on diet, lifestyle, biomarkers, and health to examine diet-disease associations. Based on the UK Biobank, we reviewed 36 studies on diet and three health conditions: type 2 diabetes (T2DM), cardiovascular disease (CVD), and cancer. Most studies used one-time dietary data instead of repeated 24 h recalls, which may lead to measurement errors and bias in estimating diet-disease associations. We also found that most studies focused on single food groups or macronutrients, while few studies adopted a dietary pattern approach. Several studies consistently showed that eating more red and processed meat led to a higher risk of lung and colorectal cancer. The results suggest that high adherence to "healthy" dietary patterns (consuming various food types, with at least three servings/day of whole grain, fruits, and vegetables, and meat and processed meat less than twice a week) slightly lowers the risk of T2DM, CVD, and colorectal cancer. Future research should use multi-omics data and machine learning models to account for the complexity and interactions of dietary components and their effects on disease risk.
Prostate cancer is the most common malignant tumour in men. Improved testing for di- agnosis, risk prediction, and response to treatment would improve care. Here, we identified a pro- teomic signature of prostate cancer in peripheral blood using data-independent acquisition mass spectrometry combined with machine learning. A highly predictive signature was derived, which was associated with relevant pathways, including the coagulation, complement, and clotting cas- cades, as well as plasma lipoprotein particle remodeling. We further validated the identified bi- omarkers against a second cohort, identifying a panel of five key markers (GP5, SERPINA5, ECM1, IGHG1, and THBS1) which retained most of the diagnostic power of the overall dataset, achieving an AUC of 0.91. Taken together, this study provides a proteomic signature complementary to PSA for the diagnosis of patients with localised prostate cancer, with the further potential for assessing risk of future development of prostate cancer. Data are available via ProteomeXchange with identi- fier PXD025484.
Lipids play a key role in many biological processes, and their accurate measurement is critical to unraveling the biology of diseases and human health. A high throughput HILIC-based (LC-MS) method for the semiquantitative screening of over 2000 lipids, based on over 4000 MRM transitions, was devised to produce an accessible and robust lipidomic screen for phospholipids in human plasma/serum. This methodology integrates many of the advantages of global lipid analysis with those of targeted approaches. Having used the method as an initial "wide class" screen, it can then be easily adapted for a more targeted analysis and quantification of key, dysregulated lipids. Robustness was assessed using 1550 continuous injections of plasma extracts onto a single column and via the evaluation of columns from 5 different batches of stationary phase. Initial screens in positive (239 lipids, 431 MRM transitions) and negative electrospray ionization (ESI) mode (232 lipids, 446 MRM transitions) were assessed for reproducibility, sensitivity, and dynamic range using analysis times of 8 min. The total number of lipids monitored using these screening methods was 433 with an overlap of 38 lipids in both modes. A polarity switching method for accurate quantification, using the same LC conditions, was assessed for intra- and interday reproducibility, accuracy, dynamic range, stability, carryover, dilution integrity, and matrix interferences and found to be acceptable. This polarity switching method was then applied to lipids important in the stratification of human prostate cancer samples.
Pharmacologic inhibition of LSD1 induces molecular and morphologic differentiation of blast cells in acute myeloid leukemia (AML) patients harboring MLL gene translocations. In addition to its demethylase activity, LSD1 has a critical scaffolding function at genomic sites occupied by the SNAG domain transcription repressor GFI1. Importantly, inhibitors block both enzymatic and scaffolding activities, in the latter case by disrupting the protein:protein interaction of GFI1 with LSD1. To explore the wider consequences of LSD1 inhibition on the LSD1 protein complex we applied mass spectrometry technologies. We discovered that the interaction of the HMG-box protein HMG20B with LSD1 was also disrupted by LSD1 inhibition. Downstream investigations revealed that HMG20B is co-located on chromatin with GFI1 and LSD1 genome-wide; the strongest HMG20B binding co-locates with the strongest GFI1 and LSD1 binding. Functional assays demonstrated that HMG20B depletion induces leukemia cell differentiation and further revealed that HMG20B is required for the transcription repressor activity of GFI1 through stabilizing LSD1 on chromatin at GFI1 binding sites. Interaction of HMG20B with LSD1 is through its coiled-coil domain. Thus, HMG20B is a critical component of the GFI1:LSD1 transcription repressor complex which contributes to leukemia cell differentiation block.
Simple Summary: Prostate cancer is the third most frequent cancer in men worldwide, with a notable increase in prevalence over the past two decades. The PSA is the only well-established protein biomarker for prostate cancer diagnosis, staging, and surveillance. It frequently leads to inaccurate diagnosis and overtreatment since it is an organ-specific biomarker rather than a tumour-specific biomarker. As a result, one of the primary goals of prostate cancer proteome research is to identify novel biomarkers that can be used with or instead of PSA, particularly in non-invasive blood samples. Thousands of peptides or assays were detected in blood samples from patients with low- to high-grade prostate cancer and healthy individuals, allowing data processing of sequential window acquisition of all theoretical mass spectra (SWATH-MS). By assisting in the detection of prostate cancer biomarkers in blood samples, this useful resource will improve our understanding of the role of proteomics in prostate cancer diagnosis and risk assessment. Prostate cancer is the most frequent form of cancer in men, accounting for more than one-third of all cases. Current screening techniques, such as PSA testing used in conjunction with routine procedures, lead to unnecessary biopsies and the discovery of low-risk tumours, resulting in overdiagnosis. SWATH-MS is a well-established data-independent (DI) method requiring prior knowledge of targeted peptides to obtain valuable information from SWATH maps. In response to the growing need to identify and characterise protein biomarkers for prostate cancer, this study explored a spectrum source for targeted proteome analysis of blood samples. We created a comprehensive prostate cancer serum spectral library by combining data-dependent acquisition (DDA) MS raw files from 504 patients with low, intermediate, or high-grade prostate cancer and healthy controls, as well as 304 prostate cancer-related protein in silico assays. The spectral library contains 114,684 transitions, which equates to 18,479 peptides translated into 1227 proteins. The robustness and accuracy of the spectral library were assessed to boost confidence in the identification and quantification of prostate cancer-related proteins across an independent cohort, resulting in the identification of 404 proteins. This unique database can facilitate researchers to investigate prostate cancer protein biomarkers in blood samples. In the real-world use of the spectrum library for biomarker detection, using a signature of 17 proteins, a clear distinction between the validation cohort's pre- and post-treatment groups was observed. Data are available via ProteomeXchange with identifier PXD028651.
Simple Summary TARGET (tumour characterisation to guide experimental targeted therapy) matches patients entering early phase cancer clinical trials to the best treatment for them based on their genetics. Selecting only fit patients for these trials means less patients need to be recruited. Fit patients have a life expectancy of >three months. Performance status (PS) is used to measure life expectancy and is decided by doctors asking questions about patient's activity levels. We created a Wellness Score using proteins in 55 patient's blood samples. This score groups patients into those who were likely to die and those who were likely to be alive within six months of their blood sample. This score was more accurately able to predict a patient's survival at six months than PS. We then reached the same conclusion in a further 77 patients. We hope this score can now be tested in an even larger group of patients. TARGET (tumour characterisation to guide experimental targeted therapy) is a cancer precision medicine programme focused on molecular characterisation of patients entering early phase clinical trials. Performance status (PS) measures a patient's ability to perform a variety of activities. However, the quality of present algorithms to assess PS is limited and based on qualitative clinician assessment. Plasma samples from patients enrolled into TARGET were analysed using the mass spectrometry (MS) technique: sequential window acquisition of all theoretical fragment ion spectra (SWATH)-MS. SWATH-MS was used on a discovery cohort of 55 patients to differentiate patients into either a good or poor prognosis by creation of a Wellness Score (WS) that showed stronger prediction of overall survival (p = 0.000551) compared to PS (p = 0.001). WS was then tested against a validation cohort of 77 patients showing significant (p = 0.000451) prediction of overall survival. WS in both sets had receiver operating characteristic curve area under the curve (AUC) values of 0.76 (p = 0.002) and 0.67 (p = 0.011): AUC of PS was 0.70 (p = 0.117) and 0.55 (p = 0.548). These signatures can now be evaluated further in larger patient populations to assess their utility in a clinical setting.
Simple Summary Endometrial cancer is the commonest cancer of the female genital tract and obesity is its main modifiable risk factor. Over 80% of endometrial cancers develop in the context of obesity-induced metabolic changes. This study focuses on the potential of plasma-based metabolites to enable the early detection of endometrial cancer in a cohort of women with body mass index (BMI) >= 30 kg/m(2). Specific lipid metabolites including phospholipids and sphingolipids (sphingomyelins) demonstrated good accuracy for the detection of endometrial cancer, especially when combined in a diagnostic model. This study advances our knowledge of the role of metabolomics in endometrial cancer and provides a basis for the minimally invasive screening of women with elevated BMI. Endometrial cancer is the most common malignancy of the female genital tract and a major cause of morbidity and mortality in women. Early detection is key to ensuring good outcomes but a lack of minimally invasive screening tools is a significant barrier. Most endometrial cancers are obesity-driven and develop in the context of severe metabolomic dysfunction. Blood-derived metabolites may therefore provide clinically relevant biomarkers for endometrial cancer detection. In this study, we analysed plasma samples of women with body mass index (BMI) >= 30 kg/m(2) and endometrioid endometrial cancer (cases, n = 67) or histologically normal endometrium (controls, n = 69), using a mass spectrometry-based metabolomics approach. Eighty percent of the samples were randomly selected to serve as a training set and the remaining 20% were used to qualify test performance. Robust predictive models (AUC > 0.9) for endometrial cancer detection based on artificial intelligence algorithms were developed and validated. Phospholipids were of significance as biomarkers of endometrial cancer, with sphingolipids (sphingomyelins) discriminatory in post-menopausal women. An algorithm combining the top ten performing metabolites showed 92.6% prediction accuracy (AUC of 0.95) for endometrial cancer detection. These results suggest that a simple blood test could enable the early detection of endometrial cancer and provide the basis for a minimally invasive screening tool for women with a BMI >= 30 kg/m(2).
Stem and progenitor cells undergo a global elevation of nascent transcription, or hypertranscription, during key developmental transitions involving rapid cell proliferation. The chromatin remodeler Chd1 mediates hypertranscription in pluripotent cells but its mechanism of action remains poorly understood. Here we report a novel role for Chd1 in protecting genome integrity at promoter regions by preventing DNA double-stranded break (DSB) accumulation in ES cells. Chd1 interacts with several DNA repair factors including Atm, Parp1, Kap1 and Topoisomerase 2 beta and its absence leads to an accumulation of DSBs at Chd1-bound Pol II-transcribed genes and rDNA. Genes prone to DNA breaks in Chd1 KO ES cells are longer genes with GC-rich promoters, a more labile nucleosomal structure and roles in chromatin regulation, transcription and signaling. These results reveal a vulnerability of hypertranscribing stem cells to accumulation of endogenous DNA breaks, with important implications for developmental and cancer biology. The chromatin remodeler Chd1 mediates hypertranscription in embryonic stem (ES) cells and has been shown to associate with genes transcribed by RNA Polymerase (Pol) I and II. Here the authors provide mechanistic insights into this process and reveal that Chd1 is involved in protecting genome integrity at promoter regions by preventing DNA break accumulation.
THOC5, a member of the THO complex, is essential for the 3′processing of some inducible genes, the export of a subset of mRNAs and stem cell survival. Here we show that THOC5 depletion results in altered 3′cleavage of >50% of mRNAs and changes in RNA polymerase II binding across genes. THOC5 is recruited close to high-density polymerase II sites, suggesting that THOC5 is involved in transcriptional elongation. Indeed, measurement of elongation rates in vivo demonstrated decreased rates in THOC5-depleted cells. Furthermore, THOC5 is preferentially recruited to its target genes in slow polymerase II cells compared with fast polymerase II cells. Importantly chromatin-associated THOC5 interacts with CDK12 (a modulator of transcription elongation) and RNA helicases DDX5, DDX17, and THOC6 only in slow polymerase II cells. The CDK12/THOC5 interaction promotes CDK12 recruitment to R-loops in a THOC6-dependent manner. These data demonstrate a novel function of THOC5 in transcription elongation. [Display omitted] •THOC5 depletion decreased transcription elongation rates globally•3′ end cleavage of more than 50% of genes was altered in THOC5-depleted cells•The rate of transcription elongation determines polyA site usage•THOC5/THOC6 recruit CDK12 and DDX5/17 to regulate R loop structures and elongation rate Molecular biology; Cell biology
Introduction Patients receiving radiotherapy are at risk of developing radiotherapy-related insufficiency fractures, which are associated with increased morbidity and pose a significant burden to patients' quality of life and to the health system. Therefore, effective preventive techniques are urgently required. The RadBone randomised controlled trial (RCT) aims to determine the feasibility and acceptability of a musculoskeletal health package (MHP) intervention in women undergoing pelvic radiotherapy for gynaecological malignancies and to preliminary explore clinical effectiveness of the intervention. Methods and analysis The RadBone RCT will evaluate the addition to standard care of an MHP consisting of a physical assessment of the musculoskeletal health, a 3-month prehabilitation personalised exercise package, as well as an evaluation of the fracture risk and if required the prescription of appropriate bone treatment including calcium, vitamin D and-for high-risk individuals-bisphosphonates. Forty participants will be randomised in each group (MHP or observation) and will be followed for 18 months. The primary outcome of this RCT will be feasibility, including the eligibility, screening and recruitment rate, intervention fidelity and attrition rates; acceptability and health economics. Clinical effectiveness and bone turnover markers will be evaluated as secondary outcomes. Ethics and dissemination This study has been approved by the Greater Manchester East Research Ethics Committee (Reference: 20/NW/0410, November 2020). The results will be published in peer-reviewed journals, will be presented in national and international conferences and will be communicated to relevant stakeholders. Moreover, a plain English report will be shared with the study participants, patients' organisations and media.
ObjectiveSystemic lupus erythematosus (SLE) is a clinically and biologically heterogenous autoimmune disease. We aimed to investigate the plasma proteome of patients with active SLE to identify novel subgroups, or endotypes, of patients.MethodPlasma was collected from patients with active SLE who were enrolled in the British Isles Lupus Assessment Group Biologics Registry (BILAG-BR). The plasma proteome was analysed using a data-independent acquisition method, Sequential Window Acquisition of All theoretical mass spectra mass spectrometry (SWATH-MS). Unsupervised, data-driven clustering algorithms were used to delineate groups of patients with a shared proteomic profile.ResultsIn 223 patients, six clusters were identified based on quantification of 581 proteins. Between the clusters, there were significant differences in age (p = 0.012) and ethnicity (p = 0.003). There was increased musculoskeletal disease activity in cluster 1 (C1), 19/27 (70.4%) (p = 0.002) and renal activity in cluster 6 (C6) 15/24 (62.5%) (p = 0.051). Anti-SSa/Ro was the only autoantibody that significantly differed between clusters (p = 0.017). C1 was associated with p21-activated kinases (PAK) and Phospholipase C (PLC) signalling. Within C1 there were two sub-clusters (C1A and C1B) defined by 49 proteins related to cytoskeletal protein binding. C2 and C6 demonstrated opposite Rho family GTPase and Rho GDI signalling. Three proteins (MZB1, SND1 and AGL) identified in C6 increased the classification of active renal disease although this did not reach statistical significance (p = 0.0617).ConclusionsUnsupervised proteomic analysis identifies clusters of patients with active SLE, that are associated with clinical and serological features, which may facilitate biomarker discovery. The observed proteomic heterogeneity further supports the need for a personalised approach to treatment in SLE.
Background The early identification of patients at high‑risk for end‑stage renal disease (ESRD) is essential for pro‑ viding optimal care and implementing targeted prevention strategies. While the Kidney Failure Risk Equation (KFRE) offers a more accurate prediction of ESRD risk compared to static eGFR‑based thresholds, it does not provide insights into the patient‑specific biological mechanisms that drive ESRD. This study focused on evaluating the effectiveness of KFRE in a UK‑based advanced chronic kidney disease (CKD) cohort and investigating whether the integration of a proteomic signature could enhance 5‑year ESRD prediction. Methods Using the Salford Kidney Study biobank, a UK‑based prospective cohort of over 3000 non‑dialysis CKD patients, 433 patients met our inclusion criteria: a minimum of four eGFR measurements over a two‑year period and a linear eGFR trajectory. Plasma samples were obtained and analysed for novel proteomic signals using SWATH‑ Mass‑Spectrometry. The 4‑variable UK‑calibrated KFRE was calculated for each patient based on their baseline clinical characteristics. Boruta machine learning algorithm was used for the selection of proteins most contributing to differ‑ entiation between patient groups. Logistic regression was employed for estimation of ESRD prediction by (1) prot‑ eomic features; (2) KFRE; and (3) proteomic features alongside KFRE. Results SWATH maps with 943 quantified proteins were generated and investigated in tandem with available clinical data to identify potential progression biomarkers. We identified a set of proteins (SPTA1, MYL6 and C6) that, when used alongside the 4‑variable UK‑KFRE, improved the prediction of 5‑year risk of ESRD (AUC = 0.75 vs AUC = 0.70). Functional enrichment analysis revealed Rho GTPases and regulation of the actin cytoskeleton pathways to be statistically significant, inferring their role in kidney function and the pathogenesis of renal disease. Conclusions Proteins SPTA1, MYL6 and C6, when used alongside the 4‑variable UK‑KFRE achieve an improved performance when predicting a 5‑year risk of ESRD. Specific pathways implicated in the pathogenesis of podocyte dysfunction were also identified, which could serve as potential therapeutic targets. The findings of our study carry
There is an unmet need for improved diagnostic testing and risk prediction for cases of prostate cancer (PCa) to improve care and reduce overtreatment of indolent disease. Here we have analysed the serum proteome and lipidome of 262 study participants by liquid chromatography-mass spectrometry, including participants diagnosed with PCa, benign prostatic hyperplasia (BPH), or otherwise healthy volunteers, with the aim of improving biomarker specificity. Although a two class machine learning model separated PCa from controls with sensitivity of 0.82 and specificity of 0.95, adding BPH resulted in a statistically significant decline in specificity for prostate cancer to 0.76, with half of BPH cases being misclassified by the model as PCa. A small number of biomarkers differentiating between BPH and prostate cancer were identified, including proteins in MAP Kinase pathways, as well as in lipids containing oleic acid; these may offer a route to greater specificity. These results highlight, however, that whilst there are opportunities for machine learning, these will only be achieved by use of appropriate training sets that include confounding comorbidities, especially when calculating the specificity of a test.
Despite the big increase in precision medicine targeted therapies developing curative treatments for many cancers is still a major challenge due mainly to the development of drug resistance in cancer stem cells. The cancer stem cells are constantly evolving to survive and targeted drug treatment often increases the selective pressure on these cells from which the disease develops. Chronic myeloid leukaemia is a paradigm of cancer stem cell research. Targeted therapies to the causative oncogene, BCR/ABL, have been developed but drug resistance remains a problem. The introduction of tyrosine kinase inhibitors targeting BCR/ABL were transformative in the management of CML. However, patients are rarely cured as the tyrosine kinase inhibitors fail to eradicate the leukaemic stem cell which often leads to loss of response to therapy as drug resistance develops and progression to more fatal forms of acute leukaemia occurs. New treatment strategies targeting other entities within the leukemic stem cell either alone or in combination with tyrosine kinase are therefore required. Drawing on our previous published work on the development of potential novel targets in CML and other myeloproliferative diseases along with analysis of the facilitates chromatin transcription (FACT) complex in CML we hypothesised that curaxin, a drug that targets the FACT complex and is in clinical trial for the treatment of other cancers, could be of use in the treatment of CML. We therefore assessed the curaxin CBL0137 as a new agent to extinguish CML primitive cells and show its ability to preferentially target CML cells compared to healthy control cells, especially in combination with clinically relevant tyrosine kinase inhibitors.
The transcriptional regulator EVI1 has an essential role in early development and haematopoiesis. However, acute myeloid leukaemia (AML) driven by aberrantly high EVI1 expression has very poor prognosis. To investigate the effects of post-translational modifications on EVI1 function, we carried out a mass spectrometry (MS) analysis of EVI1 in AML and detected dynamic phosphorylation at serine 436 (S436). Wild-type EVI1 (EVI1-WT) with S436 available for phosphorylation, but not non-phosphorylatable EVI1-S436A, conferred haematopoietic progenitor cell self-renewal and was associated with significantly higher organised transcriptional patterns. In silico modelling of EVI1-S436 phosphorylation showed reduced affinity to CtBP1, and CtBP1 showed reduced interaction with EVI1-WT compared with EVI1-S436A. The motif harbouring S436 is a target of CDK2 and CDK3 kinases, which interacted with EVI1-WT. The methyltransferase DNMT3A bound preferentially to EVI1-WT compared with EVI1-S436A, and a hypomethylated cell population associated by EVI1-WT expression in murine haematopoietic progenitors is not maintained with EVI1-S436A. These data point to EVI1-S436 phosphorylation directing functional protein interactions for haematopoietic self-renewal. Targeting EVI1-S436 phosphorylation may be of therapeutic benefit when treating EVI1-driven leukaemia.
Murine models are amongst the most widely used systems to study biology and pathology. Targeted quantitative proteomic analysis is a relatively new tool to interrogate such systems. Recently the need for relative quantification on hundreds to thousands of samples has driven the development of Data Independent Acquisition methods. One such technique is SWATH-MS, which in the main requires prior acquisition of mass spectra to generate an assay reference library. In stem cell research, it has been shown pluripotency can be induced starting with a fibroblast population. In so doing major changes in expressed proteins is inevitable. Here we have created a reference library to underpin such studies. This is inclusive of an extensively documented script to enable replication of library generation from the raw data. The documented script facilitates reuse of data and adaptation of the library to novel applications. The resulting library provides deep coverage of the mouse proteome. The library covers 29519 proteins (53% of the proteome) of which 7435 (13%) are supported by a proteotypic peptide.
Metabolic reprogramming is increasingly recognised as one of the defining hallmarks of tumorigenesis. There is compelling evidence to suggest that endometrial cancer develops and progresses in the context of profound metabolic dysfunction. Whilst the incidence of endometrial cancer continues to rise in parallel with the global epidemic of obesity, there are, as yet, no validated biomarkers that can aid risk prediction, early detection, prognostic evaluation or surveillance. Advances in high-throughput technologies have, in recent times, shown promise for biomarker discovery based on genomic, transcriptomic, proteomic and metabolomic platforms. Metabolomics, the large-scale study of metabolites, deals with the downstream products of the other omics technologies and thus best reflects the human phenotype. This review aims to provide a summary and critical synthesis of the existing literature with the ultimate goal of identifying the most promising metabolite biomarkers that can augment current endometrial cancer diagnostic, prognostic and recurrence surveillance strategies. Identified metabolites and their biochemical pathways are discussed in the context of what we know about endometrial carcinogenesis and their potential clinical utility is evaluated. Finally, we underscore the challenges inherent in metabolomic biomarker discovery and validation and provide fresh perspectives and directions for future endometrial cancer biomarker research.
Gray platelet syndrome (GPS) is a rare recessive disorder caused by biallelic variants in NBEAL2 and characterized by bleeding symptoms, the absence of platelet alpha-granules, splenomegaly, and bone marrow (BM) fibrosis. Due to the rarity of GPS, it has been difficult to fully understand the pathogenic processes that lead to these clinical sequelae. To discern the spectrum of pathologic features, we performed a detailed clinical genotypic and phenotypic study of 47 patients with GPS and identified 32 new etiologic variants in NBEAL2. The GPS patient cohort exhibited known phenotypes, including macrothrombocytopenia, BM fibrosis, megakaryocyte emperipolesis of neutrophils, splenomegaly, and elevated serum vitamin B-12 levels. Novel clinical phenotypes were also observed, including reduced leukocyte counts and increased presence of autoimmune disease and positive autoantibodies. There were widespread differences in the transcriptome and proteome of GPS platelets, neutrophils, monocytes, and CD4 lymphocytes. Proteins less abundant in these cells were enriched for constituents of granules, supporting a role for Nbeal2 in the function of these organelles across a wide range of blood cells. Proteomic analysis of GPS plasma showed increased levels of proteins associated with inflammation and immune response. One-quarter of plasma proteins increased in GPS are known to be synthesized outside of hematopoietic cells, predominantly in the liver. In summary, our data show that, in addition to the well-described platelet defects in GPS, there are immune defects. The abnormal immune cells may be the drivers of systemic abnormalities such as autoimmune disease.
Cell fate decisions during development are governed by multi-factorial regulatory mechanisms including chromatin remodeling, DNA methylation, binding of transcription factors to specific loci, RNA transcription and protein synthesis. However, the mechanisms by which such regulatory “dimensions” coordinate cell fate decisions are currently poorly understood. Here we quantified the multi-dimensional molecular changes that occur in mouse embryonic stem cells (mESCs) upon depletion of Estrogen related receptor beta (Esrrb), a key pluripotency regulator. Comparative analyses of expression changes subsequent to depletion of Esrrb or Nanog, indicated that a system of interlocked feed-forward loops involving both factors, plays a central part in regulating the timing of mESC fate decisions. Taken together, our meta-analyses support a hierarchical model in which pluripotency is maintained by an Oct4-Sox2 regulatory module, while the timing of differentiation is regulated by a Nanog-Esrrb module.
Background Rheumatic heart disease (RHD) remains a major source of morbidity and mortality in developing countries. A deeper insight into the pathogenetic mechanisms underlying RHD could provide opportunities for drug repurposing, guide recommendations for secondary penicillin prophylaxis, and/or inform development of near-patient diagnostics. Methods We performed quantitative proteomics using Sequential Windowed Acquisition of All Theoretical Fragment Ion Mass Spectrometry (SWATH-MS) to screen protein expression in 215 African patients with severe RHD, and 230 controls. We applied a machine learning (ML) approach to feature selection among the 366 proteins quantifiable in at least 40% of samples, using the Boruta wrapper algorithm. The case–control differences and contribution to Area Under the Receiver Operating Curve (AUC) for each of the 56 proteins identified by the Boruta algorithm were calculated by Logistic Regression adjusted for age, sex and BMI. Biological pathways and functions enriched for proteins were identified using ClueGo pathway analyses. Results Adiponectin, complement component C7 and fibulin-1, a component of heart valve matrix, were significantly higher in cases when compared with controls. Ficolin-3, a protein with calcium-independent lectin activity that activates the complement pathway, was lower in cases than controls. The top six biomarkers from the Boruta analyses conferred an AUC of 0.90 indicating excellent discriminatory capacity between RHD cases and controls. Conclusions These results support the presence of an ongoing inflammatory response in RHD, at a time when severe valve disease has developed, and distant from previous episodes of acute rheumatic fever. This biomarker signature could have potential utility in recognizing different degrees of ongoing inflammation in RHD patients, which may, in turn, be related to prognostic severity.