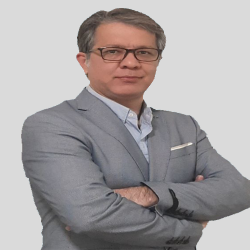
Dr Seyed Ahmad Soleymani
Publications
The advancement of mobile internet technology has created opportunities for integrating the Industrial Internet of Things (IIoT) and edge computing in smart manufacturing. These sustainable technologies enable intelligent devices to achieve high-performance computing with minimal latency. This paper introduces a novel approach to deploy edge computing nodes in smart manufacturing environments at a low cost. However, the intricate interactions among network sensors, equipment, service levels, and network topologies in smart manufacturing systems pose challenges to node deployment. To address this, the proposed sustainable game theory method identifies the optimal edge computing node for deployment to attain the desired outcome. Additionally, the standard design of Software Defined Network (SDN) in conjunction with edge computing serves as forwarding switches to enhance overall computing services. Simulations demonstrate the effectiveness of this approach in reducing network delay and deployment costs associated with computing resources. Given the significance of sustainability, cost efficiency plays a critical role in establishing resilient edge networks. Our numerical and simulation results validate that the proposed scheme surpasses existing techniques like shortest estimated latency first (SELF), shortest estimated buffer first (SEBF), and random deployment (RD) in minimizing the total cost of deploying edge nodes, network delay, packet loss, and energy consumption.
—In the Industrial Internet of Things (IIoT), outdoor electronic devices serve crucial roles across sectors, providing vital data for decision-making. However, their exposure to open outdoor environments makes them vulnerable to unauthorized access, physical theft, or compromise, endangering both the device and its data. Ensuring the security of outdoor devices and their data is thus critical. This study addresses data security in outdoor IIoT devices by supporting the encryption of all IIoT-related data in device memory. Accessing and retrieving this data requires operations on encrypted data. Hence, we introduce a Searchable Symmetric Encryption (SSE) scheme called MI3SE, which ensures each device's encryption key is unique and valid for a period based on the device's security sensitivity. Moreover, MI3SE meets key security requirements, including confidentiality, integrity, forward secrecy, and backward secrecy. It is specifically designed to mitigate physical compromise and query pattern analysis through a two-keyword query approach and withstand various attacks, as validated by rigorous security analysis. Comparative evaluations against benchmark schemes underscore the efficacy of MI3SE in terms of both security and performance. Moreover, comprehensive non-mathematical security analysis and simulation experiments affirm the enhanced accuracy and efficacy of MI3SE in securing sensitive data stored in outdoor IIoT devices.
—Edge computing improves the Internet of Vehicles (IoV) by offloading heavy computations from in-vehicle devices to high-capacity edge servers, typically roadside units (RSUs), to ensure rapid response times for intensive and latency-sensitive tasks. However, maintaining quality of service (QoS) remains challenging in dense urban settings and remote areas with limited infrastructure. To address this, we propose an SDN-driven model for UAV-assisted vehicular edge computing (VEC), integrating RSUs and UAVs to provide computing services and gather global network data via an SDN controller. UAVs serve as adaptable platforms for mobile edge computing (MEC), filling gaps left by traditional MEC frameworks in areas with high vehicle density or sparse network resources. An optimal offloading mechanism, designed to minimize the age of information (AoI) while balancing energy consumption and rental costs, is implemented through a soft actor-critic (SAC)-based algorithm that jointly optimizes UAV trajectory, user association, and offloading decisions. Experimental results demonstrate the model's superior performance, achieving up to 87.2% energy savings in energy-limited settings and a 50% reduction in time-sensitive scenarios, consistently outperforming traditional strategies across various task sizes. Index Terms—Vehicular edge computing, mobile edge computing , soft actor-critic, computation offloading, unmanned aerial vehicle, deep reinforcement learning, and age of information.
The paper presents a comprehensive investigation into a secure target-tracking system employing Unmanned Aerial Vehicles (UAVs) within urban environments. We introduce the Enhanced Multi-Agent Q-Learning (E-MAQL) algorithm designed to enhance target-tracking accuracy while minimizing energy consumption by UAVs. Additionally, we propose a robust security framework leveraging Deep Q-Networks (DQN) for Intrusion Detection Systems (IDS), alongside the implementation of Advanced Encryption Standard (AES) and Lightweight AES (LW-AES) protocols to ensure secure communication within the Open Radio Access Network (O-RAN) architecture. Our evaluations validate the effectiveness of E-MAQL in improving tracking performance and reducing energy consumption, while the proposed security framework demonstrates promising results in detecting and mitigating potential security threats within O-RAN-based systems. Furthermore, we measured the False Positive Ratio (FPR) of the IDS at 6%. Notably, our security framework significantly enhances the target-tracking system's accuracy by 33% when exposed to false injection data attacks, elevating accuracy from 53% to 86%.
In real-time medical monitoring systems, given the significance of medical data and disease symptoms, a secure and always-on connection with the medical centre over the public channels is essential. To this end, an edge-enabled Internet of Medical Things (IoMT) scheme is designed to improve flexibility and scalability of the network and provide seamless connectivity with minimum latency. The entities involved in such network are vulnerable to various attacks and can potentially be compromised. To address this issue, an authentication scheme comprised of digital signature and Authenticated Key Exchange (AKE) protocol is proposed which guarantees only authorized entities get access to the services available in the medical system. Moreover, to fulfill the privacy-preserving, each entity is mapped to a different pseudo-identity. The non-mathematical and performance analysis show that the proposed scheme is robust against various attacks such as impersonation and replay attacks.
Edge computing is a viable paradigm for supporting the Industrial Internet of Things deployment by shifting computationally demanding tasks from resource-constrained devices to powerful edge servers. In this study, mobile edge computing (MEC) services are provided for multiple ground mobile nodes (MNs) through a time-division multiple access protocol using the unmanned aerial vehicle (UAV)-enabled edge servers. Remotely controlled UAVs can serve as MEC servers due to their adaptability and flexibility. However, the current MEC approaches have proven ineffective in situations where the number of MNs rapidly increases, or network resources are sparsely distributed. Furthermore, suitable accessibility across wireless networks via MNs with an acceptable quality of service is a fundamental problem for conventional UAV-assisted communications. To tackle this issue, we present an optimized computation resource allocation model using cooperative evolutionary computation to solve the joint optimization problem of queuebased computation offloading and adaptive computing resource allocation. The developed method ensures the task computation delay of all MNs within a time block, optimizes the sum of MN’s accessibility rates, and reduces the energy consumption of the UAV and MNs while meeting task computation restrictions. Moreover, we propose a multilayer data flow processing system to make full use of the computational capability across the system. The top layer of the system contains the cloud centre, the middle layer contains the UAV-assisted MEC (U-MEC) servers, and the bottom layer contains the mobile devices. Our numerical analysis and simulation results prove that the proposed scheme outperforms conventional techniques such as equal offloading time allocation and straight-line flight.
Security and privacy of data-in-transit are critical issues in Industry 4.0, which are further amplified by the use of faster communication technologies such as 6G. Along with security issues, computation and communication costs, as well as data confidentiality, must be also accommodated. In this article, we design a cybertwin-based cloud-centric network architecture to improve the flexibility and scalability of 6G industrial networks. Cybertwin not only enables the deployment of advanced security solutions but also provides an always-on connection. However, the security of data-in-transit over wireless communication between users/things and cybertwin remains a concern. Hence, a privacy-preserving authentication scheme based on digital signature and authenticated key exchange protocol is designed to address the security concerns of data exchanged. In addition, we conduct a security analysis that proves that the scheme resists several attacks in the Industry 4.0 environment. Moreover, the evaluation performed confirmed the superiority of the proposed work comparing to the existing works.
This paper proposes a scheme for multiple un-manned aerial vehicles (UAVs) to track multiple targets in challenging 3-D environments while avoiding obstacle collisions. The scheme relies on Received-Signal-Strength-Indicator (RSSI) measurements to estimate and track target positions and uses a Q-Learning (QL) algorithm to enhance the intelligence of UAVs for autonomous navigation and obstacle avoidance. Considering the limitation of UAVs in their power and computing capacity, a global reward function is used to determine the optimal actions for the joint control of energy consumption, computation time, and tracking accuracy. Extensive simulations demonstrate the effectiveness of the proposed scheme, achieving accurate and efficient target tracking with low energy consumption.