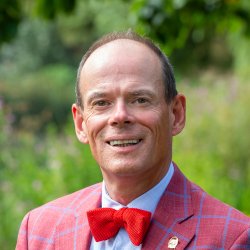
Professor Nick Ryman-Tubb
Academic and research departments
Surrey Business School, Faculty of Arts, Business and Social Sciences, Computer Science Research Centre.About
Biography
Teaching MSc Business Analytics and MSc Data Science and looking for amazing students to extend AI/ML research in the area of financial fraud.
For nearly 30-years, I have focused on the detection and reduction of financial fraud; specifically in payments. My research aims to reconcile symbolic AI and neural deep learning paradigms to move to the next generation of intelligent systems for fraud detection.
I am passionate about the practical application of machine learning and AI in business to really make a difference; teaching the next generation of "Data Whispers" is an honour.
I started my lifelong industry career in AI/Machine learning/Neural Computing in 1980s and was a pioneer of the practical deployment of machine learning in a diverse range of applications – with worldwide recognised “firsts”. I founded my own machine learning and data science company and grew this with offices in London, Singapore, Sydney and New York. I managed all aspects of the business with a focus on our research scientists and software development teams. Established myself as a leader in applying machine learning research to solve real-world problems.
I have a track record of computational neuroscience applied and deployed as solutions across industry, including credit/risk scoring (deployed in 35+ banks) and payment fraud detection (deployed at over 150+ institutions), which is today my main focus of research.
Following the sale of my business, I exited as the CEO in 2000 and continued my research, as well as a passion for teaching. Here, I work to deploy novel and practical AI, machine learning and deep learning to reduce financial crime with a focus on fraud detection in payment cards and AML.
Areas of specialism
University roles and responsibilities
- MSc Business Analytics (Machine Learning) - Teaching
- MSc Data Science (Practical Business Analytics) - Teaching
- Supervisor (MSc or PhD)
My qualifications
Previous roles
A Selection of Past AI/ML Industry Projects
- Identification of Customers Unlikely to Renew their Motor Breakdown Insurance using Neural Modelling– RAC
- Prediction of High-Spending Customers and their Product Types in Catalogue Company using Neural Data Mining– Freemans
- Blood Speckle Tracking using Neural Image Processing to Detect Cancer – Royal Marsden Hospital / Institute of Cancer Research
- Identification of Electricity Users to Predict Demand for Forward Purchasing of Electricity – Southern Electricity
- Oil Pipe Corrosion Detection – using non-intrusive sensors and neural signal processing – BP Oil (Alaska)
- Recognition of Coins for Vending Machines using Neural SOM Techniques with Low False-Positives – Mars Electronics
- Pizza Manufacturing Quality Control – Neural Image Processing – United Biscuits
- Quality Control in Manufacturing of Automotive Gear Box Assemblies using Laser Scanner and Neural Image Processing – Ford UK
- Identification of Defecting Photocopier Customers using Neural Data Mining – Rank Xerox
- Whisky Blending Analysis using a Novel bio-silicon Sensor and Neural DSP Processing – United Distillers
- Robot Control of Electric Wheelchair for Severely Disabled Persons Using Neural Computing and Fuzzy Logic for Navigation and User Input – TIC / DTI SMART Award
- Final Automatic Testing of DC Motors using Neural Digital Signal Processing – PMM plc.
- Locating Gold Ore Deposits in South Africa Using Neural Data Fusion and Anomaly Detection – Anglo America
- Early Detection of Wireless Telecom Subscribers Likely to Churn – Cellcom UK
- Early Prediction of Wireless Base-station Failure in Large UK Cellular Network using Neural Anomaly Detection – Orange plc.
- Optimising Delivery of Perishable Plants and flowers from Supplier/Depot to Shop – using Neural Combinatorial Optimisation (TSP[*]) Ring Network – Sainsbury’s Homebase
- Detection of Unusual Trading Behaviour and Fraud on the Trading Floor using Anomaly Detection – JP Morgan
- Prediction of FX US$ rates using Neural Networks – Citibank
- Scoring of Individuals for Automotive Loans to reduce Bad Debt – Lloyds Bowmaker
- Implementation of Hardware Parallel Processing System (16 Processors) for Real-Time Signal Analysis and recognition
- Implementation of a VMEbus[†]Based Image Processing Card with Neural Processor – Vero Microelectronics
- Speech Recognition system using HMM[‡]and Neural Networks to give Superior Speaker Independent Word Recognition in Noisy Environments
- SX-EW Process Optimisation for Copper Extraction
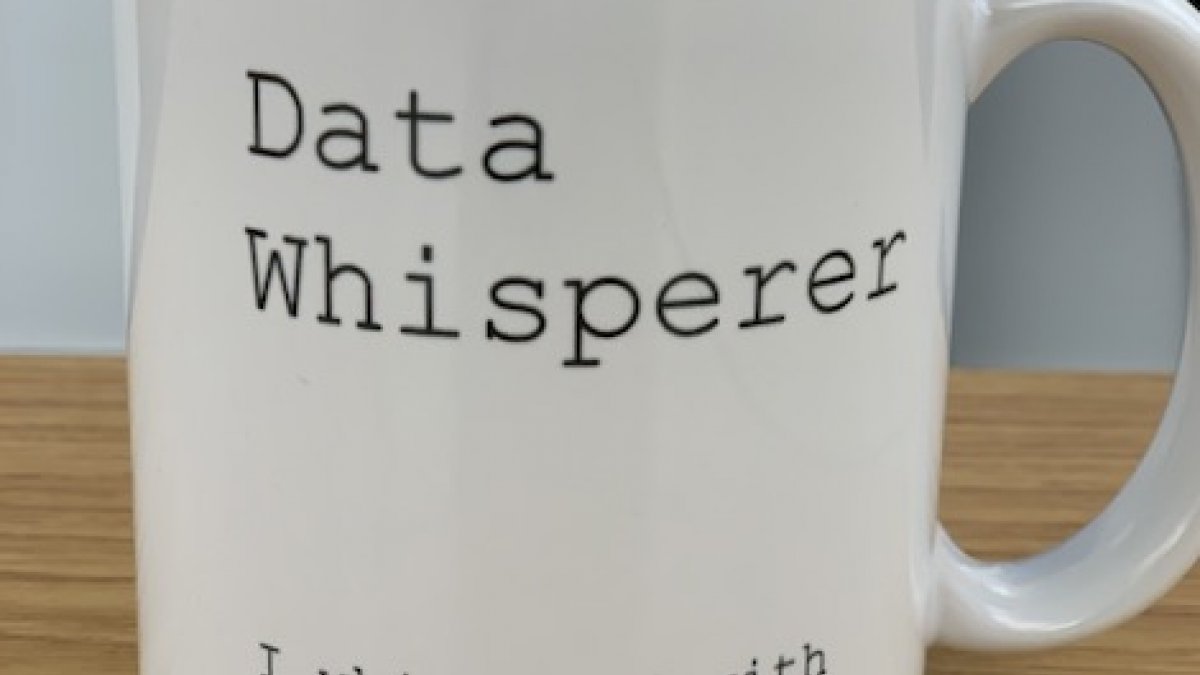
Machine Learning Class 2019
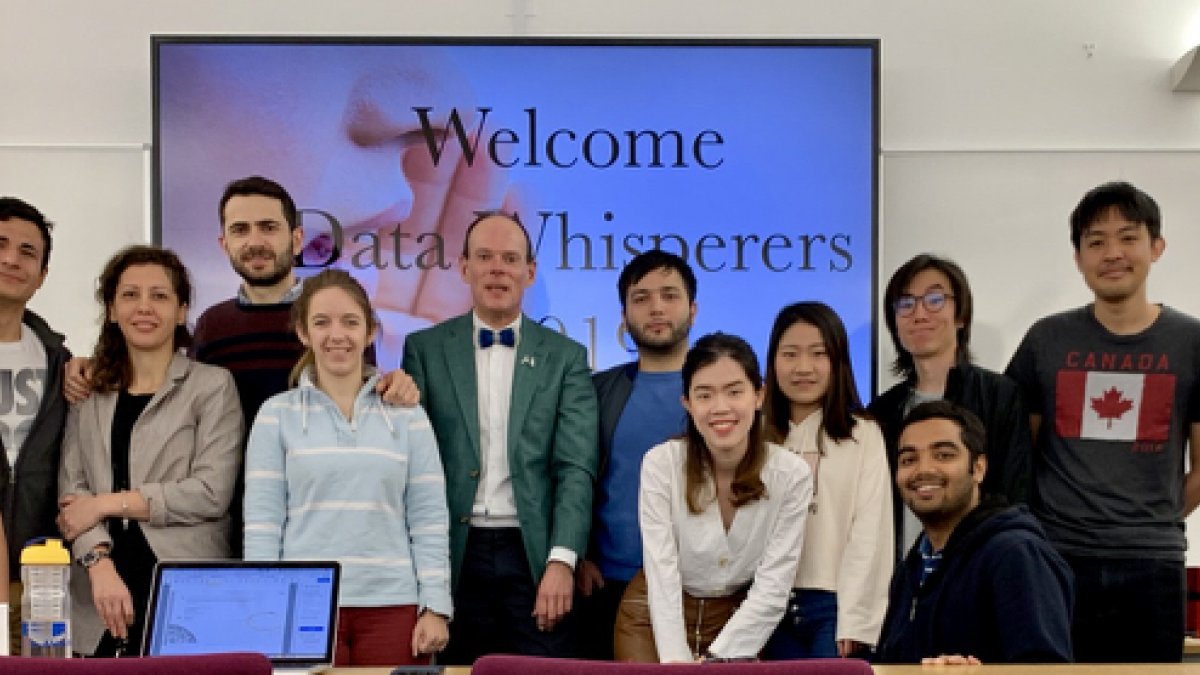
News
First: Combining Neural Network & Organic Sensors
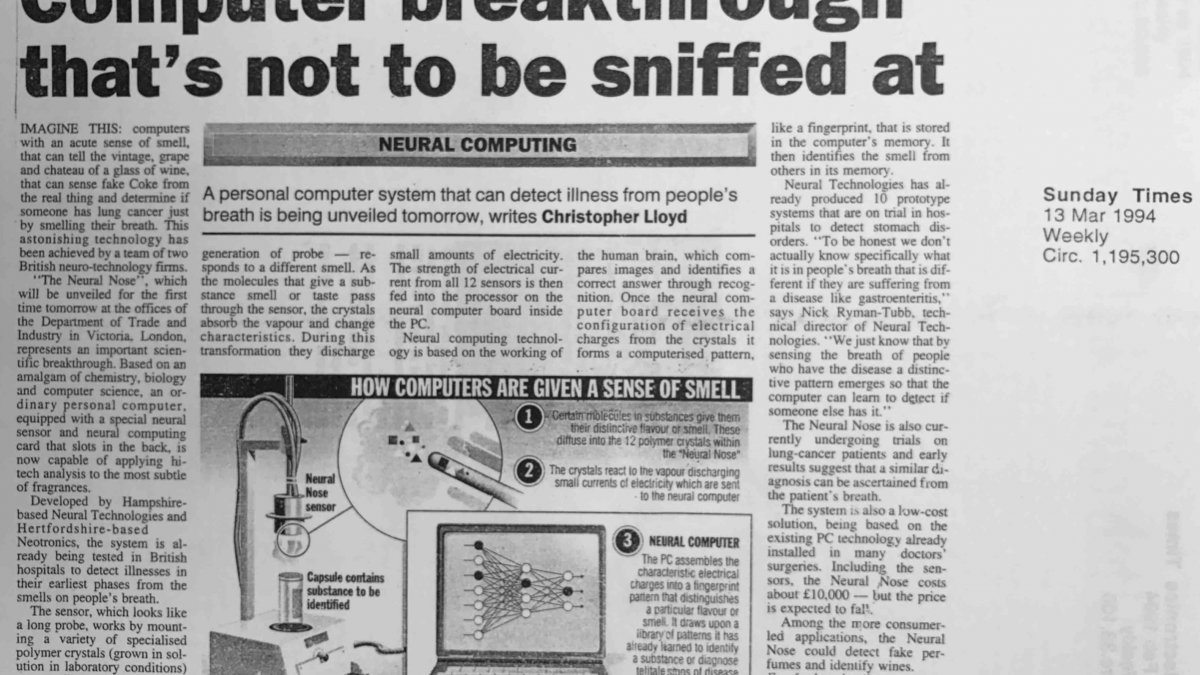
Neural computer applied to mining minerals
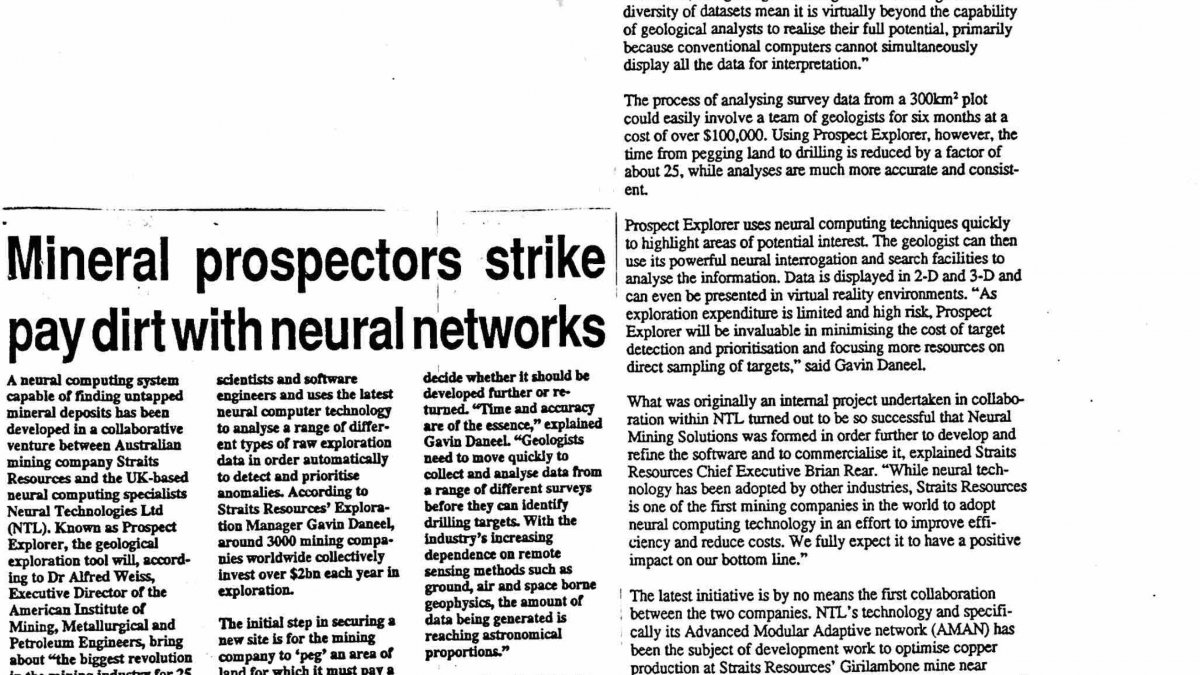
Trading pairs using neural networks
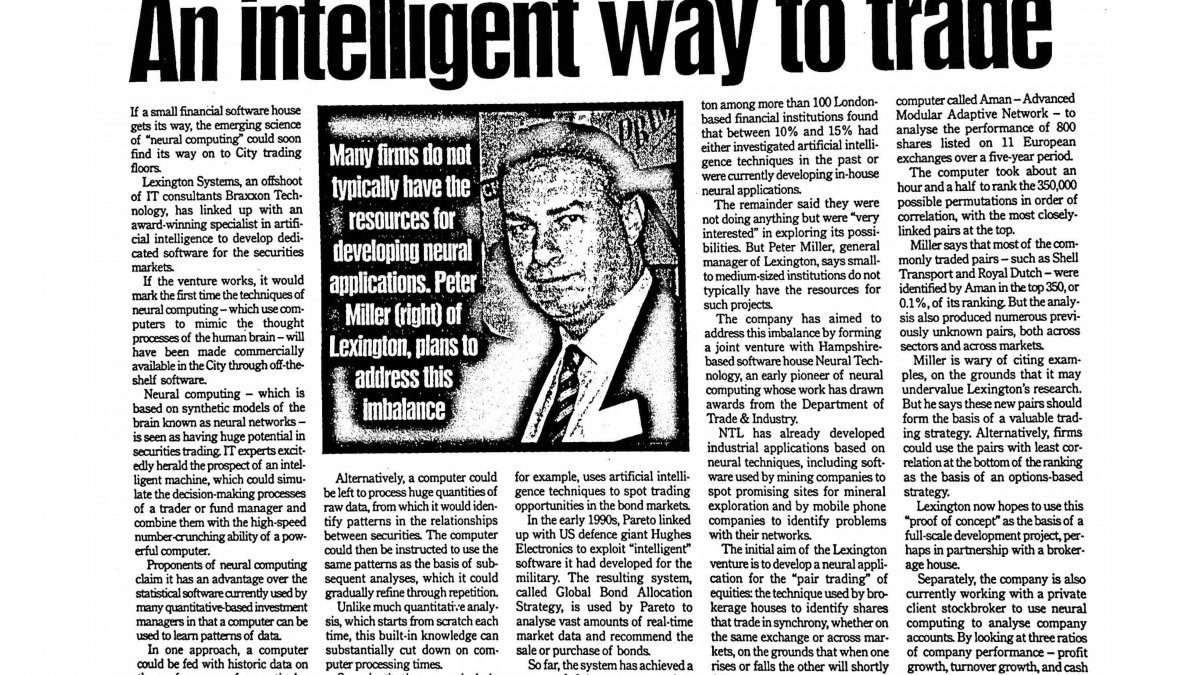
First neural network data mining application in Europe
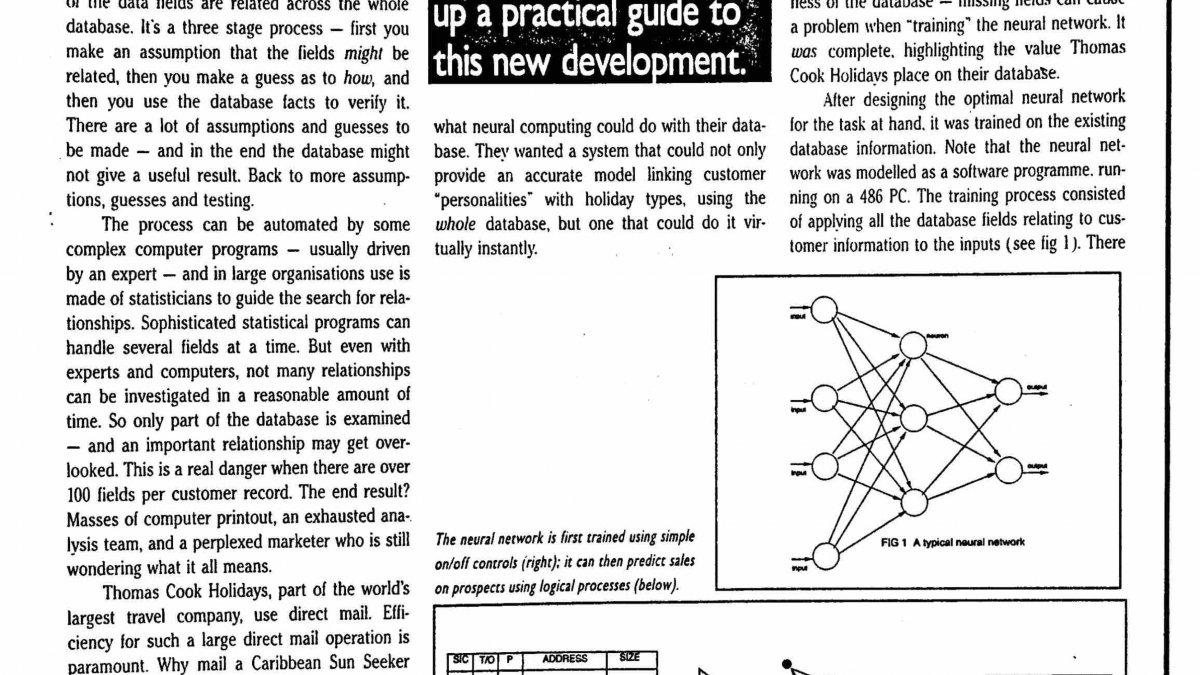
First use of AI to find minerals
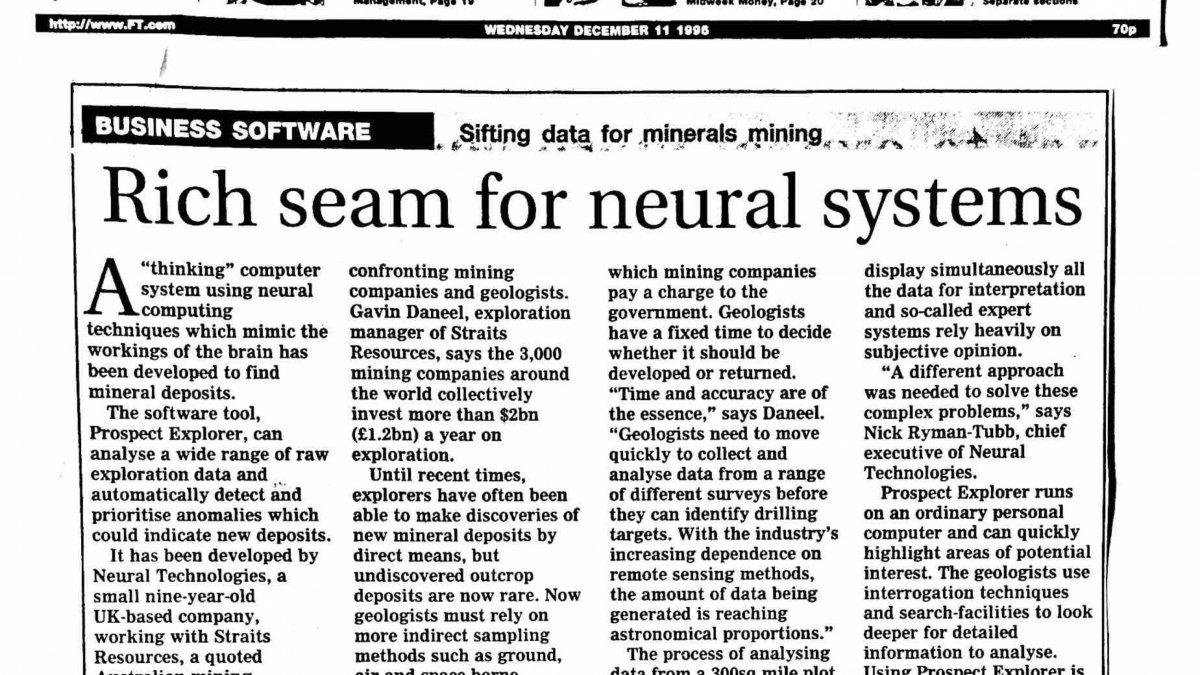
Use of Neural Networks to Detect Fraud in Telecoms
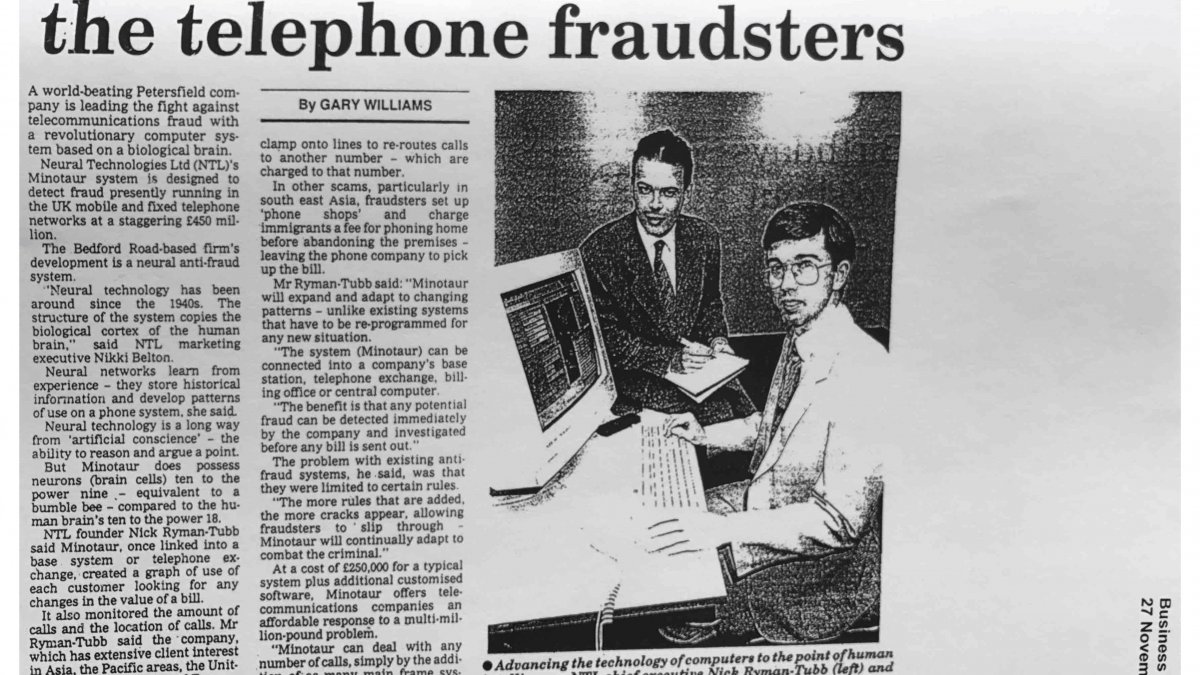
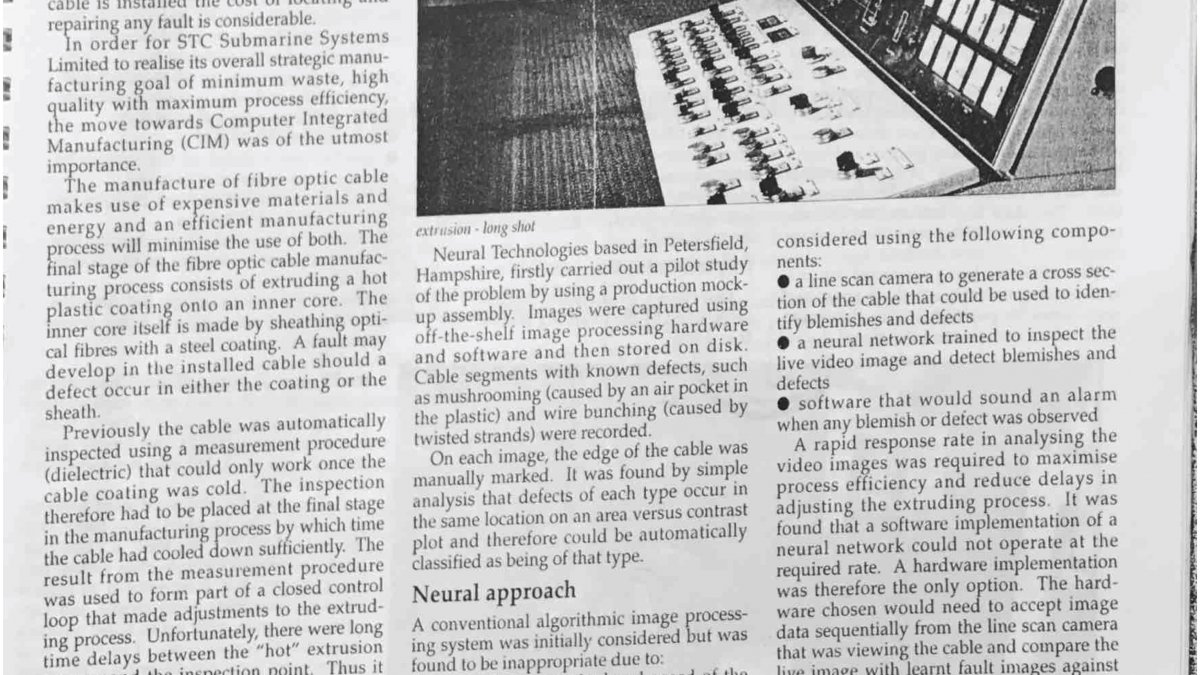
Breakthrough in Big Data Mining
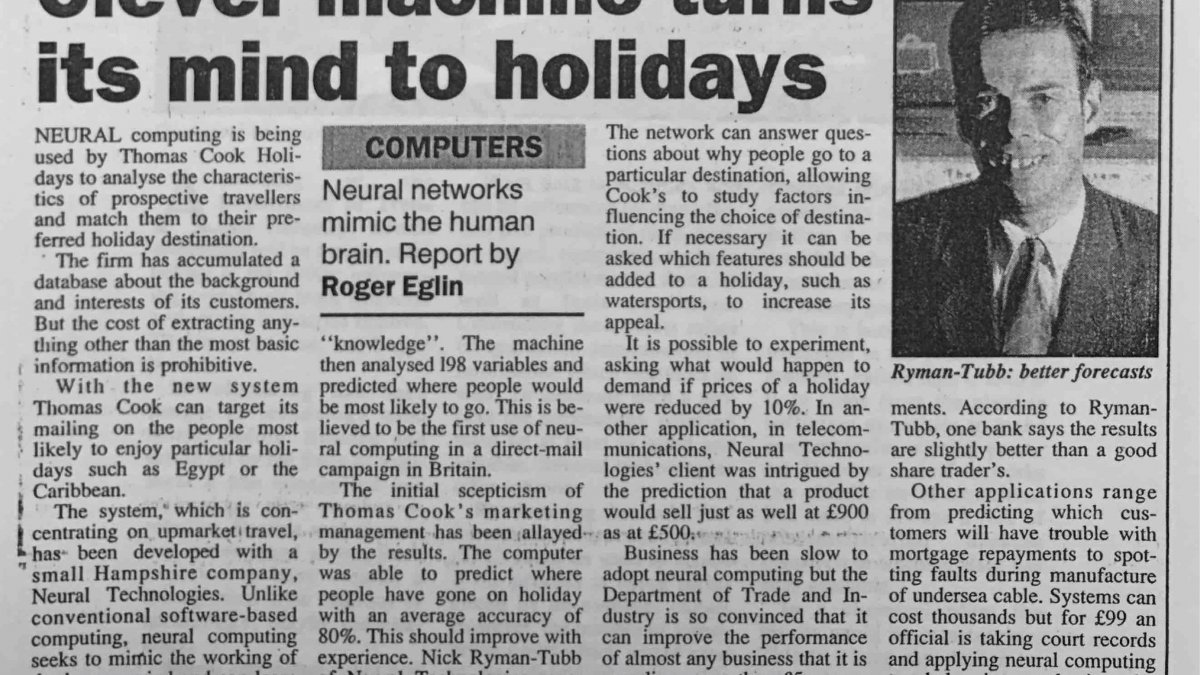
Europes First neural network chip - NiSP
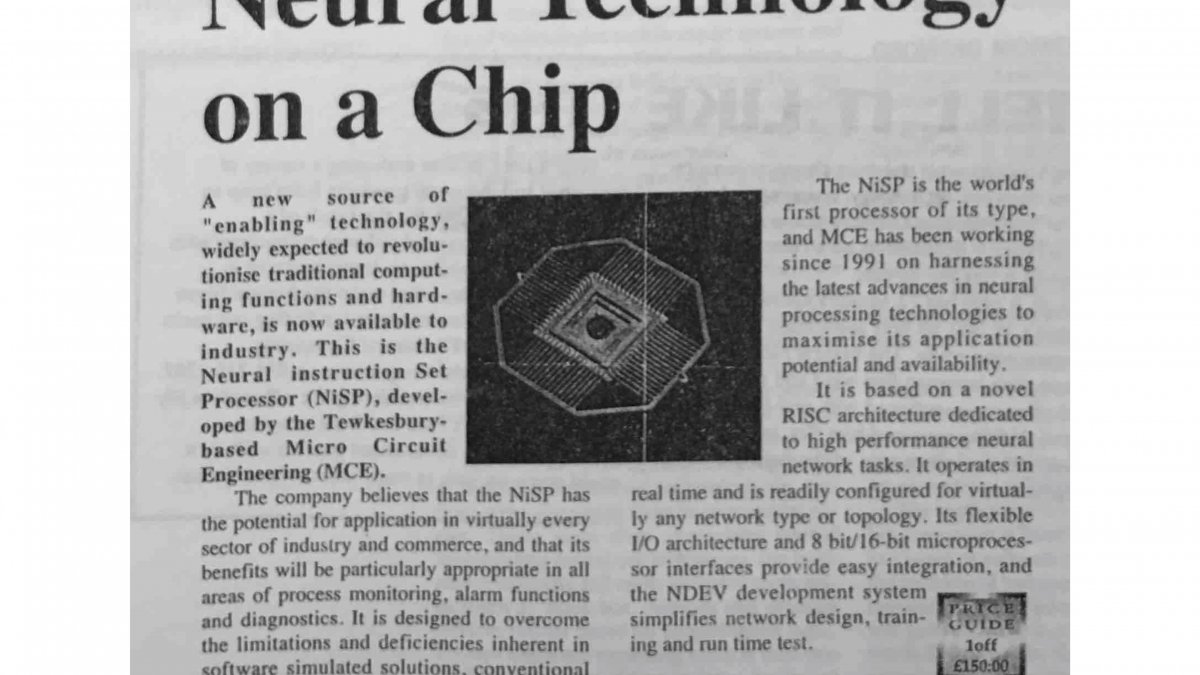
Neural Networks in USA Banking Applications
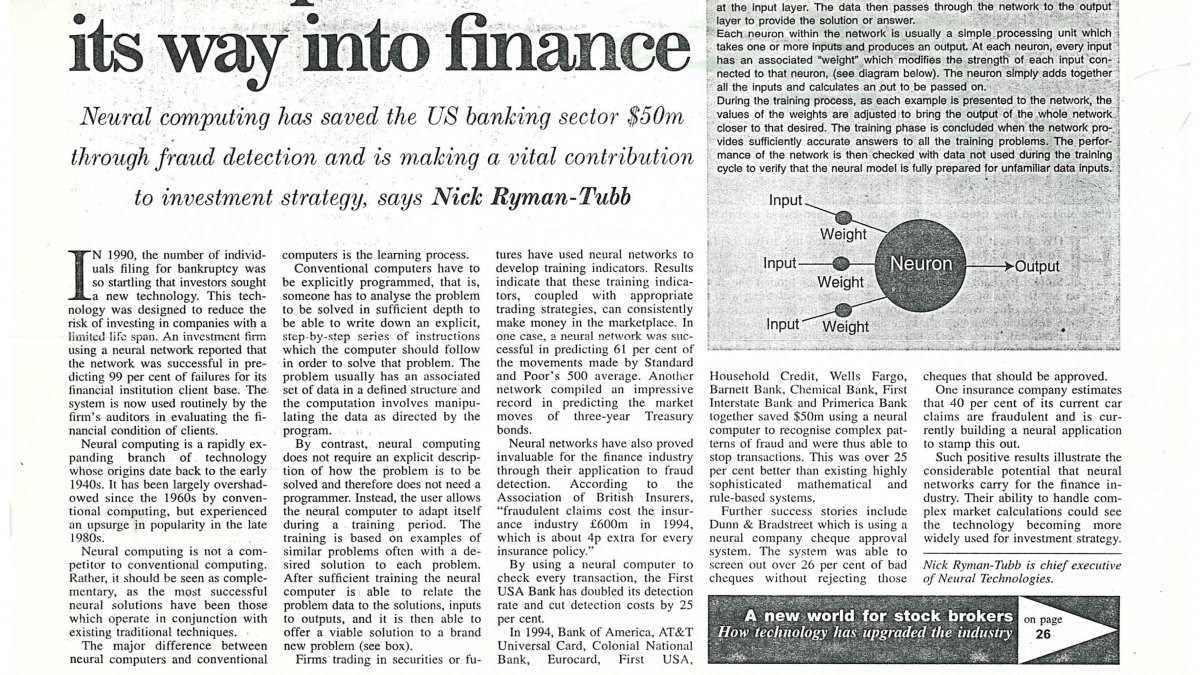
ResearchResearch interests
My research is focused on reducing and managing financial risk, fraud, anti-money laundering (AML) and all areas of cyber-crime. A neurocognitive approach with in context reasoning is proposed as a fundamental research area. I founded FITS as a not-for-profit “research institute” to tackle cyber-fraud, cyber-attacks and payment fraud:
- Using neural computing and adaptive techniques in the detection of emerging, real-time patterns in a high-volume transactional environment seeking to reconcile the symbolic and connectionist paradigms to move forward towards the next generation of intelligent systems.
- “Sea of Data” visualisation techniques to aid in the development of symbolic rules, models and “global” view.
- Automatic learning methodologies to create models for the detection of anomalous events and the automatic extraction of “rules” from models
- Inserting a neural network with fixed rules and parameters for known patterns and then automatically updating these during learning from on-line activities and presenting these changes to humans (emerging classes).
- Real-time deployment of complex neural hybrid systems in a mission-critical environment – including verification of models.
- Building timed events into a neural network architecture.
- Hybrid neural symbolic processing for the easy interpretation and knowledge extraction in real time, financial transaction environments, with robust fault-tolerant learning on in-complete or “noisy” data-feeds.
Research projects
Golden Rule - Understanding & Preventing Cyber-FraudInnovate (UK) Funded Project: £100k. Mat-17 to Apr-19
In collaboration with SnapOut Limited, This project is for development “Golden Rule” prototype software, using patented technology recently emerged from research, to explain patterns of cyber-fraud. With cyber-crime (including data breachs) fraud patterns are now rapidly changing and exisiting static detection and prevention approaches are starting to fail. Worldwide payment fraud that funds organised crime and terrorism had losses of $13.9bn & could grow to $40bn by 2020 despite all measures put in place. Recent research into neuro-symbolic processing has emerged that is able to explain abstract learnt patterns as English-like "golden" rules. Automatically explaining new and previously unknown cyber-crime fraud patterns leads to improved human understanding & better prevention; productivity is increased & the societal impact of fraud will be cut.
Research interests
My research is focused on reducing and managing financial risk, fraud, anti-money laundering (AML) and all areas of cyber-crime. A neurocognitive approach with in context reasoning is proposed as a fundamental research area. I founded FITS as a not-for-profit “research institute” to tackle cyber-fraud, cyber-attacks and payment fraud:
- Using neural computing and adaptive techniques in the detection of emerging, real-time patterns in a high-volume transactional environment seeking to reconcile the symbolic and connectionist paradigms to move forward towards the next generation of intelligent systems.
- “Sea of Data” visualisation techniques to aid in the development of symbolic rules, models and “global” view.
- Automatic learning methodologies to create models for the detection of anomalous events and the automatic extraction of “rules” from models
- Inserting a neural network with fixed rules and parameters for known patterns and then automatically updating these during learning from on-line activities and presenting these changes to humans (emerging classes).
- Real-time deployment of complex neural hybrid systems in a mission-critical environment – including verification of models.
- Building timed events into a neural network architecture.
- Hybrid neural symbolic processing for the easy interpretation and knowledge extraction in real time, financial transaction environments, with robust fault-tolerant learning on in-complete or “noisy” data-feeds.
Research projects
Innovate (UK) Funded Project: £100k. Mat-17 to Apr-19
In collaboration with SnapOut Limited, This project is for development “Golden Rule” prototype software, using patented technology recently emerged from research, to explain patterns of cyber-fraud. With cyber-crime (including data breachs) fraud patterns are now rapidly changing and exisiting static detection and prevention approaches are starting to fail. Worldwide payment fraud that funds organised crime and terrorism had losses of $13.9bn & could grow to $40bn by 2020 despite all measures put in place. Recent research into neuro-symbolic processing has emerged that is able to explain abstract learnt patterns as English-like "golden" rules. Automatically explaining new and previously unknown cyber-crime fraud patterns leads to improved human understanding & better prevention; productivity is increased & the societal impact of fraud will be cut.
Supervision
Postgraduate research supervision
MSc student dissertations in machine learning and data analytics. Please contact me if you are interested in working together.
Previous supervised student dissertations
- 2018, Andi Brajshori, Using Cost-Sensitive Artificial Neural Networks to Balance the Misclassification Costs for Fraud Detection through Threshold Moving
- 2018, Keerty Agarwal, Application of genetic algorithms for efficient field engineer scheduling: A case study for a leading energy and utilities provider in UK
- 2017, Giovanni Paolo Canuti, Deep Neural Network analysis of airlines taxi-out times at US airports.
Looking for students
I am looking to supervise PhD students; part time from industry very welcome.
Teaching
Teaching MSc Business Analytics and MSc Data Science and looking for amazing students to extend AI/ML research in the area of financial fraud.
Almost every business needs experts who can analyse their data making use of the latest machine learning and AI technologies. 80% of executives say this is creating jobs. Past students have joined companies such as Google, IBM, Tableau HSBC and British Gas based on these new skills. I will equip you with the skills necessary to kick-off an exciting career.
Only by combining data analytics with ML and AI can effective systems be created for problem-solving and making business decisions. In the US alone, there is a reported shortfall of 190,000 data analysts. In the UK, these roles are the highest job growth and attract some of the the highest salaries.
General enquiries: admissions@surrey.ac.uk, programme enquiries: n.ryman-tubb@surrey.ac.uk
Publications
Highlights
Cyber-fraud is lucrative to criminals, with a low risk of being convicted. There is a growing number of data breaches where criminals target legitimate computer systems to obtain large quantities of sensitive data that contain sufficient information so that they can then be used in payment card and other forms of bank fraud. My research over the last six years indicates that this situation will not change without changing the approach used to detect and manage fraud. My recent research focuses on the use of neural networks and AI to detect payment card fraud. I continue to work to bring a greater awareness of the issues and to promote strong research in collaboration with industry and the financial regulators. The level of fraud has been increasing since the introduction of payment cards and continues to increase. Our research has demonstrated that this is due to the nature of the complex data available, out-dated approaches to detection and management and the current economic and regulatory framework: providers see their losses through fraud as an acceptable cost to business. I believe that we can change that…
A set of payment card transactions including a sparse set of fraudulent transactions is normalized, such that continuously valued literals in each of the set of transactions are transformed to discrete literals. The normalized transactions are used to train a classifier, such as a neural network, such that the classifier is trained to classify transactions as fraudulent or genuine. The fraudulent transactions in the set of payment card transactions are clustered to form a set of prototype transactions. Each of the discrete literals in each of the prototype transactions is expanded using sensitivity analysis using the trained classifier as an oracle, and a rule for identifying fraudulent transactions is generated for each prototype transaction based on the transaction's respective expanded literals.
This paper presents a novel approach to knowledge extraction from large-scale datasets using a neural network when applied to the real-world problem of payment card fraud detection. Fraud is a serious and long term threat to a peaceful and democratic society. We present SOAR (Sparse Oracle-based Adaptive Rule) extraction, a practical approach to process large datasets and extract key generalizing rules that are comprehensible using a trained neural network as an oracle to locate key decision boundaries. Experimental results indicate a high level of rule comprehensibility with an acceptable level of accuracy can be achieved. The SOAR extraction outperformed the best decision tree induction method and produced over 10 times fewer rules aiding comprehensibility. Moreover, the extracted rules discovered fraud facts of key interest to industry fraud analysts.
In recent years there has been increased interest in developing computational and mathematical models of learning and adaptation. Computational Neuroscience for Advancing Artificial Intelligence: Models, Methods and Applications captures the latest research in this area, providing a learning theorists with a mathematically sound framework within which evaluate their models. The significance of this book lies in its theoretical advances, which are grounded in an understanding of computational and biological learning. The approach taken moves the entire field closer to a watershed moment of learning models, through the interaction of computer science, psychology and neurobiology.
Fraudulent use of credit cards has long been recognised as a major problem for the financial industry. Since the early 1990s, companies have collaborated to implement systems to combat this kind of fraud. Many of the systems employed various forms of advanced technology including rule-based systems, pre-set to recognise specific indicators in data. Initially, this strategy worked and, as more fraudsters were caught, fraud declined. While traditional fraud detection systems are in a mature state, neural computing is an exciting technology, based on proven principles, that is continuing to rapidly evolve.
Neural networks have represented a serious barrier-to-entry in their application in automated fraud detection due to their black box and often proprietary nature which is overcome here by combining them with symbolic rule extraction. A Sparse Oracle-based Adaptive Rule extraction algorithm is used to produce comprehensible rules from a neural network to aid the detection of credit card fraud. In this paper, a method to improve this extraction algorithm is presented along with results from a large real-world European credit card data set. Through this application it is shown that neural networks can assist in mission-critical areas of business and are an important tool in the transparent detection of fraud.
They all stink : food and drink, perfumery, household products, soaps, shampoos, paints, manufacturing processes, printing processes, waste products, contaminated air, and automotive emissions and environmental testing. In every case smell is a criterion of quality. Automated techniques to "smell" or "taste" liquids using mass spectroscopy and gas chromatography are time consuming, require skilled personnel and often do not give the information required for qualitative "tasting". A new technique is now available due to the advances in neural computing technology and multi-sensor array technology. The combination of these two approaches tries to simulate the human olfactory system in a simplified form. This paper shows that the recognition ability of an odour sensor array will be significantly improved using a neural computing approach in order to discriminate between similar odours.
The application of neural computing to help UK industry address some of their most pressing business needs has the potential ti improve its performance in a number of key areas, such as, business forecasting, marketing, quality control and innovative, world beating product development. The challenge to SERC (EPSRC) is to devise a strategy which will ensure that the foundational research work into the neural computing field continues while ensuring its eventual usability to industry.
A novel approach to knowledge extraction from neural network classifiers when applied to payment card fraud detection is proposed. Existing Fraud Management Systems (FMS) use neural network classifiers but do not have the ability to explain their learnt patterns of fraud. Rule extraction from such classifiers with a high level of abstraction and linguistic simplicity is proposed. Decompositional knowledge extraction methods are found to be too reliant on the architecture of the fraud classifer and current pedagogical rule extraction methods produce rules that are not sufficiently comprehensible. In this thesis the Sparse Oracle-based Adaptive Rule (SOAR) pedagogical extraction algorithm is proposed to extract generalising rules that explain patterns of fraud. SOAR uses sensitivity analysis to avoid the exhaustive searches of other pedagogical methods. By projecting into discretised space, polytopes are formed by SOAR covering the class convex hull of the classifier surface. A methodological and verifiable empirical evaluation on publicly available datasets in various domains is undertaken. These results show that SOAR extracts comprehensible rules that are sound from a deep learning neural network. When SOAR is applied to large datasets provided by payment card issuers it discovered new fraud types that were of key interest to payment risk/fraud analysts. SOAR provides an improved understanding of fraud vectors that will lead to a more secure payment process through informed payment fraud prevention steps and this work could therefore alter how fraud management is undertaken in the future.
Most mobile telecoms operators are aware that fraud is a costly business. A single handset, for example, used for two international conference calls at US$2 per minute, could generate losses of around US$8,000 in just one day, increasing to $57,000 for a week. In one recorded incident, over $800.000 was lost to fraud using just five handsets over a weekend.Despite the many measures already being taken to combat fraud, it is on the increase. Newer operators are especially vulnerable 'due to the requirement (often by shareholders) to gain new customers rapidly, leaving little time for careful credit management and customer screening. A novel neural network approach is described that learns to detect patterns of potential fraud. Early criticisms, relating to the lack of explanatory information on how a neural computer performs its task, have now been overcome. Techniques can be used to identify which input variables have the largest effect on a particular decision or prediction. Furthermore, neural computers can now be structured to incorporate prior expert knowledge and present results in a form that is meaningful to human users. In the fraud detection application, neural 'models' are trained to perform anomaly detection, i.e. detection of unusual calls based on previously observed behaviour.
The briefing was introduced by Ray Browne, Project Manager at DTI, who announced a set of neural computing (NC) awareness products. In the spirit of the campaign, the awareness product set is designed to provide UK companies with comprehensive ‘hands on’information and advice needed to evaluate how to take commercial advantage of the technology. The set was developed by Touche Ross with additional expertise provided by NC suppliers and is geared towards both technicians and the business manager.
The mining industry is increasingly using neural computing to maximise mineral recovery and to ensure that the cost of production never exceeds the revenue generated by sales of raw materials. This article describes how these systems work and presents a case study where NTL worked with Australian mining company, Straits Resources Ltd. to improve efficiency at their Girilambone copper mine in New South Wales. (Abstract quotes from original text). Gone are the days of computers which could only do what they were told by humans. The neural computer has changed all that. Neural means "of the nervous systems", and the biological comparison is no coincidence, Just like the human brain, neural computers can learn from experience and apply their knowledge...such a system has great potential in the mineral extraction industries,
The rules that govern many of the processes within the manufacturing industry can be hard to define and even harder to write down. Nick Ryman-Tubb explains how neural network technology can help.
Intelligent computing technologies promise to be a powerful weapon in the global battle against telecoms fraud. It is a sad fact that organized crime has realized that there is easy money to be made in telecommunications. Existing fraud management systems have provided only limited protection against fraud and are unable to curb this growth. Neural computers are an example of an alternative approach to tackling some of the fraud problems that other computing tools have difficulty coping with. They do not replace conventional techniques but they augment them. Inspired by the biological processes of the human brain, neural computing has many human-like qualities. Since a neural computer learns, it does not need to be programmed with fixed rules or equations. It provides a radical new way of solving complex problems.
They all stink : food and drink, perfumery, household products, soaps, shampoos, paints, manufacturing processes, printing processes, waste products, contaminated air, and automotive emissions and environmental testing. In every case smell is a criterion of quality. Automated techniques to "smell" or "taste" liquids using mass spectroscopy and gas chromatography are time consuming, require skilled personnel and often do not give the information required for qualitative "tasting". A new technique is now available due to the advances in neural computing technology and multi-sensor array technology. The combination of these two approaches tries to simulate the human olfactory system in a simplified form. This paper shows that the recognition ability of an odour sensor array will be significantly improved using a neural computing approach in order to discriminate between similar odours.
Has your customer data got millions of records, each carrying multiple data fields.
A method of locating a mineral deposit, the method comprising the steps of: (i) obtaining a plurality of data sets of indicators of physical or chemical conditions at a plurality of sites in an area known to contain the mineral, at least one of the indicators being an indicator of the mineral; (ii) training neural anomaly identifying means to identify anomalies in the data sets; (iii) obtaining a plurality of the indicators at a plurality of sites in a search area thought to contain the mineral; (iv) inputting the indicators obtained from the search area to the trained anomaly identifying means to obtain an indication of the location of the mineral deposit; and (v) visualising the indication.
Neural networks have represented a serious barrier-to-entry in their application in automated fraud detection due to their black box and often proprietary nature which is overcome here by combining them with symbolic rule extraction. A Sparse Oracle-based Adaptive Rule extraction algorithm is used to produce comprehensible rules from a neural network to aid the detection of credit card fraud. In this paper, a method to improve this extraction algorithm is presented along with results from a large real-world European credit card data set. Through this application it is shown that neural networks can assist in mission-critical areas of business and are an important tool in the transparent detection of fraud.
The core goal of this paper is to identify guidance on how the research community can better transition their research into payment card fraud detection towards a transformation away from the current unacceptable levels of payment card fraud. Payment card fraud is a serious and long-term threat to society (Ryman-Tubb and d’Avila Garcez, 2010) with an economic impact forecast to be $416bn in 2017 (see Appendix A).1 The proceeds of this fraud are known to finance terrorism, arms and drug crime. Until recently the patterns of fraud (fraud vectors) have slowly evolved and the criminals modus operandi (MO) has remained unsophisticated. Disruptive technologies such as smartphones, mobile payments, cloud computing and contactless payments have emerged almost simultaneously with large-scale data breaches. This has led to a growth in new fraud vectors, so that the existing methods for detection are becoming less effective. This in turn makes further research in this domain important. In this context, a timely survey of published methods for payment card fraud detection is presented with the focus on methods that use AI and machine learning. The purpose of the survey is to consistently benchmark payment card fraud detection methods for industry using transactional volumes in 2017. This benchmark will show that only eight methods have a practical performance to be deployed in industry despite the body of research. The key challenges in the application of artificial intelligence and machine learning to fraud detection are discerned. Future directions are discussed and it is suggested that a cognitive computing approach is a promising research direction while encouraging industry data philanthropy.