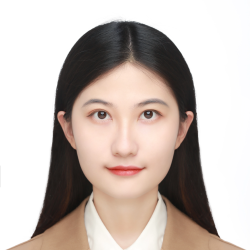
Yaru Chen
Academic and research departments
About
My research project
Multi-scale tracking of fish groups with uncertainty modelling and intent predictionTracking the trajectory of fish movement can facilitate the analysis and recognition of fish behaviour. This project aims to study novel methods for fish tracking and intent prediction for fish behaviour analysis, using the image and sounds collected by optical, acoustic, or other types of sensors. Multi-scale algorithms will be developed for the tracking of individual fish or fish groups, for the prediction of their intents. Uncertainty modelling and quantification will be incorporated to show the prediction confidence, considering the complexity of the fish living environment and the uncertainty of fish behaviour. Such algorithms will be evaluated in the context of feeding behaviour analysis using audio-visual data.
Supervisors
Tracking the trajectory of fish movement can facilitate the analysis and recognition of fish behaviour. This project aims to study novel methods for fish tracking and intent prediction for fish behaviour analysis, using the image and sounds collected by optical, acoustic, or other types of sensors. Multi-scale algorithms will be developed for the tracking of individual fish or fish groups, for the prediction of their intents. Uncertainty modelling and quantification will be incorporated to show the prediction confidence, considering the complexity of the fish living environment and the uncertainty of fish behaviour. Such algorithms will be evaluated in the context of feeding behaviour analysis using audio-visual data.
Publications
Audio-visual video parsing is the task of categorizing a video at the segment level with weak labels, and predicting them as audible or visible events. Recent methods for this task leverage the attention mechanism to capture the semantic correlations among the whole video across the audio-visual modalities. However, these approaches have overlooked the importance of individual segments within a video and the relationship among them, and tend to rely on a single modality when learning features. In this paper, we propose a novel interactive-enhanced cross-modal perception method~(CM-PIE), which can learn fine-grained features by applying a segment-based attention module. Furthermore, a cross-modal aggregation block is introduced to jointly optimize the semantic representation of audio and visual signals by enhancing inter-modal interactions. The experimental results show that our model offers improved parsing performance on the Look, Listen, and Parse dataset compared to other methods.
Sound Event Localization and Detection (SELD) is a task that involves detecting different types of sound events along with their temporal and spatial information, specifically, detecting the classes of events and estimating their corresponding direction of arrivals at each frame. In practice, real-world sound scenes might be complex as they may contain multiple overlapping events. For instance, in DCASE challenges task 3, each clip may involve simultaneous occurrences of up to five events. To handle multiple overlapping sound events, current methods prefer multiple output branches to estimate each event, which increases the size of the models. Therefore, current methods are often difficult to be deployed on the edge of sensor networks. In this paper, we propose a method called Probabilistic Localization and Detection of Independent Sound Events with Transformers (PLDISET), which estimates numerous events by using one output branch. The method has three stages. First, we introduce the track generation module to obtain various tracks from extracted features. Then, these tracks are fed into two transformers for sound event detection (SED) and localization, respectively. Finally, one output system, including a linear Gaussian system and regression network, is used to estimate each track. We give the evaluation resn results of our model on DCASE 2023 Task 3 development dataset.