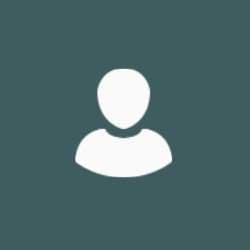
Dr Ryan Wong
Publications
Most of the vision-based sign language research to date has focused on Isolated Sign Language Recognition (ISLR), where the objective is to predict a single sign class given a short video clip. Although there has been significant progress in ISLR, its real-life applications are limited. In this paper, we focus on the challenging task of Sign Spotting instead, where the goal is to simultaneously identify and localise signs in continuous co-articulated sign videos. To address the limitations of current ISLR-based models, we propose a hierarchical sign spotting approach which learns coarse-to-fine spatio-temporal sign features to take advantage of representations at various temporal levels and provide more precise sign localisation. Specifically, we develop Hierarchical Sign I3D model (HS-I3D) which consists of a hierarchical network head that is attached to the existing spatio-temporal I3D model to exploit features at different layers of the network. We evaluate HS-I3D on the ChaLearn 2022 Sign Spotting Challenge-MSSL track and achieve a state-of-the-art 0.607 F1 score, which was the top-1 winning solution of the competition.
In natural language processing (NLP) of spoken languages, word embeddings have been shown to be a useful method to encode the meaning of words. Sign languages are visual languages, which require sign embeddings to capture the visual and linguistic semantics of sign.Unlike many common approaches to Sign Recognition, we focus on explicitly creating sign embeddings that bridge the gap between sign language and spoken language. We propose a learning framework to derive LCC (Learnt Contrastive Concept) embeddings for sign language, a weakly supervised contrastive approach to learning sign embeddings. We train a vocabulary of embeddings that are based on the linguistic labels for sign video. Additionally, we develop a conceptual similarity loss which is able to utilise word embeddings from NLP methods to create sign embeddings that have better sign language to spoken language correspondence. These learnt representations allow the model to automatically localise the sign in time.Our approach achieves state-of-the-art keypoint-based sign recognition performance on the WLASL and BOBSL datasets.
In natural language processing (NLP) of spoken languages, word embeddings have been shown to be a useful method to encode the meaning of words. Sign languages are visual languages, which require sign embeddings to capture the visual and linguistic semantics of sign. Unlike many common approaches to Sign Recognition, we focus on explicitly creating sign embeddings that bridge the gap between sign language and spoken language. We propose a learning framework to derive LCC (Learnt Contrastive Concept) embeddings for sign language, a weakly supervised contrastive approach to learning sign embeddings. We train a vocabulary of embeddings that are based on the linguistic labels for sign video. Additionally, we develop a conceptual similarity loss which is able to utilise word embeddings from NLP methods to create sign embeddings that have better sign language to spoken language correspondence. These learnt representations allow the model to automatically localise the sign in time. Our approach achieves state-of-the-art keypoint-based sign recognition performance on the WLASL and BOBSL datasets.
Automatic Sign Language Translation requires the integration of both computer vision and natural language processing to effectively bridge the communication gap between sign and spoken languages. However, the deficiency in large-scale training data to support sign language translation means we need to leverage resources from spoken language. We introduce, Sign2GPT, a novel framework for sign language translation that utilizes large-scale pretrained vision and language models via lightweight adapters for gloss-free sign language translation. The lightweight adapters are crucial for sign language translation, due to the constraints imposed by limited dataset sizes and the computational requirements when training with long sign videos. We also propose a novel pretraining strategy that directs our encoder to learn sign representations from automatically extracted pseudo-glosses without requiring gloss order information or annotations. We evaluate our approach on two public benchmark sign language translation datasets, namely RWTH-PHOENIX-Weather 2014T and CSL-Daily, and improve on state-of-the-art gloss-free translation performance with a significant margin.
In natural language processing (NLP) of spoken languages , word embeddings have been shown to be a useful method to encode the meaning of words. Sign languages are visual languages, which require sign embeddings to capture the visual and linguistic semantics of sign. Unlike many common approaches to Sign Recognition, we focus on explicitly creating sign embeddings that bridge the gap between sign language and spoken language. We propose a learning framework to derive LCC (Learnt Con-trastive Concept) embeddings for sign language, a weakly supervised contrastive approach to learning sign embed-dings. We train a vocabulary of embeddings that are based on the linguistic labels for sign video. Additionally, we develop a conceptual similarity loss which is able to utilise word embeddings from NLP methods to create sign embed-dings that have better sign language to spoken language correspondence. These learnt representations allow the model to automatically localise the sign in time. Our approach achieves state-of-the-art keypoint-based sign recognition performance on the WLASL and BOBSL datasets.