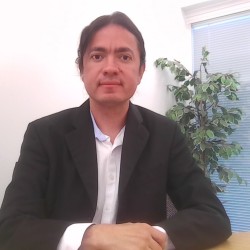
About
Biography
Fabio Dias is a computer scientist, entrepreneur and academic. A computing enthusiast, Dias self-taught computer programming while doing his secondary education and became a professional software developer at the age of 15.
Dias teaches financial modelling and applied computer science disciplines at the University of Surrey and is also the CEO of Stalwart Holdings, an academically-based investment management fintech with clients in the United Kingdom and Brazil.
Before joining the university he worked as an investment consultant in Brazil, then at the technology and risk management departments of Credit Suisse in London and New York, and subsequently became a senior manager at Lloyds Banking Group.
Areas of specialism
University roles and responsibilities
- Interim Programme Director of MSc FinTech and Policy
- Director for the relationship between the University of Surrey and Bloomberg LP
- Module leader for MANM525: Financial Modelling
- Module leader for MAN2200: Digital Coding
- Module leader for MANM493: Overview of Fintech
My qualifications
News
In the media
Supervision
Postgraduate research supervision
Supervisor to PhD student Yunwei Zhang.
Teaching
Module leader for disciplines in the fields of financial modelling and applied computer science, including but not limited to MANM525 (Financial Modelling), MANM493 (Overview of Fintech), MAN2200 (Digital Coding).
Publications
Recent technological advances have made possible the obtention of vast amounts of market data and strong computing power for advanced models which would not have been practicable for use in real market settings before. In this manuscript we devise a model-free empirical risk-neutral distribution based on Polynomial Chaos Expansions coupled with stochastic bridge interpolators that includes information from the entire set of observable European call option prices under all available strikes and maturities for a given underlying asset in a way that is guaranteed by construction to produce a valid state price distribution function at all times. We also obtain a non parametric model for the risk premium behaviour via an optimisation problem that joins the risk-neutral Polynomial Chaos Expansion result with any general model for the real-world distribution. Finally, we show an empirical application on SP500 Options on Futures using a real-world distribution that assumes the presence of signed path dependence in the returns of the underlying asset. (C) 2021 Published by Elsevier Inc.
While several tests for serial correlation in financial markets have been proposed and applied successfully in the literature, such tests provide rather limited information to construct predictive econometric models. This manuscript addresses this gap by providing a model-free definition of signed path dependence based on how the sign of cumulative innovations for a given lookback horizon correlates with the future cumulative innovations for a given forecast horizon. Such concept is then theoretically validated on well-known time series model classes and used to build a predictive econometric model for future market returns, which is applied to empirical forecasting by means of a profit-seeking trading strategy. The empirical experiment revealed strong evidence of serial correlation of unknown form in equity markets, being statistically significant and economically significant even in the presence of trading costs. Moreover, in equity markets, given a forecast horizon of one day, the forecasting strategy detected the strongest evidence of signed path dependence; however, even for longer forecast horizons such as 1 week or 1 month the strategy still detected such evidence albeit to a lesser extent. Currency markets also presented statistically significant serial dependence across some pairs, though not economically significant under the trading formulation presented.
Additional publications
The Transformative Impact of AI and Deep Learning in Business: A Literature Review; FS Dias and GA Lauretta; arXiv pre-print 2410.23443, 2024
Using conditional asymmetry to predict commodity futures prices; FS Dias; International Journal of Financial Markets and Derivatives 8 (2), 185-203, 2021
An equity-credit hybrid model for asset correlations; FS Dias; International Journal of Financial Engineering and Risk Management 3 (3), 223-239, 2020