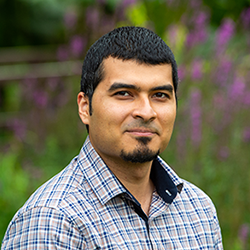
Dr Soroush Fatemifar
About
My research project
--
-
Publications
While the remarkable advances in face matching render face biometric technology more widely applicable, its successful deployment may be compromised by face spoofing. Recent studies have shown that anomaly-based face spoofing detectors offer an interesting alternative to the multiclass counterparts by generalising better to unseen types of attack. In this work, we investigate the merits of fusing multiple anomaly spoofing detectors in the unseen attack scenario via a Weighted Averaging (WA) and client-specific design. We propose to optimise the parameters of WA by a two-stage optimisation method consisting of Particle Swarm Optimisation (PSO) and the Pattern Search (PS) algorithms to avoid the local minimum problem. Besides, we propose a novel scoring normalisation method which could be effectively applied in extreme cases such as heavy-tailed distributions. We evaluate the capability of the proposed system on publicly available face anti-spoofing databases including Replay-Attack, Replay-Mobile and Rose-Youtu. The experimental results demonstrate that the proposed fusion system outperforms the majority of anomaly-based and state-of-the-art multiclass approaches.
To counteract spoofing attacks, the majority of recent approaches to face spoofing attack detection formulate the problem as a binary classification task in which real data and attack-accesses are both used to train spoofing detectors. Although the classical training framework has been demonstrated to deliver satisfactory results, its robustness to unseen attacks is debatable. Inspired by the recent success of anomaly detection models in face spoofing detection, we propose an ensemble of one-class classifiers fused by a Stacking ensemble method to reduce the generalisation error in the more realistic unseen attack scenario. To be consistent with this scenario, anomalous samples are considered neither for training the component anomaly classifiers nor for the design of the Stacking ensemble. To achieve better face-anti spoofing results, we adopt client-specific information to build both constituent classifiers as well as the Stacking combiner. Besides, we propose a novel 2-stage Genetic Algorithm to further improve the generalisation performance of Stacking ensemble. We evaluate the effectiveness of the proposed systems on publicly available face anti-spoofing databases including Replay-Attack, Replay-Mobile and Rose-Youtu. The experimental results following the unseen attack evaluation protocol confirm the merits of the proposed model.
Modern facial age estimation systems can achieve high accuracy when training and test datasets are identically distributed and captured under similar conditions. However, domain shifts in data, encountered in practice, lead to a sharp drop in accuracy of most existing age estimation algorithms. In this work, we propose a novel method, namely RAgE, to improve the robustness and reduce the uncertainty of age estimates by leveraging unlabelled data through a subject anchoring strategy and a novel consistency regularisation term. First, we propose an similarity-preserving pseudo-labelling algorithm by which the model generates pseudo-labels for a cohort of unlabelled images belonging to the same subject, while taking into account the similarity among age labels. In order to improve the robustness of the system, a consistency regularisation term is then used to simultaneously encourage the model to produce invariant outputs for the images in the cohort with respect to an anchor image. We propose a novel consistency regularisation term the noise-tolerant property of which effectively mitigates the so-called confirmation bias caused by incorrect pseudo-labels. Experiments on multiple benchmark ageing datasets demonstrate substantial improvements over the state-of-the-art methods and robustness to confounding external factors, including subject's head pose, illumination variation and appearance of expression in the face image.
The fusion of one-class classifiers (OCCs) has been shown to exhibit promising performance in a variety of machine learning applications. The ability to assess the similarity or correlation between the output of various OCCs is an important prerequisite for building of a meaningful OCCs ensemble. However, this aspect of the OCC fusion problem has been mostly ignored so far. In this paper, we propose a new method of constructing a fusion of OCCs with three contributions: (a) As a key contribution, enabling an OCC ensemble design using exclusively non anomalous samples, we propose a novel fitness function to evaluate the competency of OCCs without requiring samples from the anomalous class; (b) As a minor, but impactful contribution, we investigate alternative forms of score normalisation of OCCs, and identify a novel two-sided normalisation method as the best in coping with long tail non anomalous data distributions; (c) In the context of building our proposed OCC fusion system based on the weighted averaging approach, we find that the weights optimised using a particle swarm optimisation algorithm produce the most effective solution. We evaluate the merits of the proposed method on 15 benchmarking datasets from different application domains including medical, anti-spam and face spoofing detection. The comparison of the proposed approach with state-of-the-art methods alongside the statistical analysis confirm the effectiveness of the proposed model. (c) 2021 Elsevier Ltd. All rights reserved.
We consider a framework for taking into consideration the relative importance (ordinality) of object labels in the process of learning a label predictor function. The commonly used loss functions are not well matched to this problem, as they exhibit deficiencies in capturing natural correlations of the labels and the corresponding data. We propose to incorporate such correlations into our learning algorithm using an optimal transport formulation. Our approach is to learn the ground metric, which is partly involved in forming the optimal transport distance, by leveraging ordinality as a general form of side information in its formulation. Based on this idea, we then develop a novel loss function for training deep neural networks. A highly efficient alternating learning method is then devised to alternatively optimise the ground metric and the deep model in an end-to-end learning manner. This scheme allows us to adaptively adjust the shape of the ground metric, and consequently the shape of the loss function for each application. We back up our approach by theoretical analysis and verify the performance of our proposed scheme by applying it to two learning tasks, i.e. chronological age estimation from the face and image aesthetic assessment. The numerical results on several benchmark datasets demonstrate the superiority of the proposed algorithm.
The one-class anomaly detection approach has previously been found to be effective in face presentation attack detection, especially in an ____textit{unseen} attack scenario, where the system is exposed to novel types of attacks. This work follows the same anomaly-based formulation of the problem and analyses the merits of deploying ____textit{client-specific} information for face spoofing detection. We propose training one-class client-specific classifiers (both generative and discriminative) using representations obtained from pre-trained deep convolutional neural networks. Next, based on subject-specific score distributions, a distinct threshold is set for each client, which is then used for decision making regarding a test query. Through extensive experiments using different one-class systems, it is shown that the use of client-specific information in a one-class anomaly detection formulation (both in model construction as well as decision threshold tuning) improves the performance significantly. In addition, it is demonstrated that the same set of deep convolutional features used for the recognition purposes is effective for face presentation attack detection in the class-specific one-class anomaly detection paradigm.
•We develop a solution for the face spoofing detection problem by fusing multiple anomaly experts using Weighted Averaging(WA).•We propose a novel three-stage optimisation approach to improve the generalisation capability and accuracy of the WA fusion.•We define a new score normalisation approach to support multiple anomaly detectors fusion.•We define an effective criterion to prune the WA to achieve better classification result and generalisation performance.•We experimentally demonstrate that the proposed anomaly-based WA achieves superior performance over state-of-theart methods. Despite the recent improvements in facial recognition, face spoofing attacks can still pose a serious security threat to biometric systems. As fraudsters are coming up with novel spoofing attacks, anomaly-based detectors, compared to the binary spoofing attack counterparts, have certain generalisation performance advantages. In this work, we investigate the merits of fusing multiple anomaly classifiers using weighted averaging (WA) fusion. The design of the entire system is based on genuine-access data only. To optimise the parameters of WA, we propose a novel three-stage optimisation method with the following contributions: (a) A new hybrid optimisation method using Genetic Algorithm (GA) and Pattern Search (PS) to explore the weight space more effectively (b) a novel two-sided score normalisation method to improve the anomaly detection performance (c) a new ensemble pruning method to improve the generalisation performance. To further boost the performance of the proposed anomaly detection ensemble, we incorporate client-specific information to train the proposed model. We evaluate the capability of the proposed model on publicly available face spoofing databases including Replay-Attack, Replay-Mobile and Rose-Youtu. The experimental results demonstrate that the proposed WA fusion outperforms the state-of-the-art anomaly-based and multiclass approaches.
—Label distribution Learning (LDL) is the state-of-the-art approach to deal with a number of real-world applications , such as chronological age estimation from a face image, where there is an inherent similarity among adjacent age labels. LDL takes into account the semantic similarity by assigning a label distribution to each instance. The well-known Kullback–Leibler (KL) divergence is the widely used loss function for the LDL framework. However, the KL divergence does not fully and effectively capture the semantic similarity among age labels, thus leading to the sub-optimal performance. In this paper, we propose a novel loss function based on optimal transport theory for the LDL-based age estimation. A ground metric function plays an important role in the optimal transport formulation. It should be carefully determined based on underlying geometric structure of the label space of the application in-hand. The label space in the age estimation problem has a specific geometric structure, i.e. closer ages have more inherent semantic relationship. Inspired by this, we devise a novel ground metric function, which enables the loss function to increase the influence of highly correlated ages; thus exploiting the semantic similarity among ages more effectively than the existing loss functions. We then use the proposed loss function, namely γ–Wasserstein loss, for training a deep neural network (DNN). This leads to a notoriously computationally expensive and non-convex optimisa-tion problem. Following the standard methodology, we formulate the optimisation function as a convex problem and then use an efficient iterative algorithm to update the parameters of the DNN. Extensive experiments in age estimation on different benchmark datasets validate the effectiveness of the proposed method, which consistently outperforms state-of-the-art approaches.
One-class spoofing detection approaches have been an effective alternative to the two-class learners in the face presentation attack detection particularly in unseen attack scenarios. We propose an ensemble based anomaly detection approach applicable to one-class classifiers. A new score normalisation method is proposed to normalise the output of individual outlier detectors before fusion. To comply with the accuracy and diversity objectives for the component classifiers, three different strategies are utilised to build a pool of anomaly experts. To boost the performance, we also make use of the client-specific information both in the design of individual experts as well as in setting a distinct threshold for each client. We carry out extensive experiments on three face anti-spoofing datasets and show that the proposed ensemble approaches are comparable superior to the techniques based on the two-class formulation or class-independent settings.
Spoofing attacks on biometric systems can seriously compromise their practical utility. In this paper we focus on face spoofing detection. The majority of papers on spoofing attack detection formulate the problem as a two or multiclass learning task, attempting to separate normal accesses from samples of different types of spoofing attacks. In this paper we adopt the anomaly detection approach proposed in [1], where the detector is trained on genuine accesses only using one-class classifiers and investigate the merit of subject specific solutions. We show experimentally that subject specific models are superior to the commonly used client independent method. We also demonstrate that the proposed approach is more robust than multiclass formulations to unseen attacks.