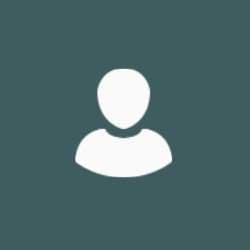
Professor Matthias Ketzel
Academic and research departments
Global Centre for Clean Air Research, School of Sustainability, Civil and Environmental Engineering.Publications
AIMS: We provide an overview of nationwide environmental data available for Denmark and its linkage potentials to individual-level records with the aim of promoting research on the potential impact of the local surrounding environment on human health.BACKGROUND: Researchers in Denmark have unique opportunities for conducting large population-based studies treating the entire Danish population as one big, open and dynamic cohort based on nationally complete population and health registries. So far, most research in this area has utilised individual- and family-level information to study the clustering of disease in families, comorbidities, risk of, and prognosis after, disease onset, and social gradients in disease risk. Linking environmental data in time and space to individuals enables novel possibilities for studying the health effects of the social, built and physical environment.METHODS: We describe the possible linkage between individuals and their local surrounding environment to establish the exposome - that is, the total environmental exposure of an individual over their life course.CONCLUSIONS: The currently available nationwide longitudinal environmental data in Denmark constitutes a valuable and globally rare asset that can help explore the impact of the exposome on human health.
OBJECTIVE: Early ecological studies have suggested links between air pollution and risk of COVID-19, but evidence from individual-level cohort studies is still sparse. We examined whether long-term exposure to air pollution is associated with risk of COVID-19 and who is most susceptible.METHODS: We followed 3 721 810 Danish residents≥30 years on March 1st 2020 in the National COVID-19 Surveillance System until the date of 1st positive test (incidence), COVID-19 hospitalization or death until April 26th 2021. We estimated residential annual mean particulate matter
Early ecological studies have suggested a link between air pollution and Coronavirus Diseases 2019 (COVID-19); however, the evidence from individual-level prospective cohort studies is still sparse. Here, we have examined, in a general population, whether long-term exposure to air pollution is associated with the risk of contracting severe acute respiratory syndrome coronavirus 2 (SARS-CoV-2) and developing severe COVID-19, resulting in hospitalization or death and who is most susceptible. We also examined whether long-term exposure to air pollution is associated with hospitalization or death due to COVID-19 in those who have tested positive for SARS-CoV-2. We included all Danish residents 30 years or older who resided in Denmark on March 1, 2020. and followed them in the National COVID-19 Surveillance System until first positive test (incidence), COVID-19 hospitalization, or death until April 26, 2021. We estimated mean levels of nitrogen dioxide (NO ), particulate matter with an aerodynamic diameter
BACKGROUND AND AIM: Several studies suggest associations between road traffic noise and incidence of dementia. However, findings are inconsistent when air pollution, a recognized risk factor for dementia, is considered. We investigated associations between air pollution, road traffic noise and incident dementia. METHOD: We followed 26,461 women from the Danish Nurses Cohort from baseline (1993 or 1999) to their first hospital contact or first prescription of dementia medication whichever came first until 2021 using Danish registers. Annual air pollution (PM2.5 and NO2) and road traffic noise estimates (Lden) were modelled at nurses’ residential address using DEHM/UBM/AirGIS and Nord2000 models, respectively. We applied time varying Cox models to estimate associations between 10- year running mean air pollution, noise and incident dementia. Models were adjusted for baselines year, individual lifestyle, socio-economic factors and municipality characteristics. RESULTS: A total of 1557 incident cases of dementia were during the follow up. Median exposures were 12.18 µg/m³ with interquartile range (IQR) of 5.79 µg/m³ for PM2.5, 16.51 µg/m³ (IQR 8.25µg/m³) for NO2 and 53.2 dB (IQR 9.3 dB) for Lden. We detected moderate associations between dementia incidence and PM2.5, with hazard ratio of 1.35 per IQR and 95% confidence interval (1.20-1.51), or NO2 (1.19; 1.09-1.29) and a weak positive association with Lden (1.04; 0.97-1.13). Associations with NO2 and Lden diminished when adjusting for PM2.5 with hazard ratios of 0.98 (0.86-1.12) and 0.95 (0.88-1.00) respectively. CONCLUSIONS: Our results shows a weak association between road traffic noise or traffic related pollutants and incident dementia, which may be partially explained by the effects of PM2.5.
Background: The etiology of childhood cancer is poorly understood. The role of environmental factors, including air pollution (AP) exposure, has been addressed previously, but results so far have been inconclusive. In this study, we investigate the association between long-term AP exposures in relation to childhood cancer subtypes in Denmark (1981À2013). Methods: We conducted a nationwide register-based case-control study. We identified 7745 incident cases of childhood cancers (
BACKGROUND AND AIM: Air pollution is major risk factor for chronic cardiorespiratory diseases, while the evidence on respiratory infections remains sparse. We examined the association between long-term exposure to air pollution and incidence and episodes of acute lower respiratory infections (ALRIs). METHOD: We followed 23,912 female nurses in Danish Nurse Cohort (aged 44) from baseline (1993 or 1999) to 2018. Using primary diagnosis, we defined ALRIs incidence as the first-ever hospital contact and ALRIs episodes as all hospital contacts after baseline. The residential annual mean concentrations of particulate matter with diameters ≤ 2.5 µm (PM2.5), nitrogen dioxide (NO2) and black carbon (BC), since 1979 were modelled by the Danish DEHM/UBM/AirGIS modeling system. Marginal Cox models with time-varying exposures were used to assess the association between 3-year mean air pollution and ALRIs incidence and episodes. Concentration-response functions (CRF) were assessed using natural cubic splines with 3 degrees of freedom, and effect modifications were assessed by adding interaction terms and likelihood ratio test. RESULTS: During mean 21.3 years’ follow-up, 4,746 ALRIs were observed, of which 2,553 were incident (first-ever) events. We observed strong associations of all three pollutants with ALRIs incidence, with hazard ratios and 95% confidence intervals of 1.19 (1.08-1.31) per 2.5 µg/m3 for PM2.5, 1.17 (1.11-1.24) per 8.0 µg/m3 for NO2, and 1.09 (1.05-1.12) per 0.3 µg/m3 for BC. We detected even stronger associations with ALRIs episodes. We found supra-linear CRFs for all pollutants, indicating a stronger association at lower concentrations. We identified people with baseline chronic respiratory diseases, or low physical activities were more susceptible to the adverse effect of air pollution. CONCLUSIONS: Long-term exposure to air pollution, even at low levels, was associated with risk of both new and subsequent ALRIs.
Background/aim Ambient air pollution has been associated with lung cancer, but the shape of the exposure-response function - especially at low exposure levels - is not well described. The aim of this study was to address the relationship between long-term low-level air pollution exposure and lung cancer incidence. Methods The “Effects of Low-level Air Pollution: a Study in Europe” (ELAPSE) collaboration pools seven cohorts from across Europe. We developed hybrid models combining air pollution monitoring, land use data, satellite observations, and dispersion model estimates for nitrogen dioxide (NO2), fine particulate matter (PM2.5), black carbon (BC), and ozone (O3) to assign exposure to cohort participants’ residential addresses in 100 m by 100 m grids. We applied stratified Cox proportional hazards models, adjusting for potential confounders (age, sex, calendar year, marital status, smoking, body mass index, employment status, and neighborhood-level socio-economic status). We fitted linear models, linear models in subsets, Shape-Constrained Health Impact Functions (SCHIF), and natural cubic spline models to assess the shape of the association between air pollution and lung cancer at concentrations below existing standards and guidelines. Results The analyses included 307,550 cohort participants. During a mean follow-up of 18.1 years, 3956 incident lung cancer cases occurred. Median (Q1, Q3) annual (2010) exposure levels of NO2, PM2.5, BC and O3 (warm season) were 24.2 µg/m3 (19.5, 29.7), 15.4 µg/m3 (12.8, 17.3), 1.6 10−5m−1 (1.3, 1.8), and 86.6 µg/m3 (78.5, 92.9), respectively. We observed a higher risk for lung cancer with higher exposure to PM2.5 (HR: 1.13, 95% CI: 1.05, 1.23 per 5 µg/m3). This association was robust to adjustment for other pollutants. The SCHIF, spline and subset analyses suggested a linear or supra-linear association with no evidence of a threshold. In subset analyses, risk estimates were clearly elevated for the subset of subjects with exposure below the EU limit value of 25 µg/m3. We did not observe associations between NO2, BC or O3 and lung cancer incidence. Conclusions Long-term ambient PM2.5 exposure is associated with lung cancer incidence even at concentrations below current EU limit values and possibly WHO Air Quality Guidelines.
It is unclear whether cancers of the upper aerodigestive tract (UADT) and gastric cancer are related to air pollution, due to few studies with inconsistent results. The effects of particulate matter (PM) may vary across locations due to different source contributions and related PM compositions, and it is not clear which PM constituents/sources are most relevant from a consideration of overall mass concentration alone. We therefore investigated the association of UADT and gastric cancers with PM elemental constituents and sources components indicative of different sources within a large multicentre population based epidemiological study. Cohorts with at least 10 cases per cohort led to ten and eight cohorts from five countries contributing to UADT- and gastric cancer analysis, respectively. Outcome ascertainment was based on cancer registry data or data of comparable quality. We assigned home address exposure to eight elemental constituents (Cu, Fe, K, Ni, S, Si, V and Zn) estimated from Europe-wide exposure models, and five source components identified by absolute principal component analysis (APCA). Cox regression models were run with age as time scale, stratified for sex and cohort and adjusted for relevant individual and neighbourhood level confounders. We observed 1139 UADT and 872 gastric cancer cases during a mean follow-up of 18.3 and 18.5 years, respectively. UADT cancer incidence was associated with all constituents except K in single element analyses. After adjustment for NO, only Ni and V remained associated with UADT. Residual oil combustion and traffic source components were associated with UADT cancer persisting in the multiple source model. No associations were found for any of the elements or source components and gastric cancer incidence. Our results indicate an association of several PM constituents indicative of different sources with UADT but not gastric cancer incidence with the most robust evidence for traffic and residual oil combustion.
Background Ambient air pollution is likely a risk factor for asthma, and recent evidence suggests the possible relevance of road traffic noise. Objectives We examined the associations of long-term exposure to air pollution and road traffic noise with adult-asthma incidence. Methods We followed 28,731 female nurses (age > 44 years) from the Danish Nurse Cohort, recruited in 1993 and 1999, for first hospital contact for asthma from 1977 until 2015. We estimated residential annual mean concentrations of particulate matter with diameter < 2.5 µm (PM2.5) since 1990 and nitrogen dioxide (NO2) since 1970 with the Danish DEHM/UBM/AirGIS modeling system, and road traffic noise (Lden) since 1970 with the Nord2000 model. Time-varying Cox regression models were used to associate air pollution and road traffic noise exposure with asthma incidence. Results During 18.6 years’ mean follow-up, 528 out of 23,093 participants had hospital contact for asthma. The hazard ratios (HR) and 95% confidence intervals for asthma incidence associated with 3-year moving average exposures were 1.29 (1.03, 1.61) per 6.3 µg/m3 for PM2.5, 1.16 (1.07, 1.27) per 8.2 µg/m3 for NO2, and 1.12 (1.00, 1.25) per 10 dB for Lden. The HR for NO2 remained unchanged after adjustment for either PM2.5 or Lden, while the HRs for PM2.5 and Lden attenuated to unity after adjustment for NO2. Conclusions Long-term exposure to air pollution was associated with adult-asthma incidence independently of road traffic noise, with NO2 most relevant. Road traffic noise was not independently associated with adult-asthma incidence.
We developed Europe-wide models of long-term exposure to eight elements (copper, iron, potassium, nickel, sulfur, silicon, vanadium, and zinc) in particulate matter with diameter
Particulate matter air pollution and diesel engine exhaust have been classified as carcinogenic for lung cancer, yet few studies have explored associations with liver cancer. We used six European adult cohorts which were recruited between 1985 and 2005, pooled within the “Effects of low-level air pollution: A study in Europe” (ELAPSE) project, and followed for the incidence of liver cancer until 2011 to 2015. The annual average exposure to nitrogen dioxide (NO2), particulate matter with diameter
Road traffic noise is the most pervasive source of ambient outdoor noise pollution in Europe. Traffic noise prediction models vary in parameterisation and therefore may produce different estimates of noise levels depending on the geographical setting in terms of emissions sources and propagation field. This paper compares three such models: the European standard, Common Noise Assessment Methods for the EU Member States (hereafter, CNOSSOS), Nord2000 and Traffic Noise Exposure (TRANEX) model based on the UK methodology, in terms of their source and propagation characteristics. The tools are also compared by analysing estimated noise (LAeq) from CNOSSOS, Nord2000 (2006 version), and TRANEX for more than one hundred test cases (N = 111) covering a variety of source and receiver configurations (e.g. varying source to receiver distance). The main aim of this approach was to investigate the potential pattern in differences between models’ performance for certain types of configurations. Discrepancies in performance may thus be linked to the differences in parameterisations of the CNOSSOS, Nord2000, and TRANEX (e.g. handling of diffraction, refraction). In most cases, both CNOSSOS and TRANEX reproduced LAeq levels of Nord2000 (2006 version) within three to five dBA (CNOSSOS: 87%, TRANEX: 94%). The differences in LAeq levels of CNOSSOS, compared to Nord2000, can be related to several shortcomings of the existing CNOSSOS algorithms (e.g. ground attenuation, multiple diffractions, and mean ground plane). The analyses show that more research is required in order to improve CNOSSOS for its implementation in the EU. In this context, amendments for CNOSSOS proposed by an EU Working Group hold significant potential. Overall, both CNOSSOS and TRANEX produced similar results, with TRANEX reproducing Nord2000 LAeq values slightly better than the CNOSSOS. The lack of measured noise data highlights one of the significant limitations of this study and needs to be addressed in future work.
Background
Particulate matter (PM) is one of the major pollutants emitted by vehicles that adversely affect human health and the environment. This study evaluates and predicts concentrations and dispersion patterns of PM10 and PM2.5 in Kuala Lumpur city centre. The OML-Highway model calculates hourly time series of PM10 and PM2.5 concentrations and distribution caused by traffic emissions under different scenarios; business as usual (BAU) and 30% traffic reduction to see the impact of traffic reduction for sustainable traffic management. Continuous PM10 and PM2.5 data from a nearby monitoring station were analysed for the year 2019 and compared with modelled concentrations. Annual average concentration at various locations of interest for PM10 and PM2.5 during BAU runs were in the ranges 41.4–65.9 µg/m3 and 30.4–43.7 µg/m3 respectively, compared to during the 30% traffic reduction run ranging at 40.5–59.5 µg/m3 and 29.9–40.3 µg/m3 respectively. The average concentration of PM10 and PM2.5 at the Continuous Air Quality Monitoring Station (CAQMS) was 36.4 µg/m3 and 28.2 µg/m3 respectively. Strong correlations were observed between the predicted and observed data for PM10 and PM2.5 in both scenarios (p < 0.05). This research demonstrated that the reduction of traffic volume in the city contributes to reducing the concentration of particulate matter pollution.
Modelling of ambient particle number concentrations (PNC) has been implemented in the Danish air quality modelling system DEHM/UBM/AirGIS and evaluated with long-term measurements. We implemented particle dynamical processes in the regional scale model DEHM using the M7 aerosol dynamics module (presented in the accompanying article by Frohn et al. 2021), and we developed models for PNC at the local scale (UBM) and street scale (OSPM), in a first approximation without including the particle dynamics as presented in this article. Outdoor concentration estimates are provided at the front door of all residential address locations in Denmark for the past 40 years (1979 – 2018) with a spatial resolution of 1 km x 1 km taking all emission sectors in Denmark into account and additionally at the street location, with significant traffic (> 500 vehicles / day). We evaluated our model with up to 18-year long measurement time series of particle number size distributions (PNSD) at Danish street, urban and rural background stations. Two particle size ranges were used for evaluation: PNC>10 (count of particles with diameter larger than 10 nm) and PNC30_250 (diameter range 30 to 250 nm), in order to exclude nucleation events from the measurements and to obtain a consistent long-term measured time series. When comparing our model estimates with PNC30_250 measurements, we obtain Pearson correlation coefficients (Rp) in the range 0.39-0.95 depending on station location (street, urban background, rural) and averaging time (hour, day, month, year). The highest correlations were found for yearly averages at a monitoring station located at a street with dense traffic (Rp=0.95) whereas shorter time averages and comparisons with monitoring stations at urban and rural background locations provided lower correlations. The model performance for PNC in terms of correlation coefficients with respect to measurements is comparable to the performance for other pollutants such as NOX , PM2.5 and better than the performance for PM10. The model generally overestimated the observed concentrations, Normalised Mean Bias (NMB) was in the range 6% to 190% compared to PNC>10 and 90% to 290% compared to PNC30_250. These relatively high NMBs are probably caused by uncertainties in the modelling process, especially the estimation of particle number emissions, which largely determine the ambient concentrations of PNC. Furthermore, uncertainties might as well originate from the complexity of modelling particle dynamical processes accurately and the great challenges in performing long-term PNC measurements. The presented model can estimate PNC at all Danish addresses over the last 40 years with a 1-hour time resolution. The data seem to provide a good indication of the relative differences in PNC at Danish addresses.
Objective Recent studies on air pollution and disease have been based on millions of participants within a region or country, relying entirely on register-based confounder adjustment. We aimed to investigate the effects of increasing adjustment for register- and questionnaire-based covariates on the association between air pollution and cardiometabolic diseases. Methods In a population-based cohort of 246,766 eligible participants randomly selected across Denmark in 2010 and 2013 and followed up until December 31, 2017, we identified 3,247 myocardial infarction (MI) cases, 4,166 stroke cases and 6,366 type 2 diabetes cases. Based on historical address-information, we calculated 5-year time-weighted exposure to PM2.5 and NO2 modelled using a validated air pollution model. We used Cox proportional hazards models to calculate hazard ratios (HR) with increasing adjustment for a number of individual- and area-level register-based covariates as well as lifestyle covariates assessed through questionnaires. Results We found that a 5 μg/m3 higher PM2.5 was associated with HRs (95% CI) for MI, stroke and diabetes, of respectively, 1.18 (0.91–1.52), 1.11 (0.88–1.40) and 1.24 (1.03–1.50) in the fully adjusted models. For all three diseases, adjustment for either individual-level, area-level or lifestyle covariates, or combinations of these resulted in higher HRs compared to HRs adjusted only for age, sex and calendar-year, most marked for MI and diabetes. Further adjustment for lifestyle in models with full register-based individual- and area-level adjustment resulted in only minor changes in HRs for all three diseases. Conclusions Our findings suggest that in studies of air pollution and cardiometabolic disease, which use an adjustment strategy with a broad range of register-based socioeconomic variables, there is no effect on risk estimates from subsequent lifestyle adjustment.
The integrated model system DEHM/UBM/AirGIS, developed at Aarhus University, Department of Environmental Science, has been extended with the dynamic aerosol module M7 to account for particle number concentrations of particles with diameters below 1 μm in the atmosphere. The aim of this development is to quantify the spatial and temporal distribution of particle number concentration across Denmark and evaluate the results with available measurements. This article presents model results for particle number concentrations from the regional scale model DEHM and the urban scale model UBM, for comparison with measurements of particle number concentrations from European and Danish measurement stations. The deterministic modelling of particle number concentration has been vitiated by the lack of consistency between emission inventories, and the evaluation of the models is challenged by the lack of consistent long-term measurements data. The performance evaluation of the DEHM and UBM models shows that both models overestimate the level of the particle number concentrations at all stations, however, the results for the correlation coefficients are 0.86 for DEHM and in the range from 0.86 to 0.87, for UBM, for annual mean particle number concentrations at Danish measurement stations. We conclude that the inclusion of particle number concentration in DEHM and UBM shows some capability of reproducing observed patterns, when comparing the results of the models with available measurements, but that there is also room for improvement, especially with respect to the emission inventories and preprocessing of emissions and to the treatment of volatile organic compounds based on natural emissions during summer time.
Few studies have investigated whether road traffic noise is associated with gestational diabetes mellitus (GDM), and have yielded inconsistent findings. We aimed to investigate whether maternal exposure to residential transportation noise, before and during pregnancy, was associated with GDM in a nationwide cohort. From the Danish population (2004–2017) we identified 629,254 pregnancies using the Danish Medical Birth Register. By linkage with the National Patient Registry, we identified 15,973 pregnancies complicated by GDM. Road traffic and railway noise (Lden) at the most and least exposed façades for all residential addresses from five years before pregnancy until birth were estimated for all. Analyses were conducted using generalized estimating equation models with adjustment for various individual and area-level sociodemographic covariates gathered from Danish registries, as well as green space and air pollution (PM2.5) estimated for all addresses. We found no positive associations between road traffic noise at either façade and GDM. For railway noise, a 10 dB increase in railway noise at the most and least exposed façades during the first trimester was associated with GDM, with an odds ratio (OR) of 1.06 (95% confidence interval (CI): 1.03–1.10) and 1.07 (95% CI: 1.02–1.13), respectively. We found indications of higher odds of GDM among women exposed to both high road traffic and railway noise at the least exposed facade during the first trimester (OR: 1.24; 95% CI: 1.07–1.44). In conclusion, this nationwide study suggests that railway noise but not road traffic noise might be associated with GDM.
Purpose Few studies have suggested that traffic noise is a risk factor for cancer, but evidence is inconclusive. We aimed to investigate whether road traffic and railway noise are associated with risk of colorectal cancer. Methods We obtained address history for all 3.5 million people above 40 years of age and living in Denmark for the period 1990-2017 and estimated road traffic and railway noise (L-den) at the most and least exposed facades of all addresses as well as air pollution (PM2.5). During follow-up (2000-2017), 35,881 persons developed colon cancer and 19,755 developed rectal cancer. Information on individual and area-level demographic and socioeconomic variables was collected from Danish registries. We analyzed data using Cox proportional hazards models, including traffic noise as time-varying 10-year average exposure. Results Exposure to road traffic noise at the most exposed facade was associated with an incidence rate ratio and 95% confidence interval for proximal colon cancer of 1.018 (0.999-1.038) per 10 dB higher noise. We observed no associations for road traffic noise at the least exposed facade or for railway noise in relation to proximal colon cancer. Also, we found no association between road traffic or railway noise and risk for distal colon cancer or rectal cancer. Conclusion Traffic noise did not seem associated with higher risk for colorectal cancer, although the suggestion of a slightly higher risk of proximal colon cancer following exposure to road traffic noise warrants further research.
In a recent study, we observed an increased risk of childhood non-Hodgkin lymphoma (NHL) associated with exposure to fine atmospheric particulate matter (PM2.5) and black carbon (BC). In this nationwide register-based case-control study, we focus on specific components of PM2.5 in relation to childhood NHL in Denmark (1981–2013) by identifying all incidents of childhood NHL cases in the Danish Cancer Registry (n = 170) and four (cancer-free) randomly selected controls matched by date of birth and sex. We applied PM2.5 concentrations and the following sub-components: secondary organic aerosols (SOA), secondary inorganic aerosols (SIA; i.e., NO3−, NH4+ and SO42−), BC, organic carbon (OC) and sea salt. We calculated a time-weighted exposure average from birth to index-date at all addresses. Odds ratios (ORs) were adjusted for register-based socio-demographic variables. We observed adjusted ORs and 95% confidence intervals (95% CI) of 2.05 (1.10, 3.83) per interquartile range (IQR, 4.83 µg/m3) PM2.5 and 1.73 (0.68, 4.41) per IQR (3.71 µg/m3) SIA, 0.95 (0.71, 1.29) per IQR (0.05 µg/m3) SOA, 1.22 (1.02, 1.46) per IQR (0.39 µg/m3) BC, 1.02 (0.83, 1.26) per IQR (0.56 µg/m3) OC and 1.01 (0.79, 1.30) per IQR (0.87 µg/m3) sea salt, respectively. The estimates were attenuated after adjustment for PM2.5, whereas the OR for PM2.5 remained increased regardless of adjustment for specific components. The findings indicate that the previously observed relation between PM2.5 and childhood NHL may be related to BC (as reported in our previous study) but also partly to SIA, but the role of specific chemical components of PM2.5 remains ambiguous.
Road transport is the main anthropogenic source of NOx in Europe, affecting human health and ecosystems. Thus, mitigation policies have been implemented to reduce on-road vehicle emissions, particularly through the Euro standard limits. To evaluate the effectiveness of these policies, we calculated NO2 and NOx concentration trends using air quality and meteorological measurements conducted in three European cities over 26 years. These data were also employed to estimate the trends in NOx emission factors (EFNOx, based on inverse dispersion modeling) and NO2:NOx emission ratios for the vehicle fleets under real-world driving conditions. In the period 1998–2017, Copenhagen and Stockholm showed large reductions in both the urban background NOx concentrations (−2.1 and −2.6% yr−1, respectively) and EFNOx at curbside sites (68 and 43%, respectively), proving the success of the Euro standards in diminishing NOx emissions. London presented a modest decrease in urban background NOx concentrations (−1.3% yr−1), while EFNOx remained rather constant at the curbside site (Marylebone Road) due to the increase in public bus traffic. NO2 primary emissions —that are not regulated— increased until 2008–2010, which also reflected in the ambient concentrations. This increase was associated with a strong dieselization process and the introduction of new after-treatment technologies that targeted the emission reduction of other species (e.g., greenhouse gases or particulate matter). Thus, while regulations on ambient concentrations of specific species have positive effects on human health, the overall outcomes should be considered before widely adopting them. Emission inventories for the on-road transportation sector should include EFNOx derived from real-world measurements, particularly in urban settings. [Display omitted] •NOx and NO2 reductions were seen in the background of the three cities in 1998–2017.•NO2 primary emissions increased until 2010 due to vehicle after-treatment systems.•EFNOx of the vehicle fleet decreased in Copenhagen (68%) and Stockholm (43%).•London showed the smallest reductions in ambient concentrations and EFNOx.•EFNOx was consistent with on-road studies but higher than simulated by emission models.
We evaluated methods for the analysis of multi-level survival data using a pooled dataset of 14 cohorts participating in the ELAPSE project investigating associations between residential exposure to low levels of air pollution (PM2.5 and NO2) and health (natural-cause mortality and cerebrovascular, coronary and lung cancer incidence). We applied five approaches in a multivariable Cox model to account for the first level of clustering corresponding to cohort specification: (1) not accounting for the cohort or using (2) indicator variables, (3) strata, (4) a frailty term in frailty Cox models, (5) a random intercept under a mixed Cox, for cohort identification. We accounted for the second level of clustering due to common characteristics in the residential area by (1) a random intercept per small area or (2) applying variance correction. We assessed the stratified, frailty and mixed Cox approach through simulations under different scenarios for heterogeneity in the underlying hazards and the air pollution effects. Effect estimates were stable under approaches used to adjust for cohort but substantially differed when no adjustment was applied. Further adjustment for the small area grouping increased the effect estimates’ standard errors. Simulations confirmed identical results between the stratified and frailty models. In ELAPSE we selected a stratified multivariable Cox model to account for between-cohort heterogeneity without adjustment for small area level, due to the small number of subjects and events in the latter. Our study supports the need to account for between-cohort heterogeneity in multi-center collaborations using pooled individual level data.
Air pollution has been shown to significantly impact human health including cancer. Gastric and upper aerodigestive tract (UADT) cancers are common and increased risk has been associated with smoking and occupational exposures. However, the association with air pollution remains unclear. We pooled European subcohorts (N = 287,576 participants for gastric and N = 297,406 for UADT analyses) and investigated the association between residential exposure to fine particles (PM ), nitrogen dioxide (NO ), black carbon (BC) and ozone in the warm season (O ) with gastric and UADT cancer. We applied Cox proportional hazards models adjusting for potential confounders at the individual and area-level. During 5,305,133 and 5,434,843 person-years, 872 gastric and 1139 UADT incident cancer cases were observed, respectively. For gastric cancer, we found no association with PM , NO and BC while for UADT the hazard ratios (95% confidence interval) were 1.15 (95% CI: 1.00-1.33) per 5 μg/m increase in PM , 1.19 (1.08-1.30) per 10 μg/m increase in NO , 1.14 (1.04-1.26) per 0.5 × 10 m increase in BC and 0.81 (0.72-0.92) per 10 μg/m increase in O . We found no association between long-term ambient air pollution exposure and incidence of gastric cancer, while for long-term exposure to PM , NO and BC increased incidence of UADT cancer was observed.
Background Long-term exposure to outdoor air pollution increases the risk of cardiovascular disease, but evidence is unclear on the health effects of exposure to pollutant concentrations lower than current EU and US standards and WHO guideline limits. Within the multicentre study Effects of Low-Level Air Pollution: A Study in Europe (ELAPSE), we investigated the associations of long-term exposures to fine particulate matter (PM2·5), nitrogen dioxide (NO2), black carbon, and warm-season ozone (O3) with the incidence of stroke and acute coronary heart disease. Methods We did a pooled analysis of individual data from six population-based cohort studies within ELAPSE, from Sweden, Denmark, the Netherlands, and Germany (recruited 1992–2004), and harmonised individual and area-level variables between cohorts. Participants (all adults) were followed up until migration from the study area, death, or incident stroke or coronary heart disease, or end of follow-up (2011–15). Mean 2010 air pollution concentrations from centrally developed European-wide land use regression models were assigned to participants’ baseline residential addresses. We used Cox proportional hazards models with increasing levels of covariate adjustment to investigate the association of air pollution exposure with incidence of stroke and coronary heart disease. We assessed the shape of the concentration-response function and did subset analyses of participants living at pollutant concentrations lower than predefined values. Findings From the pooled ELAPSE cohorts, data on 137 148 participants were analysed in our fully adjusted model. During a median follow-up of 17·2 years (IQR 13·8–19·5), we observed 6950 incident events of stroke and 10 071 incident events of coronary heart disease. Incidence of stroke was associated with PM2·5 (hazard ratio 1·10 [95% CI 1·01–1·21] per 5 μg/m3 increase), NO2 (1·08 [1·04–1·12] per 10 μg/m3 increase), and black carbon (1·06 [1·02–1·10] per 0·5 10−5/m increase), whereas coronary heart disease incidence was only associated with NO2 (1·04 [1·01–1·07]). Warm-season O3 was not associated with an increase in either outcome. Concentration-response curves indicated no evidence of a threshold below which air pollutant concentrations are not harmful for cardiovascular health. Effect estimates for PM2·5 and NO2 remained elevated even when restricting analyses to participants exposed to pollutant concentrations lower than the EU limit values of 25 μg/m3 for PM2·5 and 40 μg/m3 for NO2. Interpretation Long-term air pollution exposure was associated with incidence of stroke and coronary heart disease, even at pollutant concentrations lower than current limit values.
The contribution of vehicle emissions to air pollution is considered a large environmental and health problem in big Brazilian cities caused, among other factors, by slow renewal of the old vehicle fleet. Brazilian studies usually only consider traffic-related issues in transportation analysis, with minor assessments of emissions and close to non-existent assessment of air quality. On this background, this research aimed to calibrate and evaluate the Operational Street Pollution Model (OSPM®) to Brazilian conditions by implementing Brazilian emission factors. The urban background concentrations were modeled with the Urban Background Model (UBM) as part of the air quality system (THOR-AirPAS). In this case, we used meteorological data from a ground meteorological station outside Fortaleza processed by meteorological pre-processor and regional background concentrations from the Integrated Forecast System (IFS) as input to UBM. New air quality measurements were collected in busy streets of the city of Fortaleza during the year of 2017. The study collected samples of daily NO and PM concentrations to evaluate OSPM daily estimations. In addition, a transportation travel demand model (TRANUS) has been calibrated to the case study area with observed traffic data collected, in order to provide Annual Average Daily Traffic (AADT) as inputs to OSPM®. Two sets of emission factors were evaluated. Official Brazilian emission factors were applied to OSPM®, as well as adjusted emission factors derived in the project based on calibration that were higher than the official emission factors. Data showed that concentrations are significantly influenced by meteorological factors (such as temperature, wind speeds, wind directions), and especially precipitation for PM concentrations. OSPM® simulated results showed concentration levels and patterns close to air quality measurements with default emission factors and calibrated emission for UBM but large underestimations if official emissions were used for both UBM and OSPM. : Busy urban streets in Brazilian cities with intense flow of diesel vehicles (such as buses and trucks) can significantly increase air pollution, especially for NO and PM . With OSPM calibrated and evaluated to Brazilian conditions, the model system can be used by authorities to assess the impact of policy measures, such as vehicle access restrictions in Low Emission Zones, in order to consider not only traffic related issues, but also air pollution due to mobile sources with outdated emission technologies.
Purpose The etiology of Hodgkin lymphoma (HL) is obscure. Research on air pollution and risk of HL provides inconsistent results. We aimed to investigate the association between long-term residential exposure to air pollution and risk of adult Hodgkin lymphoma in Denmark. Methods We performed a nationwide register-based case–control study, including all (n = 2,681) Hodgkin lymphoma cases registered in the nationwide Danish Cancer Registry between 1989 and 2014. We randomly selected 8,853 age- and sex-matched controls from the entire Danish population using the Civil Registration System, and identified 20-year residential address history for all cases and controls. We modeled outdoor air pollution concentrations at all these addresses using the high-resolution multiscale air pollution model system DEHM/UBM/AirGIS. We used conditional logistic regression to estimate odds ratios adjusted for individual and neighborhood level sociodemographic variables. Results There was no association between 1, 5, 10, and 20 years’ time-weighted average exposure to fine particles (PM2.5), O3, SO2, NO2, or the PM2.5 constituents OC, NH4, NO3, and SO4 and risk of Hodgkin lymphoma. Conclusion Residential exposure to ambient air pollution does not seem to increase the risk of developing Hodgkin lymphoma.
Background: Associations between long-term exposure to air pollution and road traffic noise have been established for ischemic heart disease, but findings have been mixed for atrial fibrillation (AF). Objectives: The goal of the study was to examine associations of long-term exposure to road traffic noise and air pollution with AF. Methods: Time-varying Cox regression models were used to estimate associations of 1-, 3-, and 23-y mean road traffic noise and air pollution exposures with AF incidence in 23,528 women enrolled in the Danish Nurse Cohort (age >44y at baseline in 1993 or 1999). AF diagnoses were ascertained via the Danish National Patient Register. Annual mean weighted 24-h average road traffic noise levels (Lden) at the nurses’ residences, since 1970, were estimated using the Nord2000 model, and annual mean levels of particulate matter with a diameter 58 dB vs.
Background: While air pollution has been linked to the development of chronic obstructive pulmonary disease (COPD), evidence on the role of environmental noise is just emerging. We examined the associations of long-term exposure to air pollution and road traffic noise with COPD incidence. Methods: We defined COPD incidence for 24 538 female nurses from the Danish Nurse Cohort (age>44 years) as the first hospital contact between baseline (1993 or 1999) and 2015. We estimated residential annual mean concentrations of particulate matter with diameter
Background: Leukemia is one of the most common forms of hematologic malignancy, which can affect people of all ages. We previously showed an association between exposure to ambient particulate matter 2.5 μg (PM2.5) and risk for leukemia in adults. The aim of this study was to investigate which PM2.5 constituents were responsible for our previous observation. Methods: This is a nationwide register-based case-control study. We identified 14,983 persons diagnosed with leukemia at age 20 or above, 1989–2014, in the Danish Cancer Registry. We selected up to four sex and age-matched controls per case at random from the entire Danish population (n = 51,613). We modelled concentrations of ambient PM2.5 and its constituents at the addresses of cases and controls for the 10-year period before index date with a state-of-the-art multiscale air pollution modeling system. We used conditional logistic regression to estimate odds ratios (ORs) adjusted for individual and neighborhood level socio-demographic variables. Result: The results showed higher risk for overall leukemia in association with interquartile range exposure to PM2.5 (OR = 1.09; 95% CI: 1.02, 1.17), black carbon (BC) (OR = 1.02; 95% CI: 1.00, 1.03), secondary inorganic aerosols (SIA) (OR = 1.15; 95% CI: 1.03, 1.29) and its components ammonium (NH4) (OR = 1.08; 95% CI: 1.00, 1.17) and nitrate (NO3) (OR = 1.08; 95% CI: 1.02, 1.14). In leukemia subtype analysis, statistically significant associations were found for AML with PM2.5 (OR = 1.14; 95% CI: 1.00, 1.29), BC (OR = 1.03; 95% CI: 1.00, 1.07), SIA (OR = 1.23; 95% CI: 1.01, 1.51), NH4 (OR = 1.16; 95% CI: 1.01, 1.34) and NO3 (OR = 1.12; 95% CI: 1.01, 1.24). The association between PM2.5 and leukemia persisted in two pollutants models including sum of primary emitted black and organic carbon (BC + OC), secondary organic aerosols (SOA), or sea-salt. The association between black carbon (BC) and leukemia persisted in two pollutants models including organic carbon (OC). The three pollutant model with sulfate (SO4), NH4 and NO3 showed an association with NO3 but not with SO4 or NH4. Conclusion: Ambient concentrations of the PM2.5 components BC, NH4 and NO3 at the residence showed associations with risk of incident leukemia in adults.
The aetiology of most childhood cancers remains poorly understood. We conducted a nationwide register-based case-control study to assess the association between residential road traffic and railway noise exposure and risk of childhood cancers. We identified all cases of first cancers diagnosed in children aged 0-19 years in 1985-2013 from the Danish Cancer Registry (N = 3962) and sampled four individually matched (by sex and date of birth) controls per case (N = 14,790) using the Central Population Register. We estimated time-weighted exposure averages of residential road traffic and railway noise at the most (L max) and least (L min) exposed façades from birth to index-date (for additional analysis: in utero period) based on the individual address history for the respective time windows. We fitted conditional logistic regression models to estimate odds ratios (OR) and their 95% confidence intervals (CI). ORs varied by noise estimate and cancer type, with generally wide CIs mostly including 1.00. We found a tendency of higher ORs with increasing railway and road traffic noise for Hodgkin lymphoma (ORs for railway and road L min were 1.63 (95% CI 1.00; 2.66) and 1.14 (95% CI 0.87; 1.48) per 10 dB), as well as a tendency of higher ORs with increasing railway noise for non-Hodgkin lymphoma. For embryonal CNS tumours and astrocytoma and other glioma we observed also some weak suggestions of a positive association. Analysing exposure to traffic noise in utero revealed similar patterns to those of the main analyses. This nationwide study with minimal risk of bias suggests no strong associations between traffic noise and risk of most childhood cancers. We found however some suggestive evidence for a positive association with Hodgkin lymphoma, non-Hodgkin lymphoma and some CNS tumours. Further research is warranted to confirm these associations in other populations and elucidate the underlying biological mechanisms.
Background: Inconsistent associations between long-term exposure to particles with an aerodynamic diameter ≤2.5 μm [fine particulate matter (PM2.5)] components and mortality have been reported, partly related to challenges in exposure assessment. Objectives: We investigated the associations between long-term exposure to PM2.5 elemental components and mortality in a large pooled European cohort; to compare health effects of PM2.5 components estimated with two exposure modeling approaches, namely, supervised linear regression (SLR) and random forest (RF) algorithms. Methods: We pooled data from eight European cohorts with 323,782 participants, average age 49 y at baseline (1985–2005). Residential exposure to 2010 annual average concentration of eight PM2.5 components [copper (Cu), iron (Fe), potassium (K), nickel (Ni), sulfur (S), silicon (Si), vanadium (V), and zinc (Zn)] was estimated with Europe-wide SLR and RF models at a 100×100 m scale. We applied Cox proportional hazards models to investigate the associations between components and natural and cause-specific mortality. In addition, two-pollutant analyses were conducted by adjusting each component for PM2.5 mass and nitrogen dioxide (NO2) separately. Results: We observed 46,640 natural-cause deaths with 6,317,235 person-years and an average follow-up of 19.5 y. All SLR-modeled components were statistically significantly associated with natural-cause mortality in single-pollutant models with hazard ratios (HRs) from 1.05 to 1.27. Similar HRs were observed for RF-modeled Cu, Fe, K, S, V, and Zn with wider confidence intervals (CIs). HRs for SLR-modeled Ni, S, Si, V, and Zn remained above unity and (almost) significant after adjustment for both PM2.5 and NO2. HRs only remained (almost) significant for RF-modeled K and V in two-pollutant models. The HRs for V were 1.03 (95% CI: 1.02, 1.05) and 1.06 (95% CI: 1.02, 1.10) for SLR- and RF-modeled exposures, respectively, per 2 ng/m3, adjusting for PM2.5 mass. Associations with cause-specific mortality were less consistent in two-pollutant models. Conclusion: Long-term exposure to V in PM2.5 was most consistently associated with increased mortality. Associations for the other components were weaker for exposure modeled with RF than SLR in two-pollutant models. https://doi.org/10.1289/EHP8368