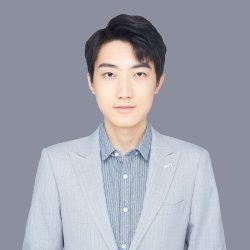
Dr Xiao Han
About
My research project
Reciprocal and explainable fusion of low-level and high-level visionThe long-term goal of my research is to explore how to effectively combine low-level and high-level vision in an explainable way, and how to improve their performance reciprocally through collaborative training.
The long-term goal of my research is to explore how to effectively combine low-level and high-level vision in an explainable way, and how to improve their performance reciprocally through collaborative training.
Publications
Large-scale Vision-and-Language (V+L) pre-training for representation learning has proven to be effective in boosting various downstream V+L tasks. However, when it comes to the fashion domain, existing V+L methods are inadequate as they overlook the unique characteristics of both fashion V+L data and downstream tasks. In this work, we propose a novel fashion-focused V+L representation learning framework, dubbed as FashionViL. It contains two novel fashion-specific pre-training tasks designed particularly to exploit two intrinsic attributes with fashion V+L data. First, in contrast to other domains where a V+L datum contains only a single image-text pair, there could be multiple images in the fashion domain. We thus propose a Multi-View Contrastive Learning task for pulling closer the visual representation of one image to the compositional multimodal representation of another image+text. Second, fashion text (e.g., product description) often contains rich fine-grained concepts (attributes/noun phrases). To capitalize this, a Pseudo-Attributes Classification task is introduced to encourage the learned unimodal (visual/textual) representations of the same concept to be adjacent. Further, fashion V+L tasks uniquely include ones that do not conform to the common one-stream or two-stream architectures (e.g., text-guided image retrieval). We thus propose a flexible, versatile V+L model architecture consisting of a modality-agnostic Transformer so that it can be flexibly adapted to any downstream tasks. Extensive experiments show that our FashionViL achieves new state of the art across five downstream tasks. Code is available at https://github.com/BrandonHanx/mmf.
In the fashion domain, there exists a variety of vision-and-language (V+L) tasks, including cross-modal retrieval, text-guided image retrieval, multi-modal classification, and image captioning. They differ drastically in each individual input/output format and dataset size. It has been common to design a task-specific model and fine-tune it independently from a pre-trained V+L model (e.g., CLIP). This results in parameter inefficiency and inability to exploit inter-task relatedness. To address such issues, we propose a novel FAshion-focused Multi-task Efficient learning method for Vision-and-Language tasks (FAME-ViL) in this work. Compared with existing approaches, FAME-ViL applies a single model for multiple heterogeneous fashion tasks, therefore being much more parameter-efficient. It is enabled by two novel components: (1) a task-versatile architecture with cross-attention adapters and task-specific adapters integrated into a unified V+L model, and (2) a stable and effective multi-task training strategy that supports learning from heterogeneous data and prevents negative transfer. Extensive experiments on four fashion tasks show that our FAME-ViL can save 61.5% of parameters over alternatives, while significantly outperforming the conventional independently trained single-task models. Code is available at https://github.com/BrandonHanx/FAME-ViL.
Interactive garment retrieval (IGR) aims to retrieve a target garment image based on a reference garment image along with user feedback on what to change on the reference garment. Two IGR tasks have been studied extensively: text-guided garment retrieval (TGR) and visually compatible garment retrieval (VCR). The user feedback for the former indicates what semantic attributes to change with the garment category preserved, while the category is the only thing to be changed explicitly for the latter, with an implicit requirement on style preservation. Despite the similarity between these two tasks and the practical need for an efficient system tackling both, they have never been unified and modeled jointly. In this paper, we propose a Unified Interactive Garment Retrieval (UIGR) framework to unify TGR and VCR. To this end, we first contribute a large-scale benchmark suited for both problems. We further propose a strong baseline architecture to integrate TGR and VCR in one model. Extensive experiments suggest that unifying two tasks in one framework is not only more efficient by requiring a single model only, it also leads to better performance. Code and datasets are available at GitHub.
Abstract In the fashion domain, there exists a variety of vision- and-language (V+L) tasks, including cross-modal retrieval, text-guided image retrieval, multi-modal classification, and image captioning. They differ drastically in each individ- ual input/output format and dataset size. It has been com- mon to design a task-specific model and fine-tune it in- dependently from a pre-trained V+L model (e.g., CLIP). This results in parameter inefficiency and inability to ex- ploit inter-task relatedness. To address such issues, we pro- pose a novel FAshion-focused Multi-task Efficient learn- ing method for Vision-and-Language tasks (FAME-ViL) in this work. Compared with existing approaches, FAME-ViL applies a single model for multiple heterogeneous fashion tasks, therefore being much more parameter-efficient. It is enabled by two novel components: (1) a task-versatile architecture with cross-attention adapters and task-specific adapters integrated into a unified V+L model, and (2) a sta- ble and effective multi-task training strategy that supports learning from heterogeneous data and prevents negative transfer. Extensive experiments on four fashion tasks show that our FAME-ViL can save 61.5% of parameters over alternatives, while significantly outperforming the conven- tional independently trained single-task models. Code is available at https://github.com/BrandonHanx/FAME-ViL
Recently, text-guided 3D generative methods have made remarkable advancements in producing high-quality textures and geometry, capitalizing on the proliferation of large vision-language and image diffusion models. However, existing methods still struggle to create high-fidelity 3D head avatars in two aspects: (1) They rely mostly on a pre-trained text-to-image diffusion model whilst missing the necessary 3D awareness and head priors. This makes them prone to inconsistency and geometric distortions in the generated avatars. (2) They fall short in fine-grained editing. This is primarily due to the inherited limitations from the pre-trained 2D image diffusion models, which become more pronounced when it comes to 3D head avatars. In this work, we address these challenges by introducing a versatile coarse-to-fine pipeline dubbed HeadSculpt for crafting (i.e., generating and editing) 3D head avatars from textual prompts. Specifically, we first equip the diffusion model with 3D awareness by leveraging landmark-based control and a learned textual embedding representing the back view appearance of heads, enabling 3D-consistent head avatar generations. We further propose a novel identity-aware editing score distillation strategy to optimize a textured mesh with a high-resolution differentiable rendering technique. This enables identity preservation while following the editing instruction. We showcase HeadSculpt's superior fidelity and editing capabilities through comprehensive experiments and comparisons with existing methods.