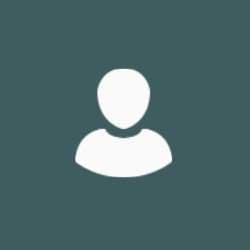
Dr Philip Foster
Publications
Leveraging machine learning techniques, in the context of object-based media production, could enable provision of personalized media experiences to diverse audiences. To fine-tune and evaluate techniques for personalization applications, as well as more broadly, datasets which bridge the gap between research and production are needed. We introduce and publicly release such a dataset, themed around a UK weather forecast and shot against a blue-screen background, of three professional actors/presenters – one male and one female (English) and one female (British Sign Language). Scenes include both production and research-oriented examples, with a range of dialogue, motions, and actions. Capture techniques consisted of a synchronized 4K resolution 16-camera array, production-typical microphones plus professional audio mix, a 16-channel microphone array with collocated Grasshopper3 camera, and a photogrammetry array. We demonstrate applications relevant to virtual production and creation of personalized media including neural radiance fields, shadow casting, action/event detection, speaker source tracking and video captioning.
Leveraging machine learning techniques, in the context of object-based media production, could enable provision of personalized media experiences to diverse audiences. To fine-tune and evaluate techniques for personalization applications, as well as more broadly, datasets which bridge the gap between research and production are needed. We introduce and publicly release such a dataset, themed around a UK weather forecast and shot against a blue-screen background, of three professional actors/presenters – one male and one female (English) and one female (British Sign Language). Scenes include both production and research-oriented examples, with a range of dialogue, motions, and actions. Capture techniques consisted of a synchronized 4K resolution 16-camera array, production-typical microphones plus professional audio mix, a 16-channel microphone array with collocated Grasshopper3 camera, and a photogrammetry array. We demonstrate applications relevant to virtual production and creation of personalized media including neural radiance fields, shadow casting, action/event detection, speaker source tracking and video captioning.
Acoustic event detection for content analysis in most cases relies on lots of labeled data. However, manually annotating data is a time-consuming task, which thus makes few annotated resources available so far. Unlike audio event detection, automatic audio tagging, a multi-label acoustic event classification task, only relies on weakly labeled data. This is highly desirable to some practical applications using audio analysis. In this paper we propose to use a fully deep neural network (DNN) framework to handle the multi-label classification task in a regression way. Considering that only chunk-level rather than frame-level labels are available, the whole or almost whole frames of the chunk were fed into the DNN to perform a multi-label regression for the expected tags. The fully DNN, which is regarded as an encoding function, can well map the audio features sequence to a multi-tag vector. A deep pyramid structure was also designed to extract more robust high-level features related to the target tags. Further improved methods were adopted, such as the Dropout and background noise aware training, to enhance its generalization capability for new audio recordings in mismatched environments. Compared with the conventional Gaussian Mixture Model (GMM) and support vector machine (SVM) methods, the proposed fully DNN-based method could well utilize the long-term temporal information with the whole chunk as the input. The results show that our approach obtained a 15% relative improvement compared with the official GMM-based method of DCASE 2016 challenge.
For the task of sound source recognition, we introduce a novel data set based on 6.8 hours of domestic environment audio recordings. We describe our approach of obtaining annotations for the recordings. Further, we quantify agreement between obtained annotations. Finally, we report baseline results for sound source recognition using the obtained dataset. Our annotation approach associates each 4-second excerpt from the audio recordings with multiple labels, on a set of 7 labels associated with sound sources in the acoustic environment. With the aid of 3 human annotators, we obtain 3 sets of multi-label annotations, for 4378 4-second audio excerpts. We evaluate agreement between annotators by computing Jaccard indices between sets of label assignments. Observing varying levels of agreement across labels, with a view to obtaining a representation of ‘ground truth’ in annotations, we refine our dataset to obtain a set of multi-label annotations for 1946 audio excerpts. For the set of 1946 annotated audio excerpts, we predict binary label assignments using Gaussian mixture models estimated on MFCCs. Evaluated using the area under receiver operating characteristic curves, across considered labels we observe performance scores in the range 0.76 to 0.98
Environmental audio tagging aims to predict only the presence or absence of certain acoustic events in the interested acoustic scene. In this paper we make contributions to audio tagging in two parts, respectively, acoustic modeling and feature learning. We propose to use a shrinking deep neural network (DNN) framework incorporating unsupervised feature learning to handle the multi-label classification task. For the acoustic modeling, a large set of contextual frames of the chunk are fed into the DNN to perform a multi-label classification for the expected tags, considering that only chunk (or utterance) level rather than frame-level labels are available. Dropout and background noise aware training are also adopted to improve the generalization capability of the DNNs. For the unsupervised feature learning, we propose to use a symmetric or asymmetric deep de-noising auto-encoder (syDAE or asyDAE) to generate new data-driven features from the logarithmic Mel-Filter Banks (MFBs) features. The new features, which are smoothed against background noise and more compact with contextual information, can further improve the performance of the DNN baseline. Compared with the standard Gaussian Mixture Model (GMM) baseline of the DCASE 2016 audio tagging challenge, our proposed method obtains a significant equal error rate (EER) reduction from 0.21 to 0.13 on the development set. The proposed asyDAE system can get a relative 6.7% EER reduction compared with the strong DNN baseline on the development set. Finally, the results also show that our approach obtains the state-of-the-art performance with 0.15 EER on the evaluation set of the DCASE 2016 audio tagging task while EER of the first prize of this challenge is 0.17.
In this paper, we present a deep neural network (DNN)-based acoustic scene classification framework. Two hierarchical learning methods are proposed to improve the DNN baseline performance by incorporating the hierarchical taxonomy information of environmental sounds. Firstly, the parameters of the DNN are initialized by the proposed hierarchical pre-training. Multi-level objective function is then adopted to add more constraint on the cross-entropy based loss function. A series of experiments were conducted on the Task1 of the Detection and Classification of Acoustic Scenes and Events (DCASE) 2016 challenge. The final DNN-based system achieved a 22.9% relative improvement on average scene classification error as compared with the Gaussian Mixture Model (GMM)-based benchmark system across four standard folds.
We describe an information-theoretic approach to the analysis of music and other sequential data, which emphasises the predictive aspects of perception, and the dynamic process of forming and modifying expectations about an unfolding stream of data, characterising these using the tools of information theory: entropies, mutual informations, and related quantities. After reviewing the theoretical foundations, we discuss a few emerging areas of application, including musicological analysis, real-time beat-tracking analysis, and the generation of musical materials as a cognitively-informed compositional aid. © 2012 IEEE.