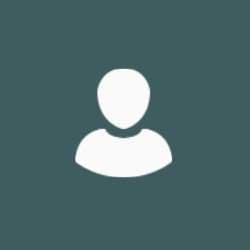
Farshad Einabadi
Publications
Leveraging machine learning techniques, in the context of object-based media production, could enable provision of personalized media experiences to diverse audiences. To fine-tune and evaluate techniques for personalization applications, as well as more broadly, datasets which bridge the gap between research and production are needed. We introduce and publicly release such a dataset, themed around a UK weather forecast and shot against a blue-screen background, of three professional actors/presenters – one male and one female (English) and one female (British Sign Language). Scenes include both production and research-oriented examples, with a range of dialogue, motions, and actions. Capture techniques consisted of a synchronized 4K resolution 16-camera array, production-typical microphones plus professional audio mix, a 16-channel microphone array with collocated Grasshopper3 camera, and a photogrammetry array. We demonstrate applications relevant to virtual production and creation of personalized media including neural radiance fields, shadow casting, action/event detection, speaker source tracking and video captioning.
Leveraging machine learning techniques, in the context of object-based media production, could enable provision of personalized media experiences to diverse audiences. To fine-tune and evaluate techniques for personalization applications, as well as more broadly, datasets which bridge the gap between research and production are needed. We introduce and publicly release such a dataset, themed around a UK weather forecast and shot against a blue-screen background, of three professional actors/presenters – one male and one female (English) and one female (British Sign Language). Scenes include both production and research-oriented examples, with a range of dialogue, motions, and actions. Capture techniques consisted of a synchronized 4K resolution 16-camera array, production-typical microphones plus professional audio mix, a 16-channel microphone array with collocated Grasshopper3 camera, and a photogrammetry array. We demonstrate applications relevant to virtual production and creation of personalized media including neural radiance fields, shadow casting, action/event detection, speaker source tracking and video captioning.
3DVHshadow contains images of diverse synthetic humans generated to evaluate the performance of cast hard shadow algorithms for humans. Each dataset entry includes (a) a rendering of the subject from the camera view point, (b) its binary segmentation mask, and (c) its binary cast shadow mask on a planar surface -- in total 3 images. The respective rendering metadata such as point light source position, camera pose, camera calibration, etc. is also provided alongside the images. Please refer to the corresponding publication for details of the dataset generation.
Scene relighting and estimating illumination of a real scene for insertion of virtual objects in a mixed-reality scenario are well-studied challenges in the computer vision and graphics fields. Classical inverse rendering approaches aim to decompose a scene into its orthogonal constituting elements, namely scene geometry, illumination and surface materials, which can later be used for augmented reality or to render new images under novel lighting or viewpoints. Recently, the application of deep neural computing to illumination estimation, relighting and inverse rendering has shown promising results. This contribution aims to bring together in a coherent manner current advances in this conjunction. We examine in detail the attributes of the proposed approaches, presented in three categories: scene illumination estimation, relighting with reflectance-aware scene-specific representations and finally relighting as image-to-image transformations. Each category is concluded with a discussion on the main characteristics of the current methods and possible future trends. We also provide an overview of current publicly available datasets for neural lighting applications.
This contribution introduces a two-step, novel neural rendering framework to learn the transformation from a 2D human silhouette mask to the corresponding cast shadows on background scene geometries. In the first step, the proposed neural renderer learns a binary shadow texture (canonical shadow) from the 2D foreground subject, for each point light source, independent of the background scene geometry. Next, the generated binary shadows are texture-mapped to transparent virtual shadow map planes which are seamlessly used in a traditional rendering pipeline to project hard or soft shadows for arbitrary scenes and light sources of different sizes. The neural renderer is trained with shadow images rendered from a fast, scalable, synthetic data generation framework. We introduce the 3D Virtual Human Shadow (3DVHshadow) dataset as a public benchmark for training and evaluation of human shadow generation. Evaluation on the 3DVHshadow test set and real 2D silhouette images of people demonstrates the proposed framework achieves comparable performance to traditional geometry-based renderers without any requirement for knowledge or computationally intensive, explicit estimation of the 3D human shape. We also show the benefit of learning intermediate canonical shadow textures, compared to learning to generate shadows directly in camera image space. Further experiments are provided to evaluate the effect of having multiple light sources in the scene, model performance with regard to the relative camera-light 2D angular distance, potential aliasing artefacts related to output image resolution, and effect of light sources' dimensions on shadow softness.