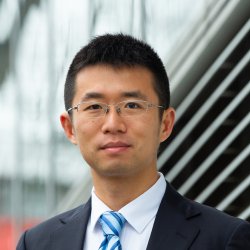
Dr Yunpeng Li
Academic and research departments
Computer Science Research Centre, School of Computer Science and Electronic Engineering.About
Biography
I am a Senior Lecturer in Artificial Intelligence in the School of Computer Science and Electronic Engineering. Prior to joining Surrey as a Lecturer in AI in 2018, I was a Postdoctoral Research Assistant in Machine Learning working with Steve Roberts in the Machine Learning Research Group, University of Oxford (2017-2018). I was also a Junior Research Fellow at Wolfson College, University of Oxford (2018). I completed my Ph.D. in electrical engineering with Mark Coates at McGill University in Montréal, Canada in 2017.
My research interests are in the design and development of machine learning solutions that improve human life. My ongoing and past research efforts particularly focus on high-dimensional, data-adaptive uncertainty quantification methodologies, as well as applications in the areas of AI for health such as dental disease detection with radiography, breast cancer detection with RF sensors, and device-free target tracking in sensor networks. My work on malaria-vectoring mosquito detection using low-cost mobile phones has received media coverage from BBC, the Guardian, New Scientist, Digital Trends, and MIT Technology Review, among other venues.
Areas of specialism
[2024.7] Our paper "Bayesian Detector Combination for Object Detection with Crowdsourced Annotations" has been accepted at ECCV'2024. Congratulations to Zhi Qin Tan on his first paper during his PhD, and to the co-authors Olga Isupova, Gustavo Carneiro, and Xiatian Zhu.
[2024.5] I gave talks at the Summer School on Bayesian filtering: fundamental theory and numerical methods (SSBF 2024) and the 6th Workshop on Sequential Monte Carlo Methods (SMC 2024) in the International Centre for Mathematical Sciences (ICMS) in the Bayes Centre, Edinburgh, between the 6th May and 17th May.
[2024.4] We are recruiting a Research Software Engineer (based at Surrey) with expertise on mobile application development and a Postdoctoral Research Assistant in Bioacoustic Ecological Monitoring (based at Oxford) to work on the NERC-funded HumBug II project on large-scale acoustic monitoring for invasive insect species.
[2024.3] Our project "HumBug II: enabling large-scale acoustic monitoring for invasive insect species" has been funded by the Natural Environment Research Council (NERC) and the Department for Environment, Food and Rural Affairs (Defra), as part of NERC's Innovation in Environmental Monitoring programme! The HumBug II project is in collaboration with Prof Stephen Roberts, Prof Kathy Willis, Dr Marianne Sinka and Eva Herreros-Moya at the University of Oxford.
[2024.2] Adarsh Kappiyath joined the group as a PhD student. Adarsh will work on the fairness and robustness of machine learning models with a particular focus on medical imaging. Welcome Adarsh!
[2024.1] Zhi Qin, John-Jo, and I presented our work in the 2024 Bellairs Workshop on Machine Learning and Statistical Signal Processing for Data on Graphs held in the Bellairs Research Institute in Barbados.
[2023.12] Congratulations Xiongjie Chen for passing his PhD Viva with minor correction, examined by Prof Arnaud Douct at the University of Oxford and Prof Simon Maskell at the University of Liverpool! His PhD thesis topic is "Data-adaptive Uncertainty Quantification and Propagation with Optimal Transport and Differentiable Particle Filters". Xiongjie is staying in the group as a postdoctoral researcher working on the NIHR-sponsored project on "Development and pre-market evaluation of AI-assisted dental disease detection with radiography".
[2023.12] I gave a talk on our Zooniverse projects for HumBug and AI for Dentistry in the Crowdsourcing Biomedical Research Meeting held at Magdalen College at the University of Oxford.
[2023.11] I presented a poster to introduce our work on "crowdsourcing for AI-assisted dental disease detection" in the Global Symposium on Artificial Intelligence in Dentistry at the Harvard University in Boston, USA.
[2023.10] I organised a Special Session on "Machine learning meets Monte Carlo" at the 57th Asilomar Conference on Signals, Systems, and Computers held in Asilomar, California, USA, where Jiaxi presented our ongoing work on online learning for differentiable particle filtering.
[2023.9] Vishal Thengane joined the group as a PhD student. He will develop machine learning tools for 3D medical imaging applications. Welcome Vishal!
[2023.9] A UK/International PhD studentship is available for research at the intersection of computer vision, natural language processing and medical imaging.
[2023.9] A 0.2FTE project manager position is available for our NIHR-sponsored project on AI-assisted dental disease detection with radiography.
[2023.8] I gave a talk on "Computational Optimal Transport Methods and Applications" in the Data Science and Computational Statistics Seminar hosted by the School of Mathematics at the University of Birmingham.
[2023.8] Press release: our collaborative project on AI-assisted dental disease detection has been awarded a £1.55M grant by the National Institute for Health and Social Care (NIHR), in collaboration with King's College London, Royal Surrey NHS Foundation Trust, Oral Health Foundation, Guy's and St. Thomas NHS Foundation Trust, Guildford Dental Academy and JM Radiology.
[2023.7] I started a 12-month Royal Academy of Engineering Enterprise Fellowship to accelerate our innovation activities in AI-assisted dental disease detection tool with radiography through a commercialisation vehicle.
[2023.7] John-Joseph Brady joined the group as a PhD student. John-Jo will develop tools and theories in the intersection between distributed Bayesian inference and machine learning. Welcome!
[2023.7] I presented a paper "Differentiable Bootstrap Particle Filters for Regime-Switching Models" at IEEE Statistical Signal Processing (SSP) Workshop 2023 in Hanoi, Vietnam.
[2023.6] I presented two papers "Batch-Ensemble Stochastic Neural Networks for Out-of-Distribution Detection" and "Particle Flow Gaussian Sum Particle Filter" at International Conference on Acoustics, Speech, and Signal Processing (ICASSP) in Rhodes, Greece. They are joint work with Xiongjie Chen (Surrey), Yongxin Yang (Queen Mary), Karthik Comandur (IIIT Hyderabad), and Santosh Nannuru (IIIT Hyderabad).
[2023.5] We are recruiting a 2.5-year Postdoctoral Research Fellow in Machine Learning for Medical Imaging.
[2023.4] We welcome Zhi Qin Tan to the group as a PhD student. Zhi Qin will work on multi-modal learning with the support from an EPSRC/NPL Industrial Cooperative Awards in Science & Technology (iCASE) studentship.
[2023.3] I presented our work on AI-assisted dental disease detection in the 2nd Dental Symposium of the ITU/WHO Focus Group “AI for Health” held at MIT in Cambridge, USA.
[2023.2] We are recruiting! A joint University of Surrey - University of Wollongong PhD project is open for applicants interested in statistical machine learning.
[2023.1] Xiongjie and I attended the 2023 Bellairs Workshop on Machine Learning and Statistical Signal Processing for Data on Graphs at the Bellairs Research Institute in Barbados, where I talked about some of the group's work on optimal transports and their applications.
[2023.1] Jiaxi Li joined the group as a PhD student. He won a Faculty of Engineering and Physical Sciences (FEPS) PhD studentship to support his work on differentiable particle filters. Welcome Jiaxi!
[2022.12] Ryan Banks and I visited School of Dentistry at the University of Sao Paulo in Brazil, hosted by Prof Fausto Medeiros Mendes and Prof Mariana Braga to enhance our collaboration on AI-assisted dental disease detection, particularly with paediatric radiographs.
[2022.12] We launched our Dental Radiograph Collection, Annotation, and Prediction System (DR CAPS) drcaps.surrey.ac.uk ! If you are dental professionals or students and would like to help collect expert-quality dental radiograph annotations, please sign up using this MS form, and we will send you a link for sign up once your application is reviewed.
[2022.12] We are hiring! New fully-funded PhD studentship for 3.5 years available on Machine learning meets sequential Monte Carlo methods for April, July 2023 start. This is a collaboration with National Physical Laboratory (NPL) and will involve a secondment for at least three months in the Data Science Department at NPL.
[2022.11] We welcomed Prof Fausto Medeiros Mendes, Prof Mariana Braga and Prof Daniela Prócida Raggio from School of Dentistry at University of Sao Paulo (Faculdade de Odontologia da Universidade de São Paulo (FOUSP)) in Brazil in a 4-day visit to Surrey as part of our University Global Partnership Network (UGPN)-supported collaboration on AI for dentistry.
[2022.10] I gave a talk on "Going with flow: transport methods and neural networks for sequential Monte Carlo methods" at the Institute for Digital Communications (IDCOM) Seminar at University of Edinburgh.
[2022.10] We are hiring! A 4-year fully-funded PhD studentship for UK and Pre-settled EU students on Statistical machine learning for medical imaging is available for January, April, July 2023 start. The application is rolling-based, so do apply early if you are interested.
[2022.9] We launched our AI-assisted dental disease detection project website https://ai4d.surrey.ac.uk as our public portal to engage with patients, dentists and the general public. The website was built by Chauwat Charoenwiriyapap with support from Ryan Banks. Chauwat's summer internship was generously sponsored by a Public Engagement with STEM Research Seed Fund (PER-STEM) grant for the dental disease detection project.
[2022.9] I presented a paper Imitation Learning with Sinkhorn Distances at European Conference on Machine Learning and Principles and Practice of Knowledge Discovery in Databases (ECML-PKDD) in Grenoble, France. The work was derived from George Papagiannis' final-year undergraduate project at Surrey before he moved to Cambridge for an MSc study and Imperial for PhD. It was fantastic experience working with George - looks like George felt the same.
[2022.8] Xiongjie and I gave a tutorial on "Differentiable Particle Filters for Data-adaptive Sequential Bayesian Inference" and a paper "Conditional Measurement Density Estimation in Sequential Monte Carlo via Normalizing Flow" at the 30th European Signal Processing Conference (EUSIPCO) in Belgrade, Serbia.
[2022.7] I presented a paper Particle Flow Gaussian Particle Filter in the 25th International Conference on Information Fusion in Linköping, Sweden. This is collaborative work with Karthik Comandur and Santosh Nannuru at IIIT Hyderabad, India.
[2022.7] More than 2000 volunteers have participated in our Dental Disease Detection Zooniverse project to contribute crowdsourced dental radiograph annotations!
[2022.5] Xiongjie and I presented our recent work on normalising flow-based differentiable particle filters (for learning dynamic models and proposal distributions and for learning measurement models) in the 5th Workshop on Sequential Monte Carlo Methods in Madrid, Spain.
[2022.3] Talk at University of Glasgow and ICMS - Research and Teaching in Statistical and Data Sciences seminar.
[2022.2] We are organising a Special Session on ''Machine Learning for Sequential Monte Carlo Methods" at EUSIPCO 2022. Submission deadline extended to 7th March 2022.
[2022.1] One paper Augmented Sliced Wasserstein Distances was accepted by ICLR2022. Congratulations to Xiongjie and Yongxin!
[2022.1] Became a Surrey AI Fellow at the Surrey Institute for People-Centred AI.
[2021.12] Talk at 2021 Bellairs Workshop on Machine Learning and Statistical Signal Processing for Data on Graphs in the Bellairs Research Institute in Barbados.
[2021.12] Talk at CIDFIC Innovation Meetup.
[2021.12] We are hiring! Two PhD studentships on "Differentiable particle filters for data-driven sequential inference" are available for April, July, October 2022 and January, April, July 2023 start. Please contact me if you are interested in working with me on the interface between machine learning and Bayesian Monte Carlo methods. We also support the application of Vice Chancellors Studentship Award, Breaking Barriers Studentship Award, Shine Scholars Studentship Award, and China Scholarship Council (CSC) - Surrey Award on the broad topics of statistical machine learning and AI for health for October 2022 starters.
[2021.12] Official Launch of the dental disease detection Zooniverse site!
[2021.12] The HumBugDB acoustic mosquito dataset was presented at NeurIPS'21 Track on Datasets and Benchmarks. Congratulations to Dr Ivan Kiskin and the Oxford team!
[2021.12] Ryan Banks joined the team as a Research Assistant in December 2021 and will start as a PhD student in January 2022, having worked in the team as a MSc student throughout 2021. Welcome back Ryan!
[2021.11] Xiongjie Chen and I attended FUSION'21 in Sun City, South Africa to present the paper Differentiable Particle Filters through Conditional Normalizing Flow.
[2021.9] We are hiring! One fully funded PhD studentships on "Uncertainty Quantification for Robust AI through Optimal Transport" is available for January 2022 start.
[2021.8] Dr Govind Sharma joined the team as a Postdoctoral Research Fellow. Welcome!
[2021.7] We organised the ICML 2021 Debate: Should AI Research and Development Be Controlled by a Regulatory Body or Government Oversight?
[2021.7] Talk at 2021 ABCP Annual Conference.
[2021.6] One paper End-To-End Semi-supervised Learning for Differentiable Particle Filters was presented at ICRA'21.
[2021.4] Talk in the Department of Computer Science at University of Cambridge.
[2021.4] Became an Associate Editor for Neurocomputing.
News
In the media
ResearchResearch interests
I am interested in foundational machine learning topics motivated by applications aiming to improve human life and environment. I am currently working on improving the ability of deep neural networks to quantify uncertainty in their predictions, which can benefit vast data science domains, from disease diagnostics to environment sensing. My work on trustworthy AI has found applications in cancer detection, biomedical imaging, and infectious disease monitoring.
Research projects
Building an AI-powered system with the capability in recognising normal anatomical structures and differentiate from subtle abnormalities in digitised dental radiographic images.
Machine learning for sequential Monte Carlo methods.
Discover malaria-vectoring mosquitoes from their buzz.
Designing Monte Carlo methods effective for high-dimensional filtering.
Developing machine learning techniques for massive breast screening with microwave scans.
Multiple target tracking in a device-free tracking setting.
Optimal Transport Methods and Applications
Differentiable Particle Filtersfor Data-adaptive Sequential Bayesian Inference
Research interests
I am interested in foundational machine learning topics motivated by applications aiming to improve human life and environment. I am currently working on improving the ability of deep neural networks to quantify uncertainty in their predictions, which can benefit vast data science domains, from disease diagnostics to environment sensing. My work on trustworthy AI has found applications in cancer detection, biomedical imaging, and infectious disease monitoring.
Research projects
Building an AI-powered system with the capability in recognising normal anatomical structures and differentiate from subtle abnormalities in digitised dental radiographic images.
Machine learning for sequential Monte Carlo methods.
Discover malaria-vectoring mosquitoes from their buzz.
Designing Monte Carlo methods effective for high-dimensional filtering.
Developing machine learning techniques for massive breast screening with microwave scans.
Multiple target tracking in a device-free tracking setting.
Optimal Transport Methods and Applications
Differentiable Particle Filtersfor Data-adaptive Sequential Bayesian Inference
Supervision
Postgraduate research supervision
Postdoctoral Research Fellow:
Xiongjie Chen (2023 - )
Govind Sharma (2021; now a senior data scientist at Reed)
Conghui Hu (2020 - 2021; now a lecturer at National University of Singapore)
PhD students (Principal Supervisor):
Adarsh Kappiyath (2024 - )
Vishal Thengane (2023 - )
John-Joseph Brady (2023 - )
Zhi Qin Tan (2023 - )
Jiaxi Li (2023 - )
Ryan Banks (2022 - )
Xiongjie Chen (2019 - 2023)
PhD students (Co-supervisor):
Al-Hussein Abutaleb (2022 - )
Parthi Parthipan (2021 - )
Xinhao Mei (2021 - )
Shiqing Liu (2020 - )
Guoyang Xie (2019 - )
Galen Wilkerson (2019 - 2022)
Xilu Wang (2019 - 2022)
Narges Pourshahrokhi (2019 - 2023)
Project Manager:
Jacky Lam (2023 - present)
MSc student researcher:
Ryan Banks (2020 - 2021)
Undergraduate student researcher:
Zion Ejechi (2023 - 2024)
Josh Everett (2023)
Zamzam Mohamed (2021)
Georgios Papagiannis (2019 - 2020; now a PhD student at Imperial College London)
Completed postgraduate research projects I have supervised
Teaching
Teaching
- COM2028: Artificial Intelligence (2021/2022, 2022/2023)
- COMM054: Data Science Principles and Practices (2019/20, 2020/21)
- COM1033: Foundations of Computing II (2018/19, 2019/20, 2020/21, 2021/22)
Departmental duties
- PGR Admissions (2022-2023)
- Year 1 (undergraduate) Coordinator and Personal Tutor (2018 - now)
- Industry Placement Visiting Tutor (2018 - 2021)
- Departmental Seminar Organiser (2018 - 2019)