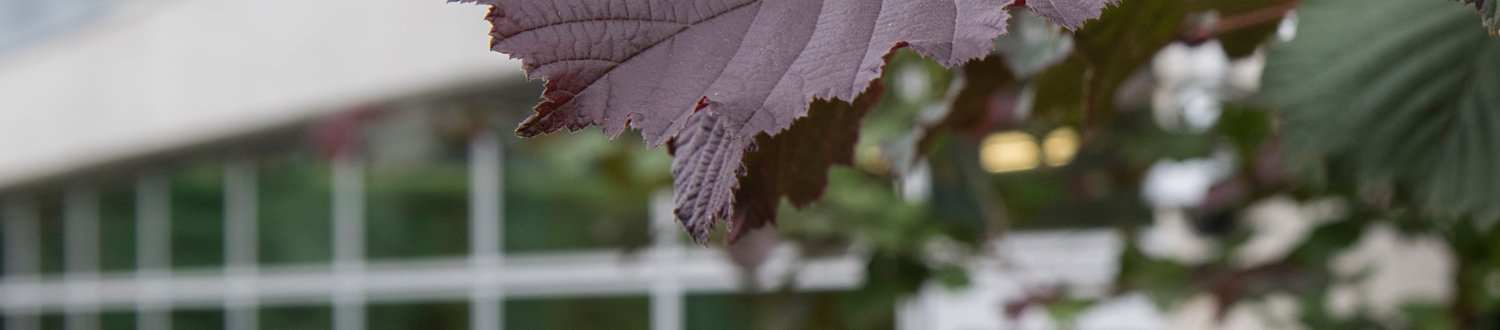
About the project
A quantitative approach to Development
The Genetics of Cell Differentiation – The Epigenetic Landscape
The complex genetic regulation that drives the differentiation process can be represented in terms of the so-called epigenetic landscape. The concept of epigenetic landscape was first introduced by C.H. Waddington in 1957 as a metaphor for the process by which a multipotent cell progresses to its eventual fate. Waddington proposed that the differentiating cell is represented by a ball rolling down the valleys of the landscape. During differentiation the cell encounters successive bifurcation points and makes successive transitions towards the end points of each valley. In Waddington's picture, these end points represent the stably differentiated cell types.
Deterministic Mathematical Modelling
The epigegetic landscape is a manifestation of the underlying gene regulatory network that supervises the differentiation process. This gene regulatory network can be modelled mathematically by using a set of ordinary differential equations, which describe deterministically the dynamics of the concentrations of the transcript and protein levels at any given time. A dynamical systems analysis can then be performed to determine the steady states of the systems and their stability properties. The stable states, also known as attractor states, correspond to the bottom end points of the valleys of the Waddington landscape, and are a mathematical representation of the fully differentiated cell types.
Modelling Stochastic Fluctuations
The deterministic nature of ordinary differential equations models does not in general capture the dynamical role that stochastic fluctuations may play in the differentiation process.
However, stochastic fluctuations, or noise, are ubiquitous in molecular biology. They occur in many aspects of gene regulation and of other cellular activities, and account for much of the variability that we see in all biological systems. When fluctuations originate outside of the gene regulatory network, for instance because of heterogeneities present in the cellular microenvironment, they are usually classified as extrinsic noise. In contrast, fluctuations due to the inherent randomness of the molecular processes internal to the network are usually classified as intrinsic noise. Intrinsic noise is particularly relevant at low copy numbers of molecules, and it can then become a predominant source of stochastic behaviour. This is the case for instance in the bursting activity of slowly transcribed genes.
Both intrinsic and extrinsic noise in a gene regulatory network lead to variability in gene expression levels, but in qualitatively and quantitatively different manners. In the Waddington landscape metaphor, intrinsic noise will change a deterministic path into a ‘noisy’ trajectory. As a consequence transitions between different cell types will become possible in principle if the Waddington valleys are very shallow, or if the noise is strong enough during differentiation. Conversely, the effects of extrinsic noise may be much more subtle and counter-intuitive. Extrinsic noise can produce modifications of the morphology of the landscape, by shifting, creating or eliminating Waddington valleys and by changing their stability properties, giving rise to so-called noise-induced transitions. Our work focuses on elucidating the interplay between stochastic variability, of both intrinsic and extrinsic origin, and cellular differentiation.
Our objectives
We aim at shedding new light on the differentiation process by using an integrated experimental and mathematical modelling approach. We use zebrafish (Danio Rerio) as a model system, and focus on two neural crest derivates, namely melanocytes and iridophores. Our studies aim to reconstruct deterministically the gene regulatory network responsible for the differentiation of melanoiridoblasts into melanocytes and iridophores. The mathematical models so obtained are extended so as to incorporate stochastic fluctuations. The amount of variability in the network measured at distinct differentiation stages serves as an input into our models. Our ultimate goal is to assess the dynamical role likely played by noise in assisting the differentiation process.