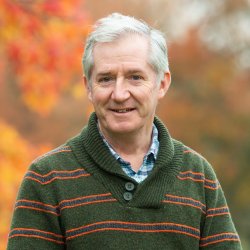
Professor Paul Krause
About
Biography
Background
I graduated in 1977 with First Class Honours in Pure Mathematics and Physics from the University of Exeter. Following that I studied for a PhD in Geomagnetism under Geraint Rosser at the same university.
During my career I have worked at three internationally leading independent research laboratories:
- The National Physical Laboratory (1980-87);
- The Imperial Cancer Research Fund (1989-1996);
- Philips Research Laboratories, UK (1996-2003).
I greatly enjoyed my seven years at the National Physical Laboratory, working with some dedicated and highly talented scientists. At the end of those seven years I had achieved an order of magnitude improvement of the repeatability of the josephson voltage standard, with also a significantly improved ease of use and reduction in liquid helium consumption when performing the measurements. However, although quantum metrology is a fascinating area, I could not see any potential for significant advances in the underlying physics, so I went for a change.
Theory and practice of artificial intelligence
I started by working for two years at the University of Surrey within their Formal Methods of Software Engineering Group. What I actually did was more in the line of automated theorem proving, spending my time working with Ron Knott on using Prolog to automatically generate behaviours implied by system specifications written in set theory and formal logic. Specification verification tools are way ahead if that now, but it was a fun introduction to the challenges of building automated reasoning systems. Incidentally, Ron is still maintaining the longest running website on recreational mathematics.
I moved on to the Imperial Cancer Research Fund (now Cancer Research UK) in 1989 to work on automated decision making and diagnosis for health care. We were typically looking at non-classical logics and symbolic reasoning in order to make the "arguments" supporting candidate decisions explicit. This lead to some of the earliest published work on computational models of argumentation; an area of research that has grown significantly since those days. Our own work defined a category theoretic semantics for our model of argumentation. We had very extensive collaborations across the research community in models of decision making and reasoning under uncertainty, and my introductory (and very successful at the time) text book with Dominic Clark on Representing Uncertainty, an AI Approach spun out of that.
After seven years at ICRF it was, of course, time to move on again. I took up a post at Philips Research Laboratories, Redhill, to work as a Principal Scientist within Paul Gough's Software Engineering and Applications research group. Sounds a bit like a change of topic, but was not in fact. Machine automation and reasoning were still key here: "robots" to automate the testing of consumer products; semantic reasoning to automatically generate links between entities in hierarchies of documents; bayesian models for software quality assessment. The last of these initiated a long standing collaboration between myself, and Norman Fenton and Martin Neil at City University and Agena Ltd.
2001 saw me moving to a joint appointment with Philips and the University of Surrey, with the aim of strengthening collaboration on computer science research between the two organisations. Sadly, the end of 2001 saw the beginning of the end of Philips Research's once world class research laboratory at Redhill. Promotion to Senior Principal Scientist was a short lived pleasure, but a climax to a wonderful time at Philips. As the research laboratory was gradually wound down, so I progressively moved to full time at the University of Surrey from the beginning of 2003.
Of course, a big difference between industry-focused research laboratories and an academic department is that in academia one is not constrained to work within defined remits of a specific industry sector (although both NPL and Philips Research at their best did indeed support a level of blue sky research). So the move to academia did of course give me more freedom in my trajectory. Despite the recent high-profile successes in AI, I believe there are some major challenges. Two key ones for me are: explainability/accountability of AI supported decision making; and, bias.
Addressing the former issue has been a major influencer of the approaches I have taken. Going back to our work on toxicological risk prediction with Lhasa Ltd, for the pharmaceutical industry an ability to classify novel chemical compounds according to potential risk classes had little value unless the reasons ("arguments") for those classifications were made explicit to the analysts. Moving on to more recent work, my work with Nick Ryman-Tubb during his PhD and subsequently is showing that 20 years' research on fraud detection in academia has not resulted in any significant advances when assessed according to industrially relevant metrics [Ryman-Tubb, Krause and Garn, 2018]. We have also shown <<citation pending>> that unless there are significant changes to the regulatory environment, the financial sector will simply continue to consider the current level of fraud as an acceptable cost of business - with no account being taken that fraud is in effect a multi-billion dollar industry that is funding terrorism and organised crime. The research challenge is to build AI/data analytic tools that help us gain understanding of the fraud vectors themselves, and also explicate the real impact of this level of crime to help facilitate change to the status quo.
The issue of bias is pernicious in AI. The problem here is that, obviously, the models are only as good as the data they are trained on. Never mind the irritation of an e-commerce recommending you products that are way off topic for your specific personality. More critical is the potential risk for a health care advisor to judge a health care scenario on the basis of a data set that has been obtained from a predominantly middle class western population. Within that population there will be ethnic groups that will have had little representation within the training sets. Even worse, there may be pressure to apply such a model on a global basis to populations that have absolutely no representation in the training of the model.
This is a real challenge in academic research as it is so hard to spend the length of time needed to collate, gain regulatory approval and quality check high quality data on a global basis. However, we are making progress on this and for the last two or three years I have been supporting Lilian Tang's work on diabetic retinopathy in collating extensive retinal image sets from disparate regions across the globe [Lutfiah Al Turk, Paul Krause, Su Wang, Hend Alsawadi, Abdulrahman Zaid Alshamrani, Tunde Peto, Andrew Bastawrous, and Hongying Lilian Tang, Automated Progression Analysis Across Three Nations, to be submitted].
Deep Learning has had a lot of successes. There is so much hype around it that it almost seems that the public eye is equating deep learning with AI. But simply thinking that throwing massive data sets at smart algorithms will generate knowledge is a big mistake. Our group's work on diabetic retinopathy, the projects mentioned above, and others, support the conviction that we must still support human-machine co-creation of knowledge. Big data sets, and smart algorithms are necessary, but they are not sufficient for the generation of machines that demonstrate "intelligent" problem solving. Now, working collectively within the NICE group in the Department of Computer Science here at Surrey, we are building out a suite of capabilities that will continue to help us build machines that can communicate and collaborate with human beings to solve complex problems. Consider, for example, the urgent need to gain deeper understanding of the links between biodiversity and the provision of ecosystem services. With Alireza Tamaddoni Nezhad, we have capability for mining ecological networks using Inductive Logic Programming and Meta-heuristic reasoning. Through Sotiris Moschoyiannis, we have an emerging toolset for the identification of the system levers or “drivers” which have high influence on the overall system behaviour. Yunpeng Li now strengthens our Bayesian analytic capability; still a powerful method for ensuring assumptions are correctly captured when reasoning with complex data sets. André Grüning gives further support on techniques for driving dynamical systems into preferred states, perhaps using reinforcement learning to identify interventions to transition a degraded ecological network into one offering enhanced functionality and resilience.
I fundamentally do not believe we can learn how to maintain a human presence in the stunningly beautiful biosphere that we are blessed with, without a richer suite of tools from AI -- and ones with which we can co-create knowledge and understanding. We won't survive on this world without them. But, of course, the world may well survive without us...
Areas of specialism
Publications
This study evaluated the effectiveness and usability of a developed collaborative online tool (chit-chat) for children with Attention Deficit Hyperactivity Disorder (ADHD). We studied whether this tool influenced children’s Knowledge and experience exchange, motivation, behavioral abilities and social skills while using another learning tool, ACTIVATE. A total of seven Saudi children with ADHD aged from 6 to 8 years were assigned to the collaborative intervention using iPads. They were asked to play mini games that positively affect children with ADHD cognitively and behaviorally, then chat using our developed collaborative online tool, for three sessions. Progress points were measured and quantitatively analyzed before and after the intervention, thematic analysis was applied on the qualitative data. Participants showed improvements in overall performance when using the learning tool ACTIVATE. E-collaboration was found to be effective to children with ADHD and positively influencing their knowledge, experience, motivation and social skills.
Attention Deficit Hyperactivity Disorder (ADHD) is a set of behavioural characteristics disorder, such as inattentiveness, hyperactivity and/or impulsiveness. It can affect people with different intelligent abilities, and it may affect their academic performance, social skills and generally, their lives. Usually, symptoms are not clearly recognized until the child enters school, most cases are identified between the ages 6 to 12. In the kingdom of Saudi Arabia (KSA), ADHD is a widely spread disorder among young children. Usually, they suffer from distraction and lack of focus, and hyperactivity, which reduce their academic achievements. As technology have been used in classrooms to facilitate the information delivery for students, and to make learning fun; some of these technologies have actually been applied in many schools in KSA with normal students, but unfortunately no studies were reported by the time of writing this paper. Specifically, there are no studies done for using any type of technology to help Saudi students with ADHD reaching up their peers academically. Because of that, our focus in this study is to investigate the effect of using technology, particularly e-games, to improve Saudi children with ADHD cognitively, behaviourally and socially. As well as evaluating the interaction between those children with the game interface. Thus, the investigation done through exploring the interaction of web-based games that runs on Tablets. The respondents are 17 ADHD children aged from 6-12 in classroom settings. The study involves focussing on interface of the games stimulate different executive functions in the brain, which is responsible for the most important cognitive capacities, such as: Sustained Attention, Working Memory, and Speed of Processing. Ethnographic method of research was used, which involved observing students' behaviour in classroom, to gather information and feedback about their interaction with the application. National Institutes of Health (NIH) tests were used in pre- and post-intervention to measure improvements in attention, processing speed and working memory. Students' test scores of main school subjects were taken pre- and post-intervention to measure enhancement in academic performance. Results show that using the application significantly improve cognitive capacities for participants, which affected their academic grades in Math, English and Science, as well as its positive influence on their behaviour. In addition, the application's interface was found easy to use and subjectively pleasing. As a conclusion, the application considered effective and usable.
The Translational Research and Patients safety in Europe (TRANSFoRm) project aims to integrate primary care with clinical research whilst improving patient safety. The TRANSFoRm International Research Readiness survey (TIRRE) aims to demonstrate data use through two linked data studies and by identifying clinical data repositories and genetic databases or disease registries prepared to participate in linked research. The TIRRE survey collects data at micro-, meso- and macro-levels of granularity; to fulfil data, study specific, business, geographical and readiness requirements of potential data providers for the TRANSFoRm demonstration studies. We used descriptive statistics to differentiate between demonstration-study compliant and non-compliant repositories. We only included surveys with >70% of questions answered in our final analysis, reporting the odds ratio (OR) of positive responses associated with a demonstration-study compliant data provider. We contacted 531 organisations within the Eurpean Union (EU). Two declined to supply information; 56 made a valid response and a further 26 made a partial response. Of the 56 valid responses, 29 were databases of primary care data, 12 were genetic databases and 15 were cancer registries. The demonstration compliant primary care sites made 2098 positive responses compared with 268 in non-use-case compliant data sources [OR: 4.59, 95% confidence interval (CI): 3.93-5.35, p < 0.008]; for genetic databases: 380:44 (OR: 6.13, 95% CI: 4.25-8.85, p < 0.008) and cancer registries: 553:44 (OR: 5.87, 95% CI: 4.13-8.34, p < 0.008). TIRRE comprehensively assesses the preparedness of data repositories to participate in specific research projects. Multiple contacts about hypothetical participation in research identified few potential sites.
The allure of interoperable systems is that they should improve patient safety and make health services more efficient. The UK's National Programme for IT has made great strides in achieving interoperability; through linkage to a national electronic spine. However, there has been criticism of the usability of the applications in the clinical environment.
Cloud computing provides the opportunity to migrate virtual machines to “follow-the-green” data centres. That is, to migrate virtual machines between green data centres on the basis of clean energy availability, to mitigate the environmental impact of carbon footprint emissions and energy consumption. The virtual machine migration problem can be modelled to maximize the utility of computing resources or minimizing the cost of using computing resources. However, this would ignore the network energy consumption and its impact on the overall CO2 emissions. Unless this is taken into account the extra data traffic due to migration of data could then cause an increase in brown energy consumption and eventually lead to an unintended increase in carbon footprint emissions. Energy consumption is a key aspect in deploying distributed service in cloud networks within decentralized service delivery architectures. In this paper, the authors address an optimiza- tion view of the problem of locating a set of cloud services on a set of sites green data centres managed by a service provider or hybrid cloud computing brokerage. The authors’ goal is to minimize the overall network energy consumption and carbon footprint emissions for accessing the cloud services for any pair of data centres i and j. The authors propose an optimization migration model based on the development of integer linear programming (ILP) models, to identify the leverage of green energy sources with data centres and the energy consumption of migrating VMs.
In the Internet of Things (IoT) + Fog + Cloud architecture, with the unprecedented growth of IoT devices, one of the challenging issues that needs to be tackled is to allocate Fog service providers (FSPs) to IoT devices, especially in a game-theoretic environment. Here, the issue of allocation of FSPs to the IoT devices is sifted with game-theoretic idea so that utility maximizing agents may be benign. In this scenario, we have multiple IoT devices and multiple FSPs, and the IoT devices give preference ordering over the subset of FSPs. Given such a scenario, the goal is to allocate at most one FSP to each of the IoT devices. We propose mechanisms based on the theory of mechanism design without money to allocate FSPs to the IoT devices. The proposed mechanisms have been designed in a flexible manner to address the long and short duration access of the FSPs to the IoT devices. For analytical results, we have proved the economic robustness, and probabilistic analyses have been carried out for allocation of IoT devices to the FSPs. In simulation, mechanism efficiency is laid out under different scenarios with an implementation in Python.
We propose a framework that can be used to produce functioning web applications from SBVR models. To achieve this, we begin by discussing the concept of declarative application generation and examining the commonalities between SBVR and the RESTful architectural style of the web. We then show how a relational database schema and RESTful interface can be generated from an SBVR model. In this context, we discuss how SBVR can be used to semantically describe hypermedia on the Web and enhance its evolvability and loose coupling properties. Finally, we show that this system is capable of exhibiting process-like behaviour without requiring explicitly defined processes.
In this paper we are concerned with providing support for business activities in moving from value chains to value networks. We describe a fully distributed P2P architecture which reflects the dynamics of business processes that are not governed by a single organisation. The temporary virtual networks of long-term business transactions are used as the building block of the overall scale-free business network. The design is based on dynamically formed permanent clusters resulting in a topology that is highly resilient to failures (and attacks) and is capable of reconfiguring itself to adapt to changes in business models and respond to global failures of conceptual hubs. This fosters an environment where business communities can evolve to meet emerging business opportunities and achieve sustainable growth within a digital ecosystem.
In the IoT+Fog+Cloud architecture, with the ever increasing growth of IoT devices, allocation of IoT devices to the Fog service providers will be challenging and needs to be addressed properly in both the strategic and non-strategic settings. In this paper, we have addressed this allocation problem in strategic settings. The framework is developed under the consideration that the IoT devices (e.g. wearable devices) collecting data (e.g. health statistics) are deploying it to the Fog service providers for some meaningful processing free of cost. Truthful and Pareto optimal mechanisms are developed for this framework and are validated with some simulations.
In this paper we explore the concept of ldquoecosystemrdquo as a metaphor in the development of the digital economy. We argue that the modelling of social ecosystems as self-organising systems is also relevant to the study of digital ecosystems. Specifically, that centralised control structures in digital ecosystems militate against emergence of innovation and adaptive response to pressures or shocks that may impact the ecosystem. We hope the paper will stimulate a more holistic approach to gaining empirical and theoretical understanding of digital ecosystems.
Recent years have witnessed a rapid rise in the development of deterministic and non-deterministic models to estimate human impacts on the environment. An important failing of these models is the difficulty that most people have understanding the results generated by them, the implications to their way of life and also that of future generations. Within the field, the measurement of greenhouse gas emissions (GHG) is one such result. The research described in this paper evaluates the potential of Bayesian Network (BN) models for the task of managing GHG emissions in the British agricultural sector. Case study farms typifying the British agricultural sector were inputted into both, the BN model and CALM, a Carbon accounting tool used by the Country Land and Business Association (CLA) in the UK for the same purpose. Preliminary results show that the BN model provides a better understanding of how the tasks carried out on a farm impact the environment through the generation of GHG emissions. This understanding is achieved by translating the emissions information into their cost in monetary terms using the Shadow Price of Carbon (SPC), something that is not possible using the CALM tool. In this manner, the farming sector should be more inclined to deploy measures for reducing its impact. At the same time, the output of the analysis can be used to generate a business plan that will not have a negative effect on a farm's capital income.
We present a Peer-to-Peer network design which aims to support business activities conducted through a network of collaborations that generate value in different, mutually beneficial, ways for the participating organisations. The temporary virtual networks formed by long-term business transactions that involve the execution of multiple services from different providers are used as the building block of the underlying scale-free business network. We show how these local interactions, which are not governed by a single organisation, give rise to a fully distributed P2P architecture that reflects the dynamics of business activities. The design is based on dynamically formed permanent clusters of nodes, the so-called Virtual Super Peers (VSPs), and this results in a topology that is highly resilient to certain types of failure (and attacks). Furthermore, the proposed P2P architecture is capable of reconfiguring itself to adapt to the usage that is being made of it and respond to global failures of conceptual hubs. This fosters an environment where business communities can evolve to meet emerging business opportunities and achieve sustainable growth within a digital ecosystem.
With REST becoming the dominant architectural paradigm for web services in distributed systems, more and more use cases are applied to it, including use cases that require transactional guarantees. We propose a RESTful transaction model that satisfies both the constraints of transactions and those of the REST architectural style. We then apply the isolation theorems to prove the robustness of its properties on a formal level.
We are seeing a steady build up in momentum of two trends that will lead to a significant, and necessary, transformation of agriculture in the 21st Century. Firstly, the move to digital with IoT facilitating the use of sensor networks to support precise decision making. Secondly, the move to "ecological intensification"; working with natural processes to lower the carbon footprint of agricultural processes and increase the biodiversity of agricultural units without sacrificing yield. Clearly data mining and machine learning have an important role to play in supporting this transformation. However, given the range of biotic, abiotic and social contexts that need to inform the development of models for decision support in agriculture, we need data mining techniques that support the use of qualitative and unstructured data as well as hard numerical data. In this tutorial we show how Bayesian Networks can be built from a wide range of sources of expert knowledge and data. Whist we use digital agriculture as a key beneficiary of these techniques, this tutorial will also be of interest to all those with an interest in building IoT systems that require combinations of social, technical and environmental understanding.
Concurrency control mechanisms such as turn-taking, locking, serialization, transactional locking mechanism, and operational transformation try to provide data consistency when concurrent activities are permitted in a reactive system. Locks are typically used in transactional models for assurance of data consistency and integrity in a concurrent environment. In addition, recovery management is used to preserve atomicity and durability in transaction models. Unfortunately, conventional lock mechanisms severely (and intentionally) limit concurrency in a transactional environment. Such lock mechanisms also limit recovery capabilities. Finally, existing recovery mechanisms themselves afford a considerable overhead to concurrency. This paper describes a new transaction model that supports release of early results inside and outside of a transaction, decreasing the severe limitations of conventional lock mechanisms, yet still warranties consistency and recoverability of released resources (results). This is achieved through use of a more flexible locking mechanism and by using two types of consistency graph. This provides an integrated solution for transaction management, recovery management and concurrency control. We argue that these are necessary features for management of long-term transactions within "digital ecosystems" of small to medium enterprises.
This conference addresses the question of embodiment, in a digital-era context, and how bodies can be computed; how they can be constituted via algorithmic or other such formal processes. The focus of the conference is on practices of embodiment and computation in a number of key cultural practices, most notably: computer animation, interaction design, and live performance (digital dance-theatre).
We describe a translation of scenarios given in UML 2.0 sequence diagrams into a tuples-based behavioural model that considers multiple access points for a participating instance and exhibits true-concurrency. This is important in a component setting since different access points are connected to different instances, which have no knowledge of each other. Interactions specified in a scenario are modelled using tuples of sequences, one sequence for each access point. The proposed unfolding of the sequence diagram involves mapping each location (graphical position) onto the so-called component vectors. The various modes of interaction (sequential, alternative, concurrent) manifest themselves in the order structure of the resulting set of component vectors, which captures the dependencies between participating instances. In previous work, we have described how (sets of) vectors generate concurrent automata. The extension to our model with sequence diagrams in this paper provides a way to verify the diagram against the state-based model.
The fast development of Internet of Things (IoT) computing and technologies has prompted a decentralization of Cloud-based systems. Indeed, sending all the information from IoT devices directly to the Cloud is not a feasible option for many applications with demanding requirements on real-time response, low latency, energy-aware processing and security. Such decentralization has led in a few years to the proliferation of new computing layers between Cloud and IoT, known as Edge computing layer, which comprises of small computing devices (e.g. Raspberry Pi) to larger computing nodes such as Gateways, Road Side Units, Mini Clouds, MEC Servers, Fog nodes, etc. In this paper, we study the challenges of processing an IoT data stream in an Edge computing layer. By using a real life data stream set arising from a car data stream as well as a real infrastructure using Raspberry Pi and Node-Red server, we highlight the complexities of achieving real time requirements of applications based on IoT stream processing.
Web service is an emerging paradigm for distributed computing. In order to verify web services rigorously, it is important to provide a formal semantics for flow-based web service languages such as BPEL. A suitable formal model should cover most features of BPEL. The existing formal models either abstract from data, cover a simple subset of BPEL, or omit the interactions between BPEL activities. This paper presents Web Service Automata, an extension of Mealy machines, to fulfil the formal model requirements of the web service domain. Secondly, the paper analyses the control handling and data handling of BPEL, so that these can be verified in a clear manner.
The aim of this paper is to facilitate e-business transactions between small and medium enterprises (SMEs), in a way that respects their local autonomy, within a digital ecosystem. For this purpose, we distinguish transactions from services (and service providers) by considering virtual private transaction networks (VPTNs) and virtual service networks (VSNs). These two virtual levels are optimised individually and in respect to each other. The effect of one on the other, can supply us with stability, failure resistance and small-world characteristics on one hand and durability, consistency and sustainability on the other hand. The proposed network design has a dynamic topology that adapts itself to changes in business models and availability of SMEs, and reflects the highly dynamic nature of a digital ecosystem.
In this paper, we present a Business Analytics (BA) framework, which addresses the challenge of analysing primary care outcomes for both patients and clinicians from multiple data sources in an accurate manner. A review of the process monitoring literature has been conducted in the context of healthcare management and decision making and its findings have informed the formulation of a BA conceptual framework for process monitoring and decision support in primary care. Furthermore, a real case study is conducted to demonstrate the application of the BA framework to implement a BA dashboard tool within one of the largest primary care providers in England. Findings: The main contributions of the presented work are the development of a conceptual BA framework and a BA dashboard tool to support management and decision making in primary care. This was evaluated through a case study of the implementation of the BA dashboard tool in London's largest primary care provider. This BA tool provides real-time information to enable simpler decision-making processes and to inform business transformation in a number of areas. The resulting increased efficiency has led to significant cost savings and improved delivery of patient care.
The ability to correctly detect the location and derive the contextual information where a concept begins to drift is essential in the study of domains with changing context. This paper proposes a Top-down learning method with the incorporation of a learning accuracy mechanism to efficiently detect and manage context changes within a large dataset. With the utilisation of simple search operators to perform convergent search and JBNC with a graphical viewer to derive context information, the identified hidden context are shown with the location of the disjoint points, the contextual attributes that contribute to the concept drift, the graphical output of the true relationships between these attributes and the Boolean characterisation which is the context.
In this paper we present a prototype of a tool that demonstrates how existing limitations in ensuring an agent’s compliance to an argumentation-based dialogue protocol can be overcome. We also present the implementation of compliance enforcement components for a deliberation dialogue protocol, and an application that enables two human participants to engage in an efficiently moderated dialogue, where all inappropriate utterances attempted by an agent are blocked and prevented from inclusion within the dialogue.
To compare supervised transfer learning to semisupervised learning for their ability to learn in-depth knowledge with limited data in the optical coherence tomography (OCT) domain. Transfer learning with EfficientNet-B4 and semisupervised learning with SimCLR are used in this work. The largest public OCT dataset, consisting of 108,312 images and four categories (choroidal neovascularization, diabetic macular edema, drusen, and normal) is used. In addition, two smaller datasets are constructed, containing 31,200 images for the limited version and 4000 for the mini version of the dataset. To illustrate the effectiveness of the developed models, local interpretable model-agnostic explanations and class activation maps are used as explainability techniques. The proposed transfer learning approach using the EfficientNet-B4 model trained on the limited dataset achieves an accuracy of 0.976 (95% confidence interval [CI], 0.963, 0.983), sensitivity of 0.973 and specificity of 0.991. The semisupervised based solution with SimCLR using 10% labeled data and the limited dataset performs with an accuracy of 0.946 (95% CI, 0.932, 0.960), sensitivity of 0.941, and specificity of 0.983. Semisupervised learning has a huge potential for datasets that contain both labeled and unlabeled inputs, generally, with a significantly smaller number of labeled samples. The semisupervised based solution provided with merely 10% labeled data achieves very similar performance to the supervised transfer learning that uses 100% labeled samples. Semisupervised learning enables building performant models while requiring less expertise effort and time by using to good advantage the abundant amount of available unlabeled data along with the labeled samples.
Purpose: The aim of this work is to demonstrate how a retinal image analysis system, DAPHNE, supports the optimization of diabetic retinopathy (DR) screening programs for grading color fundus photography. Method: Retinal image sets, graded by trained and certified human graders, were acquired from Saudi Arabia, China, and Kenya. Each image was subsequently analyzed by the DAPHNE automated software. The sensitivity, specificity, and positive and negative predictive values for the detection of referable DR or diabetic macular edema were evaluated, taking human grading or clinical assessment outcomes to be the gold standard. The automated software’s ability to identify co-pathology and to correctly label DR lesions was also assessed. Results: In all three datasets the agreement between the automated software and human grading was between 0.84 to 0.88. Sensitivity did not vary significantly between populations (94.28%–97.1%) with specificity ranging between 90.33% to 92.12%. There were excellent negative predictive values above 93% in all image sets. The software was able to monitor DR progression between baseline and follow-up images with the changes visualized. No cases of proliferative DR or DME were missed in the referable recommendations. Conclusions: The DAPHNE automated software demonstrated its ability not only to grade images but also to reliably monitor and visualize progression. Therefore it has the potential to assist timely image analysis in patients with diabetes in varied populations and also help to discover subtle signs of sight-threatening disease onset. Translational Relevance: This article takes research on machine vision and evaluates its readiness for clinical use.
Attention Deficit Hyperactivity Disorder (ADHD) is a set of behavioural characteristics disorder, such as inattentiveness, hyperactivity and/or impulsiveness. It can affect people with different intelligent abilities, and it may affect their academic performance, social skills and generally, their lives. Usually, symptoms are not clearly recognized until the child enters school, most cases are identified between the ages 6 to 12. In the kingdom of Saudi Arabia (KSA), ADHD is a widely spread disorder among young children. Usually, they suffer from distraction and lack of focus, and hyperactivity, which reduce their academic achievements. As technology have been used in classrooms to facilitate the information delivery for students, and to make learning fun; some of these technologies have actually been applied in many schools in KSA with normal students, but unfortunately no studies were reported by the time of writing this paper. Specifically, there are no studies done for using any type of technology to help Saudi students with ADHD reaching up their peers academically. Because of that, our focus in this study is to investigate the effect of using technology, particularly e-games, to improve Saudi children with ADHD cognitively, behaviourally and socially. As well as evaluating the interaction between those children with the game interface. Thus, the investigation done through exploring the interaction of web-based games that runs on Tablets. The respondents are 17 ADHD children aged from 6–12 in classroom settings. The study involves focussing on interface of the games stimulate different executive functions in the brain, which is responsible for the most important cognitive capacities, such as: Sustained Attention, Working Memory, and Speed of Processing. Ethnographic method of research was used, which involved observing students’ behaviour in classroom, to gather information and feedback about their interaction with the application. National Institutes of Health (NIH) tests were used in pre- and post- intervention to measure improvements in attention, processing speed and working memory. Students’ test scores of main school subjects were taken pre- and post-intervention to measure enhancement in academic performance. Results show that using the application significantly improve cognitive capacities for participants, which affected their academic grades in Math, English and Science, as well as its positive influence on their behaviour. In addition, the application’s interface was found easy to use and subjectively pleasing. As a conclusion, the application considered effective and usable.
In this paper we present a model for coordinating distributed long running and multi-service transactions in Digital Business EcoSystems. The model supports various forms of service composition, which are translated into a tuples-based behavioural description that allows to reason about the required behaviour in terms of ordering, dependencies and alternative execution. The compensation mechanism warranties consistency, including omitted results, without breaking local autonomy. The proposed model is considered at the deployment level of SOA, rather than the realisation level, and is targeted to business transactions between collaborating SMEs as it respects the loose-coupling of the underlying services. © 2007 IEEE.
In the IoT+Fog+Cloud architecture, with the ever increasing growth of IoT devices, allocation of IoT devices to the Fog service providers will be challenging and needs to be addressed properly in both the strategic and non-strategic settings. In this paper, we have addressed this allocation problem in strategic settings. The framework is developed under the consideration that the IoT devices (e.g. wearable devices) collecting data (e.g. health statistics) are deploying it to the Fog service providers for some meaningful processing free of cost. Truthful and Pareto optimal mechanisms are developed for this framework and are validated with some simulations.
In this final chapter, we outline a vision for a technological revolution in agriculture that would work to regain a sense of balance between food production and natural ecosystems. We promote an ecological engineering approach to crop production that draws on experience from the organic and conservation agriculture movements. However, we expand on this by promoting in addition: An Internet of Things enabled biomonitoring system that enables key (above and below ground) environmental indicators to be automatically monitored across an agricultural unit.A combination of network and thermodynamic ecosystem modelling approaches to enable a deep understanding of the response of ecosystem service functioning to changes in biodiversity, and the abiotic context, in any given agroecosystem. We also call for an explicit recognition of the “ethnosphere” (the sphere of human social and cultural experience) as a fifth geosphere which emerged from the biosphere, and the other three geospheres, and whose continued existence is therefore contingent on the health and stability of the other four geospheres.
Monitoring the activities of daily living of the elderly at home is widely recognized as useful for the detection of new or deteriorating health conditions. However, the accuracy of existing indoor location tracking systems remains unsatisfactory. The aim of this study was, therefore, to develop a localization system that can identify a patient's real-time location in a home environment with maximum estimation error of 2 m at a 95% confidence level. A proof-of-concept system based on a sensor fusion approach was built with considerations for lower cost, reduced intrusiveness, and higher mobility, deployability, and portability. This involved the development of both a step detector using the accelerometer and compass of an iPhone 5, and a radio-based localization subsystem using a Kalman filter and received signal strength indication to tackle issues that had been identified as limiting accuracy. The results of our experiments were promising with an average estimation error of 0.47 m. We are confident that with the proposed future work, our design can be adapted to a home-like environment with a more robust localization solution.
Assessing and controlling software quality is still an immature discipline. One of the reasons for this is that many of the concepts and terms that are used in discussing and describing quality are overloaded with a history from manufacturing quality. We argue in this paper that a quite distinct approach is needed to software quality control as compared with manufacturing quality control. In particular, the emphasis in software quality control is in design to fulfill business needs, rather than replication to agreed standards. We will describe how quality goals can be derived from business needs. Following that, we will introduce an approach to quality control that uses rich causal models, which can take into account human as well as technological influences. A significant concern of developing such models is the limited sample sizes that are available for eliciting model parameters. In the final section of the paper we will show how expert judgment can be reliably used to elicit parameters in the absence of statistical data. In total this provides an agenda for developing a framework for quality control in software engineering that is freed from the shackles of an inappropriate legacy.
With REST becoming a dominant architectural paradigm for web services in distributed systems, more and more use cases are applied to it, including use cases that require transactional guarantees. We believe that the loose coupling that is supported by RESTful transactions, makes this currently our preferred interaction style for digital ecosystems (DEs). To further expand its value to DEs, we propose a RESTful transaction model that satisfies both the constraints of recoverable transactions and those of the REST architectural style. We then show the correctness and applicability of the model.
BPEL (Business Process Execution Language) as a de-facto standard for web service orchestration has drawn particularly attention from researchers and industries. BPEL is a semi-formal flow language with complex features such as concurrency and hierarchy. To test a model thoroughly, we need to cover different execution scenarios. As is well known, it is tedious, time consuming, and error prone to design test cases manually, especially for complex modelling languages. Hence, it is desirable to apply existing model-based-testing techniques in the domain of web services. We proposed WSA (Web Service Automata) to be the operational semantics for BPEL. Based on WSA, we propose a model checking based test case generation framework for BPEL. The SPIN and NuSMV model checkers are used as the test generation engine, and the conventional structural test coverage criteria are encoded into LTL and CTL temporal logic. State coverage and transition coverage are used for BPEL control flow testing, and all-du-path coverage is used for BPEL data flow testing. Two levels of test cases can be generated to test whether the implementation of web services conforms to the BPEL behaviour and WSDL interface models. The generated test cases are executed on the JUnit test execution engine.
MindBeat: Kinetifying thought through movement and sound. MindBeat is a software developed at the University of Surrey that facilitates collaborative thinking within a multi-disciplinary set-up. The project involved the development of an electronic space that enabled an academic ensemble to carry out an 'ideas improvisation'. Five academics from very different disciplines were invited to post short 'beats' (texts made up of no more than 3-4 sentences), around a predefined question: what makes multidisciplinary collaborations work? The beats developed in time into a progressive thread of ideas. The aim of the software was to track the emergence, development and decline of new ideas within a multidisciplinary environment, and also to be able to understand the patterns that emerge in this process by representing the ideas visually as coloured squares. The MindBeat software was launched in June 2012 as part of an electronic theatre production of Peter Handke's 'Offending the Audience'. The five Surrey academics played the parts remotely by feeding Handke's text onto the Mindbeat website as part of a three-day durational performance. An open audience was then invited to interact with the play's five voices by sitting at one of five iMac stations set up in the studio space. These five computer monitors showed the text broken down into coloured square patterns. The audience could open the text by clicking onto the coloured squares, which would reveal the short text or beat. They could then add a comment or thought to the original text. The audience's participation produced almost 500 additional beats, creating an alternative version to the Handke script. The Mindbeat software visualised this ideation as a complex pattern of coloured squares. The installation featured generative video and generative electronic music played live throughout the entire three-days. Using the colour and shape patterns of the ideas-exchange as their score, the musicians shared the software visualisation as a basis for their durational sonic improvisation.
Background In diabetic retinopathy (DR) screening programmes feature-based grading guidelines are used by human graders. However, recent deep learning approaches have focused on end to end learning, based on labelled data at the whole image level. Most predictions from such software offer a direct grading output without information about the retinal features responsible for the grade. In this work, we demonstrate a feature based retinal image analysis system, which aims to support flexible grading and monitor progression. Methods The system was evaluated against images that had been graded according to two different grading systems; The International Clinical Diabetic Retinopathy and Diabetic Macular Oedema Severity Scale and the UK's National Screening Committee guidelines. Results External evaluation on large datasets collected from three nations (Kenya, Saudi Arabia and China) was carried out. On a DR referable level, sensitivity did not vary significantly between different DR grading schemes (91.2-94.2.0%) and there were excellent specificity values above 93% in all image sets. More importantly, no cases of severe non-proliferative DR, proliferative DR or DMO were missed. Conclusions We demonstrate the potential of an AI feature-based DR grading system that is not constrained to any specific grading scheme.
The ability to correctly detect the location and derive the contextual information where a concept begins to drift is essential in the study of domains with changing context. This paper proposes a Top-down learning method with the incorporation of a learning accuracy mechanism to efficiently detect and manage context changes within a large dataset. With the utilisation of simple search operators to perform convergent search and JBNC with a graphical viewer to derive context information, the identified hidden context are shown with the location of the disjoint points, the contextual attributes that contribute to the concept drift, the graphical output of the true relationships between these attributes and the Boolean characterisation which is the context.
Background: Medical research increasingly requires the linkage of data from different sources. Conducting a requirements analysis for a new application is an established part of software engineering, but rarely reported in the biomedical literature; and no generic approaches have been published as to how to link heterogeneous health data. Methods: Literature review, followed by a consensus process to define how requirements for research, using, multiple data sources might be modeled. Results: We have developed a requirements analysis: i-ScheDULEs - The first components of the modeling process are indexing and create a rich picture of the research study. Secondly, we developed a series of reference models of progressive complexity: Data flow diagrams (DFD) to define data requirements; unified modeling language (UML) use case diagrams to capture study specific and governance requirements; and finally, business process models, using business process modeling notation (BPMN). Discussion: These requirements and their associated models should become part of research study protocols.
Studies have shown that deaf and hearing-impaired students have many difficulties in learning applied disciplines such as Medicine, Engineering, and Computer Programming. This study aims to investigate the readiness of deaf students to pursue higher education in applied sciences, more specifically in computer science. This involves investigating their capabilities in computer skills and applications. Computer programming is an integral component in the technological field that can facilitate the development of further scientific advances. Devising a manner of teaching the deaf and hearing-impaired population will give them an opportunity to contribute to the technology sector. This would allow these students to join the scientific world when otherwise; they are generally unable to participate because of the limitations they encounter. The study showed that deaf students in Jeddah are eager to continue their higher education and that a large percentage of these students are keen on studying computer science, particularly if they are provided with the right tools.
This study evaluated the effectiveness and usability of a developed collaborative online tool (chit-chat) for children with Attention Deficit Hyperactivity Disorder (ADHD). We studied whether this tool influenced children’s Knowledge and experience exchange, motivation, behavioral abilities and social skills while using another learning tool, ACTIVATE. A total of seven Saudi children with ADHD aged from 6 to 8 years were assigned to the collaborative intervention using iPads. They were asked to play mini games that positively affect children with ADHD cognitively and behaviorally, then chat using our developed collaborative online tool, for three sessions. Progress points were measured and quantitatively analyzed before and after the intervention, thematic analysis was applied on the qualitative data. Participants showed improvements in overall performance when using the learning tool ACTIVATE. Ecollaboration was found to be effective to children with ADHD and positively influencing their knowledge, experience, motivation and social skills.
To make accurate predictions of attributes like defects found in complex software projects we need a rich set of process factors. We have developed a causal model that includes such process factors, both quantitative and qualitative. The factors in the model were identified as part of a major collaborative project. A challenge for such a model is getting the data needed to validate it. We present a dataset, elicited from 31 completed software projects in the consumer electronics industry, which we used for validation. The data were gathered using a questionnaire distributed to managers of recent projects. The dataset will be of interest to other researchers evaluating models with similar aims. We make both the dataset and causal model available for research use.
Soil health is an environmental factor that impacts a range of important issues including food production, water retention and soil organic carbon storage. Agriculture relies on healthy soil for crop growth and animal grazing. Water retention reduces the risks of desertification and of flooding as the capacity to retain water reduces the rate of surface water flow. In addition, soil organic carbon represents the largest terrestrial carbon stock and is second only to the oceans. Yet, soil health is threatened by intensive farming practices and changes of land use such as deforestation. Thus, it is important to manage soil health to maintain food security, avoid desertification and maintain or ideally increase soil organic carbon storage. A useful tool to inform this management function would be a machine learning model that can predict soil health given land cover and parameters of the abiotic context, such as terrain elevation and historical weather data. The first step in developing such a model is to be able to identify the land cover for a chosen area. Satellites provide multi-spectral images that include the visual bands. Land cover databases provide the ground truth labels for a supervised learning approach to train an image semantic segmentation model. This chapter describes how Sentinel-2 satellite image data was combined with data from the UK Centre for Ecology and Hydrology Land Cover Map 2015, to train a convolutional neural network for land cover classification for the South of England.
Information theory has gained application in a wide range of disciplines, including statistical inference, natural language processing, cryptography and molecular biology. However, its usage is less pronounced in medical science. In this chapter, we illustrate a number of approaches that have been taken to applying concepts from information theory to enhance medical decision making. We start with an introduction to information theory itself, and the foundational concepts of information content and entropy. We then illustrate how relative entropy can be used to identify the most informative test at a particular stage in a diagnosis. In the case of a binary outcome from a test, Shannon entropy can be used to identify the range of values of test results over which that test provides useful information about the patient’s state. This, of course, is not the only method that is available, but it can provide an easily interpretable visualization. The chapter then moves on to introduce the more advanced concepts of conditional entropy and mutual information and shows how these can be used to prioritise and identify redundancies in clinical tests. Finally, we discuss the experience gained so far and conclude that there is value in providing an informed foundation for the broad application of information theory to medical decision making.
We describe a true-concurrent approach for managing dependencies between distributed and concurrent coordinator components of a long-running transaction. In previous work we have described how interactions specified in a scenario can be translated into a tuples-based behavioural description, namely vector languages. In this paper we show how reasoning against order-theoretic properties of such languages can reveal missing behaviours which are not explicitly described in the scenario but are still possible. Our approach supports the gradual refinement of scenarios of interaction into a complete set of behaviours that includes all desirable orderings of execution and prohibits emergent behaviour of the transaction. Crown Copyright © 2010.
We describe a formal approach to protocol design for dialogues between autonomous agents in a digital ecosystem that involve the exchange of arguments between the participants. We introduce a vector language-based representation of argumentation protocols, which captures the interplay between different agentspsila moves in a dialogue in a way that (a) determines the legal moves that are available to each participant, in each step, and (b) records the dialogue history. We use UML protocol state machines (PSMs) to model a negotiation dialogue protocol at both the individual participant level (autonomous agent viewpoint) and the dialogue level (overall interaction viewpoint). The underlying vector semantics is used to verify that a given dialogue was played out in compliance with the corresponding protocol.
Purpose The Translational Research and Patients safety in Europe (TRANSFoRm) project aims to integrate primary care with clinical research whilst improving patient safety. The TRANSFoRm International Research Readiness survey (TIRRE) aims to demonstrate data use through two linked data studies and by identifying clinical data repositories and genetic databases or disease registries prepared to participate in linked research. Method The TIRRE survey collects data at micro-, meso- and macro-levels of granularity; to fulfil data, study specific, business, geographical and readiness requirements of potential data providers for the TRANSFoRm demonstration studies. We used descriptive statistics to differentiate between demonstration-study compliant and non-compliant repositories. We only included surveys with >70% of questions answered in our final analysis, reporting the odds ratio (OR) of positive responses associated with a demonstration-study compliant data provider. Results We contacted 531 organisations within the Eurpean Union (EU). Two declined to supply information; 56 made a valid response and a further 26 made a partial response. Of the 56 valid responses, 29 were databases of primary care data, 12 were genetic databases and 15 were cancer registries. The demonstration compliant primary care sites made 2098 positive responses compared with 268 in non-use-case compliant data sources [OR: 4.59, 95% confidence interval (CI): 3.93–5.35, p < 0.008]; for genetic databases: 380:44 (OR: 6.13, 95% CI: 4.25–8.85, p < 0.008) and cancer registries: 553:44 (OR: 5.87, 95% CI: 4.13–8.34, p < 0.008).Conclusions TIRRE comprehensively assesses the preparedness of data repositories to participate in specific research projects. Multiple contacts about hypothetical participation in research identified few potential sites.
The present-day health data ecosystem comprises a wide array of complex heterogeneous data sources. A wide range of clinical, health care, social and other clinically relevant information are stored in these data sources. These data exist either as structured data or as free-text. These data are generally individual person-based records, but social care data are generally case based and less formal data sources may be shared by groups. The structured data may be organised in a proprietary way or be coded using one-of-many coding, classification or terminologies that have often evolved in isolation and designed to meet the needs of the context that they have been developed. This has resulted in a wide range of semantic interoperability issues that make the integration of data held on these different systems changing. We present semantic interoperability challenges and describe a classification of these. We propose a four-step process and a toolkit for those wishing to work more ontologically, progressing from the identification and specification of concepts to validating a final ontology. The four steps are: (1) the identification and specification of data sources; (2) the conceptualisation of semantic meaning; (3) defining to what extent routine data can be used as a measure of the process or outcome of care required in a particular study or audit and (4) the formalisation and validation of the final ontology. The toolkit is an extension of a previous schema created to formalise the development of ontologies related to chronic disease management. The extensions are focused on facilitating rapid building of ontologies for time-critical research studies.
This paper explores the effects of virtual development on product quality, from the viewpoint of "conformance to specifications". Specifically, causes of defect injection and non- or late-detection are explored. Because of the practical difficulties of obtaining hard project-specific defect data, an approach was taken that relied upon accumulated expert knowledge. The accumulated expert knowledge based approach was found to be a practical alternative to an in-depth defect causal analysis on a per-project basis. Defect injection causes seem to be concentrated in the requirements specification phases. Defect dispersion is likely to increase, as requirements specifications are input for derived requirements specifications in multiple, related sub-projects. Similarly, a concentration of causes for the non- or late detection of defects was found in the Integration Test phases. Virtual development increases the likelihood of defects in the end product because of the increased likelihood of defect dispersion, because of new virtual development related defect causes, and because causes already existing in co-located development are more likely to occur.
BACKGROUND: Generally benefits and risks of vaccines can be determined from studies carried out as part of regulatory compliance, followed by surveillance of routine data; however there are some rarer and more long term events that require new methods. Big data generated by increasingly affordable personalised computing, and from pervasive computing devices is rapidly growing and low cost, high volume, cloud computing makes the processing of these data inexpensive. OBJECTIVE: To describe how big data and related analytical methods might be applied to assess the benefits and risks of vaccines. METHOD: We reviewed the literature on the use of big data to improve health, applied to generic vaccine use cases, that illustrate benefits and risks of vaccination. We defined a use case as the interaction between a user and an information system to achieve a goal. We used flu vaccination and pre-school childhood immunisation as exemplars. RESULTS: We reviewed three big data use cases relevant to assessing vaccine benefits and risks: (i) Big data processing using crowdsourcing, distributed big data processing, and predictive analytics, (ii) Data integration from heterogeneous big data sources, e.g. the increasing range of devices in the "internet of things", and (iii) Real-time monitoring for the direct monitoring of epidemics as well as vaccine effects via social media and other data sources. CONCLUSIONS: Big data raises new ethical dilemmas, though its analysis methods can bring complementary real-time capabilities for monitoring epidemics and assessing vaccine benefit-risk balance.
In this chapter, we study some research issues from IoT-based spectrum trading in Wireless Communication in a strategic setting. We consider the scenario in which there are multiple secondary users (such as non-governmental organizations (NGOs), institutional organizations, foundations, etc.) having available un-utilized spectrum and multiple tertiary users (such as small farms, agricultural enterprises or people residing in different localities). Tertiary users provide preferences over the subset of all the available secondary users (NGOs, hereafter). Based on their preference ordering, the tertiary users are allocated the best possible NGOs among the available ones and under the restrictions that each user is assigned to at most one NGO. However, it is to be noted that, in this model, the allocated spectrum might not be available through out a long period of time but rather for a short duration of time within a time period. Therefore, tertiary users have to be able to work off-line and access cached data at the Edges of Internet even if the Internet access is not available. For the purpose of storing and retrieving the cached data several algorithms are designed and their computational complexity is analyzed. In order to empirically measure the efficacy of the proposed mechanisms the simulations are carried out and are compared with the benchmark mechanism. The proposed allocation mechanisms are envisaged as especially useful tools for emerging scenarios of smart farming and precision agriculture, where in situ infrastructures are not available.
Standard practice in building models in software engineering normally involves three steps: collecting domain knowledge (previous results, expert knowledge); building a skeleton of the model based on step 1 including as yet unknown parameters; estimating the model parameters using historical data. Our experience shows that it is extremely difficult to obtain reliable data of the required granularity, or of the required volume with which we could later generalize our conclusions. Therefore, in searching for a method for building a model we cannot consider methods requiring large volumes of data. This paper discusses an experiment to develop a causal model (Bayesian net) for predicting the number of residual defects that are likely to be found during independent testing or operational usage. The approach supports (1) and (2), does not require (3), yet still makes accurate defect predictions (an R 2 of 0.93 between predicted and actual defects). Since our method does not require detailed domain knowledge it can be applied very early in the process life cycle. The model incorporates a set of quantitative and qualitative factors describing a project and its development process, which are inputs to the model. The model variables, as well as the relationships between them, were identified as part of a major collaborative project. A dataset, elicited from 31 completed software projects in the consumer electronics industry, was gathered using a questionnaire distributed to managers of recent projects. We used this dataset to validate the model by analyzing several popular evaluation measures (R 2, measures based on the relative error and Pred). The validation results also confirm the need for using the qualitative factors in the model. The dataset may be of interest to other researchers evaluating models with similar aims. Based on some typical scenarios we demonstrate how the model can be used for better decision support in operational environments. We also performed sensitivity analysis in which we identified the most influential variables on the number of residual defects. This showed that the project size, scale of distributed communication and the project complexity cause the most of variation in number of defects in our model. We make both the dataset and causal model available for research use.
This paper is an introduction to Business Ecosystems and its improvements as represented in Digital Business Ecosystem. In traditional non-digital economic ecosystems, the hubs, once established are static. It is difficult to create new hubs and as such these established hubs enjoy considerable power in the marketplace. Indeed, in many instances, these hubs become the main impediments for the growth of smaller nodes. In other instances, they try to misuse their powers by creating monopolies or oligopolies. In contrast, it is hoped that Digital Business Ecosystems, by virtue of their loosely-coupled and self-organizing properties, will help Small and Medium Enterprises to create a fully distributed network, sidestepping the above mentioned problems of dominance of the large hubs in the market. The authors try to provide a brief introduction to Business Ecosystems and focus on the reasons and advantages of such a move/transition towards Digital Business Ecosystems. © 2010 IEEE.
Declarative technologies have made great strides in expressivity between SQL and SBVR. SBVR models are more expressive that SQL schemas, but not as imminently executable yet. In this paper, we complete the architecture of a system that can execute SBVR models. We do this by describing how SBVR rules can be transformed into SQL DML so that they can be automatically checked against the database using a standard SQL query. In particular, we describe a formalization of the basic structure of an SQL query which includes aggregate functions, arithmetic operations, grouping, and grouping on condition. We do this while staying within a predicate calculus semantics which can be related to the standard SBVR-LF specification and equip it with a concrete semantics for expressing business rules formally. Our approach to transforming SBVR rules into standard SQL queries is thus generic, and the resulting queries can be readily executed on a relational schema generated from the SBVR model.
In this paper we describe a formal model for the distributed coordination of long-running transactions in a Digital Ecosystem for business, involving Small and Medium Enterprises (SMEs). The proposed non-interleaving model of interaction-based service composition allows for communication between internal activities of transactions. The formal semantics of the various modes of service composition are represented by standard xml schemas. The current implementation framework uses suitable asynchronous message passing techniques and reflects the design decisions of the proposed model for distributed transactions in digital ecosystems.
Plant diseases are one of the main causes of crop loss in agriculture. Machine Learning, in particular statistical and neural nets (NNs) approaches, have been used to help farmers identify plant diseases. However, since new diseases continue to appear in agriculture due to climate change and other factors, we need more data-efficient approaches to identify and classify new diseases as early as possible. Even though statistical machine learning approaches and neural nets have demonstrated state-of-the-art results on many classification tasks, they usually require a large amount of training data. This may not be available for emergent plant diseases. So, data-efficient approaches are essential for an early and precise diagnosis of new plant diseases and necessary to prevent the disease’s spread. This study explores a data-efficient Inductive Logic Programming (ILP) approach for plant disease classification. We compare some ILP algorithms (including our new implementation, PyGol) with several statistical and neural-net based machine learning algorithms on the task of tomato plant disease classification with varying sizes of training data set (6, 10, 50 and 100 training images per disease class). The results suggest that ILP outperforms other learning algorithms and this is more evident when fewer training data are available.
The concept of a digital ecosystem (DE) has been used to explore scenarios in which multiple online services and resources can be accessed by users without there being a single point of control. In previous work we have described how the so-called transaction languages can express concurrent and distributed interactions between online services in a transactional environment. In this paper we outline how transaction languages capture the history of a long-running transaction and highlight the benefits of our true-concurrent approach in the context of DEs. This includes support for the recovery of a long-running transaction whenever some failure is encountered. We introduce an animation tool that has been developed to explore the behaviours of long-running transactions within our modelling environment. Further, we discuss how this work supports the declarative approach to the development of open distributed applications. © 2012 IEEE.
Given the expense of more direct determinations, using machine-learning schemes to predict a protein secondary structure from the sequence alone remains an important methodology. To achieve significant improvements in prediction accuracy, the authors have developed an automated tool to prepare very large biological datasets, to be used by the learning network. By focusing on improvements in data quality and validation, our experiments yielded a highest prediction accuracy of protein secondary structure of 90.97%. An important additional aspect of this achievement is that the predictions are based on a template-free statistical modeling mechanism. The performance of each different classifier is also evaluated and discussed. In this paper a protein set of 232 protein chains are proposed to be used in the prediction. Our goal is to make the tools discussed available as services in part of a digital ecosystem that supports knowledge sharing amongst the protein structure prediction community.
To define the key concepts which inform whether a system for collecting, aggregating and processing routine clinical data for research is fit for purpose.
The core goal of this paper is to identify guidance on how the research community can better transition their research into payment card fraud detection towards a transformation away from the current unacceptable levels of payment card fraud. Payment card fraud is a serious and long-term threat to society (Ryman-Tubb and d’Avila Garcez, 2010) with an economic impact forecast to be $416bn in 2017 (see Appendix A).1 The proceeds of this fraud are known to finance terrorism, arms and drug crime. Until recently the patterns of fraud (fraud vectors) have slowly evolved and the criminals modus operandi (MO) has remained unsophisticated. Disruptive technologies such as smartphones, mobile payments, cloud computing and contactless payments have emerged almost simultaneously with large-scale data breaches. This has led to a growth in new fraud vectors, so that the existing methods for detection are becoming less effective. This in turn makes further research in this domain important. In this context, a timely survey of published methods for payment card fraud detection is presented with the focus on methods that use AI and machine learning. The purpose of the survey is to consistently benchmark payment card fraud detection methods for industry using transactional volumes in 2017. This benchmark will show that only eight methods have a practical performance to be deployed in industry despite the body of research. The key challenges in the application of artificial intelligence and machine learning to fraud detection are discerned. Future directions are discussed and it is suggested that a cognitive computing approach is a promising research direction while encouraging industry data philanthropy.
In this chapter, we study some research issues from IoT-based crowdsourcing in strategic setting. We have considered the scenario in the IoT-based crowdsourcing, where there are multiple task requesters and multiple IoT devices as task executors. Each task requester has multiple tasks, with the tasks having start and finish times. Based on the start and finish times, the tasks are to be distributed into different slots. On the other hand, in each slot, each IoT device requests for the set of tasks that it wants to execute along with the valuation that it will charge in exchange of its service. Both the requested set of tasks and the valuations are private informations. Given such scenario, the objective is to allocate the subset of IoT devices to the tasks in a non-conflicting manner with the objective of maximizing the social welfare. For the purpose of determining the unknown quality of the IoT devices we have utilized the concept of peer grading. Therefore, we have designed a truthful mechanism for the problem under investigation that also allows us to have the true information about the quality of the IoT devices.
We propose the use of structured natural language (English) in specifying service choreographies, focusing on the what rather than the how of the required coordination of participant services in realising a business application scenario. The declarative approach we propose uses the OMG standard Semantics of Business Vocabulary and Rules (SBVR) as a modelling language. The service choreography approach has been proposed for describing the global orderings of the invocations on interfaces of participant services. We therefore extend SBVR with a notion of time which can capture the coordination of the participant services, in terms of the observable message exchanges between them. The extension is done using existing modelling constructs in SBVR, and hence respects the standard specification. The idea is that users - domain specialists rather than implementation specialists - can verify the requested service composition by directly reading the structured English used by SBVR. At the same time, the SBVR model can be represented in formal logic so it can be parsed and executed by a machine.