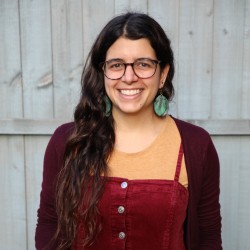
Dr Violeta Menéndez González
Publications
This paper proposes Sparse View Synthesis. This is a view synthesis problem where the number of reference views is limited, and the baseline between target and reference view is significant.Under these conditions, current radiance field methods fail catastrophically due to inescapable artifacts such 3d floating blobs, blurring and structural duplication, whenever the number of reference views is limited, or the target view diverges significantly from the reference views. Advances in network architecture and loss regularisation are unable to satisfactorily remove these artifacts. The occlusions within the scene ensure that the true contents of these regions is simply not available to the model.In this work, we instead focus on hallucinating plausible scene contents within such regions. To this end we unify radiance field models with adversarial learning and perceptual losses. The resulting system provides up to 60% improvement in perceptual accuracy compared to current state-of-the-art radiance field models on this problem.
In this work, we present an end-to-end network for stereo-consistent image inpainting with the objective of inpainting large missing regions behind objects. The proposed model consists of an edge-guided UNet-like network using Partial Convolutions. We enforce multi-view stereo consistency by introducing a disparity loss. More importantly, we develop a training scheme where the model is learned from realistic stereo masks representing object occlusions, instead of the more common random masks. The technique is trained in a supervised way. Our evaluation shows competitive results compared to previous state-of-the-art techniques.
Poster presented at AI4CC at CVPR 2022 and BMVA Symposium 2022, for the paper "SaiNet: Stereo aware inpainting behind objects with generative networks" (https://doi.org/10.48550/arXiv.2205.07014). In this work, we present an end-to-end network for stereo-consistent image inpainting with the objective of inpainting large missing regions behind objects. The proposed model consists of an edge-guided UNet-like network using Partial Convolutions. We enforce multi-view stereo consistency by introducing a disparity loss. More importantly, we develop a training scheme where the model is learned from realistic stereo masks representing object occlusions, instead of the more common random masks. The technique is trained in a supervised way. Our evaluation shows competitive results compared to previous state-of-the-art techniques.
Poster for the paper "SVS: Adversarial refinement for sparse novel view synthesis" published at BMVC 2022 (https://bmvc2022.mpi-inf.mpg.de/886/) This paper proposes Sparse View Synthesis. This is a view synthesis problem where the number of reference views is limited, and the baseline between target and reference view is significant. Under these conditions, current radiance field methods fail catastrophically due to inescapable artifacts such 3D floating blobs, blurring and structural duplication, whenever the number of reference views is limited, or the target view diverges significantly from the reference views. Advances in network architecture and loss regularisation are unable to satisfactorily remove these artifacts. The occlusions within the scene ensure that the true contents of these regions is simply not available to the model. In this work, we instead focus on hallucinating plausible scene contents within such regions. To this end we unify radiance field models with adversarial learning and perceptual losses. The resulting system provides up to 60% improvement in perceptual accuracy compared to current state-of-the-art radiance field models on this problem. Code: https://github.com/violetamenendez/svs-sparse-novel-view
In this work, we present an end-to-end network for stereo-consistent image inpainting with the objective of inpainting large missing regions behind objects. The proposed model consists of an edge-guided UNet-like network using Partial Convolutions. We enforce multi-view stereo consistency by introducing a disparity loss. More importantly, we develop a training scheme where the model is learned from realistic stereo masks representing object occlusions, instead of the more common random masks. The technique is trained in a supervised way. Our evaluation shows competitive results compared to previous state-of-the-art techniques.
In the field of media production, video editing techniques play a pivotal role. Recent approaches have had great success at performing novel view image synthesis of static scenes. But adding temporal information adds an extra layer of complexity. Previous models have focused on implicitly representing static and dynamic scenes using NeRF. These models achieve impressive results but are costly at training and inference time. They overfit an MLP to describe the scene implicitly as a function of position. This paper proposes ZeST-NeRF, a new approach that can produce temporal NeRFs for new scenes without retraining. We can accurately reconstruct novel views using multi-view synthesis techniques and scene flow-field estimation, trained only with unrelated scenes. We demonstrate how existing state-of-the-art approaches from a range of fields cannot adequately solve this new task and demonstrate the efficacy of our solution. The resulting network improves quantitatively by 15% and produces significantly better visual results.