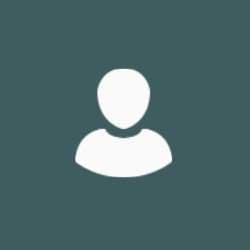
Dr Saeid Safavi
About
Biography
Saeid Safavi is a Research Fellow at the at the Centre for Vision, Speech and Signal Processing (CVSSP), and previously a Postdoctoral researcher at the University of Birmingham and University of Hertfordshire. He achieved his PhD and Masters of Engineering degrees at the University of Birmingham.
He is currently working on a project, named "Audio commons".
Prior to this, he was working on projects with the titles of "Objective Control of TAlker VErication (OCTAVE)", "Accent recognition using a fusion of acoustic and phonotactic architectures", and "Instrument player detection using machine learning".
He has published over 20+ refereed papers in leading journals and conferences. He is a reviewer for different IEEE, Springer, and Elsevier journals and conferences. He won the first prize at the "IET enterprise" competitions on topic "Intelligent literacy system for children" and on the topic "UK’s computerized child literacy support system". In addition to research, Saeid has also been involved in student supervision, teaching, and organizing lab sessions for different projects and courses such as Speech, audio and music processing; Multimedia data; and Maths for Applied computing.
Areas of specialism
University roles and responsibilities
- Research fellow under H2020 project (Audio Commons)
My qualifications
Previous roles
ResearchResearch interests
Saeid Safavi has 10+ years of leading and collaborating with multidisciplinary teams in AI. His current (and past) research activities include applications of machine learning approaches in solving a range of data science and big data.
His research interests are focused on but not limited to the following areas:
- AI, machine learning
- Natural language processing (topic modeling, semantic analysis, and data mining)
- Spoken language processing (dialect/language recognition, speaker recognition, speaker diarization)
Research projects
The Audio Commons Initiative aims at bringing Creative Commons audio content to the creative industries. But what does this mean? We realize that significant amounts of user-generated audio content, such as sound effects, field recordings, musical samples and music pieces (among others), are uploaded to online repositories and made available under creative commons licenses. Furthermore, a constantly increasing amount of multimedia content, originally released with traditional copyright licenses, is becoming public domain as its copyright expires. However, we believe that the professional creative industries (e.g. video-games, film and music industries) are not yet using much of all this content in their media productions.
Research interests
Saeid Safavi has 10+ years of leading and collaborating with multidisciplinary teams in AI. His current (and past) research activities include applications of machine learning approaches in solving a range of data science and big data.
His research interests are focused on but not limited to the following areas:
- AI, machine learning
- Natural language processing (topic modeling, semantic analysis, and data mining)
- Spoken language processing (dialect/language recognition, speaker recognition, speaker diarization)
Research projects
The Audio Commons Initiative aims at bringing Creative Commons audio content to the creative industries. But what does this mean? We realize that significant amounts of user-generated audio content, such as sound effects, field recordings, musical samples and music pieces (among others), are uploaded to online repositories and made available under creative commons licenses. Furthermore, a constantly increasing amount of multimedia content, originally released with traditional copyright licenses, is becoming public domain as its copyright expires. However, we believe that the professional creative industries (e.g. video-games, film and music industries) are not yet using much of all this content in their media productions.
Publications
Due to the widespread use of drones in an urban environment, drones present an increased risk to the safety of urban life. Reliable detection of drones becomes crucial for countering the hazard introduced by drones. However, drones are difficult to detect because of their size and customization. This paper introduces DDL, a dataset aimed at drone sound detection, classification, and localization via a specially constructed set of microphones. As a baseline, we propose a deep uncertainty-aware framework implementing Conformer for joint drone classification and localization. We employ heteroscedastic loss functions that jointly estimate means and variances for spatial localization to model prediction uncertainty. Experiments on the DDL dataset demonstrate a classification accuracy of 99.9% and a Euclidean distance mean absolute error (MAE) of approximately 16 meters. The uncertainty estimates are well-calibrated, with coverage closely matching the expected confidence intervals (68%, 95%, and 99.7%) as defined by the empirical rule, suggesting DDL as a benchmark dataset for audio-based drone localization.
In this paper, we compare different deep neural networks (DNN) in extracting speech signals from competing speakers in room environments, including the conventional fullyconnected multilayer perception (MLP) network, convolutional neural network (CNN), recurrent neural network (RNN), and the recently proposed capsule network (CapsNet). Each DNN takes input of both spectral features and converted spatial features that are robust to position mismatch, and outputs the separation mask for target source estimation. In addition, a psychacoustically-motivated objective function is integrated in each DNN, which explores perceptual importance of each TF unit in the training process. Objective evaluations are performed on the separated sounds using the converged models, in terms of PESQ, SDR as well as STOI. Overall, all the implemented DNNs have greatly improved the quality and speech intelligibility of the embedded target source as compared to the original recordings. In particular, bidirectional RNN, either along the temporal direction or along the frequency bins, outperforms the other DNN structures with consistent improvement.
Perceptual measurements have typically been recognized as the most reliable measurements in assessing perceived levels of reverberation. In this paper, a combination of blind RT60 estimation method and a binaural, nonlinear auditory model is employed to derive signal-based measures (features) that are then utilized in predicting the perceived level of reverberation. Such measures lack the excess of effort necessary for calculating perceptual measures; not to mention the variations in either stimuli or assessors that may cause such measures to be statistically insignificant. As a result, the automatic extraction of objective measurements that can be applied to predict the perceived level of reverberation become of vital significance. Consequently, this work is aimed at discovering measurements such as clarity, reverberance, and RT60 which can automatically be derived directly from audio data. These measurements along with labels from human listening tests are then forwarded to a machine learning system seeking to build a model to estimate the perceived level of reverberation, which is labeled by an expert, autonomously. The data has been labeled by an expert human listener for a unilateral set of files from arbitrary audio source types. By examining the results, it can be observed that the automatically extracted features can aid in estimating the perceptual rates.
Situated in the domain of urban sound scene classification by humans and machines, this research is the first step towards mapping urban noise pollution experienced indoors and finding ways to reduce its negative impact in peoples’ homes. We have recorded a sound dataset, called Open-Window, which contains recordings from three different locations and four different window states; two stationary states (open and close) and two transitional states (open to close and close to open). We have then built our machine recognition base lines for different scenarios (open set versus closed set) using a deep learning framework. The human listening test is also performed to be able to compare the human and machine performance for detecting the window state just using the acoustic cues. Our experimental results reveal that when using a simple machine baseline system, humans and machines are achieving similar average performance for closed set experiments.
Perceptual measures are usually considered more reliable than instrumental measures for evaluating the perceived level of reverberation. However, such measures are time consuming and expensive, and, due to variations in stimuli or assessors, the resulting data is not always statistically significant. Therefore, an (objective) measure of the perceived level of reverberation becomes desirable. In this paper, we develop a new method to predict the level of reverberation from audio signals by relating the perceptual listening test results with those obtained from a machine learned model. More specifically, we compare the use of a multiple stimuli test for within and between class architectures to evaluate the perceived level of reverberation. An expert set of 16 human listeners rated the perceived level of reverberation for a same set of files from different audio source types. We then train a machine learning model using the training data gathered for the same set of files and a variety of reverberation related features extracted from the data such as reverberation time, and direct to reverberation ratio. The results suggest that the machine learned model offers an accurate prediction of the perceptual scores.
Speech processing, automatic speech and speaker recognition are the major area of interests in the field of computational linguistics. Research and development of computer and human interaction, forensic technologies and dialogue systems have been the motivating factor behind this interest. In this paper, JSpeech is introduced, a multilingual corpus. This corpus contains 1332 hours of conversational speech from 47 different languages. This corpus can be used in a variety of studies, created from 106 public chat group the effect of language variability on the performance of speaker recognition systems and automatic language detection. To this end, we include speaker verification results obtained for this corpus using a state of the art method based on 3D convolutional neural network.
In recent years, speaker verification technologies have received an extensive amount of attention. Designing and developing machines that could communicate with humans are believed to be one of the primary motivations behind such developments. Speaker verification technologies are applied to numerous fields such as security, Biometrics, and forensics. In this paper, the authors study the effects of different languages on the performance of the automatic speaker verification (ASV) system. The MirasVoice speech corpus (MVSC), a bilingual English and Farsi speech corpus, is used in this study. This study collects results from both an I-vector based ASV system and a GMM-UBM based ASV system. The experimental results show that a mismatch between the enrolled data used for training and verification data can lead to a significant decrease in the overall system efficiency. This study shows that it is best to use an i-vector based framework with data from the English language used in the enrollment phase to improve the robustness of the ASV systems. The achieved results in this study indicate that this can narrow the degradation gap caused by the language mismatch.