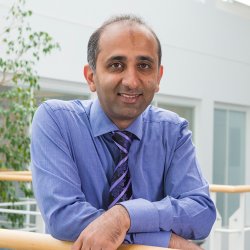
Professor Saber Fallah
Academic and research departments
School of Mechanical Engineering Sciences, Centre for Automotive Engineering, Connected and Autonomous Vehicles Lab.About
Biography
Saber Fallah is Professor of Safe AI and Autonomy and the Director of the Connected Autonomous Vehicle Research Lab (CAV-Lab) at the University of Surrey, where he leads a team of academic scholars, researchers, and CAV experts whose research has contributed to the state-of-the-art research in the areas of CAVs in the UK and globally through its collaborative projects delivered in partnership with international companies. The focus of Prof Fallah’s research is on safe and trustworthy AI-enabled autonomous robotic system. He is the recipient of 2019 Guildford Innovation Award in Emerging Technologies category.
Areas of specialism
University roles and responsibilities
- Serving as the member of departmental Athena Swan group
- Visiting tutor for professional training students
News
In the media
ResearchResearch interests
- Safe AI and Autonomy
- Deep Reinforcement Learning
- Neuro-Symbolic Learning
- Model-based Optimal Control
Research funding
- CAV Localisation using Satellite Imagery, Funding Source: SPRINT, 2022.
- Energy Management of a Fleet of Connecte Electric Vehicles, Funding Source: KTP, 2018-2021,
- GPR for Localisation of Autonomous Vehicles, Funding Source: Innovate UK, 2018-2019,
- Above the Cloud, Funding Source: Innovate UK, 2017-2019,
- Future AI and Robotics for Space (FAIR-SPACE), Funding Source: EPSRC - UK Space Agency, 2017-2021, https://fair-space.squarespace.com ,
- Improved Trustworthiness and Weather-Independence of Conditionally Automated Vehicles in Mixed Traffic Scenarios (TrustVehicle), Funding Source: European Commission (Horizon 2020), 2017-2020, http://www.trustvehicle.eu ,
- Optimization of scalable realtime models and functional testing for e-drive concepts (Obelics), Funding Source: European Commission (Horizon 2020), 2017-2020, https://obelics.eu ,
- Secure Cloud-based Distributed Control (SCDC) Systems for Connected Autonomous Cars, Funding Source: EPSRC - JLR, 2016-2021, http://gow.epsrc.ac.uk/NGBOViewGrant.aspx?GrantRef=EP/N01300X/2 ,
- Development of Pre-emptive Optimal Control for Active Suspension Systems, Funding Source: Jaguar Land Rover (JLR), Industrial Research Collaboration, 2013-2014,
- Integrated Control of Multiple-Motor and Multiple-Storage Fully Electric Vehicles, Funding Source: Seventh Framework Programme, European Commission, 2013-2016, http://www.i-compose.eu/iCompose/ ,
- Development of Regenerative Auxiliary Power Systems, Automotive Partnership Canada (APC) and Canada Foundation for Innovation (CFI), Dec. 2011.
Research interests
- Safe AI and Autonomy
- Deep Reinforcement Learning
- Neuro-Symbolic Learning
- Model-based Optimal Control
Research funding
- CAV Localisation using Satellite Imagery, Funding Source: SPRINT, 2022.
- Energy Management of a Fleet of Connecte Electric Vehicles, Funding Source: KTP, 2018-2021,
- GPR for Localisation of Autonomous Vehicles, Funding Source: Innovate UK, 2018-2019,
- Above the Cloud, Funding Source: Innovate UK, 2017-2019,
- Future AI and Robotics for Space (FAIR-SPACE), Funding Source: EPSRC - UK Space Agency, 2017-2021, https://fair-space.squarespace.com ,
- Improved Trustworthiness and Weather-Independence of Conditionally Automated Vehicles in Mixed Traffic Scenarios (TrustVehicle), Funding Source: European Commission (Horizon 2020), 2017-2020, http://www.trustvehicle.eu ,
- Optimization of scalable realtime models and functional testing for e-drive concepts (Obelics), Funding Source: European Commission (Horizon 2020), 2017-2020, https://obelics.eu ,
- Secure Cloud-based Distributed Control (SCDC) Systems for Connected Autonomous Cars, Funding Source: EPSRC - JLR, 2016-2021, http://gow.epsrc.ac.uk/NGBOViewGrant.aspx?GrantRef=EP/N01300X/2 ,
- Development of Pre-emptive Optimal Control for Active Suspension Systems, Funding Source: Jaguar Land Rover (JLR), Industrial Research Collaboration, 2013-2014,
- Integrated Control of Multiple-Motor and Multiple-Storage Fully Electric Vehicles, Funding Source: Seventh Framework Programme, European Commission, 2013-2016, http://www.i-compose.eu/iCompose/ ,
- Development of Regenerative Auxiliary Power Systems, Automotive Partnership Canada (APC) and Canada Foundation for Innovation (CFI), Dec. 2011.
Supervision
Postgraduate research supervision
Research fellows
- Dr Ainur Begalinova, 3D digital map construction for autonomous vehicle navigation, 2019 - present
- Dr Ashith Rajendar Babu, Learning-based MPC motion-planning for robotic manipulators, 2019 - present
- Dr Kaushik Halder, Robust distributed control systems for connected autonomous vehicles, 2018 - present
- Mohammad Zeeshan Babar, Multi-objective Optimsation for Planetary Rovers, 2018 - present
- Umberto Montonaro, Design of a cloud-assisted distributed control system for connected autonomous driving, 2016 - 2018.
PhD students
- Nikolaos Sarantinoudis, Sensor Fusion and digital map construction for autonomous vehicle navigation”, April 2019 - present
- Thomas Barbier, Perception of morphology & kinematic properties of space debris for grasp planning using sensor fusion, January 2019 - present
- Marco Visca, Reinforcement Learning Path Planning of Autonomous Planetary Rovers. October 2018 - present
- Salar Arbabi, Cooperative Decision Making for Automated Driving in Urban Environment, October 2018 - present
- Glen Mason, Functional Safety Analysis of Cooperative Adaptive Cruise Control Systems, October 2017 - present
- Sampo Kuutti, End-to-End Deep Learning Control for Connected Autonomous Vehicles, October 2017 - present
- Samuel Green, Fault-tolerant Cooperative Vehicle State Estimation (Cloud-assisted), October, 2017 - present
- Shayan Taherian, Vehicle Emergency Autonomous Collision Avoidance System , May 2017 - present
- Shilp Dixit, Fault-tolerant Cooperative Cruise Control with Autonomous Overtaking, October 2016 - present
- Zhengyuan Wang, Robust Optimal Vehicle Yaw Moment Stability Control, January 2014-2018
- Johan Theunissen, Model Predictive Control of Semi-active Suspension Systems, January 2014 - present.
Undergraduate students (MEng)
- Sreejith Sreekumar, Design and Numerical Validation of Advanced Distributed Control of Connected Autonomous Vehicles in Platoons, 2017-2018
- Mark Peters, Intelligent Vehicle Health Monitoring and Maintenance using Machine Learning, 2017-2018
- Salar Arbabi, Robotic Test Station for Automated Testing of Disk Pump Actuators, 2017-2018
- Sampo Kuutti, Analysis of Cooperative Vehicle Localisation Systems, 2016-2017
- Saifuddin Abdul Halim, Energy Management of a Series Hybrid Powertrain using Forward Equivalent Consumption Minimisation Strategy, 2016
- Darius Mazeika, Emission Estimation of a Conventional Diesel Bus, 2015 (In collaboration with Alexander Denis Ltd.)
- Tom Morrison, Emission Estimation of a Series Hybrid Electric Bus, 2015 (In collaboration with Alexander Denis Ltd.)
- James MacDonald, Application of Equivalent Consumption Minimisation Strategy for Series Hybrid Electric Vehicles, 2014
- Ioannis Karountzos, Power Management Control Design for a Hybrid Electric Vehicle, 2014
- Lambros Vardakis, Modeling of a Continuously Variable Transmission and Its Fuel Efficiency Analysis, 2014
- Matthew Chapman, Semi-active Suspension Simulation and Optimization using MATLAB and Simulink, 2013.
Teaching
- ENGM248: Mechatronics
- ENGM001: Multi-Disciplinary Design Project
- ENG3170: Modern Vehicle System Design
- ENG3166: Control and Dynamics
- ENG2094: Control.
Publications
Driving energy consumption plays a major role in the navigation of mobile robots in challenging environments, especially if they are left to operate unattended under limited on-board power. This paper reports on first results of an energy-aware path planner, which can provide estimates of the driving energy consumption and energy recovery of a robot traversing complex uneven terrains. Energy is estimated over trajectories making use of a self-supervised learning approach, in which the robot autonomously learns how to correlate perceived terrain point clouds to energy consumption and recovery. A novel feature of the method is the use of 1D convolutional neural network to analyse the terrain sequentially in the same temporal order as it would be experienced by the robot when moving. The performance of the proposed approach is assessed in simulation over several digital terrain models collected from real natural scenarios, and is compared with a heuristic inclination-based energy model. We show evidence of the benefit of our method to increase the overall prediction r2 score by 66.8 % and to reduce the driving energy consumption over planned paths by 5.5 %.
Deep neural networks have demonstrated their capability to learn control policies for a variety of tasks. However, these neural network-based policies have been shown to be susceptible to exploitation by adversarial agents. Therefore, there is a need to develop techniques to learn control policies that are robust against adversaries. We introduce Adversarially Robust Control (ARC), which trains the protagonist policy and the adversarial policy end-to-end on the same loss. The aim of the protagonist is to maximise this loss, whilst the adversary is attempting to minimise it. We demonstrate the proposed ARC training in a highway driving scenario, where the protagonist controls the follower vehicle whilst the adversary controls the lead vehicle. By training the protagonist against an ensemble of adversaries, it learns a significantly more robust control policy, which generalises to a variety of adversarial strategies. The approach is shown to reduce the amount of collisions against new adversaries by up to 90.25%, compared to the original policy. Moreover, by utilising an auxiliary distillation loss, we show that the fine-tuned control policy shows no drop in performance across its original training distribution.
Driving energy consumption plays a major role in the navigation of autonomous mobile robots in off-road scenarios. However, real-time constraints often limit the accuracy of the energy estimations, especially in scenarios where accurate wheel-terrain interactions are complex to model. In this paper, an adaptive deep meta-learning energy-aware path planner is proposed that can provide energy estimates of a mobile robot traversing complex uneven terrains with varying and unknown terrain properties. A novel feature of the method is the integration into the meta-learning framework of a 1D convolutional neural network to analyze the terrain sequentially, in the same temporal order as it would be experienced by the robot when moving, and efficiently adapt its energy estimates to the local terrain conditions based on a small number of local measurements. The performance of the method is assessed in a realistic 3D-body dynamic simulator over several typologies of deformable terrains and unstructured geometries. We provide evidence of the benefit of the proposed approach to retain 83% r2 score of the original simulator at 0.55% of the computing time. Finally, the method is compared with alternative state-of-the-art deep learning solutions. In this way, we show indications of its improved robustness to provide more informed driving energy estimations and energy-efficient paths when navigating over challenging uneven terrains.
Autonomous driving decision-making is a challenging task due to the inherent complexity and uncertainty in traffic. For example, adjacent vehicles may change their lane or overtake at any time to pass a slow vehicle or to help traffic flow. Anticipating the intention of surrounding vehicles, estimating their future states and integrating them into the decision-making process of an automated vehicle can enhance the reliability of autonomous driving in complex driving scenarios. This paper proposes a Prediction-based Deep Reinforcement Learning (PDRL) decision-making model that considers the manoeuvre intentions of surrounding vehicles in the decision-making process for highway driving. The model is trained using real traffic data and tested in various traffic conditions through a simulation platform. The results show that the proposed PDRL model improves the decision-making performance compared to a Deep Reinforcement Learning (DRL) model by decreasing collision numbers, resulting in safer driving.
Vehicle platooning is a promising cooperative driving vision where a group of consecutive connected autonomous vehicles (CAVs) travel at the same speed with the aim of improving fuel efficiency, road safety, and road usage. To achieve the benefits promised through platoon- ing, platoon control algorithms must coordinate the dynamics of CAVs such that the closed-loop system is stable, errors between consecutive vehicles do not amplify along the string, and the time for re-establish the platoon formation to changes in the operating conditions does not diverge when the number of CAVs increases. Linear longitudinal vehicle dynamics are often assumed in the literature to guarantee such stringent platoon control requirements and they can be attained by equipping vehicles in the fleet with mid-level control systems. However, model uncertainties and disturbances can jeopardise the tracking of the reference linear behaviour. Hence, this paper presents for the first time, at the best of the authors' knowledge, the design and the performance of an adaptive control strategy and a robust model predictive control method as possible solutions for the mid-level control problem. Numerical results confirm that both control techniques are effective at imposing the dynamics of a linear time-invariant system to the longitudinal vehicle motion and they outperform model-based feedback linearisation methods when the parameters of the nonlinear longitudinal vehicle model are affected by uncertainties.
Deep learning has become an increasingly common technique for various control problems, such as robotic arm manipulation, robot navigation, and autonomous vehicles. However, the downside of using deep neural networks to learn control policies is their opaque nature and the difficulties of validating their safety. As the networks used to obtain state-of-the-art results become increasingly deep and complex, the rules they have learned and how they operate become more challenging to understand. This presents an issue, since in safety-critical applications the safety of the control policy must be ensured to a high confidence level. In this paper, we propose an automated black box testing framework based on adversarial reinforcement learning. The technique uses an adversarial agent, whose goal is to degrade the performance of the target model under test. We test the approach on an autonomous vehicle problem, by training an adversarial reinforcement learning agent, which aims to cause a deep neural network-driven autonomous vehicle to collide. Two neural networks trained for autonomous driving are compared, and the results from the testing are used to compare the robustness of their learned control policies. We show that the proposed framework is able to find weaknesses in both control policies that were not evident during online testing and therefore, demonstrate a significant benefit over manual testing methods.
Driving energy consumption plays a major role in the navigation of mobile robots in challenging environments, especially if they are left to operate unattended under limited on-board power. This paper reports on first results of an energy-aware path planner, which can provide estimates of the driving energy consumption and energy recovery of a robot traversing complex uneven terrains. Energy is estimated over trajectories making use of a self-supervised learning approach, in which the robot autonomously learns how to correlate perceived terrain point clouds to energy consumption and recovery. A novel feature of the method is the use of 1D convolutional neural network to analyse the terrain sequentially in the same temporal order as it would be experienced by the robot when moving. The performance of the proposed approach is assessed in simulation over several digital terrain models collected from real natural scenarios, and is compared with a heuristic inclination-based energy model. We show evidence of the benefit of our method to increase the overall prediction r2 score by 66.8 % and to reduce the driving energy consumption over planned paths by 5.5 %.
Many real-world applications require agents to cooperate and collaborate to accomplish shared missions; though, there are many instances where the agents should work together without communication or prior coordination. In the meantime, agents often coordinate in a decentralised manner to complete tasks that are displaced in an environment (e.g., foraging, demining, rescue or fire-fighting). Each agent in the team is responsible for selecting their own task and completing it autonomously. However, there is a possibility of an adversary in the team, who tries to prevent other agents from achieving their goals. In this study, we assume there is an agent who estimates the model of other agents in the team to boost the team's performance regardless of the enemy's attacks. Hence, we present On-line Estimators for Ad-hoc Task Allocation with Adversary (OEATA-A), a novel algorithm to have better estimations of the teammates' future behaviour, which includes identifying enemies among friends.
Terrain traversability analysis plays a major role in ensuring safe robotic navigation in unstructured environments. However, real-time constraints frequently limit the accuracy of online tests especially in scenarios where realistic robot-terrain interactions are complex to model. In this context, we propose a deep learning framework trained in an end-to-end fashion from elevation maps and trajectories to estimate the occurrence of failure events. The network is first trained and tested in simulation over synthetic maps generated by the OpenSimplex algorithm. The prediction performance of the Deep Learning framework is illustrated by being able to retain over 94% recall of the original simulator at 30% of the computational time. Finally, the network is transferred and tested on real elevation maps collected by the SEEKER consortium during the Martian rover test trial in the Atacama desert in Chile. We show that transferring and fine-tuning of an application-independent pre-trained model retains better performance than training uniquely on scarcely available real data.
In this article, an enhanced model reference adaptive control (EMRAC) algorithm is used to design a generic lateral-tracking controller for a vehicle. This EMRAC is different from the EMRAC in the literature as it adopts a σ-modification approach to bind the adaptive gain of the switching action. Moreover, an extended Lyapunov theory for discontinuous systems is used to analytically prove the ultimate boundedness of the closed-loop control system when the adaptive gain of the switching action is bounded with a σ-modification strategy. The control algorithm is applied to a vehicle path-tracking problem and its tracking performance is investigated under conditions of: 1) external disturbances such as crosswind; 2) road surface changes; 3) modeling errors; and 4) parameter missmatches in a co-simulation environment based on IPG Carmaker/MATLAB. The simulation studies show that the controller is effective at tracking a given reference path for performing different autonomous highway driving maneuvers while ensuring the boundedness of all closed-loop signals even when the system is subjected to the conditions mentioned above.
Autonomous agents that drive on roads shared with human drivers must reason about the nuanced interactions among traffic participants. This poses a highly challenging decision making problem since human behavior is influenced by a multitude of factors (e.g., human intentions and emotions) that are hard to model. This paper presents a decision making approach for autonomous driving, focusing on the complex task of merging into moving traffic where uncertainty emanates from the behavior of other drivers and imperfect sensor measurements. We frame the problem as a partially observable Markov decision process (POMDP) and solve it online with Monte Carlo tree search. The solution to the POMDP is a policy that performs high-level driving maneuvers, such as giving way to an approaching car, keeping a safe distance from the vehicle in front or merging into traffic. Our method leverages a model learned from data to predict the future states of traffic while explicitly accounting for interactions among the surrounding agents. From these predictions, the autonomous vehicle can anticipate the future consequences of its actions on the environment and optimize its trajectory accordingly. We thoroughly test our approach in simulation, showing that the autonomous vehicle can adapt its behavior to different situations. We also compare against other methods, demonstrating an improvement with respect to the considered performance metrics.
The use of neural networks and reinforcement learning has become increasingly popular in autonomous vehicle control. However, the opaqueness of the resulting control policies presents a significant barrier to deploying neural network-based control in autonomous vehicles. In this paper, we present a reinforcement learning based approach to autonomous vehicle longitudinal control, where the rule-based safety cages provide enhanced safety for the vehicle as well as weak supervision to the reinforcement learning agent. By guiding the agent to meaningful states and actions, this weak supervision improves the convergence during training and enhances the safety of the final trained policy. This rule-based supervisory controller has the further advantage of being fully interpretable, thereby enabling traditional validation and verification approaches to ensure the safety of the vehicle. We compare models with and without safety cages, as well as models with optimal and constrained model parameters, and show that the weak supervision consistently improves the safety of exploration, speed of convergence, and model performance. Additionally, we show that when the model parameters are constrained or sub-optimal, the safety cages can enable a model to learn a safe driving policy even when the model could not be trained to drive through reinforcement learning alone.
Automated vehicles are increasingly getting main-streamed and this has pushed development of systems for autonomous manoeuvring (e.g., lane-change, merge, and overtake) to the forefront. A novel framework for situational awareness and trajectory planning to perform autonomous overtaking in high-speed structured environments (e.g., highway and motorway) is presented in this paper. A combination of a potential field like function and reachability sets of a vehicle are used to identify safe zones on a road that the vehicle can navigate towards. These safe zones are provided to a tube-based robust model predictive controller as reference to generate feasible trajectories for combined lateral and longitudinal motion of a vehicle. The strengths of the proposed framework are: 1) it is free from non-convex collision avoidance constraints; 2) it ensures feasibility of trajectory even if decelerating or accelerating while performing lateral motion; and 3) it is real-time implementable. The ability of the proposed framework to plan feasible trajectories for high-speed overtaking is validated in a high-fidelity IPG CarMaker and Simulink co-simulation environment.
Designing a controller for autonomous vehicles capable of providing adequate performance in all driving scenarios is challenging due to the highly complex environment and inability to test the system in the wide variety of scenarios which it may encounter after deployment. However, deep learning methods have shown great promise in not only providing excellent performance for complex and non-linear control problems, but also in generalising previously learned rules to new scenarios. For these reasons, the use of deep learning for vehicle control is becoming increasingly popular. Although important advancements have been achieved in this field, these works have not been fully summarised. This paper surveys a wide range of research works reported in the literature which aim to control a vehicle through deep learning methods. Although there exists overlap between control and perception, the focus of this paper is on vehicle control, rather than the wider perception problem which includes tasks such as semantic segmentation and object detection. The paper identifies the strengths and limitations of available deep learning methods through comparative analysis and discusses the research challenges in terms of computation, architecture selection, goal specification, generalisation, verification and validation, as well as safety. Overall, this survey brings timely and topical information to a rapidly evolving field relevant to intelligent transportation systems.
To plan safe maneuvers and act with foresight, autonomous vehicles must be capable of accurately predicting the uncertain future. In the context of autonomous driving, deep neural networks have been successfully applied to learning predictive models of human driving behavior from data. However, the predictions suffer from cascading errors, resulting in large inaccuracies over long time horizons. Furthermore, the learned models are black boxes, and thus it is often unclear how they arrive at their predictions. In contrast, rule-based models-which are informed by human experts-maintain long-term coherence in their predictions and are human-interpretable. However, such models often lack the sufficient expressiveness needed to capture complex real-world dynamics. In this work, we begin to close this gap by embedding the Intelligent Driver Model, a popular hand-crafted driver model, into deep neural networks. Our model's transparency can offer considerable advantages, e.g., in debugging the model and more easily interpreting its predictions. We evaluate our approach on a simulated merging scenario, showing that it yields a robust model that is end-to-end trainable and provides greater transparency at no cost to the model's predictive accuracy.
This paper presents an adaptive energy-aware prediction and planning framework for vehicles navigating over terrains with varying and unknown properties. A novel feature of the method is the use of a deep meta-learning framework to learn a prior energy model, which can efficiently adapt to the local terrain conditions based on small quantities of exteroceptive and proprioceptive data. A meta-adaptive heuristic function is also proposed for the integration of the energy model into an A* path planner. The performance of the proposed approach is assessed in a 3D-body dynamic simulator over several typologies of deformable terrains and compared with alternative machine learning solutions. We provide evidence of the advantages of the proposed method to adapt to unforeseen terrain conditions, thereby yielding more informed estimations and energy-efficient paths when navigating on unknown terrains.
Connected autonomous vehicles are considered as mitigators of issues such as traffic congestion, road safety, inefficient fuel consumption and pollutant emissions that current road transportation system suffers from. Connected autonomous vehicles utilise communication systems to enhance the performance of autonomous vehicles and consequently improve transportation by enabling cooperative functionalities, namely, cooperative sensing and cooperative manoeuvring. The former refers to the ability to share and fuse information gathered from vehicle sensors and road infrastructures to create a better understanding of the surrounding environment while the latter enables groups of vehicles to drive in a co-ordinated way which ultimately results in a safer and more efficient driving environment. However, there is a gap in understanding howand to what extent connectivity can contribute to improving the efficiency, safety and performance of autonomous vehicles. Therefore, the aim of this paper is to investigate the potential benefits that can be achieved from connected autonomous vehicles through analysing five use-cases: (i) vehicle platooning, (ii) lane changing, (iii) intersection management, (iv) energy management and (v) road friction estimation. The current paper highlights that although connectivity can enhance the performance of autonomous vehicles and contribute to the improvement of current transportation system performance, the level of achievable benefits depends on factors such as the penetration rate of connected vehicles, traffic scenarios and the way of augmenting off-board information into vehicle control systems.
Imitation learning has been widely used to learn control policies for autonomous driving based on pre-recorded data. However, imitation learning based policies have been shown to be susceptible to compounding errors when encountering states outside of the training distribution. Further, these agents have been demonstrated to be easily exploitable by adversarial road users aiming to create collisions. To overcome these shortcomings, we introduce Adversarial Mixture Density Networks (AMDN), which learns two distributions from separate datasets. The first is a distribution of safe actions learned from a dataset of naturalistic human driving. The second is a distribution representing unsafe actions likely to lead to collision, learned from a dataset of collisions. During training, we leverage these two distributions to provide an additional loss based on the similarity of the two distributions. By penalising the safe action distribution based on its similarity to the unsafe action distribution when training on the collision dataset, a more robust and safe control policy is obtained. We demonstrate the proposed AMDN approach in a vehicle following use-case, and evaluate under naturalistic and adversarial testing environments. We show that despite its simplicity, AMDN provides significant benefits for the safety of the learned control policy, when compared to pure imitation learning or standard mixture density network approaches.
Shimmy vibration is one of the major concerns in the aircraft landing gear design. In this paper, the influence of structural parameters on the shimmy dynamics is analyzed based on a nonlinear dynamical model. A computationally efficient robust model predictive control law is formulated for a linear parameter varying system with guaranteed closed-loop stability. Moreover, an attempt is made to apply the proposed robust model predictive control strategy to suppress the shimmy during the taxiing and landing of an aircraft. Compared with two current robust model predictive controls, the proposed shimmy controller can effectively suppress the shimmy with more efficient computation. To verify the efficiency of the proposed algorithm, the simulation results are presented and discussed. Copyright © 2008 by the American Institute of Aeronautics and Astronautics, Inc. All rights reserved.
For an autonomous vehicle to operate safely and effectively, an accurate and robust localisation system is essential. While there are a variety of vehicle localisation techniques in literature, there is a lack of effort in comparing these techniques and identifying their potentials and limitations for autonomous vehicle applications. Hence, this paper evaluates the state-of-the-art vehicle localisation techniques and investigates their applicability on autonomous vehicles. The analysis starts with discussing the techniques which merely use the information obtained from on-board vehicle sensors. It is shown that although some techniques can achieve the accuracy required for autonomous driving but suffer from the high cost of the sensors and also sensor performance limitations in different driving scenarios (e.g. cornering, intersections) and different environmental conditions (e.g. darkness, snow). The paper continues the analysis with considering the techniques which benefit from off-board information obtained from V2X communication channels, in addition to vehicle sensory information. The analysis shows that augmenting off-board information to sensory information has potential to design low-cost localisation systems with high accuracy and robustness however their performance depends on penetration rate of nearby connected vehicles or infrastructure and the quality of network service.
This paper proposes a novel unified structure to estimate tire forces. The proposed structure uses estimation modules to calculate/estimate tire forces by means of nonlinear observers. The novelty in the proposed approach lies in the independence of the estimates from the vehicle tire model, thereby making the structure robust against variations in vehicle mass, tire parameters due to tire wear, and, most importantly, road surface conditions. In the proposed structure, we have a dedicated module to estimate the longitudinal tire forces and another to calculate the vertical tire forces. Subsequently, these forces are fed into a third module that utilizes a nonlinear observer to estimate lateral tire forces. The proposed structure is validated through experimental studies.
Trajectory planning and trajectory tracking constitute two important functions of an autonomous overtaking system and a variety of strategies have been proposed in the literature for both functionalities. However, uncertainties in environment perception using the current generation of sensors has resulted in most proposed methods being applicable only during low-speed overtaking. In this paper, trajectory planning and trajectory tracking approaches for autonomous overtaking systems are reviewed. The trajectory planning techniques are compared based on aspects such as real-time implementation, computational requirements, and feasibility in real-world scenarios. This review shows that two important aspects of trajectory planning for high-speed overtaking are: (i) inclusion of vehicle dynamics and environmental constraints and (ii) accurate knowledge of the environment and surrounding obstacles. The review of trajectory tracking controllers for high-speed driving is based on different categories of control algorithms where their respective advantages and disadvantages are analysed. This study shows that while advanced control methods improve tracking performance, in most cases the results are valid only within well-regulated conditions. Therefore, existing autonomous overtaking solutions assume precise knowledge of surrounding environment which is not representative of real-world driving. The paper also discusses how in a connected driving environment, vehicles can access additional information that can expand their perception. Hence, the potential of cooperative information sharing for aiding autonomous high-speed overtaking manoeuvre is identified as a possible solution.
With the advent of electric vehicles with multiple motors, the steady-state and transient cornering responses can be designed based on high-level reference targets, and implemented through the continuous torque control of the individual wheels, i.e., torque-vectoring or direct yaw moment control. The literature includes several papers describing the application of the sliding mode control theory to torque-vectoring. However, the experimental implementations of sliding mode controllers on real vehicle prototypes are very limited at the moment. More importantly, to the knowledge of the authors, there is lack of experimental assessments of the performance benefits of direct yaw moment control based on sliding modes, with respect to other controllers, such as the proportional integral derivative controllers or linear quadratic regulators currently used for stability control in production vehicles. This paper aims to reduce this gap by presenting an integral sliding mode controller for concurrent yaw rate and sideslip control. A new driving mode, the Enhanced Sport mode, is proposed, inducing sustained high values of sideslip angle, which can be safely limited to a specified threshold. The system is experimentally assessed on a four-wheel-drive electric vehicle along a wide range of maneuvers. The performance of the integral sliding mode controller is compared with that of a linear quadratic regulator during step steer tests. The results show that the integral sliding mode controller brings a significant enhancement of the tracking performance and yaw damping with respect to the more conventional linear quadratic regulator based on an augmented single-track vehicle model formulation.
Latest advances in road profile sensors make the implementation of pre-emptive suspension control a viable option for production vehicles. From the control side, model predictive control (MPC) in combination with preview is a powerful solution for this application. However, the significant computational load associated with conventional implicit model predictive controllers (i-MPCs) is one of the limiting factors to the widespread industrial adoption of MPC. As an alternative, this paper proposes an explicit model predictive controller (e-MPC) for an active suspension system with preview. The MPC optimization is run offline, and the online controller is reduced to a function evaluation. To overcome the increased memory requirements, the controller uses the recently developed regionless e-MPC approach. The controller was assessed through simulations and experiments on a sport utility vehicle demonstrator with controllable hydraulic suspension actuators. For frequencies
The paper discusses novel computationally efficient torque distribution strategies for electric vehicles with individually controlled drivetrains, aimed at minimizing the overall power losses while providing the required level of wheel torque and yaw moment. Analytical solutions of the torque control allocation problem are derived and effects of load transfers due to moderate driving/braking and cornering conditions are studied and discussed in detail. Influences of different drivetrain characteristics on the front and rear axles are described. The results of the analytically-derived algorithm are contrasted with those from two other control allocation strategies, based on the off-line numerical solution of more detailed formulations of the control allocation problem (i.e., a multi-parametric non-linear programming problem). The solutions of the control allocation problem are experimentally validated along multiple driving cycles and in steady-state cornering, on an electric vehicle with four identical drivetrains. The experiments show that the computationally efficient algorithms represent a very good compromise between low energy consumption and controller complexity.
Direct yaw moment controllers improve vehicle stability and handling in severe manoeuvres. In direct yaw moment control implementations based on Linear Quadratic Regulators (LQRs), the control system performance is limited by the unmodelled dynamics and parameter uncertainties. To guarantee robustness with respect to uncertainties, this paper proposes a gain scheduled Robust Linear Quadratic Regulator (RLQR), in which an extra control term is added to the feedback contribution of a conventional LQR to limit the closed-loop tracking error in a neighbourhood of the origin of its state-space, despite the uncertainties and disturbances acting on the plant. In addition, the intrinsic parameter-varying nature of the vehicle dynamics model with respect to the longitudinal vehicle velocity can compromise the closed-loop performance of fixed-gain controllers in varying driving conditions. Therefore, in this study the control gains optimally vary with velocity to adapt the closed-loop system to the variations of this parameter. The effectiveness of the proposed RLQR in improving the robustness of a classical LQR against model uncertainties and parameter variations is proven analytically, numerically and experimentally. The simulation and vehicle test results are consistent with the formal analysis proving that the RLQR reduces the ultimate bound of the error dynamics.
Electric vehicles (EVs) with four individually controlled drivetrains are over-actuated systems, and therefore, the total wheel torque and yaw moment demands can be realized through an infinite number of feasible wheel torque combinations. Hence, an energy-efficient torque distribution among the four drivetrains is crucial for reducing the drivetrain power losses and extending driving range. In this paper, the optimal torque distribution is formulated as the solution of a parametric optimization problem, depending on the vehicle speed. An analytical solution is provided for the case of equal drivetrains, under the experimentally confirmed hypothesis that the drivetrain power losses are strictly monotonically increasing with the torque demand. The easily implementable and computationally fast wheel torque distribution algorithm is validated by simulations and experiments on an EV demonstrator, along driving cycles and cornering maneuvers. The results show considerable energy savings compared to alternative torque distribution strategies.
Deep learning is a promising class of techniques for controlling an autonomous vehicle. However, functional safety validation is seen as a critical issue for these systems due to the lack of transparency in deep neural networks and the safety-critical nature of autonomous vehicles. The black box nature of deep neural networks limits the effectiveness of traditional verification and validation methods. In this paper, we propose two software safety cages, which aim to limit the control action of the neural network to a safe operational envelope. The safety cages impose limits on the control action during critical scenarios, which if breached, change the control action to a more conservative value. This has the benefit that the behaviour of the safety cages is interpretable, and therefore traditional functional safety validation techniques can be applied. The work here presents a deep neural network trained for longitudinal vehicle control, with safety cages designed to prevent forward collisions. Simulated testing in critical scenarios shows the effectiveness of the safety cages in preventing forward collisions whilst under normal highway driving unnecessary interruptions are eliminated, and the deep learning control policy is able to perform unhindered. Interventions by the safety cages are also used to re-train the network, resulting in a more robust control policy.
This paper proposes a distributed control strategy for homogeneous platoon systems with external disturbances under random packet drop scenario which can occur due to underlying network among the vehicles in a platoon. An linear matrix inequality (LMI) based approach is used to obtain the controller gains for ensuring the stability with bounded H∞ norm for such systems. Effectiveness of the proposed method is demonstrated with numerical results considering different network topologies in a platoon under single packet drop. The variation of H∞ norm bound for different number of platoon members under the different structure of network topologies and the packet drop has been studied in this paper.
This paper proposes a discrete time distributed state feedback controller design strategy for a homogenous vehicle platoon system with undirected network topology which is resilient to both external disturbances and random consecutive network packet drop. The system incorporates a distributed state feedback controller design by satisfying bounded H-infinity norm using Lyapunov-Krasovskii based linear matrix inequality (LMI) approach that ensures internal stability and performance. The effect of packet drops on internal stability in terms of stability margin are studied for a homogenous vehicle platoon system with undirected network topology and external disturbance. The variation of stability margin, representing absolute value of least stable close-loop pole, is also studied for two common undirected network topologies for vehicle platooning, i.e., bidirectional predecessor following (BPF) and bidirectional predecessor leader following (BPLF) topologies by varying platoon members, packet drop rates with number of contiguous packets dropped. Results demonstrate that the control strategy best satisfies the requirement of maintaining a desired inter-vehicular distance with constant spacing policy and leader trajectory using two network topologies: BPF and BPLF. We show how these topologies are robust in terms of ensuring internal stability and performance to maintain cooperative motion of vehicle platoon system with different number of followers, random multiple consecutive packet drops and external disturbance.
Reinforcement learning has been used widely for autonomous longitudinal control algorithms. However, many existing algorithms suffer from sample inefficiency in reinforcement learning as well as the jerky driving behaviour of the learned systems. In this paper, we propose a reinforcement learning algorithm and a training framework to address these two disadvantages of previous algorithms proposed in this field. The proposed system uses an Advantage Actor Critic (A2C) learning system with recurrent layers to introduce temporal context within the network. This allows the learned system to evaluate continuous control actions based on previous states and actions in addition to current states. Moreover, slow training of the algorithm caused by its sample inefficiency is addressed by utilising another neural network to approximate the vehicle dynamics. Using a neural network as a proxy for the simulator has significant benefit to training as it reduces the requirement for reinforcement learning to query the simulation (which is a major bottleneck) in learning and as both reinforcement learning network and proxy network can be deployed on the same GPU, learning speed is considerably improved. Simulation results from testing in IPG CarMaker show the effectiveness of our recurrent A2C algorithm, compared to an A2C without recurrent layers.
Quantifying and encoding occupants’ preferences as an objective function for the tactical decision making of autonomous vehicles is a challenging task. This paper presents a low-complexity approach for lane-change initiation and planning to facilitate highly automated driving on freeways. Conditions under which human drivers find different manoeuvres desirable are learned from naturalistic driving data, eliminating the need for an engineered objective function and incorporation of expert knowledge in form of rules. Motion planning is formulated as a finite-horizon optimisation problem with safety constraints. It is shown that the decision model can replicate human drivers’ discretionary lane-change decisions with up to 92% accuracy. Further proof of concept simulation of an overtaking manoeuvre is shown, whereby the actions of the simulated vehicle are logged while the dynamic environment evolves as per ground truth data recordings.
—This paper presents the design of a robust distributed state-feedback controller in the discrete-time domain for homogeneous vehicle platoons with undirected topologies, whose dynamics are subjected to external disturbances and under random single packet drop scenario. A linear matrix inequality (LMI) approach is used for devising the control gains such that a bounded H∞ norm is guaranteed. Furthermore, a lower bound of the robustness measure, denoted as γ gain, is derived analytically for two platoon communication topologies, i.e., the bidirectional predecessor following (BPF) and the bidirectional predecessor leader following (BPLF). It is shown that the γ gain is highly affected by the communication topology and drastically reduces when the information of the leader is sent to all followers. Finally, numerical results demonstrate the ability of the proposed methodology to impose the platoon control objective for the BPF and BPLF topology under random single packet drop. Index Terms—vehicle platoon, LMI, distributed H∞ control with packet drops, robustness of closed-loop system.
Devising planning algorithms for autonomous driving is non-trivial due to the presence of complex and uncertain interaction dynamics between road users. In this paper, we introduce a planning framework encompassing multiple action policies that are learned jointly from episodes of human-human interactions in naturalistic driving. The policy model is composed of encoder-decoder recurrent neural networks for modeling the sequential nature of interactions and mixture density networks for characterizing the probability distributions over driver actions. The model is used to simultaneously generate a finite set of context-dependent candidate plans for an autonomous car and to anticipate the probable future plans of human drivers. This is followed by an evaluation stage to select the plan with the highest expected utility for execution. Our approach leverages rapid sampling of action distributions in parallel on a graphic processing unit, offering fast computation even when modeling the interactions among multiple vehicles and over several time steps. We present ablation experiments and comparison with two existing baseline methods to highlight several design choices that we found to be essential to our model's success. We test the proposed planning approach in a simulated highway driving environment, showing that by using the model, the autonomous car can plan actions that mimic the interactive behavior of humans.
3D object detection is a common function within the perception system of an autonomous vehicle and outputs a list of 3D bounding boxes around objects of interest. Various 3D object detection methods have relied on fusion of different sensor modalities to overcome limitations of individual sensors. However, occlusion, limited field-of-view and low-point density of the sensor data cannot be reliably and cost-effectively addressed by multi-modal sensing from a single point of view. Alternatively, cooperative perception incorporates information from spatially diverse sensors distributed around the environment as a way to mitigate these limitations. This article proposes two schemes for cooperative 3D object detection using single modality sensors. The early fusion scheme combines point clouds from multiple spatially diverse sensing points of view before detection. In contrast, the late fusion scheme fuses the independently detected bounding boxes from multiple spatially diverse sensors. We evaluate the performance of both schemes, and their hybrid combination, using a synthetic cooperative dataset created in two complex driving scenarios, a T-junction and a roundabout. The evaluation shows that the early fusion approach outperforms late fusion by a significant margin at the cost of higher communication bandwidth. The results demonstrate that cooperative perception can recall more than 95% of the objects as opposed to 30% for single-point sensing in the most challenging scenario. To provide practical insights into the deployment of such system, we report how the number of sensors and their configuration impact the detection performance of the system.
Vehicle platooning is a promising cooperative driving vision to mitigate issues in road transportation and it leverages on the use of distributed control systems to precisely control each vehicle in the fleet. This paper investigates a fully adaptive platoon control solution made possible by the advent of more reliable communication channels and off-board cloud computing systems. The components of the platoon control system, i.e., the platoon network topology and the distributed controllers, adapt based on the relative motion of the vehicles in the fleet, thus allowing the platoon to self-organise the interactions among the platoon members and the control gains to create and maintain the cooperative platoon motion. Furthermore, the adaptive mechanism to adjust the control gains is equipped with a σ-modification strategy to keep the control gains bounded in any working condition. Numerical results show the effectiveness of control method to make a heterogeneous platoon a self-organising network of intelligent vehicles, while also reducing the number of the communication links required to establish the cooperative motion.
This paper presents a functional architecture for controlling and managing platoons of vehicles assisted by a cloud computing platform for passenger vehicles in mixed traffic scenarios. The architecture modules are distributed in the three separate logical layers suggested by the on-going CARMA (Cloud-Assisted Real-time Methods for Autonomy) research project which aims to develop and test cooperative automated driving technologies and distributed control systems. The architecture also exploits the potential of the CARMA platform both in terms of computing and data sharing.
Tire forces are at the heart of the dynamic qualities of vehicles. With the advent of electric vehicles the precise and accurate control of the traction and braking forces at the individual wheel becomes a possibility and a reality outside test labs and virtual proving grounds. Benefits of individual wheel torque control, or torque-vectoring, in terms of vehicle dynamics behavior have been well documented in the literature. However, very few studies exist which analyze the individual wheel torque control integrated with vehicle efficiency considerations. This paper focuses on this aspect and discusses the possibilities and benefits of integrated, energy efficient torque vectoring control. Experiments with a four-wheel-drive electric vehicle show that considerable energy savings can be achieved by considering drivetrain and tire power losses through energy efficient torque vectoring control.
Model predictive control (MPC) is increasingly finding its way into industrial applications, due to its superior tracking performance and ability to formally handle system constraints. However, the real-time capability problems related to the conventional implicit model predictive control (i-MPC) framework are well known, especially when targeting low-cost electronic control units (ECUs) for high bandwidth systems, such as automotive active suspensions, which are the topic of this paper. In this context, to overcome the real-time implementation issues of i-MPC, this study proposes explicit model predictive control (e-MPC), which solves the optimization problem off-line, via multi-parametric quadratic programming (mp-QP). e-MPC reduces the on-line algorithm to a function evaluation, which replaces the computationally demanding on-line solution of the quadratic programming (QP) problem. An e-MPC based suspension controller is designed and experimentally validated for a case study Sport Utility Vehicle (SUV), equipped with the active ACOCAR suspension system from the Tenneco Monroe product family. The target is to improve ride comfort in the frequency range of primary ride (< 4 Hz), without affecting the performance at higher frequencies. The proposed e-MPC implementations reduce the root mean square (RMS) value of the sprung mass acceleration by > 40% compared to the passive vehicle set-up for frequencies < 4 Hz, and by up to 19% compared to the same vehicle with a skyhook controller on the 0-100 Hz frequency range.
© IFAC.Using Lyapunov theory, Pontryagin's minimum principle, and affine quadratic stability, a novel robust optimal control strategy is developed for active suspension systems to enhance vehicle ride comfort and handling performance. The controller has a simple structure, making its suitable for real-time implementation. The required sensor configuration includes a six-axis IMU and four LVDTs. The proposed controller is suitable for on-road commercial vehicles where ride comfort over bump disturbances and handling performance are the most concerns. The effectiveness of the controller is verified through simulation results using IPG CarMaker software.
The benefits of individual wheel torque control, or torque vectoring, in terms of vehicle dynamics behaviour have been well documented in the literature. However, few studies analyse individual wheel torque control integrated with electric vehicle efficiency considerations. The possibilities and benefits of energy efficient torque vectoring control for electric vehicles with multiple drivetrains are discussed. In particular, energy consumption reductions can be obtained through specific design of the wheel torque control allocation algorithm and the reference yaw rate characteristics. Experiments with a four-wheel-drive electric vehicle demonstrate considerable energy savings.
Control of vehicle suspension systems has been the focus of extensive work in the past two decades. Many control strategies have been developed to improve the overall vehicle performance, including both ride quality and stability conditions. However, the concerns regarding the wheel motions affecting significantly on the handling and steering are ignored by researchers in the control formulation. A H8 control methodology is employed to design an active suspension system so that ride quality and wheel motions are improved simultaneously. In addition, a three-dimensional kinematic model of a specific suspension system, namely Macpherson suspension, is developed to study the alteration of those of kinematic suspension parameters which represent the wheel motions. The results show that the proposed robust design provides superior kinematic and dynamic performances compared to those of the passive system. © 2009 AACC.
CARMA (Cloud-Assisted Real-time Methods for Autonomy) is a highly innovative and challenging project which aims to develop and test cooperative automated driving technology, based on a distributed control system. The approach is enabled by an ultra-low latency and highly reliable cloud-based infrastructure accessed through 5G. This paper describes the 3-tier distributed computing architecture used in the project comprising the Vehicle, the Edge cloud and the Core. It describes a methodology to test a set of use cases representative of both urban and highway driving and explores the key challenges in such an approach. The first technical challenge is the design of a mobile edge cloud infrastructure that is able to support real-time and safety critical applications. Another non-trivial problem is that of cyber-security for such a real-time cyber physical system. Progress during the first year of this five year project is described.
Astrophysicists demand larger (mirror diameter > 10m) space optical telescopes to investigate more distant events that happened during the very early period of the universe, for example formations of the earliest stars. The deployable telescope design like James Webb Space Telescope that has a 6.5m diameter primary mirror has already reached the capacity limits of the existing launch vehicles. Therefore, the space industry has been considering using robotic technologies to build future optical reflecting three-mirror structured space telescopes in orbit from smaller components. One of the design paradigms is to use a high-DOF manipulator on a free-flying platform to build the optical telescope in orbit. This approach requires high precision and accuracy in the robotic manipulation GNC system that has several challenges yet to be addressed: 1. Orbital environmental parameters that affect sensing and perception; 2. Limitations in robotic hardware, trajectory planning algorithms and controllers. To investigate these problems for in-orbit manipulation, the UK national hub on future AI and robotics for space (FAIR-SPACE) at the Surrey Space Centre (SSC) has been developing a ground-based hardware-in-the-loop (HIL) robotic demonstrator to simulate in-orbit manipulation. The key elements of the demonstrator are two 6-DOF manipulators and a re-configurable sensor system. One of the manipulators with a > 3-DOF gripping mechanism represents the assembly manipulator on a spacecraft whose orbital dynamics, kinematics, and environmental disturbances and uncertainties are propagated in a computer. The other 6-DOF manipulator with a torque/force sensor is used as a gravity offoad mechanism to carry the space telescope mirror segment. The relative motions between the service/manipulation arm and the mirror segment are computed and then executed by the second arm. The sensor system provides visual feedback of the end-effector and uses computer vision and AI to estimate the pose and position of the mirror segment respectively. The demonstrator aims to verify and validate the manipulator assembly approach for future large space optical telescopes against ground truth and benchmarks. This paper explains the motivation behind developing this testbed and introduces the current hardware setup of the testbed and its key features.
There is significant demand for an understanding of the fundamentals, technologies, and design of electric and hybrid electric vehicles and their components from researchers, engineers, and graduate students. Although there is a good body of work in the literature, there is still a great need for electric and hybrid vehicle teaching materials. Electric and Hybrid Vehicles: Technologies, Modeling and Control A Mechatronic Approach is based on the authors current research in vehicle systems and will include chapters on vehicle propulsion systems, the fundamentals of vehicle dynamics, EV and HEV technologies, chassis systems, steering control systems, and state, parameter and force estimations. The book is highly illustrated, and examples will be given throughout the book based on real applications and challenges in the automotive industry. Designed to help a new generation of engineers needing to master the principles of and further advances in hybrid vehicle technology Includes examples of real applications and challenges in the automotive industry with problems and solutions Takes a mechatronics approach to the study of electric and hybrid electric vehicles, appealing to mechanical and electrical engineering interests Responds to the increase in demand of universities offering courses in newer electric vehicle technologies
In this paper, a new nonlinear model of Macpherson strut suspension system for ride Control applications is proposed. The model includes the vertical acceleration of the sprung mass and the motions of the unsprung mass subjected to control arm rotation. In addition, it considers physical characteristics of the spindle such as mass and inertia moment. This two degree-of-freedom (DOF) model not only provides a more accurate representation of the Macpherson suspension system for ride control applications but also facilitates evaluation of the kinematic parameters such as camber, caster and king-pin angles as well as track alterations on the ride vibrations. The performances of the nonlinear and linear models are investigated and compared. Simulation results are presented and discussed. ©2008 AACC.
This paper proposes an integrated trajectory planning based on Model Predictive Control (MPC) for designing collision-free evasive trajectory and a torque vectoring controller based on optimal control to ensure lateral-yaw stabilization in autonomous emergency collision avoidance under low friction and crosswinds on highways. The trajectory for performing the evasive manoeuvre is designed to minimise the deviation of the vehicle from the lane center while ensuring the vehicle remains within the road boundaries. The steering input computed from the MPC is used to steer the vehicle along the reference trajectory while the torque vectoring controller provides additional lateral-yaw stability. The integrated control framework was implemented on IPG Carmaker-MATLAB co-simulation platform and its efficacy was evaluated under different scenarios. Simulations performed for emergency collision avoidance at high speeds with low road friction and heavy crosswinds confirm the ability of the proposed closed-loop framework at successfully avoiding collisions with moving obstacles while ensuring that the controlled vehicle remains within its limits of stability. Furthermore, the robustness of the proposed control framework to variations in road friction changes is demonstrated by simulating an evasive manoeuvre at high-speeds for wide range of road friction conditions. Comparing the performance of the proposed control framework to a vehicle without the corrective actions available via torque vectoring highlight the additional benefits provided by the proposed closed-loop scheme at ensuring lateral-yaw stability under emergency scenarios.
In this paper, kinematics and dynamics of the MacPherson suspension are studied. Displacement Matrix Method is utilized for this purpose. Camber and toe angle alterations are derived by means of the rotation matrix. Kinematical characteristics of the MacPherson suspension are displayed as functions of time and the wheel vertical displacement. Relations for velocities and accelerations of key points are also obtained. Since internal forces and external forces are important in stress analysis and for comfortability, these forces are calculated as functions of time as well. Subsequently, a general analysis of the mechanism under the wheel sinusoidal displacement is presented together with relevant figures and results. © Shiraz University.
In this paper, the driver ride comfort in a heavy vehicle (city bus) is studied under the sky-hook semi-active damping force policy. A new hybrid dynamic model composed of a continuous system and a discrete system are integrated in the current work. The chassis of the vehicle is assumed as the continuous beam supported on the discrete suspension springs and dampers. The driver and the seat are also considered as a discrete vibrating system. The dynamic equations are solved by using the assumed mode method, where the mode shapes of a free-free beam have been employed. The results of the semi-active system are compared with those of the passive one through simulations. The results indicate that the new hybrid dynamic model represents more degrees-of-freedom of the system for driver ride analysis compared to the discrete model. In addition, the results show that the semi-active system has a superior performance in terms of the ride comfort. Copyright © 2010 SAE International.
Suspension kinematic parameters such as camber, caster and king-pin angles as well as track width are important in improving handling performance and stability of a vehicle. Using a new model of the Macpherson suspension system, the effects of different hybrid semi-active control strategies on the performance of suspension kinematic parameters and on improvement of ride quality are investigated. The control strategies considered in this work comprise hybrid skyhook-groundhook, modified skyhook and, passive-skyhook controllers. It is shown that although contribution of these controllers on the improvement of ride quality of the vehicle is similar, they affect the performance of the Macpherson suspension kinematic parameters significantly different. Simulation results are presented and discussed. Copyright © 2008 SAE International.
Deep learning is a promising class of techniques for controlling an autonomous vehicle. However, functional safety validation is seen as a critical issue for these systems due to the lack of transparency in deep neural networks and the safety-critical nature of autonomous vehicles. The black box nature of deep neural networks limits the effectiveness of traditional verification and validation methods. In this paper, we propose two software safety cages, which aim to limit the control action of the neural network to a safe operational envelope. The safety cages impose limits on the control action during critical scenarios, which if breached, change the control action to a more conservative value. This has the benefit that the behaviour of the safety cages is interpretable, and therefore traditional functional safety validation techniques can be applied. The work here presents a deep neural network trained for longitudinal vehicle control, with safety cages designed to prevent forward collisions. Simulated testing in critical scenarios shows the effectiveness of the safety cages in preventing forward collisions whilst under normal highway driving unnecessary interruptions are eliminated, and the deep learning control policy is able to perform unhindered. Interventions by the safety cages are also used to re-train the network, resulting in a more robust control policy.
Automated vehicles are increasingly getting mainstreamed and this has pushed development of systems for autonomous manoeuvring (e.g., lane-change, merge, overtake, etc.) to the forefront. A novel framework for situational awareness and trajectory planning to perform autonomous overtaking in high-speed structured environments (e.g., highway, motorway) is presented in this paper. A combination of a potential field like function and reachability sets of a vehicle are used to identify safe zones on a road that the vehicle can navigate towards. These safe zones are provided to a tube-based robust model predictive controller as reference to generate feasible trajectories for combined lateral and longitudinal motion of a vehicle. The strengths of the proposed framework are: (i) it is free from nonconvex collision avoidance constraints, (ii) it ensures feasibility of trajectory even if decelerating or accelerating while performing lateral motion, and (iii) it is real-time implementable. The ability of the proposed framework to plan feasible trajectories for highspeed overtaking is validated in a high-fidelity IPG CarMaker and Simulink co-simulation environment.
With self-driving vehicles being pushed towards the main-stream, there is an increasing motivation towards development of systems that autonomously perform manoeuvres involving combined lateral-longitudinal motion (e.g., lanechange, merge, overtake, etc.). This paper presents a situational awareness and trajectory planning framework for performing autonomous overtaking manoeuvres. A combination of a potential field-like function and reachability sets of a vehicle are used to identify safe zones on a road that the vehicle can navigate towards. These safe zones are provided to a model predictive controller as reference to generate feasible trajectories for a vehicle. The strengths of the proposed framework are: (i) it is free from non-convex collision avoidance constraints, (ii) it ensures feasibility of trajectory, and (iii) it is real-time implementable. A proof of concept simulation is shown to demonstrate the ability to plan trajectories for high-speed overtaking manoeuvres.
The main focus of the present paper is on the design of a modified sky-hook control of a semi-active Macpherson suspension system by means of H∞ Output Feedback Control (OFC) theory. To this end, a new dynamic model, incorporating the kinematics of the suspension system, is used for the controller design. The combination of a Linear Matrix Inequality (LMI) solver and Genetic Algorithm (GA) is adopted to regulate the static output feedback control gain so that the stability conditions are fulfilled and control objectives are achieved. Meanwhile, a three-dimensional kinematic model of the system is incorporated to investigate the influence of the control force variation on the steering, handling and stability of the vehicle. A geometric relation of the vehicle roll center is employed to study one more extra aspect of the comfort and stability of the vehicle. The results show that the proposed controller improves the kinematic and dynamic performances of the suspension well compared with those of the passive system. Moreover, it is concluded that a superior stability of the vehicle during the cornering can be achieved by adjusting the height of the vehicle roll center passively so that the stability of the vehicle is improved while the forward motion specifications can be modified by an appropriate suspension control design. Copyright © 2009 by ASME.