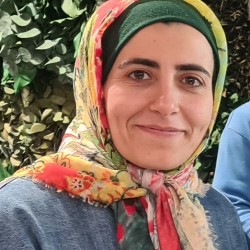
Zahra Chaghazardi
About
My research project
An Explainable and Trustworthy Traffic Sign Detection Method for Safe Autonomous DrivingI am currently engaged in a fascinating research project that focuses on implementing Computer Vision in the field of Autonomous Vehicles, utilizing the benefits of Logical Programming.
Supervisors
I am currently engaged in a fascinating research project that focuses on implementing Computer Vision in the field of Autonomous Vehicles, utilizing the benefits of Logical Programming.
Publications
Deep learning serves as a crucial component in computer vision, enabling accurate predictions from raw data. However, unlike human cognition, deep learning models are vulnerable to adversarial attacks. This paper introduces a new method for traffic sign recognition that employs Inductive Logic Programming (ILP) to generate logical rules from a limited set of examples. These rules are used to assess the logical consistency of predictions, which is then incorporated into the neural network through the loss function. The study investigates the effect of incorporating logical rules into deep learning models on the ro-bustness of vision tasks in autonomous vehicles (AV). The experimental results show that the proposed method significantly improves the accuracy of traffic sign recognition in the presence of adversarial attacks.
Even though heavy-commercial vehicles have a relatively low population density (11%), they still account for a large share of urban noise and CO2 emission (37%). Therefore, trucks are a priority for full electrification. However, heavy-duty trucks (HDT) have complex array of challenges: high energy consumption combined with high daily driving distances. This paper investigates the effect of operating temperature on HDT energy performance. A high-fidelity multi-physics model of electric machine with fixed and variable parameters as nonlinear functions of temperature and coolant flow characteristics was developed. Temperature-dependent motor maps were then generated and integrated with forward-facing vehicle model. Quantitative analyses under different operating conditions with a realistic cycle (ESK) have shown that temperature plays a crucial role for the energy efficiency of EVs.
Traffic sign detection is a critical task in the operation of Autonomous Vehicles (AV), as it ensures the safety of all road users. Current DNN-based sign classification systems rely on pixel-level features to detect traffic signs and can be susceptible to adversarial attacks. These attacks involve small, imperceptible changes to a sign that can cause traditional classifiers to misidentify the sign. We propose an Inductive Logic Programming (ILP) based approach for stop sign detection in AVs to address this issue. This method utilises high-level features of a sign, such as its shape, colour, and text, to detect categories of traffic signs. This approach is more robust against adversarial attacks, as it mimics human-like perception and is less susceptible to the limitations of current DNN classifiers. We consider two adversarial attacking methods to evaluate our approach: Robust Physical Perturbation (PR2) and Adversarial Camouflage (AdvCam). These attacks are able to deceive DNN classifiers, causing them to misidentify stop signs as other signs with high confidence. The results show that the proposed ILP-based technique is able to correctly identify all targeted stop signs, even in the presence of PR2 and ADvCam attacks. The proposed learning method is also efficient as it requires minimal training data. Moreover, it is fully explainable, making it possible to debug AVs.