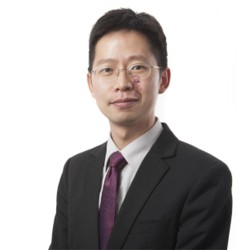
Professor Tao Chen
About
Biography
With the Associate Vice-President, International role, I am responsible for delivering the University's global engagement strategy with a focus on transnational education (TNE), through a pipeline of projects with diverse internal stakeholders and external partners. I also provide guidance to, and facilitate, our international activities in partnership development, brand building, student recruitment, collaborative research, and student/staff mobility.
I am a computational and data scientist, working on AI and digital technologies for cross-disciplinary engineering applications. These include computer modelling of chemical processes using multiscale mechanisms and machine learning, as well as model-based chemical product design and process optimisation. Hence my work can be broadly viewed as 'Digital Chemical Engineering'.
Our activities cut across both methodological research (new computational and systems engineering methods) and applications. We have used computational approaches to help address product design and process operation challenges in areas of pharmaceuticals, healthcare, cosmetics, food, agrochemicals, water processing, steelmaking, amongst others, partnering with industry through collaborative research and R&D consultancy. I currently lead a group of 8 doctoral and 2 postdoctoral researchers. I have been teaching small (ca. 10 students) and large (270+) classes, at both undergraduate and postgraduate levels. As an application-driven computational scientist, I am always keen to explore new fronts under the banner of Digital Chemical Engineering.
I trained as a control engineer with BEng (2000) and MEng (2002) degrees both from Department of Automation, Tsinghua University, China, and PhD (Chemical Engineering / Process Systems Engineering) from Newcastle University in 2006, UK. Prior to joining University of Surrey in 2011 as Lecturer, I worked as Research Associate at Newcastle University (2006-2007), then Assistant Professor at Nanyang Technological University, Singapore (2008-2010).
Areas of specialism
ResearchResearch interests
Under the banner of Digital Chemical Engineering, our work is mainly about computer modelling of chemical processes using multiscale mechanisms and machine learning, as well as model-based chemical product design and process optimisation.
We work on both methodological developments and applications. For more details, please refer to our projects and publications.
Our current projects are mainly on two fronts:
- Skin absorption and biomarker extraction: computational modelling of skin penetration/extraction pathways, topical product formulations, chemical and physical means to improve skin penetration, measurement methods and devices, and risk assessment due to skin exposure to contaminants.
- Process operation resilience: fault diagnosis / predictive maintenance, control and optimisation for resilience, integration of machine learning with mechanistic modelling, with applications to biologics production, controlled environment agriculture, radiotherapy and other processes.
As an application-driven computational scientist, I am always keen to explore new fronts.
I am also affiliated with a number of multi-disciplinary research themes, which have provided the opportunity to collaborate with colleagues in various disciplines of the university:
- Lifelong Health, a University Strategic Research Theme
- Health and Food, a research group between the Department of Chemical & Process Engineering and the Department of Chemistry
Research projects
Selected grantsTotal: £2m as principal investigator, £4m as co-investigator; also about £200k consultancy contracts.
A multiplexed micro-suction biomarker extraction device to understand atopic eczema in babiesEPSRC (EP/X013294/1), £453,816, Jan 2023 - Dec 2025, Principal Investigator
Soilless cultivation for rapid bioenergy willowBEIS (Department for Business, Energy and Industrial Strategy) Biomass Feedstocks Innovation Programme, £4m, May 2022 - Mar 2025, Co-investigator (in charge of digital twin development and process optimisation)
EPSRC (EP/S021159/1), £340,223, October 2019 - September 2022, Principal Investigator
BBSRC (BB/S020896/1), £457,264, Feb 2019 - Feb 2022, Principal Investigator
BBSRC Industrial CASE, £99,034 (BBSRC) + £50,000 (Unilever), 09/2020-09/2024, Principal Investigator
NC3Rs (NC/T001720/1) studentship grant, £90,000, October 2020 - September 2023, Principal Investigator
Innovate UK, £68,071, 02/2021-07/2022, Principal Investigator
Bioequivalence of Topical Products: Elucidating the Thermodynamic and Functional Characteristics of Compositionally Different Topical FormulationsUS Food and Drug Administration (1U01FD006496-01), USD 1,250,000 (USD 110,000 to Surrey), Sep 2018 - Aug 2023, Co-Investigator (Modelling Lead & Surrey Principal Investigator)
Dermal absorption in vitro – Assessment of study variability and harmonizationEuropean Crop Protection Association, Euro 77,871, Jan - Dec 2019, Principal Investigator.
Quantifying the Impact of Dosimetry Quality Assurance on Clinical Outcomes of RadiotherapyNational Physical Laboratory, £50,000, 01/01/2015-31/12/2018, Principal Investigator.
The Taeda Tech Project is a collaborative demonstration project led by the University of Surrey that is committed to developing a novel aeroponic technology which can rapidly grow woody crops.
Research interests
Under the banner of Digital Chemical Engineering, our work is mainly about computer modelling of chemical processes using multiscale mechanisms and machine learning, as well as model-based chemical product design and process optimisation.
We work on both methodological developments and applications. For more details, please refer to our projects and publications.
Our current projects are mainly on two fronts:
- Skin absorption and biomarker extraction: computational modelling of skin penetration/extraction pathways, topical product formulations, chemical and physical means to improve skin penetration, measurement methods and devices, and risk assessment due to skin exposure to contaminants.
- Process operation resilience: fault diagnosis / predictive maintenance, control and optimisation for resilience, integration of machine learning with mechanistic modelling, with applications to biologics production, controlled environment agriculture, radiotherapy and other processes.
As an application-driven computational scientist, I am always keen to explore new fronts.
I am also affiliated with a number of multi-disciplinary research themes, which have provided the opportunity to collaborate with colleagues in various disciplines of the university:
- Lifelong Health, a University Strategic Research Theme
- Health and Food, a research group between the Department of Chemical & Process Engineering and the Department of Chemistry
Research projects
Total: £2m as principal investigator, £4m as co-investigator; also about £200k consultancy contracts.
EPSRC (EP/X013294/1), £453,816, Jan 2023 - Dec 2025, Principal Investigator
BEIS (Department for Business, Energy and Industrial Strategy) Biomass Feedstocks Innovation Programme, £4m, May 2022 - Mar 2025, Co-investigator (in charge of digital twin development and process optimisation)
EPSRC (EP/S021159/1), £340,223, October 2019 - September 2022, Principal Investigator
BBSRC (BB/S020896/1), £457,264, Feb 2019 - Feb 2022, Principal Investigator
BBSRC Industrial CASE, £99,034 (BBSRC) + £50,000 (Unilever), 09/2020-09/2024, Principal Investigator
NC3Rs (NC/T001720/1) studentship grant, £90,000, October 2020 - September 2023, Principal Investigator
Innovate UK, £68,071, 02/2021-07/2022, Principal Investigator
US Food and Drug Administration (1U01FD006496-01), USD 1,250,000 (USD 110,000 to Surrey), Sep 2018 - Aug 2023, Co-Investigator (Modelling Lead & Surrey Principal Investigator)
European Crop Protection Association, Euro 77,871, Jan - Dec 2019, Principal Investigator.
National Physical Laboratory, £50,000, 01/01/2015-31/12/2018, Principal Investigator.
The Taeda Tech Project is a collaborative demonstration project led by the University of Surrey that is committed to developing a novel aeroponic technology which can rapidly grow woody crops.
Supervision
Postgraduate research supervision
Current research fellows
- Dr Yuqing Xia, research topic 'digital twin for optimising biomass growth in controlled environment', BEIS funded
- Anukrati Goel, research topic 'A multiplexed micro-suction biomarker extraction device to understand atopic eczema in babies', EPSRC funded.
Current doctoral students
- Yu Zhang, research topic 'digital twin for topical formulation design', (co-supervisor: Dr Dimitrios Tsaoulidis)
- Yongrui Xiao, research topic 'robotic and digital system for topical formulation design', (co-supervisor: Dr Dimitrios Tsaoulidis)
- Lingwei Jiang, research topic 'nitrogen balance and control in controlled environment aquaponics' (co-supervisor: Dr Bing Guo)
- Xiaoyang Luan, research topic 'surrogate modelling of hazardous gas leakage' (co-supervisor: Dr Michael Short, Prof Bin Zhang, Surrey - Nanjing Tech University joint PhD programme)
- Jinqi Yang, research topic 'control and optimisation of vertical farming' (co-supervisor: Prof Guoping Lian)
Former research fellows
- Dr Duo Zhang, research topic 'end-to-end workflow modelling and simulation', industry funded, 2021-2023.
- Dr Lucy Coleman, research topic 'topical formulations and dermal absorption', industry funded, 2021-2023.
- Dr Daniel Sebastia Saez, research topic 'modelling drug delivery into the skin', EPSRC funded, 2019-2022.
- Dr Hui Li, research topic 'data analytics and optimisation in aquaculture', BBSRC funded, 2019-2021.
- Dr Stella Totti, research topic 'skin exposure to and absorption of chemicals', industry funded, 2019-2021.
- Dr Olumayowa Kajero, research topic 'modelling evaporation of topical formulations', EPSRC GCRF institutional grant funded, 2016-2017.
- Dr Agnieszka Lemanska, research topic 'machine learning for patient reported outcome measures', EPSRC Impact Acceleration Account funded, 2011-2012.
- Dr Wangdong Ni, research topic 'data driven modelling of wastewater processing', industry funded, Nanyang Technological University, 2010.
Former doctoral students
- Anukrati Goel, research topic 'integrating in silico modelling and stimulated Raman spectroscopy for understanding skin penetration' (co-supervisor: Dr Natalie Belsey)
- Samadhi Silva, research topic 'impact of formulation on the bioequivalence of topical dermatological products' (co-supervisor: Prof Guoping Lian)
- Ben Deacon (2025), research topic 'tissue specific analysis of dermal absorption' (co-supervisor: Prof Guoping Lian)
- Yufan Liu (2024), Chemical safety through the lens of omics: Machine learning approaches for pathway analysis and predictive modelling (co-supervisor: Prof Guoping Lian)
- Xueliang Zhu (2003), Accidental release of superheated liquids: flashing jet breakup and spray formation, (co-supervisor: Dr Lian Liu, Prof Xuhai Pan)
- Arkadiusz Wojtasik (2003), Multivariate Data Analytics for Radiotherapy Quality Assurance, (co-supervisor: Prof Andrew Nisbet, Prof Catharine Clark)
- Babatunde Osho (2022), Multivariate Analysis of the Environmental Impact of Traffic Density on Air Quality in Lagos, Nigeria, (co-supervisor: Prof Rex Thorpe)
- Paschalia Mavrou (2022), Moisture migration in multicomponent food systems: Investigating the effect of temperature, (co-supervisor: Prof Guoping Lian)
- Weijun Li (2021), Integrating simulation and data-driven modelling for process fault diagnosis, (co-supervisor: Prof Sai Gu)
- Lucy Coleman (2021), In silico simulation of dermal xenobiotic metabolism and skin sensitisation, (co-supervisor: Prof Guoping Lian)
- Anthony Wu (2019), Process Modelling with Limited Data: Application Studies in Waste Treatment and Pharmaceutical Processes, (co-supervisors: Dr Matthew McEwan, Dr Franjo Cecelja)
- Matthew Bolt (2018). Evidence based assessment of the clinical impact of dose variations arising in the clinical radiotherapy dosimetry chain, Department of Chemical and Process Engineering, University of Surrey, UK (co-supervisors: Prof Andrew Nisbet, Dr Catharine Clark)
- Panayiotis Kattou (2018). Mathematical modelling of transdermal permeation of chemicals with special focus on the hair follicle pathway, Department of Chemical and Process Engineering, University of Surrey, UK (co-supervisor: Prof Guoping Lian)
- Olumayowa Kajero (2017). Meta-model assisted calibration of computational fluid dynamics simulation models, Department of Chemical and Process Engineering, University of Surrey, UK (co-supervisor: Prof Rex Thorpe)
- Imran Tariq (2016). Adaptive modelling of tumour volume dynamics under radiotherapy, Department of Chemical and Process Engineering, University of Surrey, UK (co-supervisor: Prof Norman Kirkby)
- Wenjin Yan (2012). Model-aided catalyst design, optimization and analysis, School of Chemical and Biomedical Engineering, Nanyang Technological University, Singapore (co-supervisor: Prof Yanhui Yang)
- Ke Wang (2012). Chemometric techniques for multivariate calibration and their application in spectroscopic sensors, School of Chemical and Biomedical Engineering, Nanyang Technological University, Singapore (co-supervisor: Prof Yanhui Yang)
Teaching
I'm currently teaching two Year 2 modules: Computation for Chemical Engineers (20%) and Transfer Processes (70%), and one MSc module Advanced Process Control (70%).
I supervise 4~6 MSc dissertation projects and 1~2 MEng research projects every year, in the areas of process modelling, simulation, optimisation and control, sometimes with experimental elements to support modelling activities.
In the past I have taught a wide range of modules at both undergraduate and postgraduate levels at the University of Surrey:
- Transferable Skills and Laboratory Skills, Year 1 BEng/MEng
- Control, Year 2 BEng/MEng
- Multidisciplinary Design Project, Year 4 MEng
- Supply Chain Management, MSc and Year 4 MEng
- Advanced Process Control, MSc
- Process Modelling and Simulation, MSc and Year 4 MEng
At Nanyang Technological University (2008-2010) I taught the following modules:
- Chemical and Biomedical Process Control and Dynamics, Year 3 BEng
- Modelling Chemical & Biological Processes, Year 4 BEng
- Mathematics for Engineers A, Year 1 BEng
- Chemical Engineering Laboratories, Year 2 and 3 BEng
- Introduction to Programming, Year 1 BEng
- Advanced Mathematics for Chemical Engineering, Year 1 PhD
Sustainable development goals
My research interests are related to the following:
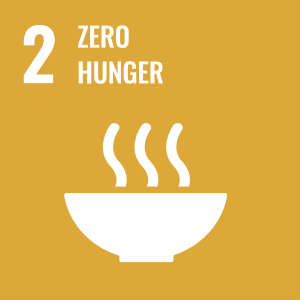
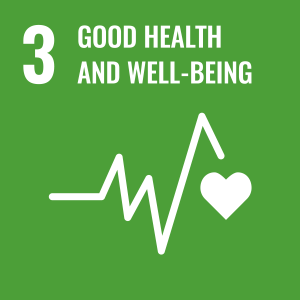
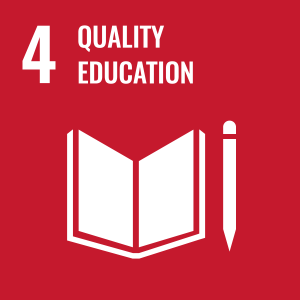
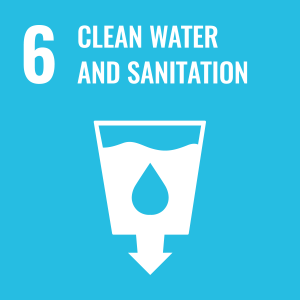
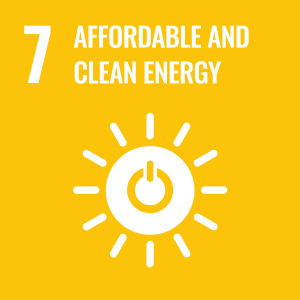
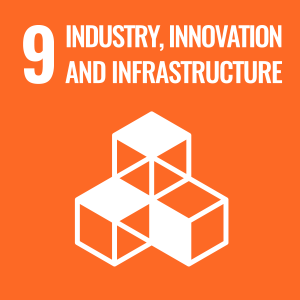
Publications
Highlights
Coleman L, Lian G, Glavin S, Sorrell I, Chen T. In silico simulation of simultaneous percutaneous absorption and xenobiotic metabolism: Model development and a case study on aromatic amines, Pharmaceutical Research, 37: 241, 2020.
Li W, Gu S, Zhang X, Chen T, 2020. Transfer learning for process fault diagnosis: Knowledge transfer from simulation to physical processes. Computers & Chemical Engineering, 139: 106904
Sebastia-Saez D, Benaouda F, Lim C, Lian G, Jones S, Chen T, Cui L, 2021. Numerical analysis of the strain distribution in skin domes formed upon the application of hypobaric pressure, Skin Research and Technology, in press.
Kattou, P., Lian, G., Glavin, S., Sorrell, I., Chen, T., 2017. Development of a Two-Dimensional Model for Predicting Transdermal Permeation with the Follicular Pathway: Demonstration with a Caffeine Study. Pharm Res 34, 2036–2048. https://doi.org/10.1007/s11095-017-2209-0
Kajero, O.T., Thorpe, R.B., Chen, T., Wang, B., Yao, Y., 2016. Kriging meta-model assisted calibration of computational fluid dynamics models. AIChE J. 62, 4308–4320. https://doi.org/10.1002/aic.15352
Greenhouse aquaponics system (GHAP) improves productivity by harmonizing internal environments. Keeping a suitable air temperature of GHAP is essential for the growth of plant and fish. However, the disturbance of various environmental factors and the complexity of temporal patterns affect the accuracy of the microclimate time-series forecasting. This work proposed an Adaptive Time Pattern Network (ATPNet) to predict GHAP air temperature, which consists of deep temporal feature (DTF) module, multiple temporal pattern convolution (MTPC) module, and spatial attention mechanism (SAM) module. The DTF module has a wide sensory range and can capture information over a long-time span. The MTPC module is designed to improve model response performance by exploiting the effective temporal information of different environmental factors at different times. At the same time, the SAM can explore the correlations among different environmental factors. The ATPNet found that air temperature of GHAP has a strong correlation with other temperature-related parameters (external air temperature, external soil temperature, and water temperature). Compared with the best performance of three baseline models (multilayer perceptron (MLP), recurrent neural network (RNN), and Temporal Convolutional Network (TCN)), the ATPNet enhanced overall prediction performance for the following 24 h by 7.44% for root mean squared error (RMSE), 2.53% for mean absolute error (MAE), and 3.15% for mean absolute percentage error (MAPE), respectively.
The mechanistic understanding of skin penetration underpins the design, efficacy and risk assessment of many high-value products including functional personal care products, topical and transdermal drugs. Stimulated Raman scattering (SRS) microscopy, a label free chemical imaging tool, combines molecular spectroscopy with submicron spatial information to map the distribution of chemicals as they penetrate the skin. However, the quantification of penetration is hampered by significant interference from Raman signals of skin constituents. This study reports a method for disentangling exogeneous contributions and measuring their permeation profile through human skin combining SRS measurements with chemometrics. We investigated the spectral decomposition capability of multivariate curve resolution - alternating least squares (MCR-ALS) using hyperspectral SRS images of skin dosed with 4-cyanophenol. By performing MCR-ALS on the fingerprint region spectral data, the distribution of 4-cyanophenol in skin was estimated in an attempt to quantify the amount permeated at different depths. The reconstructed distribution was compared with the experimental mapping of C---N, a strong vibrational peak in 4-cyanophenol where the skin is spectroscopically silent. The similarity between MCR-ALS resolved and experimental distribution in skin dosed for 4 h was 0.79 which improved to 0.91 for skin dosed for 1 h. The correlation was observed to be lower for deeper layers of skin where SRS signal intensity is low which is an indication of low sensitivity of SRS. This work is the first demonstration, to the best of our knowledge, of combining SRS imaging technique with spectral unmixing methods for direct observation and mapping of the chemical penetration and distribution in biological tissues.
Detecting anomalies in manufacturing processes is crucial for ensuringsafety. However, noise significantly undermines the reliability of data-drivenanomaly detection models. To address this challenge, we propose a slowfeature-constrained decomposition autoencoder (SFC-DAE) for anomaly detec-tion in noisy scenarios. Considering that the process can exhibit both long-termtrends and periodic properties, the process data is decomposed into trends andcycles. The repetitive information is mitigated by slicing and randomly mask-ing certain trends and cycles. Dependencies among slices are constructed toextract intrinsic information, while high-frequency noise is reduced using a slowfeature-constrained loss. Anomalies are detected and localized through a recon-struction error strategy. The effectiveness of SFC-DAE is demonstrated usingdata from a sugar factory and a secure water treatment system.
Deep learning-based just-in-time soft sensors effectively handle the strong nonlinearity of complex process industry, but their implementation faces significant challenges in interpretability and time cost. Hence, a just-in-time soft sensor based on spatiotemporal graph decoupling is proposed. To decrease time cost, it employs a global-local modeling strategy: pre-training on all historical data to build a global model, and fine-tuning with relevant samples to deliver a local model. To enhance interpretability, couplings that reflect how variables interact with each other in spatiotemporal dimensions are constructed, conforming to prior knowledge, to guide the graph neural network as a global model during pre-training. The global model decouples variables to quantify their influence as intrinsic information, enabling a clearer understanding of how each variable contributes to the prediction. Following the intrinsic information, relevant samples are then selected with the preset relevance metric to fine-tune the global model. Finally, two industrial cases demonstrate this model's low runtime, effectiveness, and physical consistency from the perspectives of underlying physics.
Data-driven process monitoring is an effective approach to assure safe operation of modern manufacturing and energy systems, such as thermal power plants being studied in this work. Industrial processes are inherently dynamic and need to be monitored using dynamic algorithms. Mainstream dynamic algorithms rely on concatenating current measurement with past data. This work proposes a new, alternative dynamic process monitoring algorithm, using dot product feature analysis (DPFA). DPFA computes the dot product of consecutive samples, thus naturally capturing the process dynamics through temporal correlation. At the same time, DPFA's online computational complexity is lower than not just existing dynamic algorithms, but also classical static algorithms (e.g., principal component analysis and slow feature analysis). The detectability of the new algorithm is analyzed for three types of faults typically seen in process systems: sensor bias, process fault and gain change fault. Through experiments with a numerical example and real data from a thermal power plant, the DPFA algorithm is shown to be superior to the state-of-the-art methods, in terms of better monitoring performance (fault detection rate and false alarm rate) and lower computational complexity.
Purpose. This study investigates in silico the contribution of the hair follicle to the overall dermal permeability of small molecules, as published experimental work provides inconclusive information on whether the follicular route favours the permeation of hydrophobic or hydrophilic permeants. Method. A study is conducted varying physico-chemical parameters of permeants such as lipophilicity, molecular weight and protein binding. The simulated data is compared to published experimental data to discuss how those properties can modulate the contribution of the hair follicle to the overall dermal permeation. Results. The results indicate that the contribution of the follicular route to dermal permeation can range from negligible to notable depending on the combination of lipophilic/hydrophilic properties of the substance filling the follicular route and the permeant. Conclusion. Characterisation of the substance filling the follicular route is required for analysing the experimental data of dermal permeation of small molecules, as changes between in vivo and in vitro due to handling of samples and cessation of vital functions can modify the contribution of the follicular route to overall dermal permeation, hence hindering data interpretation.
Comprehensive analysis of multi-omics data can reveal alterations in regulatory pathways induced by cellular exposure to chemicals by characterizing biological processes at the molecular level. Data-driven omics analysis, conducted in a dosedependent or dynamic manner, can facilitate comprehending toxicity mechanisms. This study introduces a novel multi-omics data analysis designed to concurrently examine dose-dependent and temporal patterns of cellular responses to chemical perturbations. This analysis, encompassing preliminary exploration, pattern deconstruction, and network reconstruction of multi-omics data, provides a comprehensive perspective on the dynamic behaviors of cells exposed to varying levels of chemical stimuli. Importantly, this analysis is adaptable to any number of any omics layers, including site-specific phosphoproteomics. We implemented this analysis on multiomics data obtained from HepG2 cells exposed to a range of caffeine doses over varying durations and identified six response patterns, along with their associated biomolecules and pathways. Our study demonstrates the effectiveness of the proposed multi-omics data analysis in capturing multi-dimensional patterns of cellular response to chemical perturbation, enhancing understanding of pathway regulation for chemical risk assessment.
The rich flavors and antioxidant properties within Maofeng tea are mainly attributable to numerous secondary metabolites and thus may be related to quality. Motivated by this finding, our study presents a sparse representation (SR) scheme to analyze the content of various secondary metabolites and thus identify discriminating compounds (DCs) for the modelling of Maofeng tea quality. We first identified the DCs in terms of an interpretable sparse recovery strategy with LASSO regression. The optimal regularization term was estimated by a specific Karush–Kuhn–Tucker (KKT) optimal condition. Then, qualitative analysis models were trained with screened DCs and utilized to predict the quality of unseen Maofeng samples. For this purpose, 96 Maofeng samples of 6 different quality grades were collected, and standardized stoichiometry techniques determined 21 quality-related bioactive compounds and empirical quality indicators. The experimental results show that epigallocatechin (EGC), epicatechin (EC), gallocatechin gallate (GCG) and total catechins (TC) were identified as significant discriminating features, and the KNN algorithm provided the best assessment accuracy of 95.79%. Overall, the result demonstrates the superior performance of benchmarks for enhancing the reliable prediction of Maofeng tea quality, not only prediction accuracy but also providing interpretable assessment. •Discriminating compound analysis (DCA) was devised to predict Maofeng quality.•Sparse representation strategy enhances the model accuracy and interpretability.•Karush–Kuhn–Tucker condition was performed to enhance the model robustness.•The superiority of DCA over existing method is demonstrated through experiment.•KNN model yielded best quality prediction accuracy with proposed DCA method.
Epoxy resins (EPs) have limited comprehensive applications because of their flammability. This current work combines 9,10-dihydro-9-oxa-10-phosphaphenanthrene-10-oxide, and a ligand of a metal–organic framework with zeolites prepared from electrolytic manganese residue as flame retardants (D-CoZ-BDC-1), which is reported for the first time. The flame retardant EP composite (EP/D-CoZ-BDC-1) exhibited a limiting oxygen index of 31.0%. The peak heat release rate, total heat release, peak smoke release rate, total smoke release, CO yield, and CO2 yield of EP/D-CoZ-BDC-1 were reduced by 63.4%, 48.9%, 52.8%, 60.1%, 30%, and 48.7%, respectively, when compared with those for EP. The synergistic effects of pyrolysis in the formation of Co3O4, the phospholipid biphenyl structure, Al2O3 and SiO2 in the condensed phase, and PO· in the gas phase gave excellent fire resistance. The proposed method not only produced highly efficient EP flame retardants but could also provide ideas for the design of zeolite-based flame retardants for wider applications.
Graphitic carbon nitride (GCN) has been recognized as a potential flame retardant (FR) due to its high thermal stability and nitrogen richness. Previous work has been limited to hybridization without involving covalent modification. Here, we developed a facile covalent modification approach to polycondensation that can chelate with metal ions (PCNOH–CuCo) from GCN. Structural and mechanical property characterization confirmed the ability of PCNOH–CuCo to be uniformly dispersed in the epoxy resin (EP). Fire tests showed excellent fire resistance of EP with 10 wt% PCNOH–CuCo (EP/10PCNOH–CuCo), including a limiting oxygen index of EP/10PCNOH–CuCo up to 31.5%, and the reduction in the peak heat release rate, total heat release, peak smoke production, total smoke production peak CO production, and peak CO2 production of 47.9%, 37.5%, 20%, 44.5%, 30.9%, and 42.5%, respectively. This work provides a solution for the fabrication of GCN-based FRs and their derived metal-doped FRs. [Display omitted] •A polycondensation synthesized from CNOH.•Two methods of preparing CNOH were compared.•Excellent compatibility between PCNOH–CuCo and EP.•The LOI of the EP/10PCNOH–CuCo reached 31.5%.
Fish growth prediction provides important information for optimising production in aquaculture. Fish usually exhibit different growth characteristics due to the variations in the environment, the equipment used in different fish workshops and inconsistent application by operators of empirical rules varying from one pond to another. To address this challenge, the aim of this study is to develop an adaptive fish growth prediction method in response to feeding decision. Firstly, the practical operational experience in historical feeding decisions for different fish weights is extracted to establish the feeding decision model. Then, a fish weight prediction model is established by regression analysis methods based on historical fish production data analysis. The feeding decision model is integrated as the input information of the fish weight prediction model to obtain fish weight prediction. Furthermore, an adaptive fish growth prediction strategy is proposed by continuously updating model parameters using new measurements to adapt to specific characteristics. The proposed adaptive fish growth prediction method with empirical Empirical knowledge extraction is evaluated by the collected production data of spotted knifejaw (Oplegnathus punctatus). The results show that established models can achieve a good balance between goodness-of-fit and model complexity, and the adaptive prediction method can adapt to specific fish pond's characteristics and provide a more effective way to increase fish weight prediction accuracy. The proposed method provides an important contribution to achieving adaptive fish growth prediction in a real time from the view of aquaculture practice for spotted knifejaw. (C) 2021 IAgrE. Published by Elsevier Ltd. All rights reserved.
Data-driven soft sensors in the process industry, whilst intensively investigated, struggle to handle unforeseen disruptions and operating changes not covered in the training data. Incorporating physical knowledge, such as mass/energy balances and reaction mechanisms, into a data-driven model is a potential remedy. In this study, a physical-anchored graph learning (PAGL) soft sensor is proposed, integrating process variable causality and mass balances. Knowledge-derived causality is further supplemented by mining dependencies from data. PAGL uses causality and mass balance as physical anchors to predict key indicators and evaluate whether the prediction logic aligns with physical principles, ensuring physical consistency in inference. The case study on wastewater treatment demonstrates PAGL's interpretability and reliability, maintaining physical consistency instead of acting as a black box.
Data-driven soft sensors in the process industry, whilst intensively investigated, struggle to handle unforeseen disruptions and operating changes not covered in the training data. Incorporating physical knowledge, such as mass/energy balances and reaction mechanisms, into a data-driven model is a potential remedy. In this study, a physical-anchored graph learning (PAGL) soft sensor is proposed, integrating process variable causality and mass balances. Knowledge-derived causality is further supplemented by mining dependencies from data. PAGL uses causality and mass balance as physical anchors to predict key indicators and evaluate whether the prediction logic aligns with physical principles, ensuring physical consistency in inference. The case study on wastewater treatment demonstrates PAGL’s interpretability and reliability, maintaining physical consistency instead of acting as a black box.
Topical skin products aim to address aesthetic, protective, and/or therapeutic needs through interaction with the human epidermal system. Traditionally, formulation development relies on empirical knowledge and trial-and-error experiments. In this paper, we introduced the Bayesian optimisation method and compared it with the traditional response surface methodology (RSM) for topical drug formulation. The objective was to optimise the formulation composition of ibuprofen gel-like to achieve a maximum flux through in vitro permeation tests (IVPTs). As a model system, poloxamer 407, ethanol, and propylene glycol (PG) were selected as the key excipients, whose concentrations were optimised. Strat-M membrane, serving as a surrogate for human skin, and Franz cell diffusion were employed in IVPTs. Two sets of experiments were conducted under identical conditions for 30 h. Under the RSM approach, the optimised ibuprofen gel-like formulation was identified with a poloxamer 407: ethanol: PG ratio of 20:20:10, achieving a measured permeation flux of 11.28 ± 0.35 μg cm−2h−1. In comparison, Bayesian optimisation, after four iterations, yielded an optimised formulation with a ratio of 20.95:19.44:12.14, resulting in a permeation flux of 14.15 ± 0.77 μg cm−2h−1. These findings highlight the potential of Bayesian optimisation as an effective tool for improving topical drug formulations.
Fish feeding intensity assessment (FFIA) aims to evaluate fish appetite changes during feeding, which is crucial in industrial aquaculture applications. Existing FFIA methods are limited by their robustness to noise, computational complexity, and the lack of public datasets for developing the models. To address these issues, we first introduce AV-FFIA, a new dataset containing 27,000 labeled audio and video clips that capture different levels of fish feeding intensity. Then, we introduce multi-modal approaches for FFIA by leveraging the models pre-trained on individual modalities and fused with data fusion methods. We perform benchmark studies of these methods on AV-FFIA, and demonstrate the advantages of the multi-modal approach over the single-modality based approach, especially in noisy environments. However, compared to the methods developed for individual modalities, the multimodal approaches may involve higher computational costs due to the need for independent encoders for each modality. To overcome this issue, we further present a novel unified mixed-modality based method for FFIA, termed as U-FFIA. U-FFIA is a single model capable of processing audio, visual, or audio-visual modalities, by leveraging modality dropout during training and knowledge distillation using the models pre-trained with data from single modality. We demonstrate that U-FFIA can achieve performance better than or on par with the state-of-the-art modality-specific FFIA models, with significantly lower computational overhead, enabling robust and efficient FFIA for improved aquaculture management. To encourage further research, we have released the AV-FFIA dataset, the pre-trained model and codes at https://github.com/FishMaster93/U-FFIA. Note to Practitioners-Feeding is one of the most important costs in aquaculture. However, current feeding machines usually operate with fixed thresholds or human experiences, lacking the ability to automatically adjust to fish feeding intensity. FFIA can evaluate the intensity changes in fish appetite during the feeding process and optimize the control strategies of the feeding machine to avoid inadequate feeding or overfeeding, thereby reducing the feeding cost and improving the well-being of fish in industrial aquaculture. The existing methods have mainly exploited single-modality data, and have a high sensitivity to input noise. Using video and audio offers improved chances to address the challenges brought by various environments. However, compared with processing data from single modalities, using multiple modalities simultaneously often involves increased computational resources, including memory, processing power, and storage. This can impact system performance and scalability. To address these issues, we focus on the efficient unified model, which is capable of processing both multimodal and single-modal input. Our proposed model achieved state-of-the-art (SOTA) performance in FFIA with high computational efficiency.
Rolling mill reheating furnaces are widely used in large-scale iron and steel plants, the efficient operation of which has been hampered by the complexity of the combustion mechanism. In this paper, a soft-sensing method is developed for modeling and predicting combustion efficiency since it cannot be measured directly. Statistical methods are utilized to ascertain the significance of the proposed derived variables for the combustion efficiency modeling. By employing the nonnegative garrote variable selection procedure, an adaptive scheme for combustion efficiency modeling and adjustment is proposed and virtually implemented on a rolling mill reheating furnace. The results show that significant energy saving can be achieved when the furnace is operated with the proposed model-based optimization strategy.
The effect of COVID-19 infection on the human metabolome has been widely reported, but to date all such studies have focused on a single wave of infection. COVID-19 has generated numerous waves of disease with different clinical presentations, and therefore it is pertinent to explore whether metabolic disturbance changes accordingly, to gain a better understanding of its impact on host metabolism and enable better treatments. This work used a targeted metabolomics platform (Biocrates Life Sciences) to analyze the serum of 164 hospitalized patients, 123 with confirmed positive COVID-19 RT-PCR tests and 41 providing negative tests, across two waves of infection. Seven COVID-19-positive patients also provided longitudinal samples 2–7 months after infection. Changes to metabolites and lipids between positive and negative patients were found to be dependent on collection wave. A machine learning model identified six metabolites that were robust in diagnosing positive patients across both waves of infection: TG (22:1_32:5), TG (18:0_36:3), glutamic acid (Glu), glycolithocholic acid (GLCA), aspartic acid (Asp) and methionine sulfoxide (Met-SO), with an accuracy of 91%. Although some metabolites (TG (18:0_36:3) and Asp) returned to normal after infection, glutamic acid was still dysregulated in the longitudinal samples. This work demonstrates, for the first time, that metabolic dysregulation has partially changed over the course of the pandemic, reflecting changes in variants, clinical presentation and treatment regimes. It also shows that some metabolic changes are robust across waves, and these can differentiate COVID-19-positive individuals from controls in a hospital setting. This research also supports the hypothesis that some metabolic pathways are disrupted several months after COVID-19 infection.
Volatiles are common in personal care products and dermatological drugs. Determining the impact of evaporation of volatiles on skin permeation is crucial to evaluate and understand their delivery, bioavailability, efficacy and safety. We aim to develop an in-silico model to simulate the impact of evaporation on the dermal absorption of volatiles. The evaporation of volatile permeants was modelled using vapour pressure as the main factor. This model considers evaporation as a passive diffusion process driven by the concentration gradient between the air-vehicle interface and the ambient environment. The evaporation model was then integrated with a previously published physiologically based pharmacokinetic (PBPK) model of skin permeation and compared with published in vitro permeation test data from the Cosmetics Europe ADME Task Force. The evaporation-PBPK model shows improved predictions when evaporation is considered. In particular, good agreement has been obtained for the distributions in the evaporative loss, and the overall percutaneous absorption. The model is further compared with published in-silico models from the Cosmetics Europe ADME Task Force where favourable results are achieved. The evaporation of volatile permeants under finite dose in vitro permeation test conditions has been successfully predicted using a mechanistic model with the intrinsic volatility parameter vapour pressure. Integrating evaporation in PBPK modelling significantly improved the prediction of dermal delivery.
Moisture migration occurring during storage in multicomponent food systems is one of the most common problems facing the food manufacturing industry. An example for such a food system is a mass of ice cream in contact with a wafer. In this work, a dynamic moisture migration model for a confectionery food system consisting of a wafer separated by a moisture barrier from a high water activity component (e.g. ice cream) is developed. The 1D diffusion equation was solved for the barrier and wafer each having different transport properties. The developed model predicts the moisture content of the wafer in different locations throughout the product’s shelf life.
Fruit and vegetable (F&V) harvested from plants trigger a series of stress-related physiological processes, potentially resulting in quality deterioration and considerable losses. Cold chain acting as abiotic stressors and activation of specific pathways to maintains metabolic activities is an effective way to reduce postharvest F&V loss. To this end, real-time monitoring of the micro-environment of the cold chain is an important approach. While temperature and humidity are routinely monitored nowadays, gas is much less explored despite it deeply interacts with the product quality of cold chain. This article analyzes the requirement for quality sensing via gas signal, reviews existing and emerging gas sensor technology and gas signal processing method for F&V cold chain. Furthermore, mathematical models, which interpret sensed gas data and predict product quality, are systematically analyzed and discussed. Gas sensor technology and associated modelling method is an effective approach to improve transparency and product quality for F&V cold chain. The results illustrate that the gas sensor for quality sensing of F&V cold chain should have characteristic with high precise resolution and full scale, low power consumption, low cost and smaller size, existed gas sensors have been gradually developed from a single unit to a plurality of components, specially rigid and flexible structural materials and manufacturing process. Existing mathematical models still have limited prediction accuracy that gas signal interfere product quality. Then, the model need improve the performances to explain the complex interaction relationship between gas and quality.
This paper concerns with the JY-KPLS model based transfer learning for the operation optimization of nonlinear batch processes. Due to problems of data insufficiency and uncertainties in a new nonlinear batch process that has just been put into production, the model-(new) process mismatch is usually inevitable, which is also the main reason for the poor performance of the batch process. To solve this problem, this paper first adopts the JY-KPLS model to capture the behavior of the nonlinear batch process, and takes full advantage of the information in similar batch processes to assist the modeling and operation optimization of a new process. Then, a data selection based batch-to-batch optimization control strategy is proposed in this paper to reduce the adverse effects of this mismatch on the operation of the new batch process. Finally, the feasibility of the proposed method is demonstrated by simulations. •The optimization of the batch process with strong nonlinearity and scarce process data is realized.•JY-KPLS transfer model is applied to nonlinear batch process operation optimization.•NCO mismatch problem is analyzed and reduced by model updating and data selection.•The algorithm framework of JY-KPLS model based batch-to-batch optimization is given.
Data-based empirical models, though widely used in process optimization, are restricted to a specific process being modeled. Model migration has been proved to be an effective technique to adapt a base model from a old process to a new but similar process. This paper proposes to apply the flexible Gaussian process regression (GPR) for empirical modeling, and develops a Bayesian method for migrating the GPR model. The migration is conducted by a functional scale-bias correction of the base model, as opposed to the restrictive parametric scale-bias approach. Furthermore, an iterative approach that jointly accomplishes model migration and process optimization is presented. This is in contrast to the conventional “two-step” method whereby an accurate model is developed prior to model-based optimization. A rigorous statistical measure, the expected improvement, is adopted for optimization in the presence of prediction uncertainty. The proposed methodology has been applied to the optimization of a simulated chemical process, and a real catalytic reaction for the epoxidation of trans-stilbene.
Current synthesis methods for producing single walled carbon nanotubes (SWCNTs) do not ensure uniformity of the structure and properties, in particular the length, which is an important quality indicator of SWCNTs. As a result, sorting SWCNTs by length is an important post-synthesis processing step. For this purpose, convenient analysis methods are needed to characterize the length distribution rapidly and accurately. In this study, density gradient ultracentrifugation was applied to prepare length-sorted SWCNT suspensions containing individualized surfactant-wrapped SWCNTs. The length of sorted SWCNTs was first determined by atomic force microscope (AFM), and their absorbance was measured in ultraviolet-visible near-infrared (UV-vis-NIR) spectroscopy. Chemometric methods are used to calibrate the spectra against the AFM-measured length distribution. The calibration model enables convenient analysis of the length distribution of SWCNTs through UV-vis-NIR spectroscopy. Various chemometric techniques are investigated, including pre-processing methods and non-linear calibration models. Extended inverted signal correction, extended multiplicative signal correction and Gaussian process regression are found to provide good prediction of the length distribution of SWCNTs with satisfactory agreement with the AFM measurements. In summary, spectroscopy in conjunction with advanced chemometric techniques is a powerful analytical tool for carbon nanotube research.
To advance physiologically-based pharmacokinetic modelling of xenobiotic metabolism by integrating metabolic kinetics with percutaneous absorption. Kinetic rate equations were proposed to describe the metabolism of a network of reaction pathways following topical exposure and incorporated into the diffusion-partition equations of both xenobiotics and metabolites. The published ex vivo case study of aromatic amines was simulated. Diffusion and partition properties of xenobiotics and subsequent metabolites were determined using physiologically-based quantitative structure property relationships. Kinetic parameters of metabolic reactions were best fitted from published experimental data. For aromatic amines, the integrated transdermal permeation and metabolism model produced data closely matched by experimental results following limited parameter fitting of metabolism rate constants and vehicle:water partition coefficients. The simulation was able to produce dynamic concentration data for all the dermal layers, as well as the vehicle and receptor fluid. This mechanistic model advances the dermal in silico functionality. It provides improved quantitative spatial and temporal insight into exposure of xenobiotics, enabling the isolation of governing features of skin. It contributes to accurate modelling of concentrations of xenobiotics reaching systemic circulation and additional metabolite concentrations. This is vital for development of both pharmaceuticals and cosmetics.
Process simulations can become computationally too complex to be useful for model-based analysis and design purposes. Meta-modelling is an efficient technique to develop a surrogate model using “computer data”, which are collected from a small number of simulation runs. This paper considers meta-modelling with time–space-dependent outputs in order to investigate the dynamic/distributed behaviour of the process. The conventional method of treating temporal/spatial coordinates as model inputs results in dramatic increase of modelling data and is computationally inefficient. This paper applies principal component analysis to reduce the dimension of time–space-dependent output variables whilst retaining the essential information, prior to developing meta-models. Gaussian process regression (also termed kriging model) is adopted for meta-modelling, for its superior prediction accuracy when compared with more traditional neural networks. The proposed methodology is successfully validated on a computational fluid dynamic simulation of an aerosol dispersion process, which is potentially applicable to industrial and environmental safety assessment.
Prediction uncertainty has rarely been integrated into traditional soft sensors in industrial processes. In this work, a novel autoswitch probabilistic soft sensor modeling method is proposed for online quality prediction of a whole industrial multigrade process with several steady-state grades and transitional modes. It is different from traditional deterministic soft sensors. Several single Gaussian process regression (GPR) models are first constructed for each steady-state grade. A new index is proposed to evaluate each GPR-based steady-state grade model. For the online prediction of a new sample, a prediction variance-based Bayesian inference method is proposed to explore the reliability of existing GPR-based steady-state models. The prediction can be achieved using the related steady-state GPR model if its reliability using this model is large enough. Otherwise, the query sample can be treated as in transitional modes and a local GPR model in a just-in-time manner is online built. Moreover, to improve the efficiency, detailed implementation steps of the autoswitch GPR soft sensors for a whole multigrade process are developed. The superiority of the proposed method is demonstrated and compared with other soft sensors in an industrial process in Taiwan, in terms of online quality prediction.
Permeability and partition coefficients of the skin barrier are important for assessing dermal absorption, bioavailability, and safety of cosmetics and medicine. We use the Potts and Guy equation to analyse the dependence of skin permeability on the hydrophobicity of permeants and highlight the significant differences in published datasets. Correlations of solute partition to skin are examined to understand the likely causes of the differences in the skin permeability datasets. Recently published permeability datasets show weak correlation and low dependence on hydrophobicity. As expected, early datasets show good correlation with hydrophobicity due to the related derivation. The weaker correlation of later datasets cannot be explained by the partition to skin lipids. All the datasets of solute partition to skin lipid showed a similar correlation to hydrophobicity where the log-linear correlation coefficient of partition is almost the same of the log-linear coefficient of Potts and Guy equation. Weak correlation and dependence of the late permeability datasets with SC lipid/water partition and that they are significantly under predicted by the Potts and Guy equation suggests either additional non-lipid pathway at play or a weaker skin barrier property.
Objectives To develop a simulation model to explore the interplay between mechanical stretch and diffusion of large molecules into the skin under locally applied hypobaric pressure, a novel penetration enhancement method. Methods Finite element method was used to model the skin mechanical deformation and molecular diffusion processes, with validation against in-vitro transdermal permeation experiments. Simulations and experimental data were used together to investigate the transdermal permeation of large molecules under local hypobaric pressure. Results Mechanical simulations resulted in skin stretching and thinning (20%–26% hair follicle diameter increase, and 21%–27% skin thickness reduction). Concentration of dextrans in the stratum corneum was below detection limit with and without hypobaric pressure. Concentrations in viable epidermis and dermis were not affected by hypobaric pressure (approximately 2 μg ∙ cm−2). Permeation into the receptor fluid was substantially enhanced from below the detection limit at atmospheric pressure to up to 6 μg ∙ cm−2 under hypobaric pressure. The in-silico simulations compared satisfactorily with the experimental results at atmospheric conditions. Under hypobaric pressure, satisfactory comparison was attained when the diffusion coefficients of dextrans in the skin layers were increased from ∼ 10 μm2 ∙ s−1 to between 200–500 μm2 ∙ s−1. Conclusions Application of hypobaric pressure induces skin mechanical stretching and enlarges the hair follicle. This enlargement alone cannot satisfactorily explain the increased transdermal permeation into the receptor fluid under hypobaric pressure. The results from the in-silico simulations suggest that the application of hypobaric pressure increases diffusion in the skin, which leads to improved overall transdermal permeation.
Baseline correction is a critical step for eliminating the interference of baseline drift in spectroscopic analysis. The recently proposed sparse Bayesian learning (SBL)-based method can significantly improve the baseline correction performance. However, it has at least two disadvantages: (i)it works poorly for large-scale datasets and (ii) it completely ignores the burst-sparsity structure of the sparse representation of the pure spectrum. In this paper, we present a new fast burst-sparsity learning method for baseline correction to overcome these shortcomings. The first novelty of the proposed method is to jointly adopt a down-sampling strategy and construct a multiple measurements block-sparse recovery problem with the down-samplingsequences. The down-sampling strategy can significantly reduce the dimension of the spectrum; while jointly exploiting the block sparsity among the down-sampling sequences avoids losing the information contained in the original spectrum. The second novelty of the proposed method is introducing the pattern-coupled prior into the SBL framework to characterize the inherent burst-sparsity in the sparse representation of spectrum. As illustrated in the paper, burst-sparsity commonly occurs in peak zones with more denser nonzero coefficients. Properly utilizing such burst-sparsity can further enhance the baseline correction performance. Results on both simulated and real datasets (such as FT-IR, Raman spectrum, and chromatography) verify the substantial improvement, in terms of estimation accuracy and computational complexity
Online measurement of the melt index is typically unavailable in industrial polypropyleneproductionprocesses, soft sensing models are therefore required for estimation and prediction of this important quality variable. Polymerization is a highly nonlinear process, which usually produces products with multiple quality grades. In the present paper, an effective soft sensor, named combined local Gaussian process regression (CLGPR), is developed for prediction of the melt index. While the introduced Gaussian process regression model can well address the high nonlinearity of the process data in each operation mode, the local modeling structure can be effectively extended to processes with multiple operation modes. Feasibility and efficiency of the proposed soft sensor are demonstrated through the application to an industrial polypropyleneproductionprocess.
This paper presents the application of the bagging technique for non-linear regression models to obtain more accurate and robust calibration of spectroscopy. Bagging refers to the combination of multiple models obtained by bootstrap re-sampling with replacement into an ensemble model to reduce prediction errors. It is well suited to “non-robust” models, such as the non-linear calibration methods of artificial neural network (ANN) and Gaussian process regression (GPR), in which small changes in data or model parameters can result in significant change in model predictions. A specific variant of bagging, based on sub-sampling without replacement and named subagging, is also investigated, since it has been reported to possess similar prediction capability to bagging but requires less computation. However, this work shows that the calibration performance of subagging is sensitive to the amount of sub-sampled data, which needs to be determined by computationally intensive cross-validation. Therefore, we suggest that bagging is preferred to subagging in practice. Application study on two near infrared datasets demonstrates the effectiveness of the presented approach.
Controlled environment agriculture (CEA) has some clear advantages over traditional farming, such as: reliable and consistent production capability; efficiency in water and space use; reducing the use and runoff of fertiliser and pesticides; etc. As such CEA can greatly benefit from the CAPE (computer-aided process engineering) approach – cross-fertilization of these two apparently distinct areas may result in new methods and applications to improve CEA and process engineering, with potentially significant contribution to circular economy. In this paper, we discuss several important aspects of CEA drawing from our own experiences in aquaculture and aeroponics, including product development, process design and process operation, and the potential contribution of CAPE. Finally, we postulate a systems platform for CEA, aiming to foster a long-lasting partnership between the two scientific communities.
Accidental releases of superheated liquids, such as liquefied petroleum or natural gases, are featured by depressurization across the outlet leading to liquid flashing within the upstream container and the downstream jet. In this work, dynamic behaviors of in-tank liquid with phase change throughout depressurized releases and the influence on the primary breakup of flashing jet were studied with an experimental 20 L tank. In-tank parameters (pressure, temperature, and liquid mass) and downstream jet morphology were characterized. A new nondimensional number (ηp), the ratio between the superheat levels of the saturated states corresponding to liquid temperature and pressure, was developed to describe the liquid's thermodynamic state under both release and ambient conditions. A thermodynamics-determined release rate model was established to characterize flow behaviors at the exit. Results showed a strong correlation between the initial ηp0 and key process parameters I (depressurization and release rates): I = aηp0b, where a and b are constants for a particular I. A ηp0-based criterion was derived to characterize in-tank release dynamics and thermodynamics: ηp0
•Breakup regime of superheated liquid jet under depressurized release is examined.•Both thermal nonequilibrium and mechanical forces are involved as driving effects.•The coupling regime of the two driving effects during depressurization is explored.•Non-flashing, partially flashing, and fully flashing breakup modes are identified.•The interaction of the two driving effects is quantified by dimensionless analysis. Superheated liquid jets disintegrate into numerous droplets when released into the ambient with lower saturated pressure, driven by thermal nonequilibrium induced flashing and the accompanying mechanical forces. Such a phenomenon facilitates fuel atomization in energy utilization while posing a serious threat during accidental releases. In this work, the breakup and droplet formation of superheated liquid jets under depressurized releases were investigated with an experimental 20 L tank. A high-speed camera was utilized to characterize breakup behaviors. The interaction between thermodynamic and mechanical effects during depressurization was discussed based on linear stability analysis and bubble dynamics. Furthermore, the quantitative relationship between the two driving effects under different conditions was established using dimensionless and multiple regression analyses. Results show that the thermodynamic effect increases with the decreased mechanical effect during depressurization because of the increased energy of bubble burst, regardless of the external or internal flashing regime. Non-flashing, partially flashing, and fully flashing breakup modes are identified. The dimensionless and multiple regression analyses show that in addition to thermodynamic (Ja, ρv/ρl, Rp, and ηp) and mechanical (Wev and Oh) effects, the inhibition induced by the cooling effect (Pr and Ec) should not be overlooked. The quantitative expression among them agrees well with experimental data with R2=0.976.
This paper addresses accent issues in large vocabulary continuous speech recognition. Cross-accent experiments show that the accent problem is very dominant in speech recognition. Analysis based on multivariate statistical tools (principal component analysis & independent component analysis) confirms that accent is one of the key factors in speaker variability. Considering different applications, we proposed two methods for accent adaptation. When a certain amount of adaptation data was available, pronunciation dictionary modeling was adopted to reduce recognition errors caused by pronunciation mistakes. When a large corpus was collected for each accent type, accent-dependent models were trained, & a Gaussian mixture model-based accent identification system was developed for model selection. We report experimental results for the two schemes & verify their efficiency in each situation. 13 Tables, 5 Figures, 23 References. Adapted from the source document
Purpose: The development of a new two-dimensional (2D) model to predict follicular permeation, with integration into a recently reported multi-scale model of transdermal permeation is presented. Methods: The follicular pathway is modelled by diffusion in sebum. The mass transfer and partition properties of solutes in lipid, corneocytes, viable dermis, dermis and systemic circulation are calculated as reported previously [Pharm Res 33 (2016) 1602]. The mass transfer and partition properties in sebum are collected from existing literature. None of the model input parameters was fit to the clinical data with which the model prediction is compared. Results: The integrated model has been applied to predict the published clinical data of transdermal permeation of caffeine. The relative importance of the follicular pathway is analysed. Good agreement of the model prediction with the clinical data has been obtained. The simulation confirms that for caffeine the follicular route is important; the maximum bioavailable concentration of caffeine in systemic circulation with open hair follicles is predicted to be 20% higher than that when hair follicles are blocked. Conclusions: The follicular pathway contributes to not only short time fast penetration, but also the overall systemic bioavailability. With such in silico model, useful information can be obtained for caffeine disposition and localised delivery in lipid, corneocytes, viable dermis, dermis and the hair follicle. Such detailed information is difficult to obtain experimentally.
Mass transfer studies are carried out in a bubble column with an internal diameter of 14. cm and various static liquid heights. The mass transfer coefficient is evaluated by using an oxygen sorption method. A model considering the gas holdup flushing and the sensor response is used. The interfacial mass transfer area is determined according to the measured bubble size distribution. The liquid-side mass transfer coefficient is also estimated from the volumetric mass transfer coefficient and the interfacial mass transfer area found. Results show that the effect of static liquid height on gas-liquid mass transfer is primarily on the interfacial mass transfer area. The mass transfer process is also governed by the type of gas distributor used. A single nozzle distributor is not suitable for shallow bubble column operations due to the large initial bubbles and the large volume of dead zone generated. It is also found that the different dependence of the liquid-side mass transfer coefficient on the superficial gas velocity observed in the literatures is due to the different bubble rising regimes. © 2012 Elsevier B.V.
Purpose - To study the effect of the lipophilicity of the follicular route on the overall permeation of a given substance into the skin, as in vitro and in vivo experimental results for skin permeation found in the literature provide inconclusive information on this matter. Method - We use an in silico skin permeation mechanistic model to simulate the dermal delivery of 30 small molecules (molecular weight
Temperature fluctuations can have a significant impact on the repeatability of spectral measurements and as a consequence can adversely affect the resulting calibration model. More specifically, when test samples measured at temperatures unseen in the training dataset are presented to the model, degraded predictive performance can materialise. Current methods for addressing the temperature variations in a calibration model can be categorised into two classes—calibration model based approaches, and spectra standardisation methodologies. This paper presents a comparative study on a number of strategies reported in the literature including partial least squares (PLS), continuous piecewise direct standardisation (CPDS) and loading space standardisation (LSS), in terms of the practical applicability of the algorithms, their implementation complexity, and their predictive performance. It was observed from the study that the global modelling approach, where latent variables are initially extracted from the spectra using PLS, and then augmented with temperature as the independent variable, achieved the best predictive performance. In addition, the two spectra standardisation methods, CPDS and LSS, did not provide consistently enhanced performance over the conventional global modelling approach, despite the additional effort in terms of standardising the spectra across different temperatures. Considering the algorithmic complexity and resulting calibration accuracy, it is concluded that the global modelling (with temperature) approach should be first considered for the development of a calibration model where temperature variations are known to affect the fundamental data, prior to investigating the more powerful spectra standardisation approaches. Copyright © 2007 John Wiley & Sons, Ltd.
Fault detection and diagnosis (FDD) is a critical approach to ensure safe and efficient operation of manufacturing and chemical processing plants. Multivariate statistical process monitoring (MSPM) has received considerable attention for FDD since it does not require a mechanistic process model. The diagnosis of the source or cause of the detected process fault in MSPM largely relies on contribution analysis, which is ineffective in identifying the joint contribution of multiple variables to the occurrence of fault. In this work, a missing variable analysis approach based on probabilistic principal component analysis is proposed for fault isolation. Furthermore, a branch and bound method is developed to handle the combinatorial nature of the problem involving finding the variables, which are most likely responsible for the occurrence of fault. The efficiency of the method proposed is shown through a case study on the Tennessee Eastman process.
Patient-reported outcome measures (PROMs) are a useful way of recording patient perceptions of the impact of their cancer and the consequences of treatment. Understanding the impact of radiotherapy longer term requires tools that are sensitive to change but also meaningful for patients. PROMs are useful in defining symptom severity but also the burden of illness for cancer patients. Patient-reported outcomes are increasingly being seen as a way to improve practice by enhancing communication, improving symptom management as well as identifying patient care needs. This paper provides an overview of the use of PROMs in radiotherapy and considerations for tool choice, analysis and the logistics of routine data collection. Consistent assessment is essential to detect patient problems as a result of radiotherapy, but also to address emerging symptoms promptly.
Meta-modelling for fast analysis of CFD-simulated vapour cloud dispersion processes Abstract: Released flammable chemicals can form an explosible vapour cloud, posing safety threat in both industrial and civilian environments. Due to the difficulty in conducting physical experiments, computational fluid dynamic (CFD) simulation is an important tool in this area. However, such simulation is computationally too slow for routine analysis. To address this issue, a meta-modelling approach is developed in this study; it uses a small number of simulations to build an empirical model, which can be used to predict the concentration field and the potential explosion region. The dimension of the concentration field is reduced from around 43,421,400 to 20 to allow meta-modelling, by using the segmented principal component transform-principal component analysis. Moreover, meta-modelling-based uncertainty analysis is explored to quantify the prediction variance, which is important for risk assessment. The effectiveness of the methodology has been demonstrated on CFD simulation of the dispersion of liquefied natural gas
Suction cups are widely used in applications such as in measurement of mechanical properties of skin in vivo, in drug delivery devices or in acupuncture treatment. Understanding mechanical response of skin under hypobaric pressure is of great importance for users of suction cups. The aim of this work is to predict the hypobaric pressure induced 3D stretching of the skin. Experimental skin tensile tests were carried out for mechanical property characterization. Both linear elasticity and hyperelasticity parameters were determined and implemented in Finite Element modelling. Skin suction tests were performed in both experiments and FEM simulations for model validation. 3D skin stretching is then visualized in detail in FEM simulations. The simulations showed that the skin was compressed consistently along the thickness direction, leading to reduced thickness. At the center of the dome, the radial and angular strain decreases from the top surface to the bottom surface, although always in tension. Hyperelasticity modelling showed superiority over linear elasticity modelling while predicting the strain distribution because the stretch ratio reaches values exceeding the initial linear elastic stage of the stress-strain curve for skin. Hyperelasticity modelling is an effective approach to predict the 3D strain distribution, which paves a way to accurately design safe commercial products that interface with the skin.
In chemical engineering applications, computational efficient meta-models have been successfully implemented in many instants to surrogate the high-fidelity computational fluid dynamics (CFD) simulators. Nevertheless, substantial simulation efforts are still required to generate representative training data for building meta-models. To solve this problem, in this research work an efficient meta-modeling method is developed based on the concept of transfer learning. First, a base model is built which roughly mimics the CFD simulator. With the help of this model, the feasible operating region of the simulated process is estimated, within which computer experiments are designed. After that, CFD simulations are run at the designed points for data collection. A transfer learning step, which is based on the Bayesian migration technique, is then conducted to build the final meta-model by integrating the information of the base model with the simulation data. Because of the incorporation of the base model, only a small number of simulation points are needed in meta-model training.
•Parameter and time step selection policy has been summarized and classified.•The intelligent prediction models for horticultural facilities were synthesized.•Technical trends and research values of 23 DNN models are systematically discussed.•The accuracy and generalization of intelligent prediction model are analyzed.•Summarize the application of the model from a new sight of information validity. Agricultural greenhouse production has to require a stable and acceptable environment, it is therefore essential for future greenhouse production to obtain full and precisely internal dynamic environment parameters. Dynamic modeling based on machine learning methods, e.g., intelligent time series prediction modeling, is a popular and suitable way to solve the above issue. In this article, a systematic literature review on applying advanced time series models has been systematically conducted via a detailed analysis and evaluation of 61 pieces selected from 221 articles. The historical process of time series model application from the use of data and information strategies was first discussed. Subsequently, the accuracy and generalization of the model from the selection of model parameters and time steps, providing a new perspective for model development in this field, were compared and analyzed. Finally, the systematic review results demonstrate that, compared with traditional models, deep neural networks could increase data structure mining capabilities and overall information simulation capabilities through innovative and effective structures, thereby it could also broaden the selection range of environmental parameters for agricultural facilities and achieve environmental prediction end-to-end optimization via intelligent time series model based on deep neural networks.
Impairment of the skin’s structural integrity initially results in acute wounds which can become chronic if timely wound closure is not achieved. Chronic wounds (CWs) affect more than 1% of the global population with increasing cases of this condition due to the ageing population. Current wound management relies on debridement, hyperbaric oxygen, antibiotics, and wound dressings, which lack early intervention and specificity. Herein, antibiotics-free retinol-based micellar formulations (RMF) were made and their wound healing efficacy were investigated in vitro. Five different formulations with retinol contents of 0.3% and 1% against a placebo were topically applied to an organotypic full-thickness skin wound model (FT-SWM, MatTek®) with a 3 mm punch wound, and maintained in an incubator for 6 days. The histological analysis of the FT-SWM was conducted at depths of 60 µm and 80 µm. It was found that all the micellar retinol formulations accelerated wound bed contraction, with 0.3% RMF demonstrating the highest efficacy. At the depths of 60 µm and 80 µm, the 0.3% RMF exhibited inner wound diameter contraction of 58% and 77%, respectively, in comparison to the placebo showing 15% and 8%. The RMF significantly accelerated wound healing and can thus be a potential early intervention for speedy wound recovery. It should be pointed out that these results were obtained based on a small sample size and a large sample size will be explored to further validate the results.
Dynamics are inherent characteristics of batchprocesses, and they may exist not only within a particular batch, but also from batch to batch. To model and monitor such two-dimensional (2D) batch dynamics, two-dimensionaldynamic principal component analysis (2D-DPCA) has been developed. However, the original 2D-DPCA calculates the monitoring control limits based on the multivariateGaussian distribution assumption which may be invalid because of the existence of 2D dynamics. Moreover, the multiphase features of many batchprocesses may lead to more significant non-Gaussianity. In this paper, Gaussian mixture model (GMM) is integrated with 2D-DPCA to address the non-Gaussian issue in 2D dynamicbatchprocessmonitoring. Joint probability density functions (pdf) are estimated to summarize the information contained in 2D-DPCA subspaces. Consequently, for online monitoring, control limits can be calculated based on the joint pdf. A two-phase fed-batch fermentation process for penicillin production is used to verify the effectiveness of the proposed method.
Deep learning has shown great promise in process fault diagnosis. However, due to the lack of sufficient labelled fault data, its application has been limited. This limitation may be overcome by using the data generated from computer simulations. In this study, we consider using simulated data to train deep neural network models. As there inevitably is model-process mismatch, we further apply transfer learning approach to reduce the discrepancies between the simulation and physical domains. This approach will allow the diagnostic knowledge contained in the computer simulation being applied to the physical process. To this end, a deep transfer learning network is designed by integrating the convolutional neural network and advanced domain adaptation techniques. Two case studies are used to illustrate the effectiveness of the proposed method for fault diagnosis: a continuously stirred tank reactor and the pulp mill plant benchmark problem.
Kernel principal component analysis (KPCA) has become a popular technique for process monitoring, owing to its capability of handling nonlinearity. Nevertheless, KPCA suffers from two major disadvantages. First, the underlying manifold structure of data is not considered in process modeling. Second, the selection of kernel parameters is problematic. To avoid such deficiencies, a manifold learning technique named maximum variance unfolding (MVU) is considered as an alternative. However, such method is merely able to deal with the training data, but has no means to handle new samples. Therefore, MVU cannot be applied to process monitoring directly. In this paper, an extended MVU (EMVU) method is proposed, extending the utilization of MVU to new samples by approximating the nonlinear mapping between the input space and the output space with a Gaussian process model. Consequently, EMVU is suitable to nonlinear process monitoring. A cross-validation algorithm is designed to determine the dimensionality of the EMVU output space. For online monitoring, three different types of monitoring indices are developed, including squared prediction error (SPE), Hotelling-T, and the prediction variance of the outputs. In addition, a fault isolation algorithm based on missing data analysis is designed for EMVU to identify the variables contributing most to the faults. The effectiveness of the proposed methods is verified by the case studies on a numerical simulation and the benchmark Tennessee Eastman (TE) process. © 2014 Elsevier Ltd. All rights reserved.
Renewable energy in general, and biofuels in particular, is seen as a viable solution for energy security and climate change problems. For this reason many countries, including Thailand, have set common objectives for utilisation of alternative resources. Thailand is an agricultural country and hence it has a great potential for generating renewable energy from a large amount of biomass resources. In consequence, a 15-year renewable energy development plan has been set by the Thai government, which targets an increase in electricity generation of 32%, from 2,800 MW in 2011 to 3,700 MW in 2022, and also an increase in consumption of ethanol by 200%, from 1,095 million litres in 2011 to 3,285 million litres in 2022 (Department of Alternative Energy Development and Efficiency of Thailand, 2008). Sugarcane and rice are the two main industrial crops in Thailand, with estimated production of 73.50 million tons of sugarcane per year (2009) and 31.50 million tons of rice per year (Sawangphol, 2011), and they are seen as a major source of biomass. This research focuses on the biomass from rice mill and sugar mill processes. In order to develop processing facilities that are capable of utilising available biomass and delivering the above set targets, a comprehensive and systematic methodology is required which will support the decision-making process by accounting for technological, economic and parameters. In this thesis, exhaustive simulation and optimisation are proposed as a tool. The first tool is the technology screening. The aim of the technology screening step is to show all profitability of technologies. This is done by considering various components of rice and sugar mills energy frameworks in Thailand: rice mill technology type, sugar mill technology type, ethanol technology type and biomass based power plant technology type. The modelling of processes for converting sugarcane and rice biomass into electrical energy and ethanol has been performed at the level of superstructure which has been chosen because the scope of the work is to screen available options and to compare them in different configurations in terms of economic aspects. The result of the simulation approach has shown the most profitable (shortest payback period) is the configuration that includes electrical rice mill, automated control sugar mill, gasification biomass based power plant and continuous ethanol plant. The sensitivity analysis has compared the cost of feedstock against profitability (payback period). The sensitivity analysis also compared the price of product against profitability (payback period). The result of the sensitivity analysis showed the change in the price of sugar product is the most sensitive for the rice and sugar mills energy framework. The second tool is the optimisation approach. The aim of the optimisation is to maximise the profit (NPV) impact. This is done by considering the various components of the biofuel supply chain in Thailand. All components were calculated based on candidate points including: the biofield(rice mill and sugar mill), biomass warehouse capacity and location, biofuel plant technology type, plant technology capacity, plant technology location, product warehouse capacity and location, transportation type is considered. There are four scenarios in the case study which were created to examine the proposed biomass optimisation model for Thailand to validate the mathematic formulation. The overall conclusion of the optimization approach is that the biomass power plant is profitable at the present time. The lignocellulosic plant will be the option when the process demand a lot of ethanol production. In summary, the proposed research fills the gap in the operational level and process level by multi-biomass from biofield to customer that includes warehouses and multi transportation modes towards the biofuel supply chain. From the business point of view, the research defines the data for the business investor and also analyses the risk of change in product price, feedstock cost and transportation cost.
In this study, we investigate a data-driven optimal control for a new batch process. Existing data-driven optimal control methods often ignore an important problem, namely, because of the short operation time of the new batch process, the modeling data in the initial stage can be insufficient. To address this issue, we introduce the idea of transfer learning, i.e., a latent variable process transfer model (LV-PTM) is adopted to transfer sufficient data and process information from similar processes to a new one to assist its modeling and quality optimization control. However, due to fluctuations in raw materials, equipment, etc., differences between similar batch processes are always inevitable, which lead to the serious and complicated mismatch of the necessary condition of optimality (NCO) between the new batch process and the LV-PTM-based optimization problem. In this work, we propose an LV-PTM-based batch-to-batch adaptive optimal control strategy, which consists of three stages, to ensure the best optimization performance during the whole operation lifetime of the new batch process. This adaptive control strategy includes model updating, data removal, and modifier-adaptation methodology using final quality measurements in response. Finally, the feasibility of the proposed method is demonstrated by simulations.
This paper investigates replacement scheduling for non-repairable safety-relatedsystems (SRS) with multiple components and states. The aim is to determine the cost-minimizing time for replacing SRS while meeting the required safety. Traditionally, such scheduling decisions are made without considering the interaction between the SRS and the production system under protection, the interaction being essential to formulate the expected cost to be minimized. In this paper, the SRS is represented by a non-homogeneous continuous time Markov model, and its state distribution is evaluated with the aid of the universal generating function. Moreover, a structure function of SRS with recursive property is developed to evaluate the state distribution efficiently. These methods form the basis to derive an explicit expression of the expected system cost per unit time, and to determine the optimal time to replace the SRS. The proposed methodology is demonstrated through an illustrative example.
This paper presents a Bayesian approach to the development of spectroscopic calibration models. By formulating the linear regression in a probabilistic framework, a Bayesian linear regression model is derived, and a specific optimization method, i.e. Bayesian evidence approximation, is utilized to estimate the model "hyper-parameters". The relation of the proposed approach to the calibration models in the literature is discussed, including ridge regression and Gaussian process model. The Bayesian model may be modified for the calibration of multivariate response variables. Furthermore, a variable selection strategy is implemented within the Bayesian framework, the motivation being that the predictive performance may be improved by selecting a subset of the most informative spectral variables. The Bayesian calibration models are applied to two spectroscopic data sets, and they demonstrate improved prediction results in comparison with the benchmark method of partial least squares.
A 2‐day meeting was held by members of the UK Quantitative Systems Pharmacology Network (http://www.qsp‐uk.net/) in November 2018 on the topic of Translational Challenges in Oncology. Participants from a wide range of backgrounds were invited to discuss current and emerging modeling applications in nonclinical and clinical drug development, and to identify areas for improvement. This resulting perspective explores opportunities for impactful quantitative pharmacology approaches. Four key themes arose from the presentations and discussions that were held, leading to the following recommendations: • Evaluate the predictivity and reproducibility of animal cancer models through precompetitive collaboration. • Apply mechanism of action (MoA) based mechanistic models derived from nonclinical data to clinical trial data. • Apply MoA reflective models across trial data sets to more robustly quantify the natural history of disease and response to differing interventions. • Quantify more robustly the dose and concentration dependence of adverse events through mathematical modelling techniques and modified trial design.
The aquaponic system can satisfy the different needs of aquatic products and plants by utilizing nutrient flow to improve the economic benefit. Nitrogen is a key nutrient element in such process. High nitrogen concentration can worsen water quality, which may further result in mass death of typical fish and/or shellfish. Therefore, it is particularly important to monitor the nitrogen concentration to manage aquatic products and plants growth in aquaponic systems. Sensors for measuring nitrogen concentration are commercially available, but they are often expensive and unreliable in service. At the same time, the research on the soft sensor of nitrogen concentration is very limited. Therefore, this paper proposes a new adaptive filtering-based soft sensor method for real-time estimation of total nitrogen concentration. This method provides accurate prediction by integrating mechanistic model, online measurements (e.g. fish biomass, temperature) and infrequent offline measurement of total nitrogen. A moving-horizon estimation (MHE) algorithm is used for joint state and parameter estimation, thus allowing correction of mismatch between model parameters and the real process. Furthermore, the appropriate frequency of offline nitrogen measurement is determined to balance between the soft sensor accuracy and cost. Through computer simulation study of an aquaponic system, the proposed approach is effective to provide promising real-time prediction performance. By applying the correction method, the RMSE (root-mean-square error) of nitrogen concentration estimation is reduced by 31.86% on average compared to the simulated practical situation. In conclusion, the proposed soft sensor method could provide useful monitoring of the total nitrogen in aquaponics, and such information could be used for optimizing the operations.
Explosion of methane‐air mixtures is an important research issue. Of many factors affecting this process, initial turbulence has been recognized as an important one but less studied. This work determines the important parameters in the experimental process that affect gas explosion process and investigate the characteristics of pressure, temperature and velocity in the methane‐air explosion process under quiescent and turbulent conditions. Experiments and CFD are integrated to optimize the experimental parameters and elucidate the impact of initial turbulence on the explosion behavior of methane‐air mixtures. The results show good agreement between the computed results and experimental data. For a certain CH4 concentration within the explosive limit range, initial turbulence can increase the maximum explosion pressure () and the maximum rate of the pressure rise (), and shorten the time to reach to maximum explosion pressure (), enhance the explosion strength and destructive power of CH4‐air mixtures. Furthermore, the simulated pressure field indicates that the pressure will be uniform within the short time period during the gas explosion in a small space. At the same time, the experimental results show that turbulent flow can significantly broaden the explosive limits of CH4‐air mixtures and the effect is more apparent on the upper explosive limit than on the lower one. The ignition delay time which influences the homogeneous distribution of initial turbulence was determined by numerical simulation. The combination of numerical simulation and experimental results will provide more efficient method to understand the explosion characteristics and mechanism of flammable gas mixtures.
Batch processes have been playing a crucial role for the flexibility in producing low-volume and high-value-added products. Due to the fluctuations of raw materials and operation conditions, the batch duration often varies. Prediction of batch completion time is important for the purpose of process scheduling and optimization. Existing studies of this subject have been focused on the prediction accuracy, while the importance of the prediction uncertainty has been under-explored. When the key variable defining the completion time changes slowly towards the end of a batch, the prediction uncertainty tends to be large. Under such situations, we argue that the uncertainty should always be considered along with the mean prediction for practical use. To this end, two data-driven prediction methods using probabilistic principal component analysis (PPCA) and bootstrapping case-based reasoning (Bootstrapping CBR) are developed, followed by the uncertainty quantification in the probabilistic framework. Finally, two batch processes are used to demonstrate the importance of prediction uncertainty and the efficiency of the proposed schemes.
Object counting in aquaculture is an important task, and has been widely applied on fish population estimation, lobster abundance estimation, and scallop stock, etc. However, underwater object counting is challenging for biologists and marine scientists, because of the diversity of background of the lake or ocean, the uncertainty of the object motion, and the occlusion between objects. With the rapid development of sensor, computer vision and acoustic technologies, advanced and efficient counting methods are available in aquaculture. We reviewed underwater object counting methods in aquaculture, provided a survey including more than 50 papers in the recent 10 years, and analyzed the pros and cons of the counting methods and the applicable scenarios of those methods. Finally, the major challenges and future trends of underwater object counting in aquaculture are discussed.
The prediction of gas diffusion concentration has practical significance. Because the process simulation is based on complex mechanism, it will not be able to be calculated in real time. Moreover, the requirements of computational fluid dynamics on computers limit its application. This paper proposes the computational fluid dynamics simulation surrogate models based on the GA-BP neural network to predict the concentration after aerosol dispersion. Considering the relevant influence parameters of time, space coordinates and concentration, two different models of input and output variables are constructed. The results reveal that when the prediction object is affected by high-dimensional complex factors, the GA-BP neural network can generate accurate prediction results. Compared with the traditional BP neural network, the prediction accuracies can be improved by 40.65% and 77.61%, respectively, which exhibits excellent performance for data prediction. The method proposed in this paper successfully verifies the computational fluid dynamics simulation of the aerosol dispersion processes, and the research has potential application value for environmental safety assessment.
Understanding the heterogeneous barrier property and permeation pathway of the skin is important for skin health, transdermal drug delivery, cosmetic care as well as the protection of the skin from environmental exposure to air pollution and harmful chemicals. This chapter reviews recent progress in microscopic modeling of the heterogeneous barrier property and permeation pathway of the stratum corneum (SC) barrier and their effects on the dermatopharmacokinetics and bioavailability of dermal exposure. The microscopic structure parameters of the human SC barrier depend on a number of factors such as body site, age, and sex. Studies of microscopic modeling of transdermal permeation focused on the lipid pathway, using the homogenized 1D model of solute permeation across the SC membrane at steady state. The chapter discusses the progress in applying microscopic modeling for in silico prediction of the dermatopharmacokinetic and bioavailability. Predicting systemic pharmacokinetics following dermal exposure is important for the purpose of efficacy and safety assessment.
We consider an off-line process design problem where the response variable is affected by several factors. We present a data-based modelling approach that iteratively allocates new experimental points, update the model, and search for the optimal process factors. A flexible non-linear modelling technique, the kriging (also known as Gaussian processes), forms the cornerstone of this approach. Kriging model is capable of providing accurate predictive mean and variance, the latter being a quantification of its prediction uncertainty. Therefore, the iterative algorithm is devised by jointly considering two objectives: (i) to search for the best predicted response, and (ii) to adequately explore the factor's space so that the predictive uncertainty is small. This method is further extended to consider dynamic processes, i.e. the process factors are time-varying and thus the problem becomes to design a time-dependent trajectory of these factors. The proposed approach has been demonstrated by its application to a simulated chemical process with promising results being achieved.
In silico methods to estimate and/or quantify skin absorption of chemicals as a function of chemistry are needed to realistically predict pharmacological, occupational, and environmental exposures. The Potts-Guy equation is a well- established approach, using multi-linear regression analysis describing skin permeability (Kp) in terms of the octanol/water partition coefficient (logP) and molecular weight (MW). In this work, we obtained regression equations for different human datasets relevant to environmental and cosmetic chemicals. Since the Potts-Guy equation was published in 1992, we explored recent datasets that include different skin layers, such as dermatomed (including dermis to a defined thickness) and full-skin. Our work was consistent with others who have observed that fits to the Potts-Guy equation are stronger for experiments focused on the epidermis. Permeability estimates for dermatomed skin and full skin resulted in low regression coefficients when compared to epidermis datasets. An updated regression equation uses a combination of fitted permeability values obtained with a published 2D compartmental model previously evaluated. The resulting regression equation was: logKp = = -2.55 +0.65*logP – 0.0085*MW, R2=0.91 (applicability domain for all datasets: MW ranges from 18->584 g/mol and -4 ->5 for logP) . This approach demonstrates the advantage of combining mechanistic with structural activity relationships in a single modeling approach. This combination approach results in an improved regression fit when compared to permeability estimates obtained using the Potts-Guy approach alone. The analysis presented in this work assumes a one-compartment skin absorption route, future modeling work will consider adding multiple compartments.
Accidental superheated liquid emissions into the atmosphere yield two-phase releases. The resulting flashing jet, driven by thermal nonequilibrium and mechanical forces, breaks up into massive droplets, fostering beneficial conditions for fire, explosion, and toxic diffusion. In this work, a 20 L tank was built to examine two-phase flow behaviors during depressurized releases of superheated liquids via a high-speed camera and phase Doppler anemometry. Different breakup regimes of flashing jet and dimensionless groups that effectively represent thermodynamic (RpJa) and mechanical (WevOh) driving effects were determined. Based on the interaction between the two effects, quantitative criteria to distinguish different regimes were developed. The accompanying jet characteristics, including jet angle (θ), area fraction (fA), droplet diameter (dSMD), and droplet velocity (ud), and their relationship with jet breakup were revealed. Results show that non-flashing (NFB), partially flashing (PFB), and fully flashing (FFB) breakups coincide with RpJa(WevOh)1/7
This work addresses the integrated optimization of both the plant-wide material processing system and the utility system for a polyvinyl chloride (PVC) plant. In the plant-wide material processing system, vinyl chloride monomer (VCM) production process and VCM polymerization process are optimized to determine production allocation and switching operation of parallel equipment as well as raw material supply arrangement. In the utility system, power generation/supply plan is determined by combined heat and power (CHP) units and the state grid. The nonlinear electricity consuming characteristics of calcium carbide production process, CHP process, and electrolysis process are modeled based on the industrial data. A multiperiod mixed-integer nonlinear programming (MINLP) model of a PVC plant by calcium carbide method is proposed with the intent to enhance the profit and reduce the energy consumption. The proposed MINLP model is successfully applied to two cases originated from a real-world industrial plant in China and results provide valuable guidance for the company planning. The comparative results verify the effectiveness and superiority of the proposed plant-wide integrated scheme.
In order to monitor nonlinear processes, kernel principal component analysis (KPCA) has become a popular technique. Nevertheless, KPCA suffers from two major disadvantages. First, the underlying manifold structure of data is not considered in process modeling. Second, the selection of kernel function and kernel parameters is always problematic. To avoid such deficiencies, an integrating method of manifolding learning and Gaussian process is proposed in this paper, which extends the utilization of maximum variance unfolding (MVU) to online process monitoring and fault isolation. The proposed method is named as extendable MVU (EMVU), whose effectiveness is verified by the case studies on the benchmark Tennessee Eastman (TE) process. © 2013 Elsevier B.V.
Gaussian process regression (GPR) is a Bayesian non-parametric technology that has gained extensive application in data-based modelling of various systems, including those of interest to chemometrics. However, most GPR implementations model only a single response variable, due to the difficulty in the formulation of covariance function for correlated multiple response variables, which describes not only the correlation between data points, but also the correlation between responses. In the paper we propose a direct formulation of the covariance function for multi-response GPR, based on the idea that its covariance function is assumed to be the "nominal" uni-output covariance multiplied by the covariances between different outputs. The effectiveness of the proposed multi-response GPR method is illustrated through numerical examples and response surface modelling of a catalytic reaction process. (C) 2015 Elsevier B.V. All rights reserved.
Microneedle devices offer minimally invasive and rapid biomarker extraction from the skin. However, the lack of effective assessment tools for such microneedle devices can delay their development into useful clinical applications. Traditionally, the microneedle performance is evaluated i) in vivo, using animal models, ii) ex vivo, on excised human or animal skin or iii) in vitro, using homogenised solutions with the target antigen to model the interstitial fluid. In vivo and ex vivo models are considered the gold-standard approach for the evaluation of microneedle devices because of their structural composition, however they do exhibit limitations. More specifically, they have limited availability and they present batch-to-batch variations depending on the skin origin. Furthermore, their use rises ethical concerns regarding compliance with the globally accepted 3Rs principle of reducing the use of animals for research purposes. At the same time, in vitro models fail to accurately mimic the structure and the mechanical integrity of the skin tissue that surrounds the interstitial fluid. In this study, we introduce for the first time an animal-free, mechanically robust, 3D scaffold that has great potential as an accurate in vitro evaluation tool for immunodiagnostic microneedle devices. More specifically, we demonstrate, for the first time, successful extraction and detection of a melanoma biomarker (S100B) using immunodiagnostic microneedles in the 3D culture system. Melanoma cells (A375) were cultured and expanded for 35 days in the highly porous polymeric scaffold followed by in situ capture of S100B with the microneedle device. Scanning electron microscopy showed a close resemblance between the 3D scaffold and human skin in terms of internal structure and porosity. The microneedle device detected S100B in the scaffold (with a detection pattern similar to the positive controls), while the biomarker was not detected in the surrounding liquid supernatants. Our findings demonstrate the great potential of this animal-free 3D tool for rapid and low-cost evaluation of microneedle devices.
Planning optimization considering various uncertainties has attracted increasing attentions in the process industry. In the existing studies, the uncertainty is often described with a time-invariant distribution function during the entire planning horizon, which is a questionable assumption. Particularly, for long-term planning problems, the uncertainty tends to vary with time and it usually increases when a model is used to predict the parameter (e.g. price) far into the future. In this paper, time-varying uncertainties are considered in robust planning problems with a focus on a polyvinyl chloride (PVC) production planning problem. Using the stochastic programming techniques, a stochastic model is formulated, and then transformed into a multi-period mixed-integer linear programming (MILP) model by chance constrained programming and piecewise linear approximation. The proposed approach is demonstrated on industrial-scale cases originated from a real-world PVC plant. The comparisons show that the model considering varying-uncertainty is superior in terms of robustness under uncertainties.
In this study, a multi-fidelity surrogate modeling method based on physics-informed neural network (PINN) was proposed, which integrates high-fidelity simulation data and low-fidelity governing equations described by differential equations. By leveraging governing equations in the training of deep neural networks, the reliance on large amount of data has been relaxed. In the meantime, imposing physical laws ensures that the achieved surrogate models have clear physical meanings, which also improves the extrapolation performance of the models. Herein, the proposed multi-fidelity PINN surrogate modeling method was implemented to the simulation of the startup phase of a continuous stirred-tank reactor (CSTR) for illustrating its feasibility and advantages. From the computer experiment results, it is observed that the proposed method successfully reduced the sample size needed in model training and significantly improved the model extrapolation performance, facilitating its potential industrial applications.
Aquaculture is a growing industry that provides high-quality protein, however, the growth of aquaculture production has a range of environmental concerns. Recirculating aquaculture systems (RAS) are a popular solution to accomplish intensifying fish production, and integrating renewable technologies is an attractive option that may provide low-carbon energy and promote sustainability. This paper presents an optimisation model for the control and operation of a grid-connected distributed energy system (DES) integrated with an RAS. A linear optimal power flow (OPF) combined with model predictive control (MPC) strategy is developed which simulates the thermal and electricity balances present throughout the renewable energy integrated electricity network and RAS, to make real-time optimal operation scheduling plans on different time horizons. The optimal results show that the MPC strategy improves the control performance of system operation and improves the process economics. With renewable energy sources (RES), operational costs can be reduced by up to 27%. Compared with a conventional scheduling plan, the rolling horizon approach can provide 3% energy cost saving, while maintaining fish well-being and system safety.
Fault detection and diagnosis is a crucial approach to ensure safe and efficient operation of chemical processes. This paper reports a new fault diagnosis method that exploits dynamic process simulation and pattern matching techniques. The proposed method consists of a simulated fault database which, through pattern matching, helps narrow down the fault candidates in an efficient way. An optimisation based fault reconstruction method is then developed to determine the fault pattern from the candidates, and the corresponding magnitude and time of occurrence of the fault. A major advantage of this approach is capable of diagnosing both single and multiple faults. We illustrate the effectiveness of the proposed method through case studies of the Tennessee Eastman benchmark process.
Optimizing scheduling is an effective way to improve the profit of refineries; it usually requires accurate models to describe the complex and nonlinear refining processes. However, conventional nonlinear models will result in a complex mixed integer nonlinear programming (MINLP) problem for scheduling. This paper presents a piecewise linear (PWL) modeling approach, which can describe global nonlinearity with locally linear functions, to refinery scheduling. Specifically, a high level canonical PWL representation is adopted to give a simple yet effective partition of the domain of decision variables. Furthermore, a unified partitioning strategy is proposed to model multiple response functions defined on the same domain. Based on the proposed PWL partitioning and modeling strategy, the original MINLP can be replaced by mixed integer linear programming (MILP), which can be readily solved using standard optimization algorithms. The effectiveness of the proposed strategy is demonstrated by a case study originated from a refinery in China.
This work presents a global sensitivity analysis and simulations of a perfusion bioreactor process using the method of high-dimensional model representation (HDMR). This method was developed to express the input–output relationships of a complex model with a high dimensional input space. The comprehensive mathematical model of convection and diffusion in a perfusion bioreactor, combined with cell growth kinetics, is developed and implemented using Computational Fluid Dynamics (with the commercial software COMSOL Multiphysics v5.5). The model describes the spatio-temporal evolution of glucose concentration, oxygen concentration, lactate concentration and cell density within a 3D polymeric scaffold. A quantitative analysis of the complex kinetic mechanisms using recent development of advanced mathematical approaches to global sensitivity and uncertainty analysis through HDMR can be exploited to investigate the important features of the perfusion bioreactor process as well as possible factors underlying qualitative discrepancies.
This paper uses the method of high-dimensional model representation (HDMR) to present a global sensitivity analysis and simulations of a perfusion bioreactor operation. This method enables the analysis of the input-output relationships of a complex model containing a high dimensional input space focusing on the parameters that could be used in the control of a perfusion bioreactor process. The model used in this work is a comprehensive mathematical model for diffusion and convection for a perfusion bioreactor, which is combined with cell growth kinetics that describes the spatio-temporal glucose concentration, oxygen concentration, lactate concentration and cell density evolution in a 3D polymeric scaffold. To investigate the important features of the perfusion bioreactor process as well as possible factors underlying qualitative discrepancies a quantitative analysis of the complex kinetic mechanisms using recent development of advanced mathematical approaches to global sensitivity and uncertainty analysis through HDMR is used. Assessing the effect of the model inputs on the model's predicted outputs is an important step especially when trying to develop control strategies
The paper presents a safe control method for the operation of dense medium coal preparation process based on Bayesian network (BN) and active learning. The dense medium coal preparation process fluctuates significantly in operating conditions and abnormal operating conditions occur frequently due to the uncertainty in the environment. To address this issue, we establish the BN model of safety control by integrating qualitative expert knowledge and quantitative data information based on the analysis of causes of abnormal conditions and corresponding operation schemes, which acts as the basis for the safety control. Because the previous BN structure learning method based on observation data information can only get Markov equivalence class and needs a large amount of data, it is difficult to establish an accurate BN. To address this issue, we propose a BN structure learning method based on active learning to improve its efficiency and accuracy. Simulation results show that the proposed method can effectively provide safety control decisions for abnormal conditions in the dense medium coal preparation process.
TP274; 针对过程数据不足,且具有强非线性和多尺度特性的新间歇过程,结合迁移学习方法与多尺度核学习方法的优势,提出了一种基于多尺度核JYMKPLS(Joint-Y multi-scale kernel partial least squares)迁移模型的间歇过程产品质量在线预测方法.该方法首先通过迁移学习利用相似源域的旧过程数据提高新间歇过程建模效率和质量预测的精度.然后,针对间歇过程数据的非线性和多尺度特性问题,引入了多尺度核函数以更好地拟合数据变化的趋势,从而提高模型的预测精度.此外,提出模型在线更新和数据剔除,通过在线持续改善迁移模型对新间歇过程的匹配程度,以消除相似过程间的差异性给迁移学习带来的不利影响,从而不断地提升预测精度.最后,通过仿真验证了所提方法的有效性,结果表明,与传统的数据驱动建模方法相比,本文所提方法能够有效提高建模效率和预测精度.
Gas explosions are destructive disasters in coal mines. Coal mine gas is a multi-component gas mixture, with methane (CH₄) being the dominant constituent. Understanding the process and mechanism of mine gas explosions is of critical importance to the safety of mining operations. In this work, three flammable gases (CO, C₂H₆, and H₂) which are commonly present in coal mines were selected to explore how they affect a methane explosion. The explosion characteristics of the flammable gases were investigated in a 20 L spherical closed vessel. Experiments on binary- (CH₄/CO, CH₄/C₂H₆, and CH₄/H₂) and multicomponent (CH₄/CO/C₂H₆/H₂) mixtures indicated that the explosion of such mixtures is more dangerous and destructive than that of methane alone in air, as measured by the explosion pressure. Furthermore, a self-promoting microcirculation reaction network is proposed to help analyze the chemical reactions involved in the multicomponent (CH₄/CO/C₂H₆/H₂) gas explosion. This work will contribute to a better understanding of the explosion mechanism of gas mixtures in coal mines and provide a useful reference for determining the safety limits in practice.
Identification of faulty variables is an important component of multivariate statistical process monitoring (MSPM); it provides crucial information for further analysis of the root cause of the detected fault. The main challenge is the large number of combinations of process variables under consideration, usually resulting in a combinatorial optimization problem. This paper develops a generic reconstruction based multivariate contribution analysis (RBMCA) framework to identify the variables that are the most responsible for the fault. A branch and bound (BAB) algorithm is proposed to efficiently solve the combinatorial optimization problem. The formulation of the RBMCA does not depend on a specific model, which allows it to be applicable to any MSPM model. We demonstrate the application of the RBMCA to a specific model: the mixture of probabilistic principal component analysis (PPCA mixture) model. Finally, we illustrate the effectiveness and computational efficiency of the proposed methodology through a numerical example and the benchmark simulation of the Tennessee Eastman process. © 2012 Elsevier Ltd. All rights reserved.
Despite a number of studies showed that hair follicular pathway contributed significantly to transdermal delivery, there have been limited studies on the diffusion properties of chemicals in sebum. Here, the diffusion property of 17 chemical compounds across artificial sebum has been measured using diffusion cell. The diffusion flux showed two types of distinctive behaviors: that reached steady-state and that did not. Mathematical models have been developed to fit the experimental data and derive the sebum diffusion and partition coefficients. The models considered the uneven thickness of the sebum film and the additional resistance of the unstirred aqueous boundary layer and the supporting filter. The derived sebum-water partition coefficients agreed well with the experimental data measured previously using equilibrium depletion method. The obtained diffusion coefficients in artificial sebum only depended on the molecular size. Change in pH for ionic chemicals did not affect the diffusion coefficients but influenced their diffusion flux due to the change of sebum-water partition coefficients. Generally, the measured diffusion coefficients of chemicals in artificial sebum are about one order of magnitude higher than those in the stratum corneum lipids, suggesting the hair follicle might have a non-negligible contribution to the overall permeation.
In this paper, a new Lagrange relaxation based decomposition algorithm for the integrated offshore oil production planning optimization is presented. In our previous study (Gao et al. Computers and Chemical Engineering, 2020, 133, 106674), a multiperiod mixed-integer nonlinear programming (MINLP) model considering both well operation and flow assurance simultaneously had been proposed. However, due to the large-scale nature of the problem, i.e., too many oil wells and long planning time cycle, the optimization problem makes it difficult to get a satisfactory solution in a reasonable time. As an effective method, Lagrange relaxation based decomposition algorithms can provide more compact bounds and thus result in a smaller duality gap. Specifically, Lagrange multiplier is introduced to relax coupling constraints of multi-batch units and thus some moderate scale sub-problems result. Moreover, dual problem is constructed for iteration. As a result, the original integrated large-scale model is decomposed into several single-batch subproblems and solved simultaneously by commercial solvers. Computational results show that the proposed method can reduce the solving time up to 43% or even more. Meanwhile, the planning results are close to those obtained by the original model. Moreover, the larger the problem size, the better the proposed LR algorithm is than the original model.
The basis of dynamic data rectification is a dynamic process model. The successful application of the model requires the fulfilling of a number of objectives that are as wide-ranging as the estimation of the process states, process signal denoising and outlier detection and removal. Current approaches to dynamic data rectification include the conjunction of the Extended Kalman Filter (EKF) and the expectation-maximization algorithm. However, this approach is limited due to the EKF being less applicable where the state and measurement functions are highly non-linear or where the posterior distribution of the states is non-Gaussian. This paper proposes an alternative approach whereby particle filters, based on the sequential Monte Carlo method, are utilized for dynamic data rectification. By formulating the rectification problem within a probabilistic framework, the particle filters generate Monte Carlo samples from the posterior distribution of the system states, and thus provide the basis for rectifying the process measurements. Furthermore, the proposed technique is capable of detecting changes in process operation and thus complements the task of process fault diagnosis. The appropriateness of particle filters for dynamic data rectification is demonstrated through their application to an illustrative non-linear dynamic system, and a benchmark pH neutralization process.
In the field of response surface methodology (RSM), the prediction uncertainty of the empirical model needs to be considered for effective process optimisation. Current methods combine the prediction mean and uncertainty through certain weighting strategies, either explicitly or implicitly, to form a single objective function for optimisation. This paper proposes to address this problem under the multi-objective optimisation framework. Overall, the method iterates through initial experimental design, empirical modelling and model-based optimisation to allocate promising experiments for the next iteration. Specifically, the Gaussian process regression is adopted as the empirical model due to its demonstrated prediction accuracy and reliable quantification of prediction uncertainty in the literature. The non-dominated sorting genetic algorithm II (NSGA-II) is used to search for Pareto points that are further clustered to give experimental points to be conducted in the next iteration. The application study, on the optimisation of a catalytic epoxidation process, demonstrates that the proposed method is a powerful tool to aid the development of chemical and potentially other processes. © 2011 The Institution of Chemical Engineers.
Owing to their health-boosting properties and other appreciable properties, citrus fruit is widely consumed and commercialized worldwide. Destination markets around the world vary in their fruit quality requirements and are also highly influenced by climatic conditions, agronomical and postharvest practices. Hence, harvesting decisions are arduous. Maturity indices in citrus fruit are highly variable and dependent on the species and varieties, growing regions, and destination markets. For decades, determination of the maturity of citrus fruit and predicting the near time of harvesting was a challenge for producers, researchers, and food safety agencies. Thus, the current review provides a correlation between maturity and internal components and an overview of techniques of maturity determination for citrus fruits. Also, stress has been given to the destructive and nondestructive methods to determine the maturity level of different citrus species. The techniques presented in this review portray continuous productiveness as an excellent quality assessment, particularly as ripening and maturity analysis tools for citrus fruits. Traditional techniques are time-consuming, laborious, costly, destructive, and tedious. Thus, these nondestructive techniques hold great potential to replace conventional procedures.
The advantages of maximally transferring similar process data for modeling make the process transfer model attract increasing attention in quality prediction and optimal control. Unfortunately, due to the difference between similar processes and the uncertainty of data-driven model, there are usually a more serious mismatch between the process transfer model and the actual process, which may result in the deterioration of process transfer model-based control strategies. In this research, a process transfer model based optimal compensation control strategy using just-in-time learning and trust region method is proposed to cope with this problem for batch processes. First, a novel JITL-JYKPLS (Just-in-time learning Joint-Y kernel partial least squares) model combining the JYKPLS (Joint-Y kernel partial least squares) process transfer model and just-in-time learning is proposed and employed to obtain the satisfactory approximation in a local region with the assistance of sufficient similar process data. Then, this paper integrates JITL-JYKPLS model with the trust region method to further compensate for the NCO (necessary condition of optimality) mismatch in the batch-to-batch optimization problem, and the problem of estimating experimental gradients is also avoided. Meanwhile, a more elaborate model update scheme is designed to supplement the lack of new data and gradually eliminate the adverse effects of partial differences between similar process production processes. Finally, the feasibility of the proposed optimal compensation control strategy is demonstrated through a simulated cobalt oxalate synthesis process. (C) 2020 Published by Elsevier Ltd on behalf of The Franklin Institute.
The in vitro skin penetration of pharmaceutical or cosmetic ingredients is usually assessed in human or animal tissue. However, there are ethical and practical difficulties associated with sourcing these materials; variability between donors may also be problematic when interpreting experimental data. Hence, there has been much interest in identifying a robust and high throughput model to study skin permeation that would generate more reproducible results. Here we investigate the permeability of a model active, niacinamide (NIA), in (i) conventional vertical Franz diffusion cells with excised human skin or porcine skin and (ii) a recently developed Parallel Artificial Membrane Permeation Assay (PAMPA) model. Both finite and infinite dose conditions were evaluated in both models using a series of simple NIA solutions and one commercial preparation. The Franz diffusion cell studies were run over 24 h while PAMPA experiments were conducted for 2.5 h. A linear correlation between both models was observed for the cumulative amount of NIA permeated in tested models under finite dose conditions. The corresponding correlation coefficients (r²) were 0.88 for porcine skin and 0.71 for human skin. These results confirm the potential of the PAMPA model as a useful screening tool for topical formulations. Future studies will build on these findings and expand further the range of actives investigated.
Background and Purpose Audit is imperative in delivering consistent and safe radiotherapy and the UK has a strong history of radiotherapy audit. The National Physical Laboratory (NPL) has undertaken audit measurements since 1994 and this work examines results from these audits. Materials and Methods This paper reviews audit results from 209 separate beams from 82 on-site visits to National Health Service (NHS) radiotherapy departments conducted between June 1994 and February 2015. Measurements were undertaken following the relevant UK code of practice. The accuracy of the implementation of absorbed dose calibration across the UK is quantified for MV photon, MeV electron and kV x-ray radiotherapy beams. Results Over the measurement period the standard deviation of MV photon beam output has reduced from 0.8 % to 0.4 %. The switch from air kerma- to absorbed dose-based electron code of practice contributed to a reduction in the difference of electron beam output of 0.6 % (p < 0.01). The mean difference in NPL to local measurement for radiation output calibration was less than 0.25 % for all beam modalities. Conclusions The introduction of the 2003 electron code of practice based on absorbed dose to water decreased the difference between absolute dose measurements by the centre and NPL. The use of a single photon code of practice over the period of measurements has contributed to a reduction in measurement variation. Within the clinical setting, on-site audit visits have been shown to identify areas of improvement for determining and implementing absolute dose calibrations.
Refinery scheduling attracts increasing concerns in both academic and industrial communities in recent years. However, due to the complexity of refinery processes, little has been reported for success use in real world refineries. In academic studies, refinery scheduling is usually treated as an integrated, large-scale optimization problem, though such complex optimization problems are extremely difficult to solve. In this paper, we proposed a way to exploit the prior knowledge existing in refineries, and developed a decision making system to guide the scheduling process. For a real world fuel oil oriented refinery, ten adjusting process scales are predetermined. A C4.5 decision tree works based on the finished oil demand plan to classify the corresponding category (i.e. adjusting scale). And then, a specific sub-scheduling problem with respect to the determined adjusting scale is solved. The proposed strategy is demonstrated with a scheduling case originated from a real world refinery.
This paper presents a spreadsheet calculator to estimate biogas production and the operational revenue and costs for UK-based farm-fed anaerobic digesters. There exist sophisticated biogas production models in published literature, but the application of these in farm-fed anaerobic digesters is often impractical. This is due to the limited measuring devices, financial constraints, and the operators being non-experts in anaerobic digestion. The proposed biogas production model is designed to use the measured process variables typically available at farm-fed digesters, accounting for the effects of retention time, temperature and imperfect mixing. The estimation of the operational revenue and costs allow the owners to assess the most profitable approach to run the process. This would support the sustained use of the technology. The calculator is first compared with literature reported data, and then applied to the digester unit on a UK Farm to demonstrate its use in a practical setting.
Purpose: Sebum is an important shunt pathway for transdermal permeation and targeted delivery, but there have been limited studies on its permeation properties. Here we report a measurement and modelling study of solute partition to artificial sebum. Methods: Equilibrium experiments were carried out for the sebum-water partition coefficients of 23 neutral, cationic and anionic compounds at different pH. Results: Sebum-water partition coefficients not only depend on the hydrophobicity of the chemical but also on pH. As pH increases from 4.2 to 7.4, the partition of cationic chemicals to sebum increased rapidly. This appears to be due to increased electrostatic attraction between the cationic chemical and the fatty acids in sebum. Whereas for anionic chemicals, their sebum partition coefficients are negligibly small, which might result from their electrostatic repulsion to fatty acids. Increase in pH also resulted in a slight decrease of sebum partition of neutral chemicals. Conclusions: Based on the observed pH impact on the sebum-water partition of neutral, cationic and anionic compounds, a new quantitative structure-property relationship (QSPR) model has been proposed. This mathematical model considers the hydrophobic interaction and electrostatic interaction as the main mechanisms for the partition of neutral, cationic and anionic chemicals to sebum.
Background and purpose: Dose delivered during radiotherapy has uncertainty arising from a number of sources including machine calibration, treatment planning and delivery and can impact outcomes. Any systematic uncertainties will impact all patients and can continue for extended periods. The impact on tumour control probability (TCP) of the uncertainties within the radiotherapy calibration process has been assessed. Materials and methods: The linear-quadratic model was used to simulate the TCP from two prostate cancer and a head and neck (H&N) clinical trial. The uncertainty was separated into four components; 1) initial calibration, 2) systematic shift due to output drift, 3) drift during treatment and 4) daily fluctuations. Simulations were performed for each clinical case to model the variation in TCP present at the end of treatment arising from the different components. Results: Overall uncertainty in delivered dose was +/-2.1% (95% confidence interval (CI)), consisting of uncertainty standard deviations of 0.7% in initial calibration, 0.8% due to subsequent calibration shift due to output drift, 0.1% due to drift during treatment, and 0.2% from daily variations. The overall uncertainty of TCP (95% CI) for a population of patients treated on different machines was +/-3%, +/-5%, and +/-3% for simulations based on the two prostate trials and H&N trial respectively. Conclusion: The greatest variation in delivered target volume dose arose from calibration shift due to output drift. Careful monitoring of beam output following initial calibration remains vital and may have a significant impact on clinical outcomes.
With the increasing energy requirement and decreasing onshore reserves, offshore oil production has attracted increasing attention. A major challenge in offshore oil production is to minimize both the operational costs and risks; one of the major risks is anomalies in the flows. However, optimization methods to simultaneously consider well operation and flow assurance in operation planning have not been explored. In this paper, an integrated planning problem both considering well operation and flow assurance is reported. In particular, a multi-period mixed integer nonlinear programming (MINLP) model was proposed to minimize the total operation cost, taking into account of well production state, polymer flooding, energy consumption, platform inventory and flow assurance. By solving this integrated model, each well's working state, flow rates and chemicals injection rates can be optimally determined. The proposed model was applied to a case originated from a real-world offshore oil site and the results illustrate the effectiveness.
The addition of organic phosphorus-containing flame retardants (OPFRs) has greatly improved the fire resistance of epoxy resins (EPs). Developing the relationship of the fire resistance with the structure of OPFRs and their addition amount will help discover high-performance EP composites, which was achieved in this work by machine learning (ML). By combining descriptors encoded from OPFR molecules and the addition amount as features, an ML model with the limiting oxygen index (LOI) as the target was developed with a coefficient of determination (R2) of the ML model on the test set of 0.642. The trained ML model indicated that fire retardants containing conjugated systems with penta-substituted phosphorus containing a P=O bond and the nitrogen element can significantly increase the LOI of EPs, which led to the synthesis of a 9,10-dihydro-9-oxa-10-phosphaphenanthrene-10-oxide derivative (BDOPO) in this work. Furthermore, the accuracy of the ML model was validated through experiments. The predicted LOI values of the EP/BDOPO composites followed the same trend as the experimental values, with an average error of 5.1%. The model can also illustrate the molecular structure required for synthesizing an OPFR and predict the amount of this OPFR to be added into EPs for enhanced LOI of the EPs.
This paper introduces a Gaussian process regression (GPR) model which could adapt to both linear and nonlinear systems automatically without prior introduction of kernel functions. The applications of GPR model for two industrial examples are presented. The first example addresses a biological anaerobic system in a wastewater treatment plant and the second models a nonlinear dynamic process of propylene polymerization. Special emphasis is placed on signal preprocessing methods including the Savitzky-Golay and Kalman filters. Applications of these filters are shown to enhance the performance of the GPR model, and facilitate bias update leading to reduction of the offset between the predicted and measured values. © 2012 Elsevier Ltd.
To better utilize historical process data from faulty operations, supervised learning methods, such as Fisher discriminant analysis (FDA), have been adopted in process monitoring. However, such methods can only separate known faults from normal operations, and they have no means to deal with unknown faults. In addition, most of these methods are not designed for handling non-Gaussian distributed data; however, non-Gaussianity is frequently observed in industrial processes. In this paper, a hybrid multivariate approach named mixture discriminant monitoring (MDM) was proposed, in which supervised learning and statistical process control (SPC) charting techniques are integrated. MDM is capable of solving both of the above problems simultaneously during online process monitoring. Then, for known faults, a root-cause diagnosis can be automatically achieved, while for unknown faults, abnormal variables can be isolated through missing variable analysis. MDM was used on the benchmark Tennessee Eastman (TE) process, and the results showed the capability of the proposed approach.
Purpose: To investigate the role of symptom clusters in the analysis and utilisation of Patient-Reported Outcome Measures (PROMs) for data modelling and clinical practice. To compare symptom clusters with scales, and explore their value in PROMs interpretation and symptom management. Methods: A dataset called RT01 (ISCRTN47772397) of 843 prostate cancer patients was used. PROMs were reported with the University of California, Los Angeles Prostate Cancer Index (UCLA-PCI). Symptom clusters were explored with hierarchical cluster analysis (HCA) and average linkage method (correlation >0.6). The reliability of the Urinary Function Scale was evaluated with Cronbach's Alpha. The strength of the relationship between the items was investigated with Spearman's correlation. Predictive accuracy of the clusters was compared to the scales by receiver operating characteristic (ROC) analysis. Presence of urinary symptoms at 3 years measured with the Late Effects on Normal Tissue: Subjective, Objective, Management tool (LENT/SOM) was an endpoint. Results: Two symptom clusters were identified (Urinary Cluster and Sexual Cluster). The grouping of symptom clusters was different than UCLA-PCI Scales. Two items of the Urinary Function Scales (“Number of pads” and “Urinary leak interfering with sex”) were excluded from the Urinary Cluster. The correlation with the other items in the scale ranged from 0.20-0.21 and 0.31-0.39 respectively. Cronbach's Alpha showed low correlation of those items with the Urinary Function Scale (0.14-0.36 and 0.33-0.44 respectively). All Urinary Function Scale items were subject to a ceiling effect. Clusters had better predictive accuracy, AUC = 0.70-0.65, while scales AUC = 0.67-0.61. Conclusion: This study adds to the knowledge on how cluster analysis can be applied for the interpretation and utilisation of PROMs. We conclude that multiple-item scales should be evaluated and that symptom clusters provide an adaptive and study specific approach for modelling and interpretation of PROMs.
The primary objective of this note is to reduce the falsealarms in multivariatestatisticalprocesscontrol (MSPC). The issue of falsealarms is inherent within MSPC as a result of the definition of control limits. It has been observed that under normal operating conditions, the occurrence of “out-of-control” data, i.e. falsealarms, conforms to a Bernoulli distribution. Therefore, this issue can be formally addressed by developing a Binomial distribution for the number of “out-of-control” data points within a given time window, and a second-level control limit can be established to reduce the falsealarms. This statistical approach is further extended to consider the combination of multiple control charts. The proposed methodology is demonstrated through its application to the monitoring of a benchmark simulated chemical process, and it is observed to effectively reduce the falsealarms whilst retaining the capability of detecting process faults.
Probabilistic models, including probabilistic principal component analysis (PPCA) and PPCA mixture models, have been successfully applied to statisticalprocess monitoring. This paper reviews these two models and discusses some implementation issues that provide alternative perspective on their application to process monitoring. Then aprobabilisticcontributionanalysis method, based on the concept of missingvariable, is proposed to facilitate the diagnosis of the source behind the detected process faults. The contributionanalysis technique is demonstrated through its application to both PPCA and PPCA mixture models for the monitoring of two industrial processes. The results suggest that the proposed method in conjunction with PPCA model can reduce the ambiguity with regard to identifying the processvariables that contribute to process faults. More importantly it provides a fault identification approach for PPCA mixture model where conventional contributionanalysis is not applicable.
Proportional-integral-derivative (PID) controller design based on the Gaussian process (GP) model is proposed in this study. The GP model, defined by its mean and covariance function, provides predictive variance in addition to the predicted mean. GP model highlights areas where prediction quality is poor, due to the lack of data, by indicating the higher variance around the predicted mean. The variance information is taken into account in the PID controller design and is used for the selection of data to improve the model at the successive stage. This results in a trade-off between safety and the performance due to the controller avoiding the region with large variance at the cost of not tracking the set point to ensure process safety. The proposed direct method evaluates the PID controller design by the gradient calculation. In order to reduce computation the characteristic of the instantaneous linearized GP model is extracted for a linearized framework of PID controller design. Two case studies on continuous and batch processes were carried out to illustrate the applicability of the proposed method.
The statistical monitoring of batch manufacturing processes is considered. It is known that conventional monitoring approaches, e.g. principal component analysis (PCA), are not applicable when the normal operating conditions of the process cannot be sufficiently represented by a Gaussian distribution. To address this issue, Gaussian mixture model (GMM) has been proposed to estimate the probability density function of the process nominal data, with improved monitoring results having been reported for continuous processes. This paper extends the application of GMM to on-line monitoring of batch processes, and the proposed method is demonstrated through its application to a batch semiconductor etch process.
Computational fluid dynamics (CFD) is a simulation technique widely used in chemical and process engineering applications. However, computation has become a bottleneck when calibration of CFD models with experimental data (also known as model parameter estimation) is needed. In this research, the kriging meta-modelling approach (also termed Gaussian process) was coupled with expected improvement (EI) to address this challenge. A new EI measure was developed for the sum of squared errors (SSE) which conforms to a generalised chi-square distribution and hence existing normal distribution-based EI measures are not applicable. The new EI measure is to suggest the CFD model parameter to simulate with, hence minimising SSE and improving match between simulation and experiments. The usefulness of the developed method was demonstrated through a case study of a single-phase flow in both a straight-type and a convergent-divergent-type annular jet pump, where a single model parameter was calibrated with experimental data.
The least absolute shrinkage and selection operator (LASSO) is an established sparse representation approach for variable selection, and its performance relies on finding a good value for the regularization parameter, typically through cross-validation. However, cross-validation is a computationally intensive step and requires a properly determined search range and step size. In the present study, the ridge-adding homotopy (RAH) algorithm is applied with LASSO to overcome the aforementioned shortcomings. The homotopy algorithm can fit the entire solution of the LASSO problem by tracking the Karush-Kuhn Tucker (KKT) conditions and yields a finite number of potential regularization parameters. Considering the singularities, a M×1 random ridge vector will be added to the KKT conditions, which ensures that only one element is added to or removed from the active set. Finally, we can select the optimal regularization parameter by traversing the potential parameters with modelling and evaluation metrics. The selected variables are the nonzero elements in the sparse regression coefficient vector derived by the optimal regularization parameter. The proposed method has been demonstrated on three near-infrared (NIR) datasets with regard to wavelength selection and calibration. The results suggested that the “RAH-LASSO + PLS” outperforms “LASSO + PLS” and “full-wavelength PLS” in most cases. Importantly, the RAH method provides a systematic, as opposed to trial-and-error, procedure to determine the regularization parameter in LASSO.
Fault diagnosis plays a vital role in ensuring safe and efficient operation of modern process plants. Despite the encouraging progress in its research, developing a reliable and interpretable diagnostic system remains a challenge. There is a consensus among many researchers that an appropriate modelling, representation and use of fundamental process knowledge might be the key to addressing this problem. Over the past four decades, different techniques have been proposed for this purpose. They use process knowledge from different sources, in different forms and on different details, and are also named model-based methods in some literature. This paper first briefly introduces the problem of fault detection and diagnosis, its research status and challenges. It then gives a review of widely used model- and knowledge-based diagnostic methods, including their general ideas, properties, and important developments. Afterwards, it summarises studies that evaluate their performance in real processes in process industry, including the process types, scales, considered faults, and performance. Finally, perspectives on challenges and potential opportunities are highlighted for future work.
This paper presents a new piecewise linear modeling method for the planning of polyvinyl chloride (PVC) plants. In our previous study (hid. Eng. Chem. Res., 2016, SS, 12430-12443, DOI: 10.1021/acs.iecr.6b02825), a multiperiod mixed-integer nonlinear programming (MINLP) model was developed to demonstrate the importance of integrating both the material processing and the utility systems. However, the optimization problem is really difficult to solve due to the process intrinsic nonlinearities, i.e., the operating cost or energy-consuming characteristics of calcium carbide furnaces, electrolytic cells, and CHP units. The present paper intends to address this challenge by using the piecewise linear modeling approach that provides good approximation of the global nonlinearity with locally linear models. Specifically, a hinging hyperplanes (HH) model is introduced to approximate the nonlinear items in the original MINLP model. HH model is a kind of continuous piecewise linear (CPWL) model, which is proven to be effective for any continuous linear functions with arbitrary dimensions on compact sets in any given precision, and is the basis for the linearization MINLP model. As a result, with the help of auxiliary variables, the original MINLP can be transformed into a mixed-integer linear program (MILP) model, which then can be solved by many established efficient and mature algorithms. Computational results show that the proposed model can reduce the solving time by up to 97% or more and the planning results are close to or even better than those obtained by the MINLP approach.
This work sets the foundations for the design of control algorithms to facilitate manufacturing of a cell growth process using a continuous perfusion bioreactor. The algorithms are designed to work with different types of cell cultures and deal with major disturbances that might appear in the process. Different types of control strategies are designed, implemented and tested. First, a comprehensive mathematical model of convection and diffusion in a perfusion bioreactor, combined with cell growth kinetics, is developed and implemented using Computational Fluid Dynamics. The model describes the spatio-temporal evolution of glucose concentration and cell density within a 3D polymeric scaffold. Since such a model is too complex to be used directly for control studies, a simplified version is used for the design of the controllers. Finally, the performances of the control strategies are validated against the original high-fidelity CFD model, thus closing the loop. The simulations show good performances and satisfactory behavior.
Fish feeding intensity assessment (FFIA) aims to evaluate the intensity change of fish appetite during the feeding process, which is vital in industrial aquaculture applications. The main challenges surrounding FFIA are two-fold. 1) robustness: existing work has mainly leveraged single-modality (e.g., vision, audio) methods, which have a high sensitivity to input noise. 2) efficiency: FFIA models are generally expected to be employed on devices. This presents a challenge in terms of computational efficiency. In this work, we first introduce an audio-visual dataset, called AV-FFIA. AV-FFIA consists of 27,000 labeled audio and video clips that capture different levels of fish feeding intensity. To our knowledge, AV-FFIA is the first large-scale multimodal dataset for FFIA research. Then, we introduce a multi-modal approach for FFIA by leveraging single-modality pre-trained models and modality-fusion methods, with benchmark studies on AV-FFIA. Our experimental results indicate that the multi-modal approach substantially outperforms the single-modality based approach, especially in noisy environments. While multimodal approaches provide a performance gain for FFIA, it inherently increase the computational cost. To overcome this issue, we further present a novel unified model, termed as U-FFIA. U-FFIA is a single model capable of processing audio, visual, or audio-visual modalities, by leveraging modality dropout during training and knowledge distillation from single-modality pre-trained models. We demonstrate that U-FFIA can achieve performance better than or on par with the state-of-the-art modality-specific FFIA models, with significantly lower computational overhead. Our proposed U-FFIA approach enables a more robust and efficient method for FFIA, with the potential to contribute to improved management practices and sustainability in aquaculture.
The dermal absorption potential of 14C-Caffeine applied as a 4 mg/mL concentration (10 μL/cm2 finite dose) was investigated in six laboratories under Good Laboratory Practice conditions using an OECD TG 428-compliant in vitro assay with flow-through cells and split-thickness human skin. Potential sources of variation were reduced by a standardized protocol, test item and skin source. Particularly, skin samples from same donors were distributed over two repeats and between labs in a non-random, stratified design. Very similar recovery was achieved in the various assay compartments between laboratories, repeats and donors, demonstrating that the assay can be robustly and reliably performed. The absorption in one laboratory was 5-fold higher than in the others. This did not clearly correlate with skin integrity parameters but might be associated with an accidental COVID-19 pandemic-related interruption in sample shipment. It is possible that other factors may affect dermal absorption variation not routinely assessed or considered in the current method. The mean receptor fluid recovery, potential absorption (recovery in receptor fluid and skin except tape strips 1 and 2) and mass balance of caffeine was 6.99%, 7.14% and 99.13%, respectively, across all and 3.87%, 3.96% and 99.00% in the subset of five laboratories.
non-linear regression techniques have been widely used for data-based modeling of chemical processes, and they form the basis of process design under the framework of response surface methodology (RSM). These non-linear models typically achieve more accurate approximation to the factor–response relationship than traditional polynomial regressions. However, non-linear models usually lack a clear interpretation as to how the factors contribute to the prediction of process response. This paper applies the technique of sensitivity analysis (SA) to facilitate the interpretation of non-linear process models. By recognizing that derivative-based local SA is only valid within the neighborhood of certain “nominal” values, global SA is adopted to study the entire range of the factors. Global SA is based on the decomposition of the model and the variance of response into contributing terms of main effects and interactions. Therefore, the effect of individual factors and their interactions can be both visualized by graphs and quantified by sensitivity indices. The proposed methodology is demonstrated on two catalysis processes where non-linear data-based models have been developed to aid process design. The results indicate that global SA is a powerful tool to reveal the impact of process factors on the response variables.
Gaussian process regression (GPR) has been shown to be a powerful and effective non- parametric method for regression, classification and interpolation, due to many of its desirable properties. However, most GPR models consider univariate or multivariate covariates only. In this paper we extend the GPR models to cases where the covariates include both functional and multivariate variables and the response is multidimen- sional. The model naturally incorporates two different types of covariates: multivari- ate and functional, and the principal component analysis is used to de-correlate the multivariate response which avoids the widely recognised difficulty in the multi-output GPR models of formulating covariance functions which have to describe the correla- tions not only between data points but also between responses. The usefulness of the proposed method is demonstrated through a simulated example and two real data sets in chemometrics.
Recent development of non-destructive optical techniques, such as spectroscopy and machine vision technologies, have laid a good foundation for real-time monitoring and precise management of crop N status. However, their advantages and disadvantages have not been systematically summarized and evaluated. Here, we reviewed the state-of-the-art of non-destructive optical methods for monitoring the N status of crops, and summarized their advantages and disadvantages. We mainly focused on the contribution of spectral and machine vision technology to the accurate diagnosis of crop N status from three aspects: system selection, data processing and estimation methods. Finally, we discussed the opportunities and challenges of the application of these technologies, followed by recommendations for future work to address the challenges.
Chronic wounds and their treatment present a significant burden to patients and healthcare systems alike, with their management further complicated by bacterial infection. Historically, antibiotics have been deployed to prevent and treat infections, but the emergence of bacterial antimicrobial resistance and the frequent development of biofilms within the wound area necessitates the identification of novel treatment strategies for use within infected chronic wounds. Here, several non-antibiotic compounds, polyhexamethylene biguanide (PHMB), curcumin, retinol, polysorbate 40, ethanol, and D-& alpha;-tocopheryl polyethylene glycol succinate 1000 (TPGS) were screened for their antibacterial and antibiofilm capabilities. The minimum inhibitory concentration (MIC) and crystal violet (CV) biofilm clearance against two bacteria frequently associated with infected chronic wounds, Staphylococcus aureus and Pseudomonas aeruginosa, were determined. PHMB was observed to have highly effective antibacterial activity against both bacteria, but its ability to disperse biofilms at MIC levels was variable. Meanwhile, TPGS had limited inhibitory activity but demonstrated potent antibiofilm properties. The subsequent combination of these two compounds in a formulation resulted in a synergistic enhancement of their capability to kill both S. aureus and P. aeruginosa and disperse their biofilms. Collectively, this work highlights the utility of combinatory approaches to the treatment of infected chronic wounds where bacterial colonization and biofilm formation remains significant issues.
Correct detection of drilling abnormal incidents while minimizing false alarms is a crucial measure to decrease the non-productive time and thus decrease the total drilling cost. With the recent development of drilling technology and innovation of down-hole signal transmitting method, abundant drilling data are collected and stored in the electronic driller’s database. The availability of such data provides new opportunities for rapid and accurate fault detection; however, data-driven fault detection has seen limited practical application in well drilling processes. One particular concern is how to distinguish “controllable” process changes, e.g. due to set-point changes, from truly abnormal events that should be considered as faults. This is highly relevant for the managed pressure drilling (MPD) technology, where the operating pressure window is often narrow resulting in necessary set-point changes at different depths. However, the classical data-driven fault detection methods, such as principal component analysis (PCA) and independent component analysis (ICA), are unable to distinguish normal set-point changes from abnormal faults. To address this challenge, a slow feature analysis (SFA) based fault detection method is applied. SFA-based method furnishes four monitoring charts containing more information that could be synthetically utilized to correctly differentiate set-point changes from faults. Furthermore, the evaluation about controller performance is provided for drilling operator. Simulation studies with a commercial high-fidelity simulator, Drillbench, demonstrate the effectiveness of the introduced approach.
In order to monitor nonlinear processes, kernel principal component analysis (KPCA) has become a popular technique. Nevertheless, KPCA suffers from two major disadvantages. First, the underlying manifold structure of data is not considered in process modeling. Second, the selection of kernel function and kernel parameters is always problematic. To avoid such deficiencies, an integrating method of manifolding learning and Gaussian process is proposed in this paper, which extends the utilization of maximum variance unfolding (MVU) to online process monitoring and fault isolation. The proposed method is named as extendable MVU (EMVU), whose effectiveness is verified by the case studies on the benchmark Tennessee Eastman (TE) process. © 2013 Elsevier B.V.
This paper presents a model-aided approach to the development of catalysts for CO oxidation. This is in contrast to the traditional methodology whereby experiments are guided based on experience and intuition of chemists. The proposed approach operates in two stages. To screen a promising combination of active phase, promoter and support material, a powerful “space-filling” experimental design (specifically, Hammersley sequence sampling) was adopted. The screening stage identified Au–ZnO/Al2O3 as a promising recipe for further optimization. In the second stage, the loadings of Au and ZnO were adjusted to optimize the conversion of CO through the integration of a Gaussian process regression (GPR) model and the technique of maximizing expected improvement. Considering that Au constitutes the main cost of the catalyst, we further attempted to reduce the loading of Au with the aid of GPR, while keeping the low-temperature conversion to a high level. Finally we obtained 2.3%Au–5.0%ZnO/Al2O3 with 21 experiments. Infrared reflection absorption spectroscopy and hydrogen temperature-programmed reduction confirmed that ZnO significantly promotes the catalytic activity of Au.
Fault detection and diagnosis is a critical approach to ensure safe and efficient operation of manufacturing and chemical processing plants. Although multivariate statistical process monitoring has received considerable attention, investigation into the diagnosis of the source or cause of the detected process fault has been relatively limited. This is partially due to the difficulty in isolating multiple variables, which jointly contribute to the occurrence of fault, through conventional contribution analysis. In this work, a method based on probabilistic principal component analysis is proposed for fault isolation. Furthermore, a branch and bound method is developed to handle the combinatorial nature of problem involving finding the contributing variables, which are most likely to be responsible for the occurrence of fault. The efficiency of the method proposed is shown through benchmark examples, such as Tennessee Eastman process, and randomly generated cases.
Use of computational fluid dynamics to model chemical process system has received much attention in recent years. However, even with state-of-the-art computing, it is still difficult to perform simulations with many physical factors taken into accounts. Hence, translation of such models into computationally easy surrogate models is necessary for successful applications of such high fidelity models to process design optimization, scale-up and model predictive control. In this work, the methodology, statistical background and past applications to chemical processes of meta-model development were reviewed. The objective is to help interested researchers be familiarized with the work that has been carried out and problems that remain to be investigated.
Various neutral, cationic and anionic chemicals contained in hair care products can be absorbed into hair fiber to modulate physicochemical properties such as color, strength, style and volume. For environmental safety, there is also an interest in understanding hair absorption to wide chemical pollutants. There have been very limited studies on the absorption properties of chemicals into hair. Here, an experimental and modelling study has been carried out for the hair-water partition of a range of neutral, cationic and anionic chemicals at different pH. The data showed that hair-water partition not only depends on the hydrophobicity of the chemical but also the pH. The partition of cationic chemicals to hair increased with pH and this is due to their electrostatic interaction with hair increased from repulsion to attraction. For anionic chemicals, their hair-water partition coefficients decreased with increasing pH due to their electrostatic interaction with hair decreased from attraction to repulsion. Increase in pH didn’t change the partition of neutral chemicals significantly. Based on the new physicochemical insight of the pH effect on hair-water partition, a new QSPR model has been proposed, taking into account of both the hydrophobic interaction and electrostatic interaction of chemical with hair fiber.
This work explores the potentialities of combining a multi-layer eco-friendly panel with a phase change material coating. Although the work is based on a numerical approach performed by Comsol Multiphysics® computer program, it can be considered as rigorous, robust and optimized since the most important parameters added to the model were experimentally evaluated. The scientific soundness was guaranteed by a comparative analysis performed in two different times. The cork-coconut-cork panel was firstly investigated as it was, and secondly it was analysed with a phase change material layer applied on. In the second step, the panel undergone to a mechanical process concerning the realization of a subsurface defect simulating a detachment. The aim was based on the conduction of a thermal conductivity analysis to characterize the benefits deriving from the application of the coating, as well as the negative effects introduced by the subsurface defect resembling a potential thermal bridge. The experiments were performed in Italy in a place identified into the text by means of geographical coordinates.
Background and Purpose Motor failure in multi-leaf collimators (MLC) is a common reason for unscheduled accelerator maintenance, disrupting the workflow of a radiotherapy treatment centre. Predicting MLC replacement needs ahead of time would allow for proactive maintenance scheduling, reducing the impact MLC replacement has on treatment workflow. We propose a multivariate approach to analysis of trajectory log data, which can be used to predict upcoming MLC replacement needs. Materials and Methods Trajectory log files from two accelerators, spanning six and seven months respectively, have been collected and analysed. The average error in each of the parameters for each log file was calculated and used for further analysis. A performance index (PI) was generated by applying moving window principal component analysis to the prepared data. Drops in the PI were thought to indicate an upcoming MLC replacement requirement; therefore, PI was tracked with exponentially weighted moving average (EWMA) control charts complete with a lower control limit. Results The best compromise of fault detection and minimising false alarm rate was achieved using a weighting parameter (λ) of 0.05 and a control limit based on three standard deviations and an 80 data point window. The approach identified eight out of thirteen logged MLC replacements, one to three working days in advance whilst, on average, raising a false alarm, on average, 1.1 times a month. Conclusions This approach to analysing trajectory log data has been shown to enable prediction of certain upcoming MLC failures, albeit at a cost of false alarms.
The preparation of effective phosphorus-nitrogen flame retardants (PNFRs) is still limited using organic solvent. In this work, a solvent-free mechanochemical method was introduced to prepare a phosphorus-containing hypercrosslinked aromatic polymer (HCAP) from triphenylphosphine. Subsequently, nitrogen-rich graphitized carbon nitride was introduced to prepare a series of phosphorus and nitrogen-containing heterojunctions named HCN. The formation of the HCAP and HCN was verified by a combination of density functional theory (DFT) calculations and experiments. Afterwards, upon addition of 5 wt% 20HCN to epoxy resin (EP), the limiting oxygen index and vertical combustion test level reached 30.3% and V-0, respectively. The peak heat release rate, total heat release, peak smoke production rate and total smoke production were reduced by 41.2%, 38.4%, 34.9% and 36.0%, respectively, relative to those of the pure EP. The combined scores of multiple flame retardant properties were evaluated through machine learning. The mechanical properties and thermal conductivity remained at the same level as those of EP. These results confirmed the role of HCN in reducing the fire hazard of EP, stemming from its lamellar char and ability to blow out flames. This work provides a new method of preparing PNFRs. [Display omitted] •Solvent-free synthesized of a new phosphorus-nitrogen flame retardant (PNFR).•Combining density functional theory calculation and experiments.•The fire resistance of EP with this new PNFR was improved.•A lamellar char and a “blowing out” phenomenon were formed.•Machine learning was adopted to compare fire resistance.
Gaussianprocesses have received significant interest for statistical data analysis as a result of the good predictive performance and attractive analytical properties. When developing a Gaussianprocess regression model with a large number of covariates, the selection of the most informative variables is desired in terms of improved interpretability and prediction accuracy. This paper proposes a Bayesian method, implemented through the Markov chain Monte Carlo sampling, for variableselection. The methodology presented here is applied to the chemometriccalibration of near infrared spectrometers, and enhanced predictive performance and model interpretation are achieved when compared with benchmark regression method of partial least squares.
Solid acid catalysts were synthesized from macroalgae Sargassum horneri via hydrothermal carbonization followed by sulfuric acid sulfonation. A three-variable Box-Behnken design and optimization was used to maximize surface acidity. The optimal preparation conditions were found to be at the carbonization temperature of 217 °C, the carbonization time of 4.6 hours and the sulfonation temperature of 108.5 °C. Under these conditions, the highest surface acidity achieved was 1.62 mmol g-1. Physical and chemical properties of prepared solid acid catalyst were characterized by powder X-ray diffraction (PXRD), Fourier transform infrared (FTIR) spectroscopy, and elemental analysis. The results proved the grafting of -SO3H groups on an amorphous carbon structure. The catalyst activity was evaluated by the esterification of oleic acid with methanol. The sample prepared achieved 96.6% esterification yield, which was higher than the 86.7% yield achieved by commercial Ambersyst-15 under the same reaction conditions.
•A review and comparison of mathematical models for bioreactorsDevelopment and implementation of a mathematical model for a perfusion bioreactor•Global sensitivity analysis is performed, and the results are analyzed for two different scenarios•A relative gain analysis is performed and interpreted Perfusion bioreactors are important tools in tissue engineering that are used for cell cultivation. Unfortunately these types of processes are not yet fully understood in literature and information about the model is scarce. Furthermore, mathematical models that are used for perfusion bioreactors have posed significant challenges. This work presents a concise overview and analysis of mathematical models for a perfusion bioreactor process. The comprehensive mathematical model of convection and diffusion in a perfusion bioreactor, combined with cell growth kinetics, is developed using Computational Fluid Dynamics. The model describes the spatio-temporal evolution of glucose concentration, oxygen concentration, lactate concentration and cell density within a polymeric scaffold. For an in-depth understanding of this type of processes, global sensitivity analysis and simulations is performed using the method of high-dimensional model representation (RS-HDMR). A quantitative analysis of the complex kinetic mechanisms using recently developed advanced mathematical approaches to global sensitivity and uncertainty analysis through RS-HDMR can be exploited to investigate the important features of the perfusion bioreactor process as well as possible factors underlying qualitative discrepancies. Moreover, for a further understanding of the process, a relative gain analysis is performed. The results will help us gain an in depth understanding of the process and will be used as the foundation for advanced control algorithms that will facilitate manufacturing for any type of cell culture using a continuous perfusion bioreactor thus paving the way towards Industry 4.0.
Due to frequent changes in thermal load and drift of online oxygen analyzer, the heater’s thermal efficiency optimization system with limited maintenance resources seldom works in long term. To solve this problem, a novel and practical optimization method combing RIMER (i.e. belief rule-base inference methodology using the evidential reasoning) approach and SA (stochastic approximation) online self-optimization is proposed. The optimization scheme consists of (i) an off-line expert system that determines the optimal steady state operation for a given thermal load, and (ii) an on-line optimization system that further improves the thermal efficiency to alleviate the influence caused by sensors’ drift. In more details, at the off-line stage, a belief-rule-base (BRB) expert system is constructed to determine good initial references of operating conditions for a specified thermal load, which quickly drives the system to a near optimal operation point when confronted with the thermal load change; this is based on RIMER. During on-line operation, these off-line determined initial values are further refined by using the SA approach to optimize the thermal efficiency. The newly obtained optimal operating condition then is updated online to compensate the sensor’s drift. The optimized profile is implemented through a practical control strategy for the flue gas - air system of fired heaters, which is applied to the flue gas oxygen concentration and chamber negative pressure control on the basis of flue gas-air control system. Simulation results on the UniSimTM Design platform demonstrate the feasibility of the proposed optimization scheme. Furthermore, the field implementation results at a real process illustrate the effectiveness of this optimization system. Both simulation and field application show that the thermal efficiency can be nearly improved by c. 1%.
Many autonomous microgrids have extensive penetration of distributed generation (DG). Optimal power flow (OPF) is necessary for the optimal dispatch of networked microgrids (NMGs). Existing convex relaxation methods for three-phase OPF are limited to radial networks. In light of this, we develop a semidefinite programming (SDP) convex relaxation model which can cope with meshed networks and also includes a model of three-phase DG and under-load voltage regulators with different connection types. The SDP model solves the OPF problem of multi-phase meshed network effectively, with satisfactory accuracy, as validated by real 6-bus, 9-bus, and 30-bus NMGs, and the IEEE 123-bus test cases. In the SDP model, the convex symmetric component of the three-phase DG model is demonstrated to be more accurate than a three-phase DG modelled as three single-phase DG units in three-phase unbalanced OPF. The proposed method also has higher accuracy than the existing convex relaxation methods. The resultant optimal control variables obtained from the convex relaxation optimization can be used for both final optimal dispatch strategy and initial value of the non-convex OPF to obtain the globally optimal solution efficiently.
Fish feed accounts for more than 50% of total production cost in intensive aquaculture. Feeding fish with low-quality feed or adopting inappropriate feeding strategies causes not only food waste and consequent loss of income but also lead to water pollution. The aim of this study was to develop a mechanistic model based optimization method to determine aquaculture feeding programs. In particular, we integrate a fish weight prediction model and a requirement analysis model to establish an optimization method for designing balanced and sustainable feed formulations and effective feeding programs. The optimization strategy is necessary to maximise the fish weight at harvest, while constraints include specific feed requirements and fish growth characteristics. The optimization strategy is re-solved with new available fish weight measurement by using the error between measurement and model prediction to adjust the requirement analysis model and update feeding amount decision. The mechanistic models are parameterised using the existing nutritional data on gilthead seabream (Sparus aurata) to demonstrate the usefulness of proposed method. The simulation results show that the proposed approach can significantly improve aquaculture production. This particular simulation study reveals that when “Only prediction” method is considered as benchmark, the average improvement in fish weight of proposed method would be 13.25% when fish weight is measured once per four weeks (mimicking manual sampling practice), and 38.43% when daily measurement of fish weight is possible (e.g. through automatic image-based methods). Furthermore, if feed composition (460 g protein.kg feed−1; 18.9 MJ kg feed−1) is adjusted, the average improvement of proposed method could reach 46.85%. Compared with traditional feeding methods, the improvement of proposed method could reach 36.36% of the final fish weight at harvest. Further studies will consider improving the quality of feed plus executing more appropriate mathematical prediction models to optimize production performance.
OBJECTIVE: In the process of hair treatment, various cationic actives contained in hair care products can be absorbed into hair fiber to modulate the physicochemical properties of hair such as color, strength, style and volume. There have been very limited studies on the binding and partition properties of hair care actives to hair. This study aimed to investigate the pH effects on cationic solute absorption into hair and binding to keratin. METHODS: The keratin binding and hair partition properties of three cationic solutes (theophylline, nortriptyline and amitriptyline) have been measured at different pH using fluorescence spectroscopy and equilibrium absorption experiment. The binding constants, thermodynamic parameters and hair-water partition coefficients determined at different pH were compared and analyzed. RESULTS: Increasing the pH from 2.0 to 6.0 resulted in the net charge of hair keratin changed from positive to negative. As a consequence, the binding constants of the three cationic solutes with keratin increased with the increasing pH. This correlated with the variation of the electrostatic interaction between cationic solutes and keratin from repulsion to attraction. The positive H and S values indicated that hydrophobic interaction also played a major role in the binding of the three cationic solutes to keratin. There was a good correlation between solutes binding to keratin and hair-water partition of solutes. CONCLUSION: It appears that solute binding to hair keratin is driven first by hydrophobic interaction and then by electrostatic interaction. The fitted thermodynamic parameters suggested that hydrophobic interaction dominates for the binding of the three cationic solutes to keratin. That binding of cationic solutes to keratin correlates with the partition of the solutes to hair could provide theoretical guidance for further developing mathematical models of hair partition and penetration properties.
Response surface methodology (RSM) relies on the design of experiments and empirical modelling techniques to find the optimum of a process when the underlying fundamental mechanism of the process is largely unknown. This paper proposes an iterative RSM framework, where Gaussian process (GP) regression models are applied for the approximation of the response surface. GP regression is flexible and capable of modelling complex functions, as opposed to the restrictive form of the polynomial models that are used in traditional RSM. As a result, GP models generally attain high accuracy of approximating the response surface, and thus provide great chance of identifying the optimum. In addition, GP is capable of providing both prediction mean and variance, the latter being a measure of the modelling uncertainty. Therefore, this uncertainty can be accounted for within the optimization problem, and thus the process optimal conditions are robust against the modelling uncertainty. The developed method is successfully applied to the optimization of trans-stilbene conversion in the epoxidation of trans-stilbene over cobalt ion-exchanged faujasite zeolites (Co2+–NaX) catalysts using molecular oxyge
Advanced therapies are commonly administered via injection even when they act within the skin tissue and this increases the chances of off-target effects. Here we report the use of a skin patch containing a hypobaric chamber that induces skin dome formation to enable needleless delivery of advanced therapies directly into porcine, rat, and mouse skin. Finite element method (FEM) modeling showed that the hypobaric chamber in the patch opened the skin appendages by 32%, thinned the skin, and compressed the appendage wall epithelia. These changes allowed direct delivery of an H1N1 vaccine antigen and a diclofenac nanotherapeutic into the skin. Fluorescence imaging and infrared mapping of the skin showed needleless delivery via the appendages. The in vivo utility of the patch was demonstrated by a superior IgG response to the vaccine antigen in mice compared to intramuscular injection and a 70% reduction in rat paw swelling in vivo over 5 h with diclofenac without skin histology changes.
Background and Purpose Radiotherapy requires tight control of the delivered dose. This should include the variation in beam output as this may directly affect treatment outcomes. This work provides results from a multi-centre analysis of routine beam output measurements. Materials and Methods A request for 6MV beam output data was submitted to all radiotherapy centres in the UK, covering the period January 2015 – July 2015. An analysis of the received data was performed, grouping the data by manufacturer, machine age, and recording method to quantify any observed differences. Trends in beam output drift over time were assessed as well as inter-centre variability. Annual trends were calculated by linear extrapolation of the fitted data. Results Data was received from 204 treatment machines across 52 centres. Results were normally distributed with mean of 0.0% (percentage deviation from initial calibration) and a 0.8% standard deviation, with 98.1% of results within ±2%. There were eight centres relying solely on paper records. Annual trends varied greatly between machines with a mean drift of +0.9%/year with 95th percentiles of +5.1%/year and -2.2%/year. For the machines of known age 25% were over ten years old, however there was no significant differences observed with machine age. Conclusions Machine beam output measurements were largely within ±2% of 1.00cGy/MU. Clear trends in measured output over time were seen, with some machines having large drifts which would result in additional burden to maintain within acceptable tolerances. This work may act as a baseline for future comparison of beam output measurements.
Calibration and sensitivity studies in the computational fluid dynamics (CFD) simulation of process equipment such as the annular jet pump are useful for design, analysis and optimisation. The use of CFD for such purposes is computationally intensive. Hence, in this study, an alternative approach using kriging-based meta-models was utilised. Calibration via the adjustment of two turbulent model parameters, C_μ and C_2ε, and likewise two parameters in the simulation correlation for C_μ was considered; while sensitivity studies were based on C_μ as input. The meta-model based calibration aids exploration of different parameter combinations. Computational time was also reduced with kriging-assisted sensitivity studies which explored effect of different C_μ values on pressure distribution.
When analyzing heterogeneous samples using spectroscopy, the light scattering effect introduces non-linearity into the measurements and deteriorates the prediction accuracy of conventional linear models. This paper compares the prediction performance of two categories of chemometric methods: pre-processing techniques to remove the non-linearity and non-linear calibration techniques to directly model the non-linearity. A rigorous statistical procedure is adopted to ensure reliable comparison. The results suggest that optical path length estimation and correction (OPLEC) and Gaussian process (GP) regression are the most promising among the investigated methods. Furthermore, the combination of pre-processing and non-linear models is explored with limited success being achieved.
Additional publications
ORCiD record of publications: https://orcid.org/0000-0002-3941-1603