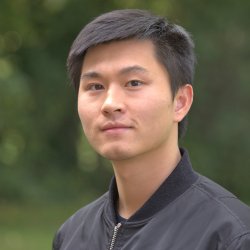
Dr Xiongjie Chen
Academic and research departments
Computer Science Research Centre, School of Computer Science and Electronic Engineering.About
Biography
I am currently a postdoctoral researcher in the Computer Science Research Centre, School of Computer Science and Electronic Engineering at the University of Surrey.
I completed my PhD in Computer Science from the University of Surrey in 2023, supervised by Dr Yunpeng Li. My research is in the area of statistical machine learning (Bayesian inference and uncertainty quantification), sequential Monte Carlo methods, machine learning in dentistry, and optimal transport. My ongoing projects particularly focus on the application of AI techniques in healthcare such as dental disease detection with radiography, as well as data-adaptive uncertainty quantification methods in complex high-dimensional environments
Prior to joining the University of Surrey, I received my B.Sc. and M.Eng. degrees at Fujian University of Technology and Tongji University, respectively.
Areas of specialism
ResearchResearch interests
My research focuses on the development of uncertainty quantification methods to improve the robustness and trustworthiness of machine learning models. I am currently working on calibrating machine learning models' predictions on dental radiographs, as well as the quantification and propagation of uncertainties in complex high-dimensional dynamical systems. My past and ongoing research efforts have found applications in the field of AI-assisted healthcare systems, anomaly detection, and object tracking/localisation.
Research projects
AI-assisted Automatic Dental Disease Detection with RadiographyDeveloping an AI-assisted system capable of identifying dental diseases such as caries, calculus, and periapical radiolucency, aimed at aiding dentists in diagnosing through dental X-rays.
Differentiable Particle FilteringBuilding particle filtering approaches with modern neural networks to quantify and propagate uncertainties in complex high-dimensional partially observed dynamical systems.
Uncertainty quantification in deep neural networksCalibrating neural networks' prediction to mitigate the overconfidence/underconfidence problem in neural network-based machine learning models.
Efficient optimal transport methods for machine learningReducing the computational cost of optimal transport methods for their integration into large-scale machine learning applications.
Research interests
My research focuses on the development of uncertainty quantification methods to improve the robustness and trustworthiness of machine learning models. I am currently working on calibrating machine learning models' predictions on dental radiographs, as well as the quantification and propagation of uncertainties in complex high-dimensional dynamical systems. My past and ongoing research efforts have found applications in the field of AI-assisted healthcare systems, anomaly detection, and object tracking/localisation.
Research projects
Developing an AI-assisted system capable of identifying dental diseases such as caries, calculus, and periapical radiolucency, aimed at aiding dentists in diagnosing through dental X-rays.
Building particle filtering approaches with modern neural networks to quantify and propagate uncertainties in complex high-dimensional partially observed dynamical systems.
Calibrating neural networks' prediction to mitigate the overconfidence/underconfidence problem in neural network-based machine learning models.
Reducing the computational cost of optimal transport methods for their integration into large-scale machine learning applications.
Teaching
- Demonstrator: COM3013 Computational Intelligence, October 2021- December 2021
- Demonstrator: COMM054 Data Science, October 2021- December 2021
Publications
Tuning of measurement models is challenging in real-world applications of sequential Monte Carlo methods. Recent advances in differentiable particle filters have led to various efforts to learn measurement models through neural networks. But existing approaches in the differentiable particle filter framework do not admit valid probability densities in constructing measurement models, leading to incorrect quantification of the measurement uncertainty given state information. We propose to learn expressive and valid probability densities in measurement models through conditional normalizing flows, to capture the complex likelihood of measurements given states. We show that the proposed approach leads to improved estimation performance and faster training convergence in a visual tracking experiment.
Differentiable particle filters are an emerging class of particle filtering methods that use neural networks to construct and learn parametric state-space models. In real-world applications, both the state dynamics and measurements can switch between a set of candidate models. For instance, in target tracking, vehicles can idle, move through traffic, or cruise on motorways, and measurements are collected in different geographical or weather conditions. This paper proposes a new differentiable particle filter for regime-switching state-space models. The method can learn a set of unknown candidate dynamic and measurement models and track the state posteriors. We evaluate the performance of the novel algorithm in relevant models, showing its great performance compared to other competitive algorithms.