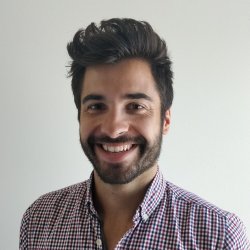
Dr Rúben Borralho
About
My research project
ss
s
Publications
Seamless and ubiquitous coverage are key factors for future cellular networks. Despite capacity and data rates being the main topics under discussion when envisioning the Fifth Generation (5G) and beyond of mobile communications, network coverage remains one of the major issues since coverage quality highly impacts the system performance and end-user experience. The increasing number of base stations and user terminals is anticipated to negatively impact the network coverage due to increasing interference. Furthermore, the "ubiquitous coverage" use cases, including rural and isolated areas, present a significant challenge for mobile communication technologies. This survey presents an overview of the concept of coverage, highlighting the ways it is studied, measured, and how it impacts the network performance. Additionally, an overlook of the most important key performance indicators influenced by coverage, which may affect the envisioned use cases with respect to throughput, latency, and massive connectivity, are discussed. Moreover, the main existing developments and deployments which are expected to augment the network coverage, in order to meet the requirements of the emerging systems, are presented as well as implementation challenges.
The evolution of network technologies has witnessed a paradigm shift toward open and intelligent networks, with the Open Radio Access Network (O-RAN) architecture emerging as a promising solution. O-RAN introduces disaggregation and virtualization, enabling network operators to deploy multi-vendor and interoperable solutions. However, managing and automating the complex O-RAN ecosystem presents numerous challenges. To address this, machine learning (ML) techniques have gained considerable attention in recent years, offering promising avenues for network automation in O-RAN. This paper presents a comprehensive survey of the current research efforts on network automation usingML in O-RAN.We begin by providing an overview of the O-RAN architecture and its key components, highlighting the need for automation. Subsequently, we delve into O-RAN support forML techniques. The survey then explores challenges in network automation usingML within the O-RAN environment, followed by the existing research studies discussing application of ML algorithms and frameworks for network automation in O-RAN. The survey further discusses the research opportunities by identifying important aspects whereML techniques can benefit.
Adequate and uniform network coverage provision is one of the main objectives of cellular service providers. Additionally, the densification of cells exacerbates coverage and service provision challenges, particularly at the cell-edges. In this paper, we present a new approach of cell-sweeping-based Base Stations (BSs) deployments in cellular Radio Access Networks (RANs) where the coverage is improved by enhancing the cell-edge performance. In essence, the concept of cell-sweeping rotates/sweeps the sectors of a site in azimuth continuously/discretely resulting in near-uniform distribution of the signal-to-interference-plus-noise ratio (SINR) around the sweeping site. This paper investigates the proposed concept analytically by deriving expressions for the PDF/CDF of SINR and achievable rate; and with the help of system-level simulations, it shows that the proposed concept can provide throughput gains of up to 125% at the cell-edge. Then, using a link-budget analysis, it is shown that the maximum allowable path loss (MAPL) increases by 2.1 dB to 4.1 dB corresponding to the gains in wideband SINR and post-equalized SINR, respectively. This increase in MAPL can be translated to cell-radius/area with the help of the Okumura-Hata propagation model and results in cell-coverage area enhancement by 30% to 66% in a Typical Urban cell deployment scenario.
—Network coverage is an increasing concern for the Quality of Service (QoS) targets of new mobile technologies. New solutions designed to fulfill the requirements of the existing fifth-generation (5G) and upcoming sixth-generation (6G) emerging scenarios are based on deploying a high number of network access points (APs), which tend to considerably degrade coverage and cell-edge performance due to added interference and increase the energy consumption of cellular systems. In this paper, we present new results on our recently proposed novel concept of cell-sweeping that aims to minimize the coverage dead-spots and improve cell-edge user performance. More specifically, the concept is explored further in this paper analyzing the impact of different cell-sweeping configurations and evaluating the potential benefits towards achieving energy efficiency. By means of system level computer simulations, it is shown that cell-sweeping provides energy savings of 11% and 26.5% for a similar average and cell-edge user throughput performance, respectively, when compared to the conventional static cell deployment in a typical urban macro cell scenario.
The exponential growth of the network elements and data traffic exchange in the last few years elevated the need of network providers for optimized and cost-efficient solutions regarding network management and monitorization. Solutions such as drive-tests (DTs) are becoming extremely expensive with the vast extension and complexity of nowadays mobile networks. Therefore, this paper provides a solution for optimized networkcontext knowledge acquisition, towards the self-organizing networks (SONs) concept. The presented framework incorporates an entire scheme for network Traces processing and positioning, based on network measurements and fingerprinting techniques. This framework enables a series of different use cases for network management and optimization, with real-time data processing capabilities within the network Traces collection interval (15 minutes), and achieving a median positioning error of 90 m.
Good network coverage is an important element of Quality of Service (QoS) provision that mobile cellular operators aim to provide. The established requirements for the existing Fifth Generation (5G) and the emerging scenarios for upcoming Sixth Generation (6G) cellular communication technologies highly depend on the coverage quality that the network is able to provide. In addition, some proposed 5G solutions such as densification, are complex, costly, and tend to degrade network coverage due to increased interference which is critical for the cell-edge performance. In this direction, we present a novel concept of cell-sweeping for coverage enhancement in cellular networks. One of the main objectives behind this mechanism relies on overcoming the cell-edge problem which directly translates into better network coverage. In sequence, the concept operation is introduced and compared to the conventional static cell scenarios. These comparisons target mostly the benefits at the cell-edge locations. Additionally, the use of schedulers that take advantage of the sweeping system is expected to extend the cell-edge benefits to the entire network. This is observed when deploying cellsweeping with the Proportional Fair (PF) scheduler. A 5thpercentile improvement of 125% and an average throughput increase of 35% were obtained through system level simulations. The preliminary results presented in this paper suggest that cellsweeping can be adopted as an emerging technology for future Radio Access Network (RAN) deployments.