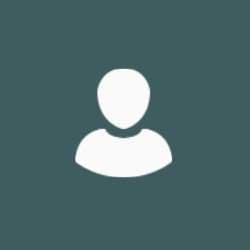
Dr Dimitrios Lazos
Publications
A Machine Learning approach to the problem of calculating the proton paths inside a scanned object in proton Computed Tomography is presented. The method is developed in order to mitigate the loss in both spatial resolution and quantitative integrity of the reconstructed images caused by multiple Coulomb scattering of protons traversing the matter. Two Machine Learning models were used: a forward neural network and the XGBoost method. A heuristic approach, based on track averaging was also implemented in order to evaluate the accuracy limits on track calculation, imposed by the statistical nature of the scattering. Synthetic data from anthropomorphic voxelized phantoms, generated by the Monte Carlo Geant4 code, were utilised to train the models and evaluate their accuracy, in comparison to a widely used analytical method that is based on likelihood maximization and Fermi-Eyges scattering model. Both neural network and XGBoost model were found to perform very close or at the accuracy limit, further improving the accuracy of the analytical method (by 12% in the typical case of 200MeV protons on 20 cm of water object), especially for protons scattered at large angles. Inclusion of the material information along the path in terms of radiation length did not show improvement in accuracy, for the phantoms simulated in the study. A neural network was also constructed to predict the error in path calculation, thus enabling a criterion to filter out proton events that may have a negative effect on the quality of the reconstructed image. By parametrizing a large set of synthetic data, the Machine Learning models were proved capable to bring - in an indirect and time efficient way - the accuracy of the Monte Carlo method into the problem of proton tracking.
A maximum likelihood approach to the problem of calculating the proton paths inside the scanned object in proton computed tomography is presented. Molière theory is used for first time to derive a physical model that describes proton multiple Coulomb scattering, avoiding the need for the Gaussian approximation currently used. To enable this, the proposed method approximates proton paths with cubic Bézier curves and subsequently maximizes the path likelihood through parametric optimization, based on the Molière model. Results from the Highland formula-based Gaussian approximation are also presented for comparison. The simplex method is utilized for optimisation. The scattering properties of the material(s) of the scanned object are taken into account by appropriately calculating the scattering parameters from the stopping power map that is calculated/updated at every iteration of the algebraic reconstruction process. Proton track length constraint imposed by the proton energy loss is also accounted for. The method is also applied in the case that no exit angle data are measured. Geant4 Monte Carlo simulations were performed for model validation. Our results show that use of Molière probability density function for modelling the multiple Coulomb scattering presents a modest 2% accuracy improvement over the Gaussian approximation and most-likely-path method. Simulations of voxelized phantom showed no essential benefit from the inclusion of the material information into the optimization, while path optimization with energy constraint slightly increased path resolution in a bone/water interface phantom. Method error was found to depend on energy, proton track-length within the medium, and proportion of data filtering.