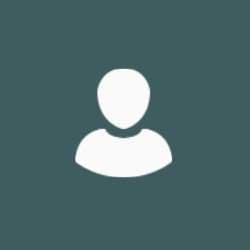
Chong Huang
Publications
The space-air-ground integrated network (SAGIN) has become a crucial research direction in future wireless communications due to its ubiquitous coverage, rapid and flexible deployment, and multi-layer cooperation capabilities. However, integrating hierarchical federated learning (HFL) with edge computing and SAGINs remains a complex open issue to be resolved. This paper proposes a novel framework for applying HFL in SAGINs, utilizing aerial platforms and low Earth orbit (LEO) satellites as edge servers and cloud servers, respectively, to provide multi-layer aggregation capabilities for HFL. The proposed system also considers the presence of inter-satellite links (ISLs), enabling satellites to exchange federated learning models with each other. Furthermore, we consider multiple different computational tasks that need to be completed within a limited satellite service time. To maximize the convergence performance of all tasks while ensuring fairness, we propose the use of the distributional soft-actor-critic (DSAC) algorithm to optimize resource allocation in the SAGIN and aggregation weights in HFL. Moreover, we address the efficiency issue of hybrid action spaces in deep reinforcement learning (DRL) through a decoupling and recoupling approach, and design a new dynamic adjusting reward function to ensure fairness among multiple tasks in federated learning. Simulation results demonstrate the superiority of our proposed algorithm, consistently outperforming baseline approaches and offering a promising solution for addressing highly complex optimization problems in SAGINs.
—In recent years, the amalgamation of satellite communications and aerial platforms into space-air-ground integrated network (SAGINs) has emerged as an indispensable area of research for future communications due to the global coverage capacity of low Earth orbit (LEO) satellites and the flexible Deployment of aerial platforms. This paper presents a deep reinforcement learning (DRL)-based approach for the joint optimization of offloading and resource allocation in hybrid cloud and multi-access edge computing (MEC) scenarios within SAGINs. The proposed system considers the presence of multiple satellites, clouds and unmanned aerial vehicles (UAVs). The multiple tasks from ground users are modeled as directed acyclic graphs (DAGs). With the goal of reducing energy consumption and latency in MEC, we propose a novel multi-agent algorithm based on DRL that optimizes both the offloading strategy and the allocation of resources in the MEC infrastructure within SAGIN. A hybrid action algorithm is utilized to address the challenge of hybrid continuous and discrete action space in the proposed problems, and a decision-assisted DRL method is adopted to reduce the impact of unavailable actions in the training process of DRL. Through extensive simulations, the results demonstrate the efficacy of the proposed learning-based scheme, the proposed approach consistently outperforms benchmark schemes, highlighting its superior performance and potential for practical applications. Index Terms—Space-air-ground integrated networks, edge computing , resource allocation, unmanned aerial vehicle, deep reinforcement learning.
This paper presents a general framework for probabilistic relay selection in asymmetric buffer-aided cooperative relaying systems, which caters to scenarios with both perfect and imperfect channel state information (CSI) during the relay selection (RS) process. The framework extends and generalizes many existing buffer-aided RS schemes. In particular, we introduce an auxiliary stochastic process which assigns varying selection probabilities to different links, considering the dynamic wireless channel and buffer states. Subsequently, we leverage the obtained outage probability and average packet delay (APD) to formulate outage optimization problems while adhering to APD. To address the intricate high-dimensional optimization problems, we employ a deep learning (DL) approach, which involves designing the probability mass function of the auxiliary stochastic process and developing an effective loss function to update the neural network. Simulation results unequivocally demonstrate the superior performance of the proposed DL-based probabilistic relay selection scheme compared to benchmark schemes, particularly in scenarios involving imperfect CSI.
This paper established a novel multi-input multi-output (MIMO) communication network, in the presence of full-duplex (FD) transmitters and receivers with the assistance of dual-side intelligent omni surface (IOS). Compared with the traditional IOS, the dual-side IOS allows signals from both sides to reflect and refract simultaneously, which further exploits the potential of metasurfaces to avoid frequency dependence, and size, weight, and power (SWaP) limitations. By considering both the downlink and uplink transmissions, we aim to maximize the weighted sum rate, subject to the transmit power constraints of the transmitter, the users and the dual-side reflecting and refracting phase shifts constraints. However, the formulated sum rate maximization problem is not convex, hence we exploit the weighted minimum mean square error (WMMSE) approach, and tackle the original problem iteratively by solving two sub-problems. For the beamforming matrices optimization of the downlink and uplink, we resort to the Lagrangian dual method combined with a bisection search to obtain the results. Furthermore , we resort to the quadratically constrained quadratic programming (QCQP) method to optimize the reflecting and refracting phase shifts of both sides of the IOS. Simulation results validate the efficacy of the proposed algorithm and demonstrate the superiority of the dual-side IOS.
This paper proposes a secure transmission in reconfigurable intelligent surfaces (RIS) aided non-terrestrial cooperative networks (NTCN), where the practical phase-dependent model is considered in which the RIS reflection amplitudes change with the corresponding discrete phase shifts. Moreover, we employ a full-duplex transmission scheme at the relay nodes to reduce the long-range signal loss and improve the security between the satellite and the relay node. To solve the complex non-convex optimization problem of the joint RIS reflection coefficient and relay selection optimization, we propose the deep cascade correlation learning (DCCL) algorithm to enhance optimization efficiency. Simulation results show that the proposed DCCL-based method significantly improves the secrecy capacity compared to the random relay selection and RIS coefficient methods.
Reconfigurable intelligent surfaces (RISs) have emerged as a promising technology in wireless communications. Simultaneously transmitting and reflecting RIS (STAR-RISs) in particular have garnered significant attention due to their dual capabilities of simultaneous transmission and reflection, underscoring their potential applications in critical scenarios within the forthcoming sixth-generation (6G) technology landscape. Moreover, full-duplex (FD) systems have emerged as a breakthrough research direction in wireless transmission technology due to their high spectral efficiency. This paper explores the application potential of STAR-RIS in FD systems for future wireless communications, presenting an innovative technology that provides robust self-interference cancellation (SIC) capabilities for FD systems. We utilize the refraction functionality of STAR-RIS enhances the transmission capacity of FD systems, while its reflection functionality is used to eliminate self interference within the FD system. We delve into the applications of two different types of STAR-RIS in FD systems and compare their performance through simulations. Furthermore, we discuss the performance differences of STAR-RIS empowered FD systems under various configurations in a case study, and demonstrate the superiority of the proposed deep learning-based optimization algorithm. Finally, we discuss possible future research directions for STAR-RIS empowered FD systems.
This letter investigates machine learning approach for the joint optimal phase shift and beamforming in the reconfigurable intelligent surface (RIS) assisted multiple-input and multiple-output (MIMO) network, consisting of one source node, one RIS panel and one destination node. If individual source-to-RIS and RIS-to-destination channels are known, the joint optimization is similar to that in the traditional MIMO network, which has been well studied. However, the channel estimation for the individual channels is complicated and often inaccurate. On the other hand, while estimating the cascaded channels for the source-RIS-destination links are more accessible, the corresponding joint optimization is complicated. In this letter, we propose a novel double deep learning network model which is superior to the conventional reinforcement learning in the RIS joint optimization. Numerical simulations are given to verify the proposed algorithm.
This paper proposes a new framework for reconfigurable intelligent surface (RIS)-equipped unmanned aerial vehicles (UAVs) in free-space optical (FSO) communication. To ensure practicality, we consider atmospheric loss caused by fog, which leads to an inhomogeneous medium for laser propagation. In addition, we incorporate the pointing error loss caused by the power fraction on the photodetector (PD) into the system and derive a closed-form expression for the elliptical beam footprint in the pointing error loss. We then propose a leading angle assisted particle swarm optimization (PSO) method to efficiently optimize the numerical results of pointing error loss. Furthermore, after obtaining these numerical results as a precondition, the UAV trajectory is optimized using the proximal policy optimization (PPO) method to achieve the maximum average capacity. Numerical simulations demonstrate that the proposed optimization method achieves greater efficiency and accuracy compared to the decode-and-forward (DF) relay and deep Q-learning (DQN) methods.
This paper investigates a deep learning-based algorithm to optimize the unmanned aerial vehicle (UAV) trajectory and reconfigurable intelligent surface (RIS) reflection coefficients in UAV-RIS-aided cell-free (CF) hybrid non-orthogonal multiple-access (NOMA)/orthogonal multiple-access (OMA) networks. The practical RIS reflection model and user grouping optimization are considered in the proposed network. A double cascade correlation network (DCCN) is proposed to optimize the RIS reflection coefficients , and based on the results from DCCN, an inverse-variance deep reinforcement learning (IV-DRL) algorithm is introduced to address the UAV trajectory optimization problem. Simulation results show that the proposed algorithms significantly improve the performance in UAV-RIS-assisted CF networks.
This article proposes a novel machine-learning-based routing optimization for the multiple reconfigurable intelligent surfaces (M-RIS)-assisted multihop cooperative networks, in which a practical phase model for reconfigurable intelligent surface (RIS) with the amplitude variation based on the corresponding discrete phase shift is considered. We aim to maximize the end-to-end data rate in the proposed network by jointly optimizing the data transmission path, the passive beamforming design of RIS, and transmit power allocation. To tackle this complicated nonconvex problem, we divide it into two subtasks: 1) the passive beamforming design of the RIS and 2) joint routing and power allocation optimization. First, for the passive beamforming design of RIS, we develop a distributed learning algorithm that employs a cascade forward backpropagation network in each relay node to solve the RIS coefficients optimization problem by directly using the optimization target to train the cascade networks. This solution can avoid the curse of dimensionality of traditional reinforcement learning algorithms in the RIS optimization problem. Then, based on the result of RIS optimization, we introduce the proximal policy optimization (PPO) algorithm with the clipping method to find solutions for joint optimization of routing and power allocation via achieving the long-term benefit in the Markov decision process (MDP). Simulation results show that the proposed learning-based scheme can learn from the environment to improve its policy stability and efficiency in the iterative training process for optimizing routing and RIS and significantly outperform the benchmark schemes.
In this paper, we study the simultaneous wireless information and power transfer (SWIPT) cooperative system, where one source forwards information to one destination with the assistance of multiple relays. Each relay is equipped with a finite data buffer and a finite energy buffer storing the harvested energy by radio-frequency (RF). An optimization problem is formulated for throughput maximization of the SWIPT cooperative system, taking into consideration the strict delay constraint, dynamic channel conditions, time-varying discrete data buffer states and time-varying continuous energy buffer states. A discrete-time Markov decision process (MDP) is adopted to model the relay selection process referring to data buffer states and energy buffer states. Two deep Q-network (DQN)-based methods named invalid action penalty (IAP) and invalid action mask (IAM) are proposed. The simulation results show that the proposed IAM method can achieve better convergence and throughput performance than the IAP method.
This paper investigates a learning-based approach autonomously and jointly optimizing the trajectory of unmanned aerial vehicle (UAV), phase shifts of reconfigurable intelligent surfaces (RIS), and aggregation weights for federated learning (FL) in wireless communications, forming an autonomous RIS-assisted UAV-enabled network. The proposed network considers practical RIS reflection models and FL transmission errors in wireless communications. To optimize the RIS phase shifts, a double cascade correlation network (DCCN) is introduced. Additionally, the deep deterministic policy gradient (DDPG) algorithm is employed to address the optimization problem of UAV trajectory and FL aggregation weights based on the results obtained from DCCN. Simulation results demonstrate the substantial improvement in FL performance within the autonomous RIS-assisted UAV-enabled network setting achieved by the proposed algorithms compared to the benchmarks.